Comparison of the Spi and Spei on Predicting Drought Conditions and Streamflow in the Canadian Prairies
Total Page:16
File Type:pdf, Size:1020Kb
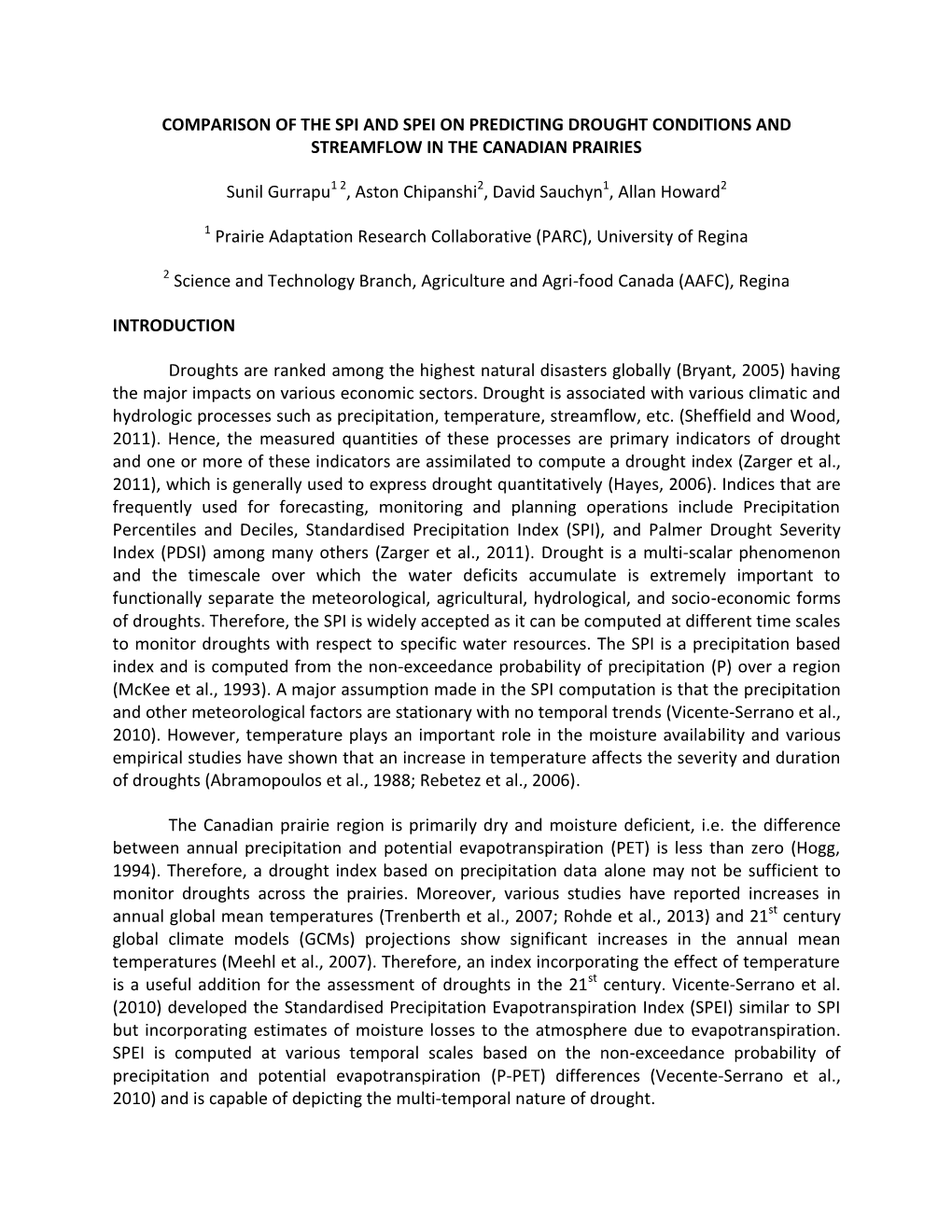
Load more
Recommended publications
-
Drought Planning and Adaptation
Running Head: DROUGHT PLANNING AND ADAPTATION Drought Disaster Planning and Adaptation in Rural British Columbia by Evan Lloyd A Thesis Submitted to the Faculty of Social and Applied Sciences in Partial Fulfillment of the Requirements for the Degree of Master of Arts In Disaster and Emergency Management Royal Roads University Victoria, British Columbia, Canada Supervisor: Professor Ann Dale June, 2017 Evan Lloyd, 2017 DROUGHT PLANNING AND ADAPTATION 2 COMMITTEE APPROVAL The members of Evan Lloyd’s Thesis Committee certify that they have read the thesis titled Drought Disaster Planning and Adaptation in Rural British Columbia and recommend that it be accepted as fulfilling the thesis requirements for the Degree of Master of Arts in Disaster and Emergency Management: Dr. Ross Pink [signature on file] Dr. Julie Drolet [signature on file] Final approval and acceptance of this thesis is contingent upon submission of the final copy of the thesis to Royal Roads University. The thesis supervisor confirms to have read this thesis and recommends that it be accepted as fulfilling the thesis requirements: Dr. Ann Dale [signature on file] DROUGHT PLANNING AND ADAPTATION 3 Creative Commons Statement This work is licensed under the Creative Commons Attribution-NonCommercial- ShareAlike 2.5 Canada License. To view a copy of this license, visit http://creativecommons.org/licenses/by-nc-sa/2.5/ca/. Some material in this work is not being made available under the terms of this licence: • Third-Party material that is being used under fair dealing or with permission. • Any photographs where individuals are easily identifiable. DROUGHT PLANNING AND ADAPTATION 4 Abstract Drought is one of the more devastating climate-driven hazards across the world. -
Assessment of the Influence of Nonstationary Climate on Extreme Hydrology of Southwestern Canada
ASSESSMENT OF THE INFLUENCE OF NONSTATIONARY CLIMATE ON EXTREME HYDROLOGY OF SOUTHWESTERN CANADA A Thesis Submitted to the Faculty of Graduate Studies and Research For the Degree of Special Case Doctor of Philosophy in Geography University of Regina By Sunil Gurrapu Regina, Saskatchewan January 2020 Copyright 2020: S. Gurrapu UNIVERSITY OF REGINA FACULTY OF GRADUATE STUDIES AND RESEARCH SUPERVISORY AND EXAMINING COMMITTEE Sunil Gurrapu, candidate for the degree of Special Case Doctor of Philosophy in Geography, has presented a thesis titled, Assessment of the Influence of Nonstationary Climate on Extreme Hydrology in Southwestern Canada, in an oral examination held on November 7, 2019. The following committee members have found the thesis acceptable in form and content, and that the candidate demonstrated satisfactory knowledge of the subject material. External Examiner: *Dr. Stephen Jacques Dery, University of Northern British Columbia Co-Supervisor: Dr. David Sauchyn, Department of Geography & Environmental Studies Co-Supervisor: *Dr. Kyle Hodder, Department of Geography & Environmental Studies Committee Member: *Dr. Jeannine St. Jacques, Adjunct Committee Member: Dr. Peng Wu, Environmental System Engineering Committee Member: Dr. Ulrike Hardenbicker, Department of Geography & Environmental Studies Chair of Defense: Dr. Fanhua Zeng, Faculty of Graduate Studies & Research *Via ZOOM conferencing ABSTRACT The demand for water supplies across southwestern Canada has increased substantially over the past century with growing population and economic activities. At the same time, the region’s resilience to the impacts of hydrological extremes, floods and droughts is challenged by the increasing frequency of these extreme events. In addition, increased winter temperatures over the past century have led to declines in winter snowpack and caused earlier snowmelt, leading in some years to a substantial shortage of water during late summer and fall seasons. -
Seasonality of the Water Balance of the Sooke Reservoir, BC, Canada
Seasonality of the Water Balance of the Sooke Reservoir, BC, Canada by Arelia Taymen Werner B.Sc., University of British Columbia, 2000 A Thesis Submitted in Partial Fulfillment of the Requirements for the Degree of MASTER OF SCIENCE in the Department of Geography Arelia Taymen Werner, 2007 University of Victoria All rights reserved. This thesis may not be reproduced in whole or in part, by photocopy or other means, without the permission of the author. ii Supervisory Committee _____________________________________________________________________ Dr. Terry D. Prowse, Co-Supervisor (Department of Geography) _____________________________________________________________________ Dr. John J. Gibson, Co-Supervisor (Department of Geography) _____________________________________________________________________ Dr. Dan L. Peters, Departmental Member (Department of Geography) _____________________________________________________________________ Dr. Barrie R. Bonsal, Departmental Member (Department of Geography) Examining Committee All members of the Supervisory Committee and _____________________________________________________________________ Dr. Garth van der Kamp, External Examiner (University of Saskatchewan) iii Supervisory Committee Dr. Terry D. Prowse, Co-Supervisor (Department of Geography) Dr. John J. Gibson, Co-Supervisor (Department of Geography) Dr. Dan L. Peters, Departmental Member (Department of Geography) Dr. Barrie R. Bonsal, Departmental Member (Department of Geography) Abstract Climate change and population growth is putting -
Drought Preparedness
-- CHECK AGAINST DELIVERY -- Statement by the Delegation of Canada to the Sixteenth Session of the United Nations Commission on Sustainable Development Wednesday, May 7 2008, 10:00 am – Drought Preparedness Mr/Madame Chairperson, Canada experienced serious land degradation and severe drought in the 1930s. In response, Canada developed soil and water conservation initiatives and converted fragile lands into grassland to inhibit land degradation. Despite these efforts drought can still cause loss of income and pose adaptation challenges. This was seen in the 2001 - 2002 drought, which affected regions not normally affected by drought such as parts of Eastern Canada and northern areas of Canada’s prairies. This drought cost an estimated $6 billion, in total, to the agriculture, forestry, petroleum and mining, hydro power, and water supply sectors. Climate change experts are anticipating greater variability and shifts in timing of available water supplies in the future, with a predicted increase in the frequency and duration of droughts. Because droughts are expensive, efforts are being applied to deal with drought proactively as a part of a comprehensive Canadian approach. Canada has established a range of drought identification activities. These activities require partnerships across jurisdictions and sectors. One example of drought monitoring and reporting is ‘Drought Watch’. This is a national program that provides timely information about climatic impacts on water supply and agriculture. Drought Watch products combine physical information, such as precipitation and soil moisture, with historic climate information to assess the status of drought in Canada’s agricultural regions. During each growing season, the Climate Production Risk Committee uses Drought Watch maps and regional information supplied by representatives from provinces and other government departments to assess drought impacts on soil conservation, crop and livestock management, and range, pest and water supply management. -
“A Multi-Millennia Perspective on Drought and Implications for the Future"
CLIVAR/PAGES/IPCC Workshop “A multi-millennia perspective on drought and implications for the future" November 18-21, 2003 Tucson, AZ Organized by: Jonathan Overpeck, University of Arizona Kevin Trenberth, National Center For Atmospheric Research Sponsored by: US National Science Foundation US National Oceanographic and Atmospheric Administration Intergovernmental Panel on Climate Change For full background materials, venue, agenda, speakers and participants see: http://ipcc-wg1.ucar.edu/meeting/wg1/Drght/ also: Trenberth, K., J. Overpeck and S. Solomon, 2004: Exploring drought and its implications for the future. Eos, 85, No. 3, 20 Jan. 2004, p27. 1 Table of Contents 1. Organizational Acronyms Used..............................................................................3 2. Introduction ...........................................................................................................3 3. Initial Plenary Talks and Discussion.......................................................................4 3.1. Opening session.......................................................................................4 3.2. Instrumental and paleoclimatic records of drought...................................6 3.3. Drought processes and modeling .............................................................8 4. Breakout Working Groups ...................................................................................10 4.1. Introduction...........................................................................................10 4.2. Summary -
Canadian Drought Monitor Conditions As of March 31, 2014
Canadian Drought Monitor Conditions as of March 31, 2014 The frigid Canadian winter continued into March, with monthly mean temperatures more than 5 degrees Celsius below-normal from Alberta all the way to the Atlantic region. This continued to delay snowmelt across the country, resulting in little change in drought conditions. However, between February and March, the amount and severity of drought in Canada did decrease slightly. Some new abnormally dry areas were identified in central British Columbia and northwestern Alberta, but other dry areas showed improvement. The areas of Moderate Drought (D1) and Severe Drought (D2) were smaller in March than in February. Precipitation in March was above-average in southern British Columbia, east central Alberta, and the Atlantic region, average in northern Ontario and Quebec, and below-average throughout the Prairie region and southern Ontario. Over the winter season, the areas receiving the least precipitation were east central Alberta and southern Manitoba. As a result, an area of Moderate Drought (D1) has been declared in east central Alberta, as precipitation has been record dry to extremely low since September 2013 in that area. In southern Manitoba, the area of Moderate Drought (D1) expanded slightly. The other area of concern was southern British Columbia, but this area has improved since February due to significant precipitation. There remains some Moderate Drought (D1) and a small strip of Severe Drought (D2), but provincial records are showing average to above-average snow water content in that area, which should bring further improvements upon snowmelt. High elevation snowmelt in British Columbia typically begins in the middle of April. -
Drought and Institutional Adaptation in Alberta and Saskatchewan, 1914
Drought and institutional adaptation in Alberta and Saskatchewan, 1914-1939 Gregory P. Marchildon, Suren Kulshreshtha, Elaine Wheaton, and Dave Sauchyn Originally Submitted, 7 November 2006 Final Revision, 30 March 2007 _________________________________ G.P. Marchildon (Corresponding author) Graduate School of Public Policy, University of Regina, 110 – 2 Research Drive, Regina, SK, Canada, S4S 0A2 Phone: 306-585-5464 Fax: 306-585-5461 E-mail: [email protected] S.N. Kulshreshtha Department of Agricultural Economics, University of Saskatchewan, Saskatoon, SK, Canada, S7N 5A8 E. Wheaton Senior Research Scientist, Saskatchewan Research Council, Saskatoon, SK, S7N 2X8 D.J. Sauchyn Earth Science Research Coordinator, Prairie Adaptation Research Consortium Regina, SK, S4S 7J7 1. Introduction and aims During the past one hundred years, at least 40 droughts have occurred in the Canadian Prairie Provinces. In the Great Plains of Alberta and Saskatchewan (and the southwestern corner of the province of Manitoba), extensive, multi-year droughts were observed in the1930s, 1980s and early 21st century. While the prolonged drought of the 1930s is one of the more significant of this series, intense, large-area and multi-year droughts still occur, producing severe hardship, even to those regions accustomed to coping with droughts (O’Brien, 1994; Wheaton, 2000; Wheaton et al., 2005). The drought of 2001 and 2002 is an example of such a recent event. This drought covered much of Canada and may be one of the first cost to coast droughts on record. As in past droughts, the provinces of Alberta and Saskatchewan were hardest hit by meteorological drought resulting in adverse economic impacts. -
Vulnerability and Adaptation to Drought: the Canadian Prairies and South America
University of Calgary PRISM: University of Calgary's Digital Repository University of Calgary Press University of Calgary Press Open Access Books 2016-07 Vulnerability and Adaptation to Drought: The Canadian Prairies and South America Diaz, Harry; Hurlbert, Margot; Warren, Jim University of Calgary Press Diaz, H., Hurlbert, M. & Warren, J. (2016). "Vulnerability and Adaptation to Drought: The Canadian Prairies and South America." Energy, ecology, and the environment series; no. 9. University of Calgary Press, Calgary, Alberta. http://hdl.handle.net/1880/51490 book http://creativecommons.org/licenses/by-nc-nd/4.0/ Attribution Non-Commercial No Derivatives 4.0 International Downloaded from PRISM: https://prism.ucalgary.ca VULNERABILITY AND ADAPTATION: The Canadian Prairies and South America Edited by Harry Diaz, Margot Hurlbert, and Jim Warren ISBN 978-1-55238-820-4 THIS BOOK IS AN OPEN ACCESS E-BOOK. It is an electronic version of a book that can be purchased in physical form through any bookseller or on-line retailer, or from our distributors. Please support this open access publication by requesting that your university purchase a print copy of this book, or by purchasing a copy yourself. If you have any questions, please contact us at [email protected] Cover Art: The artwork on the cover of this book is not open access and falls under traditional copyright provisions; it cannot be reproduced in any way without written permission of the artists and their agents. The cover can be displayed as a complete cover image for the purposes of publicizing this work, but the artwork cannot be extracted from the context of the cover of this specific work without breaching the artist’s copyright. -
Rural Communities Adaptation to Drought
RCAD RURAL COMMUNITIES ADAPTATION TO DROUGHT RESEARCH REPORT | AUGUST 2012 i | RCAD RESEARCH REPORT ACKNOWLEDGEMENTS The Rural Communities Adaptation to Drought (RCAD) project is grateful for the collaboration of the Canadian Plains Research Center CONTENTS and the Prairie Adaptation Research Collaborative of the University of Regina; the University of Saskatchewan; the University of Waterloo; the Saskatchewan Research Council; the Agri-Environment Services SUMMARY OBSERVATIONS—2 Branch of Agriculture and Agri-Food Canada; and the Saskatchewan Watershed Authority. The project offers thanks to the Social Sciences PART I: INTRODUCTION—4 and Humanities Research Council of Canada for its financial support Organization of the Report—4 of this project. Project Objectives—4 We are no less grateful for the generosity of the community members Methodology—5 who donated their time to participate in the sometimes long and tedious interviews. Their contribution was essential to developing a better understanding of how extreme climate events impact rural PART II: THE IMPACTS OF DROUGHT IN 2001-2002—8 people. Characteristics of the Drought—8 The project benefited from the contributions of the following research Impacts on Agriculture—9 team members: Adaptations—10 SAIMA ABASI FANNY LUK Conclusions—11 (MA Candidate, University (MA Geography, University of Saskatchewan) of Waterloo) PART III: STUDY RESULTS— 12 DARRELL CORKAL, P. ENG. JEREMY PITTMAN 12 (Agri-Environment Services (Saskatchewan Watershed Community Overviews— Branch, Agriculture and Authority) Maple Creek Study Area—12 Agri-Food Canada) DR. DAVID SAUCHYN Shaunavon Study Area—13 DR. HARRY DIAZ (Geography, University of Regina, Coronach Study Area—14 (Sociology and Social Studies, and Research Coordinator, University of Regina) Prairie Adaptation Research Gravelbourg Study Area—14 Collaborative) Kindersley Study Area—15 SAM HAGE (PhD Candidate, Sociology, LYLE THOMSON Maidstone Study Area—15 University of Regina) (Saskatchewan Watershed Community Vulnerability—Exposures and Sensitivity—16 Authority) DR. -
Agricultural Adaptation to Drought (ADA) in Canada
Agricultural Adaptation to Drought (ADA) in Canada: The Case of 2001 to 2002 By E. Wheaton, G. Koshida, B. Bonsal, T. Johnston, W. Richards, V. Wittrock May 2007 Prepared for Government of Canada’s Climate Change Impacts and Adaptation Program Saskatchewan Research Council Environment and Forestry SRC Publication No. 11927-1E07 SYNTHESIS REPORT Agricultural Adaptation to Drought (ADA) in Canada: the Case of 2001 to 2002 Prepared for Government of Canada’s Climate Change Impacts and Adaptation Program, Project A932 By E. Wheaton, G. Koshida, B. Bonsal, T. Johnston, W. Richards, V. Wittrock SRC Publication No. 11927-1E07 May 2007 Saskatchewan Research Council 125 – 15 Innovation Blvd. Saskatoon, SK S7N 2X8 Tel: 306-933-5400 Fax: 306-933-7817 Agricultural Adaptation to Drought (ADA) in Canada: May 2007 The Case of 2001 to 2002 Photo Captions: Cover Photo Captions: Cattle grazing on native rangeland in southwest Saskatchewan. May 30, 2006 (B. Godwin, Saskatchewan Research Council). Centre pivot irrigation system and blowing dust near Carberry, Manitoba. May 17, 2007 (A. Nadler, Manitoba Agriculture, Food and Rural Initiatives). Photos used with permission. SRC Publication No. 11927-1E07 i May, 2007 Agricultural Adaptation to Drought (ADA) in Canada: The Case of 2001 to 2002 PROJECT TEAM Principal Investigators • Elaine Wheaton, Saskatchewan Research Council; University of Saskatchewan, email: wheaton*src.sk.ca • Virginia Wittrock, Saskatchewan Research Council, email: wittrock*src.sk.ca • Barrie Bonsal, Environment Canada, National Water -
Text of the Previous Instrumental Record
Second-Order Draft Chapter 3 IPCC WG1 Fourth Assessment Report 1 Chapter 3: Observations: Surface and Atmospheric Climate Change 2 3 Coordinating Lead Authors: Kevin E. Trenberth, Philip D. Jones 4 5 Lead Authors: Peter G. Ambenje, Roxana Bojariu, David R. Easterling, Albert M. G. Klein Tank, David E. 6 Parker, James A. Renwick, Fatemeh Rahimzadeh, Matilde M. Rusticucci, Brian J. Soden, Pan-Mao Zhai 7 8 Contributing Authors: Robert Adler, Lisa Alexander, Hans Alexandersson, Richard P. Allan, Mark P. 9 Baldwin, Martin Beniston, David H. Bromwich, Inés Camilloni, Christophe Cassou, Daniel R. Cayan, 10 Edmund K. M. Chang, John R. Christy, Aiguo Dai, Clara Deser, Nikolai Dotzek, John Fasullo, Ryan L. 11 Fogt, Christopher K. Folland, Piers Forster, Melissa Free, Christoph Frei, Byron Gleason, Jürgen Grieser, 12 Pavel Y. Groisman, Sergey K. Gulev, James W. Hurrell, Masayoshi Ishii, Simon A. Josey, Per W. Kållberg, 13 George N. Kiladis, Ramesh H. Kripalani, Kenneth E. Kunkel, Chiu Ying Lam, John R. Lanzante, Jay H. 14 Lawrimore, David H. Levinson, Beate G. Liepert, Gareth J. Marshall, Carl A. Mears, Philip W. Mote, 15 Hishashi Nakamura, Neville Nicholls, Joel R. Norris, Taikan Oki, Franklin R. Robertson, Karen H. 16 Rosenlof, Fred H. Semazzi, Dennis J. Shea, J. Marshall Shepherd, Theodore G. Shepherd, Steven. C. 17 Sherwood, Adrian J. Simmons, Ian Simmonds, Peter C. Siegmund, Chris D. Thorncroft, Peter W. Thorne, 18 Sakari M. Uppala, Russell S. Vose, Bin Wang, Stephen G. Warren, Richard Washington, Matthew C. 19 Wheeler, Bruce A. Wielicki, Takmeng Wong, David B. Wuertz. 20 21 Review Editors: Brian J. Hoskins, Bubu P. -
The North American Drought Monitor - History, Approach and Benefits
The North American Drought Monitor - History, Approach and Benefits - Trevor Hadwen National Agroclimate Information Service Agriculture &Agri-Food Canada Prepared for the CARIWIN 2nd Senior Administrators Workshop Caribbean Institute for Meteorology and Hydrology, Barbados January 19-20, 2009 PresentationPresentation OverviewOverview • Why Monitor Drought? • NADM Background • USDM / NADM History • Objectives • Participants • Procedure • Input Indicators • Where do we go from here? 2 WhyWhy MonitorMonitor Drought?Drought? • Drought is a normal part of the Climatic Cycle • Drought events will likely be more frequent in the future. • Drought Impacts are significant & widespread • Many economic sectors are affected • Drought is expensive – Since 1980, major droughts and heat waves within the U.S. alone have resulted in costs exceeding 100 billion dollars – In Canada, over the same period, Droughts have resulted in close to 30 billion dollars, with single year droughts in excess of more than 5 billion 3 WhyWhy MonitorMonitor Drought?Drought? From National Drought Mitigation Center 4 Lincoln, Nebraska WhyWhy aa ““DroughtDrought MonitorMonitor””?? • Drought Difficult to Quantify and Measure – Many Drought Tools; None Universally Applicable • Important at the National Level – Efforts to improve the planning, preparation and mitigation of drought now include drought monitoring programs at levels from national to regional and local • Important at the International Level • In today’s global economy the costs and effects of drought often extend beyond international borders – Drought in the Southwest U.S. and a prolonged period of drought in Mexico led to debates about shared water rights – Multi-year drought in the U.S. northern Rockies and western Great Plains extends into the agricultural prairies of Canada, affecting agricultural productivity in both countries • Consistent Drought Depiction Across International Boundaries 5 AA BriefBrief HistoryHistory ofof thethe U.S.U.S.