Exploring the Usefulness of Twitter Data for Political Analysis in Switzerland
Total Page:16
File Type:pdf, Size:1020Kb
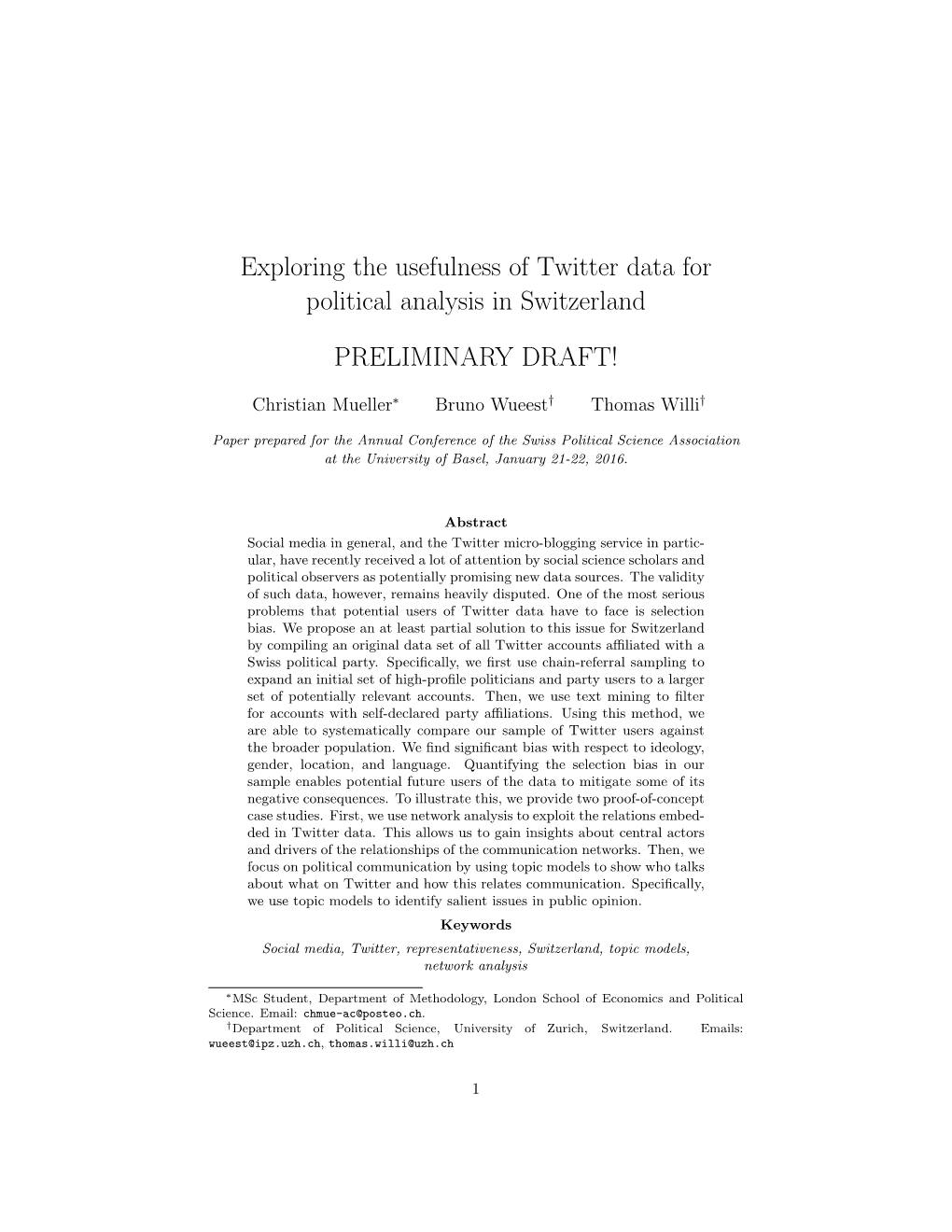
Load more
Recommended publications
-
1 Switzerland Michael O'leary Interviewed
Switzerland Michael O’Leary interviewed Cornelio Sommaruga Interview dates: 5 March 2020 and 1 April 2020 O’Leary: What are your first associations with your place of birth? Sommaruga: Although my Mama’s family had come from Lombardy, she was Roman and I had inherited that. However, I always also had a strong Swiss identity. It would never have crossed my mind to say that I was Italian. No, I identify as Swiss, or rather as Italian- speaKing Swiss. O’Leary: And your father was from Ticino? Sommaruga: Yes, he was born in Lugano, where he completed secondary school before he left for St Gallen to study economics. From there he went to Bern to get a Ph.D. After that he went to work in London until his aunt Carolina Maraini Sommaruga called him to come to Rome and help her with managing the villa and the fortune she had been left with since her husband had died. That is why my father went to Rome. O’Leary: You spent most of your childhood in Rome. Did you go to school with local children? Sommaruga: Because my father was an anti-fascist living in fascist Italy, he put me into a Montessori school, which was a private school, so that I did not have to join Mussolini’s youth organisations such as the [Opera Nazionale] Balilla or the Figli della Lupa. So, I was very lucky that I did not become indoctrinated into fascism, but of course the Montessori education I received was also very valuable in and of itself. -
Ausgewählte Beiträge Zur Schweizer Politik
Ausgewählte Beiträge zur Schweizer Politik Suchabfrage 26.09.2021 Thema Energie Schlagworte Revision des Arbeitslosenversicherungsgesetzes (AVIG), Coronavirus (Covid-19) Akteure Sommaruga, Simonetta (sp/ps) BR UVEK / CF DETEC, Keller, Bernhard (SG, cvp/pdc) Prozesstypen Bundesratsgeschäft Datum 01.01.1965 - 26.09.2021 ANNÉE POLITIQUE SUISSE — AUSGEWÄHLTE BEITRÄGE DER SCHWEIZER POLITIK 01.01.65 - 26.09.21 Impressum Herausgeber Année Politique Suisse Institut für Politikwissenschaft Universität Bern Fabrikstrasse 8 CH-3012 Bern www.anneepolitique.swiss Beiträge von Bieri, Niklaus Bühlmann, Marc Bevorzugte Zitierweise Bieri, Niklaus; Bühlmann, Marc 2021. Ausgewählte Beiträge zur Schweizer Politik: Energie, Revision des Arbeitslosenversicherungsgesetzes (AVIG), Coronavirus (Covid- 19), Bundesratsgeschäft, 2020. Bern: Année Politique Suisse, Institut für Politikwissenschaft, Universität Bern. www.anneepolitique.swiss, abgerufen am 26.09.2021. ANNÉE POLITIQUE SUISSE — AUSGEWÄHLTE BEITRÄGE DER SCHWEIZER POLITIK 01.01.65 - 26.09.21 Inhaltsverzeichnis Allgemeine Chronik 1 Grundlagen der Staatsordnung 1 Institutionen und Volksrechte 1 Regierungspolitik 1 Infrastruktur und Lebensraum 6 Verkehr und Kommunikation 6 Luftfahrt 6 ANNÉE POLITIQUE SUISSE — AUSGEWÄHLTE BEITRÄGE DER SCHWEIZER POLITIK 01.01.65 - 26.09.21 I Abkürzungsverzeichnis AHV Alters- und Hinterlassenenversicherung LPK-NR Legislaturplanungskommission des Nationalrates EU Europäische Union IKT Informations- und Kommunikationstechnologien AVS Assurance-vieillesse et survivants CPL-CN Commission -
Dieköpfeundthemen Ausallen26kantonen
Samstag,7.September 2019 Beilage zu den eidgenössischenWahlen Die Köpfeund Themen ausallen 26 Kantonen Bei denWahlendes National-und Ständerats vom20. Oktoberwerden ungewöhnlichstarke Verschiebungen erwartet.UnserePolitikressorts haben dieAusgangslageinsämtlichen Kantonen analysiert. Wohin steuertdie Schweiz?AntwortenindiesemSonderbundder «Schweiz am Wochenende». Die Schweiz will weiter. Machen wir es möglich. Damit unser Land auch in Zukunft erfolgreich ist, wollen wir: · neue Arbeitsplätze schaffen und bestehende sichern. · den Zugang zu weltweiten Märkten ausbauen. ·InnovationenfördernunddigitaleChancennutzen. ·eineUmweltpolitik, die ökonomisch und sozial verträglich ist. · eine faire, nachhaltig finanzierte Vorsorge. · flexible, moderne Arbeitsmodelle. · eine harte, aber faire, Migrationspolitik. Gemeinsam weiterkommen. 3 Samstag, 7. September 2019 Editorial Analyse zu denWahlenvom 20.Oktober 2019 Klima, Frauenund einFest Eidgenössische Wahlen sind eineernste Sache. Immerhingehtesumdie Frage, wiedie bei- denKammern desBundesparlaments künftig zusammengesetzt sind. Auch in einem Land, in demder Machtdes Parlamentsdurch das Volk enge Grenzengesetzt sind, istdas von erheblicher politischer Bedeutung.Denn zu erwarten ist, dass die Wahlen 2019 wichtige Gewichteverschiebungenbringen. Zunächst sind eidgenössischeWahlenaber auch einFestder Demokratie.Und unter diesem Titel gibt es bereits jetztzweiDinge zu feiern. Erstenshat die Zahl derKandidierenden einen neuenRekordwerterreicht. Dasbelegt, dass – allen Unkenrufen zum Trotz–das Interesse und -
170807 Articolo
Fr. 3.– MONTAG, 7. AUGUST 2017 AZ 5000 Aarau | Nr. 188 | 22. Jahrgang [email protected] 058 200 58 58 [email protected] 058 200 55 55 [email protected] 058 200 53 53 T A R E S N Badenfahrt I Adrien Brody Vom Ursprung und der Ehrenleopard für Faszination des Volksfestes Oscar-Preisträger SEITE 16/17 KULTUR 13 KOMMENTAR Cassis, Moret und Maudet: Die Schweiz hat sich verändert ls Franz Steinegger 1989 Nach- Kommt es zum Dreierticket? folger von Bundesrätin Elisa- bet Kopp werden wollte, wur- Bundesratswahlen Auch Isabelle Moret, Mutter schulpflichtiger Kinder, kandidiert de er gestoppt. Das Parlament Awollte keinen Bundesrat, der im Konku- binat lebte, obwohl er noch verheiratet VON OTHMAR VON MATT UND DORIS KLECK einen Sohn (6) und eine Tochter (11) Dreierticket bevorzuge: «Ich kann mit je- Nachfolge von Didier Burkhalter. Zu war – und zog den verheirateten Kaspar und lebt getrennt von ihrem Ehemann. dem Entscheid unserer Fraktion leben.» den Westschweizer Ambitionen äus- Villiger vor. Auch Christiane Brunner, Nun ist es klar: Auch die Waadtländer Damit kommt es am 1. September vor Beat Walti, der Vize-Fraktionschef der sert er sich im Interview nur zurück- zweimal verheiratet, mit fünf Söhnen – Nationalrätin Isabelle Moret, die sich der FDP-Fraktion mit hoher Wahrschein- FDP und zuständig für das Prozedere, haltend: «Die Romandie ist im Bun- eigenen und adoptierten – erlitt 1993 wochenlang in Schweigen hüllte, kandi- lichkeit zum Dreikampf zwischen Ignazio betont: «Das ist eine wichtige Frage, die desrat sicher nicht untervertreten.» diert für die Nachfolge von Aussenmi- Cassis (TI), Isabelle Moret (VD) und Pi- wir in der Fraktion diskutieren werden. -
Ausgewählte Beiträge Zur Schweizer Politik
Ausgewählte Beiträge zur Schweizer Politik Suchabfrage 01.10.2021 Thema Gesundheit, Sozialhilfe, Sport Schlagworte Gesundheitspolitik Akteure Carobbio Guscetti, Marina (sp/ps, TI) SR/CE Prozesstypen Keine Einschränkung Datum 01.01.1965 - 01.10.2021 ANNÉE POLITIQUE SUISSE — AUSGEWÄHLTE BEITRÄGE DER SCHWEIZER POLITIK 01.01.65 - 01.10.21 Impressum Herausgeber Année Politique Suisse Institut für Politikwissenschaft Universität Bern Fabrikstrasse 8 CH-3012 Bern www.anneepolitique.swiss Beiträge von Heidelberger, Anja Bevorzugte Zitierweise Heidelberger, Anja 2021. Ausgewählte Beiträge zur Schweizer Politik: Gesundheit, Sozialhilfe, Sport, Gesundheitspolitik, 2020. Bern: Année Politique Suisse, Institut für Politikwissenschaft, Universität Bern. www.anneepolitique.swiss, abgerufen am 01.10.2021. ANNÉE POLITIQUE SUISSE — AUSGEWÄHLTE BEITRÄGE DER SCHWEIZER POLITIK 01.01.65 - 01.10.21 Inhaltsverzeichnis Allgemeine Chronik 1 Sozialpolitik 1 Gesundheit, Sozialhilfe, Sport 1 Gesundheitspolitik 1 ANNÉE POLITIQUE SUISSE — AUSGEWÄHLTE BEITRÄGE DER SCHWEIZER POLITIK 01.01.65 - 01.10.21 I Abkürzungsverzeichnis VBS Eidgenössische Departement für Verteidigung, Bevölkerungsschutz und Sport SECO Staatssekretariat für Wirtschaft BAG Bundesamt für Gesundheit ALV Arbeitslosenversicherung BASPO Bundesamt für Sport EO Erwerbsersatzordnung EFV Eidgenössische Finanzverwaltung ATSG Bundesgesetz über den Allgemeinen Teil des Sozialversicherungsrechts DDPS Département fédéral de la défense, de la protection de la population et des sports SECO Secrétariat d'Etat à l'économie -
Ausgewählte Beiträge Zur Schweizer Politik
Ausgewählte Beiträge zur Schweizer Politik Suchabfrage 24.09.2021 Thema Keine Einschränkung Schlagworte Keine Einschränkung Akteure Blocher, Christoph, Lüscher, Christian (fdp/plr, GE) NR/CN, Freisinnig Demokratische Partei.Die Liberalen (FDP) Prozesstypen Keine Einschränkung Datum 01.01.1965 - 24.09.2021 ANNÉE POLITIQUE SUISSE — AUSGEWÄHLTE BEITRÄGE DER SCHWEIZER POLITIK 01.01.65 - 24.09.21 Impressum Herausgeber Année Politique Suisse Institut für Politikwissenschaft Universität Bern Fabrikstrasse 8 CH-3012 Bern www.anneepolitique.swiss Beiträge von Bernhard, Laurent Bühlmann, Marc Hirter, Hans Hohl, Sabine Zumbach, David Bevorzugte Zitierweise Bernhard, Laurent; Bühlmann, Marc; Hirter, Hans; Hohl, Sabine; Zumbach, David 2021. Ausgewählte Beiträge zur Schweizer Politik: Freisinnig Demokratische Partei.Die Liberalen (FDP), 2008 - 2017. Bern: Année Politique Suisse, Institut für Politikwissenschaft, Universität Bern. www.anneepolitique.swiss, abgerufen am 24.09.2021. ANNÉE POLITIQUE SUISSE — AUSGEWÄHLTE BEITRÄGE DER SCHWEIZER POLITIK 01.01.65 - 24.09.21 Inhaltsverzeichnis Allgemeine Chronik 1 Grundlagen der Staatsordnung 1 Politische Grundfragen 1 Nationale Identität 1 Institutionen und Volksrechte 2 Bundesrat 2 Wirtschaft 7 Wirtschaftspolitik 7 Gesellschaftsrecht 7 Sozialpolitik 7 Bevölkerung und Arbeit 7 Arbeitszeit 8 Parteien, Verbände und Interessengruppen 9 Parteien 9 Grosse Parteien 9 ANNÉE POLITIQUE SUISSE — AUSGEWÄHLTE BEITRÄGE DER SCHWEIZER POLITIK 01.01.65 - 24.09.21 I Abkürzungsverzeichnis RK-NR Kommission für Rechtsfragen des -
Es Grünt Sogar Im Ständerat
Datum: 21.10.2019 Blick / Auto-Extra Medienart: Print 8008 Zürich Medientyp: Tages- und Wochenpresse 044/ 259 62 62 Auflage: 107'119 Seite: 6 Auftrag: 3007101 Referenz: 75152773 https://www.blick.ch/ Erscheinungsweise: 6x wöchentlich Fläche: 234'663 mm² Themen-Nr.: 999.222 Ausschnitt Seite: 1/8 WAHLEN 2019 Es grüntso«,_rim Ständerat Bollwerk: ImmuStenrckulimsdot Stockh dominiertmänindieenrtknaancitilswieieihvorermdalechCtUP. nach wie vor die CVP Unter Umstanden kann sie ihre Macht- position sogarsoar ausbauenausbauen. ARGUS DATA INSIGHTS® Schweiz AG | Rüdigerstrasse 15, Postfach, 8027 Zürich T +41 44 388 82 00 | E [email protected] | www.argusdatainsights.ch Datum: 21.10.2019 Blick / Auto-Extra Medienart: Print 8008 Zürich Medientyp: Tages- und Wochenpresse 044/ 259 62 62 Auflage: 107'119 Seite: 6 Auftrag: 3007101 Referenz: 75152773 https://www.blick.ch/ Erscheinungsweise: 6x wöchentlich Fläche: 234'663 mm² Themen-Nr.: 999.222 Ausschnitt Seite: 2/8 verteidigt Aber die NVP ihre Bastion Er ist der Trumpf der CVP: der Ständerat. Hier grün- det sich ihre Machtpositi- on. Denn während die Christde- mokraten im Nationalrat mitt- lerweile mit dem fünften Platz vorliebnehmen müssen, bleibt das Stöckli ihr Bollwerk. Seit Jahrzehnten ist die CVP die dominierende Partei in der kleinen Kammer.Fast immer Sitzverteilung Ständerat stellte sie die meisten Standes- herren - auch aktuell mit 14 Mandaten.Entsprechend liess die Mittepartei immer wieder ihre Muskeln spielen, etwa beim AHV-Steuer-Deal. Auch im neuen Parlament 1 dürfte die CVP ihre Machtposi- tion verteidigen und allenfalls SitzverteilungSitzverteilung nachnach denden WahlenWahlen 2019 sogar leicht ausbauen. Von ih- Resultat: 20.10.2019,20.10.2019, 22.1522.15 UhrUhr ren bisher 14 Sitzen hat sie be- reits acht Sitze im Trockenen.In den zweiten Wahlgängen hat sie UDC 3 reelle Chancen auf sieben Sitze, wobei es sechs bisherige Sitze Parteilos zu verteidigen gilt. -
Ausgewählte Beiträge Zur Schweizer Politik
Ausgewählte Beiträge zur Schweizer Politik Suchabfrage 01.10.2021 Thema Keine Einschränkung Schlagworte Personenfreizügigkeit, Beziehungen zur Europäischen Union (EU) Akteure Borer, Roland (svp/udc, SO) NR/CN, Moret, Isabelle (fdp/plr, VD) NR/CN Prozesstypen Keine Einschränkung Datum 01.01.1965 - 01.10.2021 ANNÉE POLITIQUE SUISSE — AUSGEWÄHLTE BEITRÄGE DER SCHWEIZER POLITIK 01.01.65 - 01.10.21 Impressum Herausgeber Année Politique Suisse Institut für Politikwissenschaft Universität Bern Fabrikstrasse 8 CH-3012 Bern www.anneepolitique.swiss Beiträge von Bühlmann, Marc Caroni, Flavia Guignard, Sophie Hulliger, Caroline Schubiger, Maximilian Bevorzugte Zitierweise Bühlmann, Marc; Caroni, Flavia; Guignard, Sophie; Hulliger, Caroline; Schubiger, Maximilian 2021. Ausgewählte Beiträge zur Schweizer Politik: Personenfreizügigkeit, Beziehungen zur Europäischen Union (EU), 2014 - 2020. Bern: Année Politique Suisse, Institut für Politikwissenschaft, Universität Bern. www.anneepolitique.swiss, abgerufen am 01.10.2021. ANNÉE POLITIQUE SUISSE — AUSGEWÄHLTE BEITRÄGE DER SCHWEIZER POLITIK 01.01.65 - 01.10.21 Inhaltsverzeichnis Allgemeine Chronik 1 Grundlagen der Staatsordnung 1 Institutionen und Volksrechte 1 Bundesrat 1 Aussenpolitik 5 Beziehungen zur EU 5 Sozialpolitik 6 Gesundheit, Sozialhilfe, Sport 6 Medikamente 6 Sozialversicherungen 7 Invalidenversicherung (IV) 7 Soziale Gruppen 7 ANNÉE POLITIQUE SUISSE — AUSGEWÄHLTE BEITRÄGE DER SCHWEIZER POLITIK 01.01.65 - 01.10.21 I Abkürzungsverzeichnis SGK-NR Kommission für soziale Sicherheit und Gesundheit -
Bericht HERBSTSESSION 2020 7
Bericht HERBSTSESSION 2020 7. - 25. September 2020 Mit diesem Bericht informieren wir Sie über die gesundheitspolitischen Geschäfte der vergangenen Session. Indem Sie auf die unterstrichenen Nummern klicken, gelangen Sie direkt zur Parlamentari- schen Geschäftsdatenbank Curia Vista und können sich eingehend über die einzelnen Vorstösse ori- entieren. Sämtliche Informationen sind auf Curia Vista auch in Französisch, Italienisch und Englisch zugänglich, die Sprachwahl finden Sie in der Navigation oben rechts. Wir danken Ihnen für Ihr Ver- ständnis, dass wir den FMH-Sessionsbericht jeweils nur in Deutsch publizieren. Le présent rapport revient sur les objets parlementaires en lien avec la santé traités au cours de la dernière session. En cliquant sur les numéros soulignés, vous accéderez directement à la base de données des objets parlementaires Curia Vista, où vous obtiendrez de plus amples informations sur les différentes interventions. Toutes les informations figurant sur le site Curia Vista sont également disponibles en français, italien et anglais; vous pouvez sélectionner la langue désirée en haut à droite. Le rapport de session de la FMH est publié uniquement en allemand, nous vous remercions de votre compréhension. 2 Inhalt 1 Zusammenfassung ....................................................................................................... 4 2 Behandelte Vorstösse .................................................................................................. 5 3 Auszug neu eingereichte Vorstösse ........................................................................ -
Ausgewählte Beiträge Zur Schweizer Politik
Ausgewählte Beiträge zur Schweizer Politik Suchabfrage 27.09.2021 Thema Keine Einschränkung Schlagworte Wein, Sport Akteure Minder, Thomas (parteilos/indépendant, SH) SR/CE, Stöckli, Hans (sp/ps, BE) SR/CE Prozesstypen Bundesratsgeschäft Datum 01.01.1965 - 27.09.2021 ANNÉE POLITIQUE SUISSE — AUSGEWÄHLTE BEITRÄGE DER SCHWEIZER POLITIK 01.01.65 - 27.09.21 Impressum Herausgeber Année Politique Suisse Institut für Politikwissenschaft Universität Bern Fabrikstrasse 8 CH-3012 Bern www.anneepolitique.swiss Beiträge von Heidelberger, Anja Bevorzugte Zitierweise Heidelberger, Anja 2021. Ausgewählte Beiträge zur Schweizer Politik: Wein, Sport, Bundesratsgeschäft, 2020. Bern: Année Politique Suisse, Institut für Politikwissenschaft, Universität Bern. www.anneepolitique.swiss, abgerufen am 27.09.2021. ANNÉE POLITIQUE SUISSE — AUSGEWÄHLTE BEITRÄGE DER SCHWEIZER POLITIK 01.01.65 - 27.09.21 Inhaltsverzeichnis Allgemeine Chronik 1 Sozialpolitik 1 Gesundheit, Sozialhilfe, Sport 1 Gesundheitspolitik 1 ANNÉE POLITIQUE SUISSE — AUSGEWÄHLTE BEITRÄGE DER SCHWEIZER POLITIK 01.01.65 - 27.09.21 I Abkürzungsverzeichnis VBS Eidgenössische Departement für Verteidigung, Bevölkerungsschutz und Sport SECO Staatssekretariat für Wirtschaft BAG Bundesamt für Gesundheit ALV Arbeitslosenversicherung BASPO Bundesamt für Sport EO Erwerbsersatzordnung EFV Eidgenössische Finanzverwaltung ATSG Bundesgesetz über den Allgemeinen Teil des Sozialversicherungsrechts DDPS Département fédéral de la défense, de la protection de la population et des sports SECO Secrétariat d'Etat à l'économie -
«Genève À La Rencontre Des Suisses»
Album souvenir «Genève à la rencontre des Suisses» Du 18 avril au 27 juin 2015 2 Genève à la rencontre des suisses - fondation pour Genève Genève à la rencontre des suisses - fondation pour Genève 3 Album souvenir POURPOUR UNE UNE Sommaire «Genève à la rencontre GENEVEGENÈVE 6 RAYONNANTE des Suisses» PREAMBULE RAYONNANTE Du 18 avril au 27 juin 2015 8 ET OUVERTE OBJECTIFS & RESULTAT Une tournée initiée et organisée SUR LE MONDE par la Fondation pour Genève 10 à l’occasion du bicentenaire de l’entrée CONCEPT de Genève dans la Confédération - Le voyage - Les messages véhiculés - Les contenus - les programmes 18 ANALYSE D’IMPACT 26 SOUVENIRS DE 45 ETAPES - Ils ont dit de Genève 32 REMERCIEMENTS AUX - Parrains - Intervenants - Délégations - Equipe de la Fondation pour Genève 4 Genève à la rencontre des suisses - fondation pour Genève Genève à la rencontre des suisses - fondation pour Genève 5 Il y a deux cent ans, dans l’Acte d’union de la République de Genève à la Confédération Fiers d’être du 19 mai 1815, la Diète juge cette union SUISSES, OUI «avantageuse aux deux parties, et faite ourtant, ils sont souvent perçus comme étant un peu à part. Est-ce le fait de compter parmi leur population une pour renforcer par une entière communauté P LES GENEVOIS forte présence étrangère? Est-ce de partager avec la France de destinées et d’intérêts, les sentiments plus de 90% de leur frontière? Ou est-ce parce que confinés au d’affection qui les unissent depuis des siècles.» bout du lac, il leur est devenu plus aisé de prendre l’avion pour LE SONT le bout du monde plutôt que de traverser la Versoix, cette ri- vière qui les lie avec le canton de Vaud et le reste de la Suisse? C’est dans le cadre d’une réflexion menée par les Autorités genevoises sur le bicentenaire de l’entrée de Genève dans la Confédération que la Fondation pour Genève, dont la mission est de contribuer au renom de la Cité tant en Suisse que dans le monde, propose d’aller à la rencontre de la population suisse. -
Nein Zur AHV-Scheinreform Liberales Symposium Das Internationale Liberale Sympo- Am 24
Nr. 3 / 15. September 2017 AZB / 3001 Bern er lib ale s a d ORIGINAL 8 seit 184 SCHWEIZER FREISINN Aus den Kantonen 2 / 3 Jungfreisinnige 6 Page Romande 10 Vorschau Herbstsession 16 FDP Service Public 4 FDP Frauen 7 Tag der FDP 12 / 13 Unter Freisinnigen 22 39. Jahrgang / Erscheint viermal jährlich / Offizielles Organ der FDP.Die Liberalen Inhalt Altersvorsorge 2020 Internationales Nein zur AHV-Scheinreform Liberales Symposium Das Internationale Liberale Sympo- Am 24. September geht die Schweiz an die Urnen: Abgestimmt wird über sium, organisiert durch die FDP Inter- den Gegenvorschlag zur Ernährungssicherheit sowie über die Reform national, war auch in seiner zweiten Ausgabe ein Erfolg. Liberale Redner der Altersvorsorge 2020 und die damit verbundene Erhöhung der Mehr- aus Wirtschaft, Gesellschaft und Poli- wertsteuer. FDP.Die Liberalen empfiehlt ein Ja zur Ernährungssicherheit tik widmeten sich dieses Jahr den The- men Digitalisierung, Freihandel und und zweimal Nein zu den Vorlagen über die Altersvorsorge. Populismus. Trotz grossem Defizit soll die AHV mit jungen Generationen damit zusätzliche endgültig vom Tisch. Der Weg für eine Seite 5 der Altersreform noch ausgebaut wer- Lasten auf. Anstatt einer Scheinreform, faire und vernünftige Reform wird frei. den – das kann nicht gut gehen. Die welche die AHV gefährdet, brauchen Die FDP ist bereit, Verantwortung zu AHV-Reform löst die strukturellen wir eine echte Reform, welche die So- übernehmen und stimmt deshalb Nein. Digitalisierung als Chance Probleme der AHV nicht, im Gegen- zialwerke nachhaltig sichert. Mit Ihrem Deshalb dürfen wir die AHV-Schein- Der Begriff «Digitalisierung» ist in teil: Sie vergrössert die strukturellen Nein am 24. September ist der unge- reform mit gutem Gewissen ablehnen.