Best-Fit Probability Models for Maximum Monthly Rainfall in Bangladesh Using Gaussian Mixture Distributions
Total Page:16
File Type:pdf, Size:1020Kb
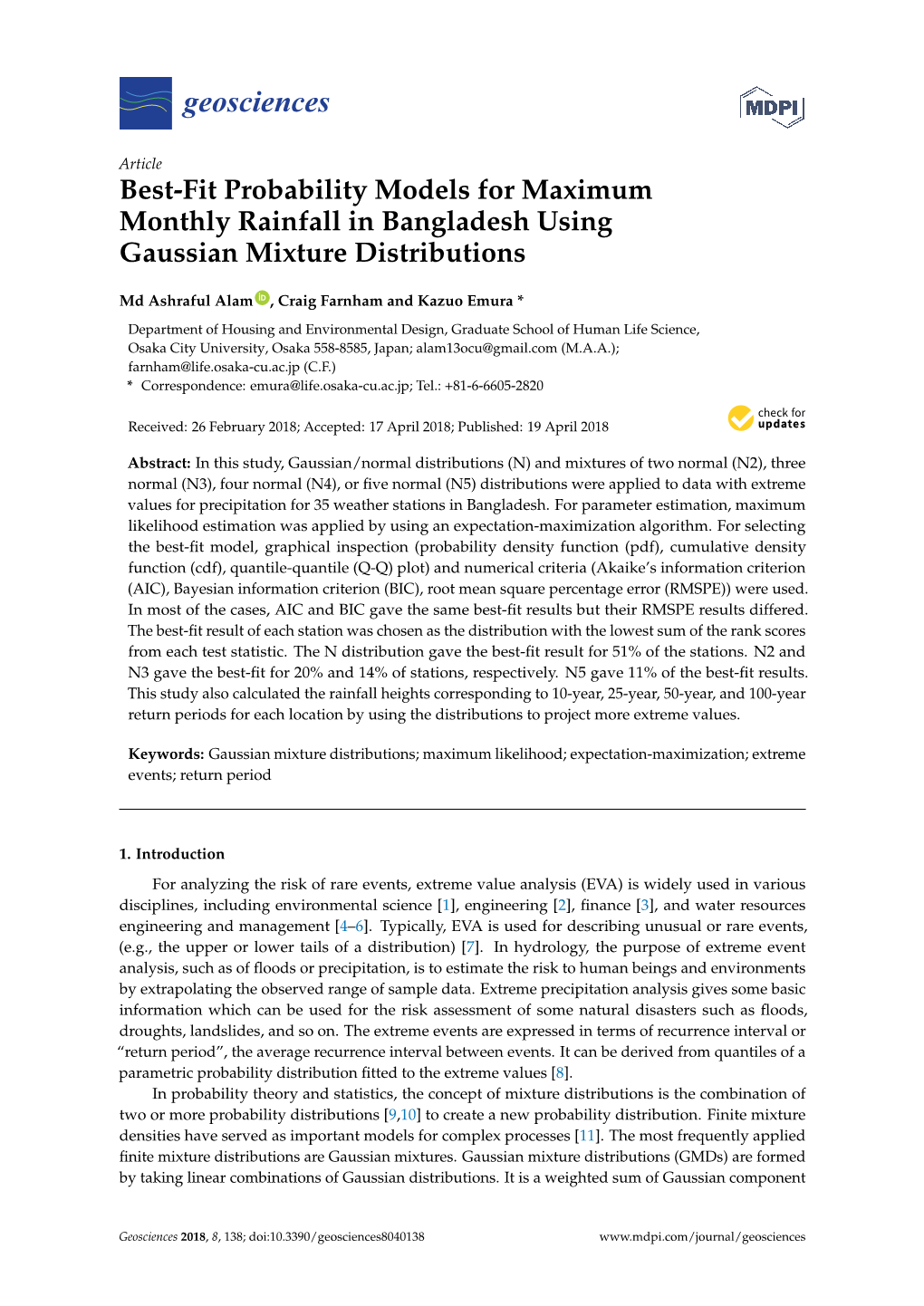
Load more
Recommended publications
-
12. FORMULATION of the URBAN TRANSPORT MASTER PLAN Development of the RSTP Urban Transportation Master Plan (1) Methodology
The Project on The Revision and Updating of the Strategic Transport Plan for Dhaka (RSTP) Final Report 12. FORMULATION OF THE URBAN TRANSPORT MASTER PLAN Development of the RSTP Urban Transportation Master Plan (1) Methodology The development of the RSTP Urban Transportation Master Plan adopted the following methodology (see Figure 12.1): (i) Elaborate the master plan network through a screen line analysis by comparing the network capacity and future demand. (ii) Identify necessary projects to meet future demand at the same time avoiding excessive capacity. (iii) Conducts economic evaluation of each project to give priority on projects with higher economic return. (iv) Conduct preliminary environmental assessment of every project and consider countermeasures against environmental problems, if any. (v) Make a final prioritization of all physical projects by examining their respective characteristics from different perspectives. (vi) Classify the projects into three categories, namely short-, medium- and long-term projects, by considering the financial constraints. (vii) Prepare an action plan for short-term projects together with “soft” measures. Mid-term Project Source: RSTP Study Team Figure 12.1 Development Procedure for the Master Plan 12-1 The Project on The Revision and Updating of the Strategic Transport Plan for Dhaka (RSTP) Final Report (2) Output of the Transportation Network Plan The RSTP urban transportation network plan was developed based on a review and a modification of the STP network plan. The main points of the modification or adoption of the STP network master plan are as follows: i. Harmonization with future urban structure, land-use plan and development of network plan. -
Connecting Bangladesh: Economic Corridor Network
Connecting Bangladesh: Economic Corridor Network Economic corridors are anchored on transport corridors, and international experience suggests that the higher the level of connectivity within and across countries, the higher the level of economic growth. In this paper, a new set of corridors is being proposed for Bangladesh—a nine-corridor comprehensive integrated multimodal economic corridor network resembling the London Tube map. This paper presents the initial results of the research undertaken as an early step of that development effort. It recommends an integrated approach to developing economic corridors in Bangladesh that would provide a strong economic foundation for the construction of world-class infrastructure that, in turn, could support the growth of local enterprises and attract foreign investment. About the Asian Development Bank COnnecTING BANGLADESH: ADB’s vision is an Asia and Pacific region free of poverty. Its mission is to help its developing member countries reduce poverty and improve the quality of life of their people. Despite the region’s many successes, it remains home to a large share of the world’s poor. ADB is committed to reducing poverty through inclusive economic growth, environmentally sustainable growth, and regional integration. ECONOMIC CORRIDOR Based in Manila, ADB is owned by 67 members, including 48 from the region. Its main instruments for helping its developing member countries are policy dialogue, loans, equity investments, guarantees, grants, NETWORK and technical assistance. Mohuiddin Alamgir -
July 2016 Volume 3 No 3
6 VOLUME 3 NO 3 JULY 2016 DHAKA CENTRAL INTERNATIONAL MEDICAL COLLEGE JOURNAL (APPROVED BY BMDC) July 2016, Vol. 3 No. 3 Contents From the Desk of Editor-in-Chief 3 Instructions for Authors 4 Editorial Novel Treatment of Diabetic Nephropathy 12 Original Articles Incidence of Malignancy in Thyroid Nodule 14 Abedin SAMA, Alam MM, Islam MS, Fakir MAY Dyslipidemia and Atherogenic Index among the 21 Young Female Doctors ofBangladesh. Khanduker S, Hoque MM, Khanduker N, Chowdhury MAA, Nazneen M A Study on Stroke in Young Patients due to Cardiac 26 Disease in a Tertiary Care Hospital in Dhaka City Mukta M, Mohammad QD, Mir AS Variation of Transverse Diameter ofDry Ossified 33 Human Atlas Vertebra of Male and Female Rahman S, Ara S, Sayeed S, Rashid S, Ferdous Z, Kashem K Study on Health Effects of Teenage Pregnancies among the Patients 36 Attending Antenatal Care Centre of Chittagong Medical College Hospital Tarafdar MA, Begum N, Das SR, Begum S, Sultana A, Rahman R, Begum R Identification ofDifferent Clinical Features and Complications of 41 Type 2 Diabetes Mellitus in Bengaladeshi Males Begum F, Shamim KM, Akter S, Hossain S, Nazma N, Afrin M, Moureen A Review Articles Female Genital Tuberculosis- A Review Article 46 Shaheed S, Mamun SMAA, Khanom M Case Reports Round Worm induced Acute Appendicitis- an Incidental 51 Finding during Colonoscopy Masum QAA, Islam MN 1 Dhaka Central International Medical College Journal Vol.13 No. 3July 2016 An Official Organ of Dhaka Central International Medical College CHIEF PATRON ADVISORS The Dhaka Central International Prof. Md. Anwarul Islam Md. -
UNC Parking Zone Map UNC Transportation & Parking
UNC Parking Zone Map UNC Transportation & Parking Q R S T U V W X Y Z A B C D E F G H I J K L M N O P 26 **UNC LEASES SPACE CAROLINA . ROAD IN THESE BUILDINGS 21 21 MT HOMESTEAD NORTH LAND MGMT. PINEY OPERATIONS CTR. VD. (NC OFFICE HORACE WILLIAMS AIRPORT VD., HILL , JR. BL “RR” 41 1 1 Resident 41 CommuterRR Lot R12 UNC VD AND CHAPEL (XEROX) TE 40 MLK BL A PRINTING RIVE EXTENSION MLK BL ESTES D SERVICES TIN LUTHER KING TERST PLANT N O I AHEC T EHS HOMESTEAD ROAD MAR HANGER VD. 86) O I-40 STORAGE T R11 TH (SEE OTHER MAPS) 22 22 O 720, 725, & 730 MLK, JR. BL R1 T PHYSICAL NOR NORTH STREET ENVRNMEN HL .3 MILES TO TH. & SAFETY ESTES DRIVE 42 COMMUTER LOT T. 42 ER NC86 ELECTRICAL DISTRICENTBUTION OPERATIONS SURPLUS WA REHOUSE N1 ST GENERAL OREROOM 2 23 23 2 R1 CHAPEL HILL ES MLK JR. BOULE NORTH R1 ARKING ARD ILITI R1 / R2OVERFLOW ZONEP V VICES C R A F SHOPS GY SE EY 43 RN 43 ENERBUILDING CONSTRUCTION PRITCHARD STREET R1 NC 86 CHURCH STREET . HO , JR. BOULE ES F R1 / V STREET SER L BUILDING VICE ARD A ST ATIO GI EET N TR AIRPOR R2 S T DRIVE IN LUTHER KING BRANCH T L MAR HIL TH WEST ROSEMARY STREET EAST ROSEMARY STREET L R ACILITIES DRIVE F A NO 24 STUDRT 24 TH COLUMBI IO CHAPE R ADMINIST OFF R NO BUILDINGICE ATIVE R10 1700 N9 MLK 208 WEST 3 N10 FRANKLIN ST. -
The Completeness and Vulnerability of Road Network in Bangladesh
M. A. Ali, S. M. Seraj and S. Ahmad (eds): ISBN 984-823-002-5 The Completeness and Vulnerability of Road Network in Bangladesh A. S. M. Abdul Quium and S. A. M. Aminul Hoque Department of Urban and Regional Planning Bangladesh University of Engineering and Technology, Dhaka-1000, Bangladesh Abstract Road transportation has emerged as the most popular mode of transportation in Bangladesh. Despite being the most popular mode, it suffers heavily from network failures due to natural as well as man-made disruptions. This paper presents the major findings of a study relating to the completeness of the road network with respect to the pattern of traffic flow and its vulnerability with reference to the disruptions caused by the flood of 1998. The study considered development of road network by regions on the basis of degree of network completeness by connectivity indices namely, γ and α indices that apply the Graph Theory to measure the geometric pattern of a network. The analysis revealed that the road network development in Bangladesh at the national and regional levels is still in its early stage. The existing network has a very few circuits for movement at all the levels and, therefore, susceptible to high risk of disruption. In terms of circuitry, the network has a bare marginal grid configuration at the divisional and national levels. The present overwhelming dependence on road transportation system will continue in the future. In this context, completeness of the whole road network should be considered in all future road planning exercises. Assessment of vulnerability of links around Dhaka deserves special consideration as any link failure of the national highways around Dhaka affects almost the whole system. -
X-Ray Single Crystal Structure, Tautomerism Aspect, DFT, NBO
crystals Article X-ray Single Crystal Structure, Tautomerism Aspect, DFT, NBO, and Hirshfeld Surface Analysis of a New Schiff Bases Based on 4-Amino-5-Indol-2-yl-1,2,4-Triazole-3-Thione Hybrid Ahmed T. A. Boraei 1,*, Saied M. Soliman 2 , Matti Haukka 3 , El Sayed H. El Tamany 1 , Abdullah Mohammed Al-Majid 4 and Assem Barakat 4,* 1 Chemistry Department, Faculty of Science, Suez Canal University, Ismailia 41522, Egypt; [email protected] 2 Department of Chemistry, Faculty of Science, Alexandria University, P.O. Box 426, Ibrahimia, Alexandria 21321, Egypt; [email protected] or [email protected] 3 Department of Chemistry, University of Jyväskylä, P.O. Box 35, FI-40014 Jyväskylä, Finland; matti.o.haukka@jyu.fi 4 Department of Chemistry, College of Science, King Saud University, P.O. Box 2455, Riyadh 11451, Saudi Arabia; [email protected] * Correspondence: [email protected] (A.T.A.B.); [email protected] (A.B.); Tel.: +966-11467-5901 (A.B.); Fax: +966-11467-5992 (A.B.) Abstract: Four different new Schiff basses tethered indolyl-triazole-3-thione hybrid were designed Citation: Boraei, A.T.A.; Soliman, and synthesized. X-ray single crystal structure, tautomerism, DFT, NBO and Hirshfeld analysis were S.M.; Haukka, M.; El Tamany, E.S.H.; explored. X-ray crystallographic investigations with the aid of Hirshfeld calculations were used Al-Majid, A.M.; Barakat, A. X-ray to analyze the molecular packing of the studied systems. The H···H, H···C, S···H, Br···C, O···H, Single Crystal Structure, C···C and N···H interactions are the most important in the molecular packing of 3. -
Northwest Corridor Road Project, Phase 2
Initial Environmental Examination May 2017 BAN: South Asia Subregional Economic Cooperation Dhaka – Northwest Corridor Road Project, Phase 2 Hatikamrul - Rangpur Road Appendices A-G (Part 2 of 3) Prepared by Roads and Highways Department, Government of Bangladesh for the Asian Development Bank. 202 Appendix A APPENDIX A: TERMS OF REFERENCE FOR ENVIRONMENTAL IMPACT ASSESSMENT OF ROAD DEVELOPMENT PROJECTS UNDER SUBREGIONAL TRANSPORT PROJECT (SRTP) A. Background 1. The Government of Bangladesh (GoB) has received a loan from Asian Development Bank (ADB) for the Subregional Transport Project Preparatory Facility under Technical Assistance for Subregional Road Transport Project Preparatory Facility (ADB Loan 2688-BAN). GoB has resolved to apply a portion of the loan to meet the expenditure for consultancy services to be rendered by international consultants to prepare (a) feasibility studies and (b) detailed engineering designs for upgrading selected national highways and zilla roads from 2-lanes to 4-lanes to promote subregional development. The Ministry of Communications (MOC) is the Executing Agency and Roads and Highways Department (RHD) is the Implementation Agency. 2. The environmental impact assessment (EIA) process will be based on current information, including an accurate project description, and appropriate environmental and social baseline data. In the environmental assessment, Roads and Highways Department (RHD) as the project proponent will consider all potential impacts and risks of the road development works on physical, biological, socioeconomic (occupational health and safety, community health and safety, vulnerable groups and gender issues, and impacts on livelihoods and physical cultural resources) in an integrated way. This TOR is prepared to carryout detailed EIA study for the ‘Subregional Transport Project Preparatory Facility’ in accordance with the relevant laws and regulations in Bangladesh and the Asian Development Bank’s Safeguard Policy Statement, 2009. -
Whispers to Voices: Gender and Social Transformation in Bangladesh (March 2008)
43045 Public Disclosure Authorized Public Disclosure Authorized Public Disclosure Authorized Public Disclosure Authorized WHISPERS TO VOICES Gender and Social Transformation in Bangladesh Bangladesh Development Series Paper No. 22 South Asia Sustainable Development Department South Asia Region The World Bank March 2008 www.worldbank.org.bd/bds Document of the World Bank The World Bank World Bank Office Dhaka Plot- E-32, Agargaon, Sher-e-Bangla Nagar, Dhaka-1207, Bangladesh Tel: 880-2-8159001-28 Fax: 880-2-8159029-30 www.worldbank.org.bd The World Bank 1818 H Street, N.W. Washington DC 20433, USA Tel: 1-202-473-1000 Fax: 1-207-477-6391 www.worldbank.org All Bangladesh Development Series (BDS) publications are downloadable at: www.worldbank.org.bd/bds Standard Disclaimer: This volume is a product of the staff of the International Bank for Reconstruction and Development/The World Bank. The findings, interpretations, and conclusions expressed in this paper do not necessarily reflect the views of the Executive Directors of the World Bank or the governments they represent. The World Bank does not guarantee the accuracy of the data included in this work. The boundaries, colors, denominations, and other information shown on any map in this work do not imply any judgment on the part of the World Bank concerning the legal status of any territory or the endorsement or acceptance of such boundaries. Copyright Statement: The material in this publication is copyrighted. The World Bank encourages dissemination of its work and will normally grant permission to reproduce portion of the work promptly. A publication produced by the World Bank with partial funding support from the Australian Agency for International Development (AusAID), the Australian Government's overseas aid agency. -
Pin Information for Hardcopy® II HC240 / Stratix® II EP2S180 F1508 Companion Devices Version
Pin Information for HardCopy® II HC240 / Stratix® II EP2S180 F1508 Companion Devices Version 1.1 Bank Number VREF Group Pin Name/Function Optional Function(s)/DQ Configuration Function for F1508 DQ Group for DQS DQ Group for DQS DQ Group for DQS Group for DQS x4 Mode Stratix II Only (Note 1) x8/x9 Mode x16/x18 Mode x32/x36 Mode VCCD_PLL7 L29 VCCA_PLL7 M29 GNDA_PLL7 K29 GNDA_PLL7 K30 B2 FPLL7CLKp INPUT C39 B2 FPLL7CLKn INPUT C38 NC (Note 3) D38 NC (Note 4) J32 NC (Note 4) J31 NC (Note 4) C37 NC (Note 4) C36 NC (Note 4) L31 NC (Note 4) L30 NC (Note 4) D37 NC (Note 4) D36 NC (Note 4) K32 NC (Note 4) K31 NC (Note 4) B38 NC (Note 4) B37 NC (Note 4) H32 NC (Note 4) H31 NC (Note 4) J34 NC (Note 4) J33 NC (Note 4) F35 NC (Note 4) F34 NC (Note 4) G35 NC (Note 4) G34 NC (Note 4) L33 NC (Note 4) L32 NC (Note 3) G33 NC (Note 4) H35 NC (Note 4) H34 NC (Note 4) M31 NC (Note 4) M30 NC (Note 4) E37 NC (Note 4) E36 NC (Note 4) E35 NC (Note 4) E34 NC (Note 4) F37 NC (Note 4) F36 B2 IO DIFFIO_TX71p M33 B2 IO DIFFIO_TX71n M32 B2 IO DIFFIO_RX70p K35 B2 IO DIFFIO_RX70n K34 B2 IO DIFFIO_TX70p N31 B2 IO DIFFIO_TX70n N30 B2 IO DIFFIO_RX69p E39 B2 IO DIFFIO_RX69n E38 B2 IO DIFFIO_TX69p N29 B2 IO DIFFIO_TX69n N28 B2 IO DIFFIO_RX68p F39 B2 IO DIFFIO_RX68n F38 B2 IO DIFFIO_TX68p P27 B2 IO DIFFIO_TX68n P26 B2 IO DIFFIO_RX67p L35 B2 IO DIFFIO_RX67n L34 B2 IO DIFFIO_TX67p P29 B2 IO DIFFIO_TX67n P28 B2 IO DIFFIO_RX66p G39 B2 IO DIFFIO_RX66n G38 B2 IO DIFFIO_TX66p R28 B2 IO DIFFIO_TX66n R27 B2 IO DIFFIO_RX65p J37 B2 IO DIFFIO_RX65n J36 B2 IO DIFFIO_TX65p N33 B2 IO DIFFIO_TX65n N32 NC (Note 3) J35 B2 IO DIFFIO_RX64p H37 B2 IO DIFFIO_RX64n H36 B2 IO DIFFIO_TX64p M35 B2 IO DIFFIO_TX64n M34 PT-HCS205-1.1 Copyright © 2007 Altera Corp. -
On the Existence of Partitioned Incomplete Latin Squares with Five Parts
Marshall University Marshall Digital Scholar Mathematics Faculty Research Mathematics 2019 On the Existence of Partitioned Incomplete Latin Squares with Five Parts Jaromy Kuhl Donald McGinn Michael W. Schroeder Follow this and additional works at: https://mds.marshall.edu/mathematics_faculty Part of the Discrete Mathematics and Combinatorics Commons AUSTRALASIAN JOURNAL OF COMBINATORICS Volume 74(1) (2019), Pages 46–60 On the existence of partitioned incomplete Latin squares with five parts Jaromy Kuhl Donald McGinn Department of Mathematics and Statistics University of West Florida Pensacola, FL 32514 U.S.A. [email protected] [email protected] Michael William Schroeder Department of Mathematics Marshall University Huntington, WV 25755 U.S.A. [email protected] Abstract Let a, b, c, d,ande be positive integers. In 1982 Heinrich showed the existence of a partitioned incomplete Latin square (PILS) of type (a, b, c) and (a, b, c, d) if and only if a = b = c and 2a ≥ d. For PILS of type (a, b, c, d, e) with a ≤ b ≤ c ≤ d ≤ e, it is necessary that a + b + c ≥ e, but not sufficient. In this paper we prove an additional necessary condition and classify the existence of PILS of type (a, b, c, d, a + b + c)andPILS with three equal parts. Lastly, we show the existence of a family of PILS in which the parts are nearly the same size. 1 Introduction Let a, n ∈ Z+ and S be a symbol set of order n.Let[n]={1,...,n}, a+S = {a+s | s ∈ S},andaS be the multiset in which each element of S occurs a times. -
Tuberculosis Infectious Pool and Associated Factors in East Gojjam Zone, Northwest Ethiopia Mulusew Andualem Asemahagn1* , Getu Degu Alene1 and Solomon Abebe Yimer2,3
Asemahagn et al. BMC Pulmonary Medicine (2019) 19:229 https://doi.org/10.1186/s12890-019-0995-3 RESEARCH ARTICLE Open Access Tuberculosis infectious pool and associated factors in East Gojjam Zone, Northwest Ethiopia Mulusew Andualem Asemahagn1* , Getu Degu Alene1 and Solomon Abebe Yimer2,3 Abstract Background: Globally, tuberculosis (TB) lasts a major public health concern. Using feasible strategies to estimate TB infectious periods is crucial. The aim of this study was to determine the magnitude of TB infectious period and associated factors in East Gojjam zone. Methods: An institution-based prospective study was conducted among 348 pulmonary TB (PTB) cases between December 2017 and December 2018. TB cases were recruited from all health facilities located in Hulet Eju Enesie, Enebse Sarmider, Debay Tilatgen, Dejen, Debre-Markos town administration, and Machakel districts. Data were collected through an exit interview using a structured questionnaire and analyzed by IBM SPSS version25. The TB infectious period of each patient category was determined using the TB management time and sputum smear conversion time. The sum of the infectious period of each patient category gave the infectious pool of the study area. A multivariable logistic regression analysis was used to identify factors associated with the magnitude of TB infectious period. Results: Of the total participated PTB cases, 209(60%) were male, 226(65%) aged < 30 years, 205(59%) were from the rural settings, and 77 (22%) had comorbidities. The magnitude of the TB infectious pool in the study area was 78,031 infectious person-days. The undiagnosed TB cases (44,895 days), smear-positive (14,625 days) and smear- negative (12,995 days) were major contributors to the infectious pool. -
Antigenic and Genetic Characteristics of Zoonotic Influenza Viruses and Development of Candidate Vaccine Viruses for Pandemic Preparedness
4 Antigenic and genetic characteristics of zoonotic influenza viruses and development of candidate vaccine viruses for pandemic preparedness February 2019 The development of influenza candidate vaccine viruses (CVVs), coordinated by WHO, remains an essential component of the overall global strategy for pandemic preparedness. Selection and development of CVVs are the first steps towards timely vaccine production and do not imply a recommendation for initiating manufacture. National authorities may consider the use of one or more of these CVVs for pilot lot vaccine production, clinical trials and other pandemic preparedness purposes based on their assessment of public health risk and need. Zoonotic influenza viruses continue to be identified and evolve both genetically and antigenically, leading to the need for additional CVVs for pandemic preparedness purposes. Changes in the genetic and antigenic characteristics of these viruses relative to existing CVVs, and their potential risks to public health justify the need to select and develop new CVVs. This document summarizes the genetic and antigenic characteristics of recent zoonotic influenza viruses and related viruses circulating in animals1 that are relevant to CVV updates. Institutions interested in receiving these CVVs should contact WHO at [email protected] or the institutions listed in announcements published on the WHO website2. Influenza A(H5) Since their emergence in 1997, highly pathogenic avian influenza (HPAI) A(H5) viruses of the A/goose/Guangdong/1/96 haemagglutinin (HA) lineage have become enzootic in some countries, have infected wild birds and continue to cause outbreaks in poultry and sporadic human infections. These viruses have diversified genetically and antigenically, including the emergence of viruses with replacement of the N1 gene segment by N2, N3, N5, N6, N8 or N9 gene segments, leading to the need for multiple CVVs.