The Perception-Cognition Border: a Case for Architectural Division
Total Page:16
File Type:pdf, Size:1020Kb
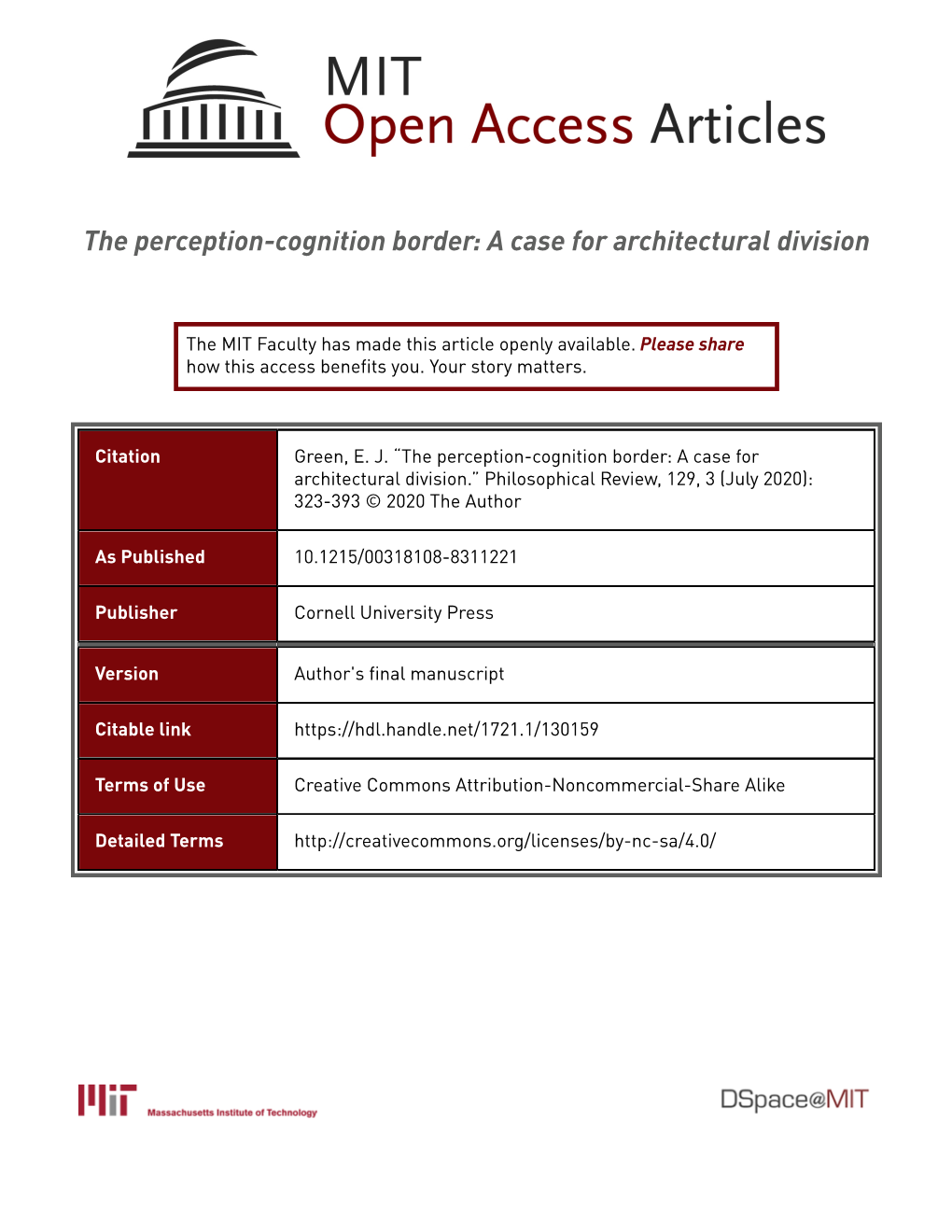
Load more
Recommended publications
-
Andy Clark, ‘Whatever Next?’
BBS Commentary on: Andy Clark, ‘Whatever Next?’ Word Counts Abstract: 61 Main text: 994 References: 69 Entire text: 1,180 Commentary Title Perception vs Action: The Computations May Be The Same But The Direction Of Fit Differs Author Information Nicholas Shea Faculty of Philosophy University of Oxford 10 Merton Street Oxford OX1 4JJ UK tel. 01865 286896 [email protected] www.philosophy.ox.ac.uk/members/nicholas_shea Abstract Although predictive coding may offer a computational principle that unifies perception and action, states with different directions of fit are involved (with indicative and imperative contents, respectively). Predictive states are adjusted to fit the world in the course of perception, but in the case of action the corresponding states act as a fixed target towards which the agent adjusts the world. Main Text One of the central insights motivating Clark’s interest in the potential for predictive coding to provide a unifying computational principle is the fact that it can be the basis of effective algorithms in both the perceptual and motor domains (Eliasmith 2007, 380). That is surprising because perceptual inference in natural settings is based on a rich series of sensory inputs at all times, whereas a natural motor control task only specifies a final outcome. Many variations in the trajectory are irrelevant to achieving the final goal (Todorov and Jordan 2002), a redundancy that is absent from the perceptual inference problem. Despite this disanalogy, the two tasks are instances of the same general mathematical problem (Todorov 2006). Clark emphasises the “deep unity” between the two problems, which is justified but might serve to obscure an important difference. -
Bootstrap Hell: Perceptual Racial Biases in a Predictive Processing Framework
Bootstrap Hell: Perceptual Racial Biases in a Predictive Processing Framework Zachariah A. Neemeh ([email protected]) Department of Philosophy, University of Memphis Memphis, TN 38152 USA Abstract This enables us to extract patterns from ambiguous signals Predictive processing is transforming our understanding of the and establish hypotheses about how the world works. brain in the 21st century. Whereas in the 20th century we The training signals that we get from the world are, understood the brain as a passive organ taking in information however, biased in all the same unsightly ways that our from the world, today we are beginning to reconceptualize it societies are biased: by race, gender, socioeconomic status, as an organ that actively creates and tests hypotheses about its nationality, and sexual orientation. The problem is more world. The promised revolution of predictive processing than a mere sampling bias. Our societies are replete with extends beyond cognitive neuroscience, however, and is prejudice biases that shape the ways we think, act, and beginning to make waves in the philosophy of perception. Andy Clark has written that predictive processing creates a perceive. Indeed, a similar problem arises in machine “bootstrap heaven,” enabling the brain to develop complex learning applications when they are inadvertently trained on models of the world from limited data. I argue that the same socially biased data (Avery, 2019; N. T. Lee, 2018). The principles also create a “bootstrap hell,” wherein prejudice basic principle in operation here is “garbage in, garbage biases inherent in our inegalitarian societies result in out”: a predictive system that is trained on socially biased permanent perceptual modifications. -
The Extended Mind Andy Clark & David Chalmers1
The extended mind Andy Clark & David Chalmers1 1. Introduction Where does the mind stop and the rest of the world begin? The question invites two standard replies. Some accept the boundaries of skin and skull, and say that what is outside the body is outside the mind. Others are impressed by arguments suggesting that the meaning of our words ‘just ain’t in the head’, and hold that this externalism about meaning carries over into an externalism about mind. We propose to pursue a third posi- tion. We advocate a very different sort of externalism: an active externalism, based on the active role of the environment in driving cogni- tive processes. 2. Extended cognition Consider three cases of human problem-solving: (1) A person sits in front of a computer screen which displays images of various two-dimensional geometric shapes and is asked to answer questions concerning the potential fit of such shapes into depicted ‘sockets’. To assess fit, the person must mentally rotate the shapes to align them with the sockets. (2) A person sits in front of a similar computer screen, but this time can choose either to physically rotate the image on the screen, by pressing a rotate button, or to mentally rotate the image as before. We can also suppose, not unrealistically, that some speed advantage accrues to the physical rotation operation. (3) Sometime in the cyberpunk future, a person sits in front of a similar computer screen. This agent, however, has the benefit of a neural implant which can perform the rotation operation as fast as the computer in the previous example. -
Archives De Pierre Buser
Fonds Pierre Buser (1921-2013) Centre d’Archives en Philosophie, Histoire et Édition des Sciences (UMS3610, CNRS-ENS Paris) Fonds Pierre Buser (1921-2013) Plan de l’inventaire : Informations liminaires I. Carrière (PB 1.1) II. Textes de Pierre Buser (PB 1.2-PB 4) III. Cours de Pierre Buser (PB 5.1-PB 5.3) IV. Journées d’études (PB 5.4-PB 6.8) V. Correspondance (PB 6.9-PB 7.5) VI. Documentation de travail (PB 7.6-PB 19.4) Index des noms cités dans l’inventaire CAPHÉS -2018 Page 1 Fonds Pierre Buser (1921-2013) Biographie : Notice issue du site de l’Académie des sciences Pierre Buser, né le 19 août 1921 est décédé le 29 décembre 2013. Il avait été élu correspondant de l'Académie le 4 février 1980, puis membre le 6 juin 1988 dans la section actuellement dénommée Biologie intégrative. Ancien élève de l'École normale supérieure, il était professeur émérite à l'Université Pierre-et-Marie-Curie. Neurophysiologiste, il fut un des fondateurs, en France et dans le monde, de la physiologie intégrative moderne du système nerveux. Il appartenait à l'École de neurophysiologie du comportement qui poursuit ses investigations dans le champ de la psychophysiologie et des fonctions infiniment complexes du cerveau. Avec lui, c'est le maître de toute une génération de neurobiologistes qui [a disparu]. Pierre Buser a consacré ses travaux à la neurophysiologie et à la psychophysiologie. Il [a poursuivi] ses investigations dans le champ infiniment complexe des fonctions du cerveau. Les travaux de Pierre Buser se sont de façon permanente centrés autour des activités électrophysiologiques du cerveau, comme marqueurs de son activité, en liaison avec le comportement de l’animal. -
Environmental, Cultural, Economic, and Social Sustainability
Eleventh International Conference on Environmental, Cultural, Economic, and Social Sustainability 21–23 JANUARY 2015 | SCANDIC HOTEL COPENHAGEN COPENHAGEN, DENMARK | ONSUSTAINABILITY.COM Sustainability Conference 1 Dear Delegate, The Sustainability knowledge community is an international conference, a cross-disciplinary scholarly journal, a book imprint, and an online knowledge community which, together, set out to describe, analyze and interpret the role of Sustainability. These media are intended to provide spaces for careful, scholarly reflection and open dialogue. The bases of this endeavour are cross- disciplinary. The community is brought together by a common concern for sustainability in an holistic perspective, where environmental, cultural, economic and, social concerns intersect. In addition to organizing the Sustainability Conference, Common Ground publishes papers from the conference at http://onsustainability.com/publications/journal. We do encourage all conference participants to submit an article based on their conference presentation for peer review and possible publication in the journal. We also publish books at http://onsustainability.com/publications/books, in both print and electronic formats. We would like to invite conference participants to develop publishing proposals for original works or for edited collections of papers drawn from the journal which address an identified theme. Finally, please join our online conversation by subscribing to our monthly email newsletter, and subscribe to our Facebook, RSS, or Twitter feeds at http://onsustainability.com. Common Ground also organizes conferences and publishes journals in other areas of critical intellectual human concern, including diversity, museums, technology, humanities and the arts, to name several (see http://commongroundpublishing.com). Our aim is to create new forms of knowledge community, where people meet in person and also remain connected virtually, making the most of the potentials for access using digital media. -
Language, Embodiment, and the Cognitive Niche
Opinion TRENDS in Cognitive Sciences Vol.10 No.8 Full text provided by www.sciencedirect.com Language, embodiment, and the cognitive niche Andy Clark School of Philosophy, Psychology and Language Sciences, University of Edinburgh, David Hume Tower, George Square, Edinburgh, EH8 9JX, UK Embodied agents use bodily actions and environmental in ways that (when successful) aid thinking and reasoning interventions to make the world a better place to think about some target domain or domains [11–13]. These in. Where does language fit into this emerging picture of physical structures combine with appropriate culturally the embodied, ecologically efficient agent? One useful transmitted practices to enhance problem-solving, and way to approach this question is to consider language (in the most dramatic cases) to make possible whole new itself as a cognition-enhancing animal-built structure. To forms of thought and reason. take this perspective is to view language as a kind of I present three ways in which to begin to flesh out this self-constructed cognitive niche: a persisting but never idea, and sketch a computational (connectionist and dyna- stationary material scaffolding whose crucial role in mical) framework in which to embed it. I end the argument promoting thought and reason remains surprisingly by suggesting an additional (non-essential but potentially poorly understood. It is the very materiality of this important) refinement: that linguistic activity might turn linguistic scaffolding, I suggest, that gives it some key out to be a mode of cognition-enhancing self-stimulation in benefits. By materializing thought in words, we create a system with no ‘Central Meaner’ orchestrating the whole structures that are themselves proper objects of percep- [14,15]. -
Attention Modulates Maintenance of Representations in Visual Short-Term Memory
Attention Modulates Maintenance of Representations in Visual Short-term Memory Bo-Cheng Kuo, Mark G. Stokes, and Anna Christina Nobre Downloaded from http://mitprc.silverchair.com/jocn/article-pdf/24/1/51/1780230/jocn_a_00087.pdf by MIT Libraries user on 17 May 2021 Abstract ■ Recent studies have shown that selective attention is of consid- retrospective cues during the maintenance period could predict a erable importance for encoding task-relevant items into visual specific item (spatial retrocue) or multiple items (neutral retrocue) short-term memory (VSTM) according to our behavioral goals. thatwouldbeprobedattheendofthememorydelay.Ourresults However, it is not known whether top–down attentional biases revealed that VSTM performance is significantly improved by ori- can continue to operate during the maintenance period of VSTM. enting attention to the location of a task-relevant item. The behav- We used ERPs to investigate this question across two experiments. ioral benefit was accompanied by modulation of neural activity Specifically, we tested whether orienting attention to a given spa- involved in VSTM maintenance. Spatial retrocues reduced the tial location within a VSTM representation resulted in modulation magnitude of the CDA, consistent with a reduction in memory of the contralateral delay activity (CDA), a lateralized ERP marker load. Our results provide direct evidence that top–down control of VSTM maintenance generated when participants selectively modulates neural activity associated with maintenance in VSTM, encode memory -
Dissociable Prior Influences of Signal Probability and Relevance on Visual Contrast Sensitivity
Correction PSYCHOLOGICAL AND COGNITIVE SCIENCES Correction for “Dissociable prior influences of signal probability and relevance on visual contrast sensitivity,” by Valentin Wyart, Anna Christina Nobre, and Christopher Summerfield, which appeared in issue 9, February 28, 2012, of Proc Natl Acad Sci USA (109:3593–3598; first published February 13, 2012; 10.1073/ pnas.1120118109). The authors note that on page 3597, right column, the third equation (for soft threshold nonlinearity) appeared incorrectly. The corrected equation appears below: x Γ½x ¼ x þ α − exp α www.pnas.org/cgi/doi/10.1073/pnas.1204601109 6354 | PNAS | April 17, 2012 | vol. 109 | no. 16 www.pnas.org Downloaded by guest on September 30, 2021 Dissociable prior influences of signal probability and relevance on visual contrast sensitivity Valentin Wyarta,1, Anna Christina Nobrea,b, and Christopher Summerfielda aDepartment of Experimental Psychology, University of Oxford, Oxford OX1 3UD, United Kingdom; and bOxford Centre for Human Brain Activity, Department of Psychiatry, University of Oxford, Warneford Hospital, Oxford OX3 7JX, United Kingdom Edited by Ranulfo Romo, Universidad Nacional Autonoma de Mexico, Mexico City, DF, Mexico, and approved January 18, 2012 (received for review December 7, 2011) According to signal detection theoretical analyses, visual signals increasing the baseline activity of signal-selective units or by occurring at a cued location are detected more accurately, whereas shifting the observer’s decision criterion toward one of the two frequently occurring ones are reported more often but are not better responses. However, the binary classification of stimuli as signal- − distinguished from noise. However, conventional analyses that present (S+) and signal-absent (S ) used by conventional SDT estimate sensitivity and bias by comparing true- and false-positive analyses makes it difficult to arbitrate between these different rates offer limited insights into the mechanisms responsible for these possibilities, for a number of reasons. -
Imagining a Brighter Future the Effect of Positive Imagery Training on Mood, Prospective Mental Imagery and Emotional Bias in O
Author’s Accepted Manuscript Imagining a brighter future: The effect of positive imagery training on mood, prospective mental imagery and emotional bias in older adults Susannah E. Murphy, M. Clare O’Donoghue, Erin H.S. Drazich, Simon E. Blackwell, Anna Christina Nobre, Emily A. Holmes www.elsevier.com/locate/psychres PII: S0165-1781(15)00516-8 DOI: http://dx.doi.org/10.1016/j.psychres.2015.07.059 Reference: PSY9111 To appear in: Psychiatry Research Received date: 17 October 2014 Revised date: 3 July 2015 Accepted date: 19 July 2015 Cite this article as: Susannah E. Murphy, M. Clare O’Donoghue, Erin H.S. Drazich, Simon E. Blackwell, Anna Christina Nobre and Emily A. Holmes, Imagining a brighter future: The effect of positive imagery training on mood, prospective mental imagery and emotional bias in older adults, Psychiatry Research, http://dx.doi.org/10.1016/j.psychres.2015.07.059 This is a PDF file of an unedited manuscript that has been accepted for publication. As a service to our customers we are providing this early version of the manuscript. The manuscript will undergo copyediting, typesetting, and review of the resulting galley proof before it is published in its final citable form. Please note that during the production process errors may be discovered which could affect the content, and all legal disclaimers that apply to the journal pertain. Imagining a brighter future: the effect of positive imagery training on mood, prospective mental imagery and emotional bias in older adults Susannah E. Murphya *, M. Clare O’Donoghuea, Erin H. S. Drazicha, Simon E. -
The Functional Consequences of Social Distraction: Attention And
Cognition 158 (2017) 215–223 Contents lists available at ScienceDirect Cognition journal homepage: www.elsevier.com/locate/COGNIT Original Articles The functional consequences of social distraction: Attention and memory for complex scenes ⇑ Brianna Ruth Doherty a, Eva Zita Patai a,b, Mihaela Duta a, Anna Christina Nobre a,b, Gaia Scerif a, a Department of Experimental Psychology, University of Oxford, Oxford, United Kingdom b Oxford Centre for Human Brain Activity, University of Oxford, Oxford, United Kingdom article info abstract Article history: Cognitive scientists have long proposed that social stimuli attract visual attention even when task irrel- Received 13 November 2015 evant, but the consequences of this privileged status for memory are unknown. To address this, we com- Revised 12 October 2016 bined computational approaches, eye-tracking methodology, and individual-differences measures. Accepted 26 October 2016 Participants searched for targets in scenes containing social or non-social distractors equated for low- level visual salience. Subsequent memory precision for target locations was tested. Individual differences in autistic traits and social anxiety were also measured. Eye-tracking revealed significantly more atten- Keywords: tional capture to social compared to non-social distractors. Critically, memory precision for target loca- Social distraction tions was poorer for social scenes. This effect was moderated by social anxiety, with anxious Visual attention Memory individuals remembering target locations better under conditions -
European Journal of Pragmatism and American Philosophy, IV - 1 | 2012, « Pragmatism and the Social Sciences: a Century of Influences and Interactions, Vol
European Journal of Pragmatism and American Philosophy IV - 1 | 2012 Pragmatism and the Social Sciences: A Century of Influences and Interactions, vol. 2 Roberto Frega and Filipe Careira Da Silva (dir.) Electronic version URL: http://journals.openedition.org/ejpap/758 DOI: 10.4000/ejpap.758 ISSN: 2036-4091 Publisher Associazione Pragma Electronic reference Roberto Frega and Filipe Careira Da Silva (dir.), European Journal of Pragmatism and American Philosophy, IV - 1 | 2012, « Pragmatism and the Social Sciences: A Century of Influences and Interactions, vol. 2 » [Online], Online since 23 July 2012, connection on 23 September 2020. URL : http://journals.openedition.org/ejpap/758 ; DOI : https://doi.org/10.4000/ejpap.758 This text was automatically generated on 23 September 2020. Author retains copyright and grants the European Journal of Pragmatism and American Philosophy right of first publication with the work simultaneously licensed under a Creative Commons Attribution- NonCommercial-NoDerivatives 4.0 International License. 1 TABLE OF CONTENTS Symposia. Pragmatism and the Social Sciences: A Century of Influences and Interactions, vol.2 Pragmatism and the Social Sciences A Century of Influences and Interactions, vol. 2 Roberto Frega and Filipe Carreira da Silva Section I. Classical Pragmatists and Contemporary Sociology Peirce and Iconology Habitus, Embodiment, and the Analogy between Philosophy and Architecture Tullio Viola Experiencing Practical Knowledge Emerging Convergences of Pragmatism and Sociological Practice Theory Tanja Bogusz The Social Scientist, the Public, and the Pragmatist Gaze Exploring the Critical Conditions of Sociological Inquiry Philippe Gonzalez and Laurence Kaufmann Section II. Law, Power, and the Prospects of a Pragmatist Social Theory Naturalistic Values and Progressive Politics A Missing Link Between Pragmatism and Social Theory Christoph Henning American Pragmatism and European Social Theory Holmes, Durkheim, Scheler, and the Sociology of Legal Knowledge Frederic R. -
Extended Cognition and Extended Consciousness∗
Extended Cognition and Extended Consciousness∗ David J. Chalmers It’s a pleasure to contribute this paper to Andy Clark and his Critics. Andy has been an influential figure for me ever since I read his first book Microcognition while I was in graduate school. A few years later I took up my first job as a post-doctoral fellow working with him in the newly initiated Philosophy-Neuroscience-Psychology program at Washington University in St Louis. We had a lot of adventures, but the one that seems to have left the most traces is our joint paper on “The Extended Mind”, which argued that cognitive processes can extend outside the brain and the body to include objects that we interact with in our environment. I recently came across hard copies of two early drafts of “The Extended Mind” in my files. The first draft was by Andy on his own, and is covered in handwritten comments by me. The second draft is a co-authored version with revisions by me, and is covered in handwritten comments by Andy. (I have put these two online at consc.net/papers/e-drafts.html.) There were many further iterations after that. The whole thing is a wonderful record of distributed and extended cognition, involving a complex cognitive process spread between Andy, me, various notes on paper, and computer files. I’m proud to have played a role in constituting this process. That said, I have to acknowledge that as with many cases of distributed cognition, there was an individual at the core who set the whole process into motion and who bears primary responsbility, and that individual was Andy.