A Proposal for a Data-Driven Approach to the Influence of Music
Total Page:16
File Type:pdf, Size:1020Kb
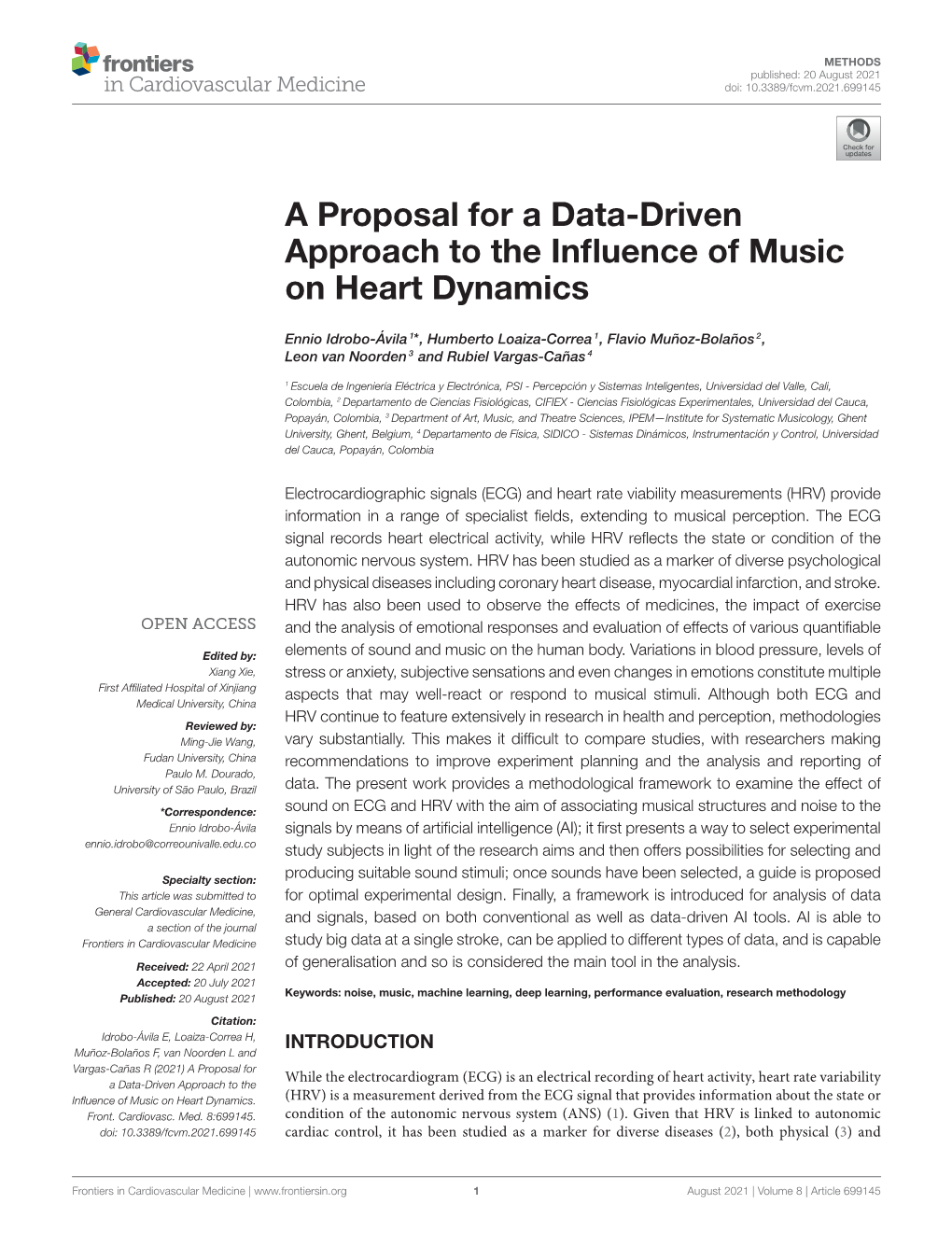
Load more
Recommended publications
-
Grey Noise, Dubai Unit 24, Alserkal Avenue Street 8, Al Quoz 1, Dubai
Grey Noise, Dubai Stéphanie Saadé b. 1983, Lebanon Lives and works between Beirut, Paris and Amsterdam Education and Residencies 2018 - 2019 3 Package Deal, 1 year “Interhistoricity” (Scholarship received from the AmsterdamFonds Voor Kunst and the Bureau Broedplaatsen), In partnership with Museum Van Loon Oude Kerk, Castrum Peregrini and Reinwardt Academy, Amsterdam, Netherlands 2018 Studio Cur’art and Bema, Mexico City and Guadalajara 2017 – 2018 Maison Salvan, Labège, France Villa Empain, Fondation Boghossian, Brussels, Belgium 2015 – 2016 Cité Internationale des Arts, Paris, France (Recipient of the French Institute residency program scholarship) 2014 – 2015 Jan Van Eyck Academie, Maastricht, The Netherlands 2013 PROGR, Bern, Switzerland 2010 - 2012 China Academy of Arts, Hangzhou, China (State-funded Scholarship for post- graduate program) 2005 – 2010 École Nationale Supérieure des Beaux-Arts, Paris, France (Diplôme National Supérieur d’Arts Plastiques) Awards 2018 Recipient of the 2018 NADA MIAMI Artadia Art Award Selected Solo Exhibitions 2020 (Upcoming) AKINCI Gallery, Amsterdam, Netherlands 2019 The Travels of Here and Now, Museum Van Loon, Amsterdam, Netherlands with the support of Amsterdams fonds voor de Kunst The Encounter of the First and Last Particles of Dust, Grey Noise, Dubai L’espace De 70 Jours, La Scep, Marseille, France Unit 24, Alserkal Avenue Street 8, Al Quoz 1, Dubai, UAE / T +971 4 3790764 F +971 4 3790769 / [email protected] / www.greynoise.org Grey Noise, Dubai 2018 Solo Presentation at Nada Miami with Counter -
ACCESSORIES and BATTERIES MTX Professional Series Portable Two-Way Radios MTX Series
ACCESSORIES AND BATTERIES MTX Professional Series Portable Two-Way Radios MTX Series Motorola Original® Accessories let you make the most of your MTX Professional Series radio’s capabilities. You made a sound business decision when you chose your Motorola MTX Professional Series Two-Way radio. When you choose performance-matched Motorola Original® accessories, you’re making another good call. That’s because every Motorola Original® accessory is designed, built and rigorously tested to the same quality standards as Motorola radios. So you can be sure that each will be ready to do its job, time after time — helping to increase your productivity. After all, if you take a chance on a mismatched battery, a flimsy headset, or an ill-fitting carry case, your radio may not perform at the moment you have to use it. We’re pleased to bring you this collection of proven Motorola Original® accessories for your important business communication needs. You’ll find remote speaker microphones for more convenient control. Discreet earpiece systems to help ensure privacy and aid surveillance. Carry cases for ease when on the move. Headsets for hands-free operation. Premium batteries to extend work time, and more. They’re all ready to help you work smarter, harder and with greater confidence. Motorola MTX Professional Series radios keep you connected with a wider calling range, faster channel access, greater privacy and higher user and talkgroup capacity. MTX PROFESSIONAL SERIES PORTABLE RADIOS TM Intelligent radio so advanced, it practically thinks for you. MTX Series Portable MTX850 Two-Way Radios are the smart choice for your business - delivering everything you need for great business communication. -
Portable Radio: Headsets XTS 3000, XTS 3500, XTS 5000, XTS 1500, XTS 2500, MT 1500, and PR1500
portable radio: headsets XTS 3000, XTS 3500, XTS 5000, XTS 1500, XTS 2500, MT 1500, and PR1500 Noise-Cancelling Headsets with Boom Microphone Ideal for two-way communication in extremely noisy environments. Dual-Muff, tactical headsets RMN4052A include 2 microphones on outside of earcups which reproduce ambient sound back into headset. Harmful sounds are suppressed to safe levels and low sounds are amplified up to 5 times original level, but never more than 82dB. Has on/off volume control on earcups. Easily replaceable ear seals are filled with a liquid and foam combination to provide excellent sealing and comfort. 3-foot coiled cord assembly included. Adapter Cable RKN4095A required. RMN4052A Tactical Headband Style Headset. Grey, Noise reduction = 24dB. RMN4053A Tactical Hardhat Mount Headset. Grey, Noise reduction = 22dB. RKN4095A Adapter Cable with In-Line Push-to-Talk For use with RMN4051B/RMN4052A/RMN4053A. REPLACEMENTEARSEALS RLN4923B Replacement Earseals for RMN4051B/RMN4052A/RMN4053A Includes 2 snap-in earseals. Hardhat Mount Headset With Noise-Cancelling Boom Microphone RMN4051B Ideal for two-way communication in high-noise environments, this headset mounts easily to hard- hats with slots, or can be worn alone. Easily replaceable ear seals are filled with a liquid and foam combination to provide excellent sealing and comfort. 3-foot coiled cord assembly included. Noise reduction of 22dB. Separate In-line PTT Adapter Cable RKN4095A required. Hardhat not included. RMN4051B* Two-way, Hardhat Mount Headset with Noise-Cancelling Boom Microphone. Black. RKN4095A Adapter cable with In-Line PTT. For use with RMN4051B/RMN4052A/RMN4053A. Medium Weight Headsets Medium weight headsets offer high-clarity sound, with the additional hearing protection necessary to provide consistent, clear, two-way radio communication in harsh, noisy environments or situations. -
LPM Woofer Sample Report
HTML Report - Klippel GmbH Page 1 of 10 KLIPPEL ANALYZER SYSTEM Report with comments Linear Parameter Measurement (LPM) Driver Name: w2017 midrange Driver Comment: Measurement: LPM south free air Measurement Measureslinear parameters of woofers. Comment : Driver connected to output SPEAKER 2. Overview The Introductory Report illustrates the powerful features of the Klippel Analyzer module dedicated to the measurement of the linear speaker parameters. Additional comments are added to the results of a practical measurement applied to the speaker specified above. After presenting short information to the measurement technique the report comprises the following results • linear speaker parameters + mechanical creep factor • electrical impedance response • mechanical transfer response (voltage to voice coil displacement) • acoustical transfer response (voltage to SPL) • time signals of the speaker variables during measurement • spectra of the speaker variables (fundamental, distortion, noise floor) • summary on the signal statistics (peak value, SNR, headroom,…). MEASUREMENT TECHNIQUE The measurement module identifies the electrical and mechanical parameters (Thiele-Small parameters) of electro-dynamical transducers. The electrical parameters are determined by measuring terminal voltage u(t) and current i(t) and exploiting the electrical impedance Z(f)=U(f)/I(f). The mechanical parameters can either be identified using a laser displacement sensor or by a second (comparative) measurement where the driver is placed in a test enclosure or an additional mass is attached to it. For the first method the displacement of the driver diaphragm is measured in order to exploit the function Hx(f)= X(f)/U(f). So the identification dispenses with a second measurement and avoids problems due to leakage of the test enclosure or mass attachment. -
An Introduction to Opensound Navigator™
WHITEPAPER 2016 An introduction to OpenSound Navigator™ HIGHLIGHTS OpenSound Navigator™ is a new speech-enhancement algorithm that preserves speech and reduces noise in complex environments. It replaces and exceeds the role of conventional directionality and noise reduction algorithms. Key technical advances in OpenSound Navigator: • Holistic system that handles all acoustical environments from the quietest to the noisiest and adapts its response to the sound preference of the user – no modes or mode switch. • Integrated directional and noise reduction action – rebalance the sound scene, preserve speech in all directions, and selectively reduce noise. • Two-microphone noise estimate for a “spatially-informed” estimate of the environmental noise, enabling fast and accurate noise reduction. The speed and accuracy of the algorithm enables selective noise reduction without isolating the talker of interest, opening up new possibilities for many audiological benefits. Nicolas Le Goff,Ph.D., Senior Research Audiologist, Oticon A/S Jesper Jensen, Ph.D., Senior Scientist, Oticon A/S; Professor, Aalborg University Michael Syskind Pedersen, Ph.D., Lead Developer, Oticon A/S Susanna Løve Callaway, Au.D., Clinical Research Audiologist, Oticon A/S PAGE 2 WHITEPAPER – 2016 – OPENSOUND NAVIGATOR The daily challenge of communication To make sense of a complex acoustical mixture, the brain in noise organizes the sound entering the ears into different Sounds occur around us virtually all the time and they auditory “objects” that can then be focused on or put in start and stop unpredictably. We constantly monitor the background. The formation of these auditory objects these changes and choose to interact with some of the happens by assembling sound elements that have similar sounds, for instance, when engaging in a conversation features (e.g., Bregman, 1990). -
Exploring the Role of Language in Two Systems for Categorization Kayleigh Ryherd University of Connecticut - Storrs, [email protected]
University of Connecticut OpenCommons@UConn Doctoral Dissertations University of Connecticut Graduate School 4-26-2019 Exploring the Role of Language in Two Systems for Categorization Kayleigh Ryherd University of Connecticut - Storrs, [email protected] Follow this and additional works at: https://opencommons.uconn.edu/dissertations Recommended Citation Ryherd, Kayleigh, "Exploring the Role of Language in Two Systems for Categorization" (2019). Doctoral Dissertations. 2163. https://opencommons.uconn.edu/dissertations/2163 Exploring the Role of Language in Two Systems for Categorization Kayleigh Ryherd, PhD University of Connecticut, 2019 Multiple theories of category learning converge on the idea that there are two systems for categorization, each designed to process different types of category structures. The associative system learns categories that have probabilistic boundaries and multiple overlapping features through iterative association of features and feedback. The hypothesis-testing system learns rule-based categories through explicit testing of hy- potheses about category boundaries. Prior research suggests that language resources are necessary for the hypothesis-testing system but not for the associative system. However, other research emphasizes the role of verbal labels in learning the probabilistic similarity-based categories best learned by the associative system. This suggests that language may be relevant for the associative system in a different way than it is relevant for the hypothesis-testing system. Thus, this study investigated the ways in which language plays a role in the two systems for category learning. In the first experiment, I tested whether language is related to an individual’s ability to switch between the associative and hypothesis-testing systems. I found that participants showed remarkable ability to switch between systems regardless of their language ability. -
Predictive Decoding of Neural Data Yaroslav O
New Jersey Institute of Technology Digital Commons @ NJIT Dissertations Theses and Dissertations Spring 2009 Predictive decoding of neural data Yaroslav O. Halchenko New Jersey Institute of Technology Follow this and additional works at: https://digitalcommons.njit.edu/dissertations Part of the Computer Sciences Commons Recommended Citation Halchenko, Yaroslav O., "Predictive decoding of neural data" (2009). Dissertations. 901. https://digitalcommons.njit.edu/dissertations/901 This Dissertation is brought to you for free and open access by the Theses and Dissertations at Digital Commons @ NJIT. It has been accepted for inclusion in Dissertations by an authorized administrator of Digital Commons @ NJIT. For more information, please contact [email protected]. Cprht Wrnn & trtn h prht l f th Untd Stt (tl , Untd Stt Cd vrn th n f phtp r thr rprdtn f prhtd trl. Undr rtn ndtn pfd n th l, lbrr nd rhv r thrzd t frnh phtp r thr rprdtn. On f th pfd ndtn tht th phtp r rprdtn nt t b “d fr n prp thr thn prvt td, hlrhp, r rrh. If , r rt fr, r ltr , phtp r rprdtn fr prp n x f “fr tht r b lbl fr prht nfrnnt, h ntttn rrv th rht t rf t pt pn rdr f, n t jdnt, flfllnt f th rdr ld nvlv vltn f prht l. l t: h thr rtn th prht hl th r Inttt f hnl rrv th rht t dtrbt th th r drttn rntn nt: If d nt h t prnt th p, thn lt “ fr: frt p t: lt p n th prnt dl rn h n tn lbrr h rvd f th prnl nfrtn nd ll ntr fr th pprvl p nd brphl th f th nd drttn n rdr t prtt th dntt f I rdt nd flt. -
ML Cheatsheet Documentation
ML Cheatsheet Documentation Team Sep 02, 2021 Basics 1 Linear Regression 3 2 Gradient Descent 21 3 Logistic Regression 25 4 Glossary 39 5 Calculus 45 6 Linear Algebra 57 7 Probability 67 8 Statistics 69 9 Notation 71 10 Concepts 75 11 Forwardpropagation 81 12 Backpropagation 91 13 Activation Functions 97 14 Layers 105 15 Loss Functions 117 16 Optimizers 121 17 Regularization 127 18 Architectures 137 19 Classification Algorithms 151 20 Clustering Algorithms 157 i 21 Regression Algorithms 159 22 Reinforcement Learning 161 23 Datasets 165 24 Libraries 181 25 Papers 211 26 Other Content 217 27 Contribute 223 ii ML Cheatsheet Documentation Brief visual explanations of machine learning concepts with diagrams, code examples and links to resources for learning more. Warning: This document is under early stage development. If you find errors, please raise an issue or contribute a better definition! Basics 1 ML Cheatsheet Documentation 2 Basics CHAPTER 1 Linear Regression • Introduction • Simple regression – Making predictions – Cost function – Gradient descent – Training – Model evaluation – Summary • Multivariable regression – Growing complexity – Normalization – Making predictions – Initialize weights – Cost function – Gradient descent – Simplifying with matrices – Bias term – Model evaluation 3 ML Cheatsheet Documentation 1.1 Introduction Linear Regression is a supervised machine learning algorithm where the predicted output is continuous and has a constant slope. It’s used to predict values within a continuous range, (e.g. sales, price) rather than trying to classify them into categories (e.g. cat, dog). There are two main types: Simple regression Simple linear regression uses traditional slope-intercept form, where m and b are the variables our algorithm will try to “learn” to produce the most accurate predictions. -
Claus-Christian Carbon
curriculum vitae Claus-Christian Carbon 25-May-13 1. Personal Data Name: Claus-Christian CARBON Date of Birth: 23 March 1971 Place of Birth: Schweinfurt, Federal Republic of Germany Profession: Full Professor Phone (office): +49 (951) 863 1860 E-mail: [email protected] Nationality: German Education (short track): Dipl.-Psych. (Psychology), 1998 MA (Philosophy), 1999 PhD (Psychology), 2003 Habilitation (Psychology), 2006 2. Education 03/2006 Habilitation (PD/Dr. habil) in Psychology, University of Vienna; title of habilitation thesis: “On the processing and representation of complex visual objects”; exhaustive venia docendi for all scientific areas of Psychology 02/2003 Doctorate (PhD, Dr.phil.) in Psychology, Freie Universität Berlin, “magna cum laude”; title of thesis: “Face Processing: Early processing in the recognition of faces” 10/1999-09/2000 Undergraduate student of economics (BWL), Fernuniversität Hagen 12/1999 Magister (MA) in Philosophy, University of Trier (1.2 – “very good”); title of thesis: “Connectionism: may connectionist systems develop form of consciousness?” 11/1998 Dipl.-Psych. (Diplompsychologe/MSc) in Psychology, University of Trier (1.2 – “very good”); title of thesis: “Influences of emotional and configural variables on the processing of spatial information” 10/1994-09/1997 Undergraduate student of art history and politics, University of Trier 10/1991-03/1992 Undergraduate student for the studies of traffic system (Verkehrswesen), University of Technology, Berlin Claus-Christian Carbon curriculum vitae - 2/21 - 07/1990-12/1990 Training and qualification for a national paramedic (Bavarian Red Cross) 06/1990 Abitur (GCSE) with focus on mathematics and physics, Dominikus Zimmermann Gymnasium, Landsberg am Lech Summer/Spring Schools 03/2008 EOG and Eyetracking in Psychological Research: Workshop of the University of Cologne (Prof. -
Neural Processing of Facial Expressions As Modulators of Communicative Intention Facial Expression in Communicative Intention
Neural processing of facial expressions as modulators of communicative intention Facial expression in communicative intention Rasgado-Toledo Jalil1, Valles-Capetillo Elizabeth1, Giudicessi Averi2, Giordano Magda1*. 1Departament of Behavioral and Cognitive Neurobiology, Instituto de Neurobiología, Universidad Nacional Autónoma de México, Blvd. Juriquilla 3001, Juriquilla, Querétaro, 76230 México 2 Department of Psychiatry, University of California, San Diego 9500 Gilman Drive La Jolla, CA * Corresponding author: E-mail address: [email protected] (Giordano, Magda) Number of pages: 31 Number of figures: 8 Number of tables: 1 Number of words: Abstract: 250 Introduction: 621 Discussion: 1499 Conflict of Interest The authors declare no competing financial interests Acknowledgments We thank Drs. Erick Pasaye, Leopoldo González-Santos, Juan Ortiz, and the personnel at the National Laboratory for Magnetic Resonance Imaging (LANIREM UNAM), for their valuable assistance. This work received support from Luis Aguilar, Alejandro De León, Carlos Flores, and Jair García of the Laboratorio Nacional de Visualización Científica Avanzada (LAVIS). Additional thanks to Azalea Reyes-Aguilar for the valuable feedback for the entire study process. Significance Statement In the present study, we tested whether previous observation of a facial expression associated with an emotion can modify the interpretation of a speech act that follows a facial expression; thus providing empirical evidence that facial expression impacts communication. Our results could help to understand how we communicate and the aspects of communication that are necessary to comprehend pragmatic forms of language as speech acts. We applied both multivariate and univariate analysis models to compare brain structures involved in speech acts comprehension, and found that the pattern of hemodynamic response of the frontal gyrus and medial prefrontal cortex can be used to decode classification decisions. -
Psychology Software for Python Release 3.0.2
PsychoPy - Psychology software for Python Release 3.0.2 Jonathan Peirce Jan 29, 2019 Contents 1 About PsychoPy 1 2 General issues 3 3 Installation 29 4 Manual install 31 5 Getting Started 35 6 Builder 43 7 Coder 75 8 Running studies online 95 9 Reference Manual (API) 103 10 Troubleshooting 325 11 Recipes (“How-to”s) 329 12 Frequently Asked Questions (FAQs) 341 13 Resources (e.g. for teaching) 343 14 For Developers 345 15 PsychoPy Experiment file format (.psyexp) 359 Python Module Index 363 i ii CHAPTER 1 About PsychoPy 1.1 Citing PsychoPy If you use this software, please cite one of the publications that describe it. • Peirce, J. W., & MacAskill, M. R. (2018). Building Experiments in PsychoPy. London: Sage. • Peirce J. W. (2009). Generating stimuli for neuroscience using PsychoPy. Frontiers in Neuroinformatics, 2 (10), 1-8. doi:10.3389/neuro.11.010.2008 • Peirce, J. W. (2007). PsychoPy - Psychophysics software in Python. Journal of Neuroscience Methods, 162 (1-2):8-13 doi:10.1016/j.jneumeth.2006.11.017 Citing these papers gives the reviewer/reader of your study information about how the system works and it attributes some credit for its original creation. Academic assessment (whether for promotion or even getting appointed to a job in the first place) prioritises publications over making useful tools for others. Citations provide a way for the developers to justify their continued involvement in the development of the package. 1 PsychoPy - Psychology software for Python, Release 3.0.2 2 Chapter 1. About PsychoPy CHAPTER 2 General issues These are issues that users should be aware of, whether they are using Builder or Coder views. -
D2.3 Data Collection Protocol
Ref. Ares(2019)4156542 - 01/07/2019 D2.3 - DATA COLLECTION PROTOCOL Smart Age-friendly Living and DOCUMENT ID - TYPE: D2.3 (REPORT) PROJECT TITLE: Working Environment DELIVERABLE LEADER: RRD GRANT AGREEMENT Nº: 826343 (H2020-SC1-DTH-2018-1) DUE DATE: 30/06/2019 CONTRACT START DATE: 1 January 2019 DELIVERY DATE: 30/06/2019 CONTRACT DURATION: 36 Months DISSEMINATION LEVEL: Public (PU) PROJECT COORDINATOR: BYTE S.A. STATUS - VERSION: Final – v1.0 (Page intentionally blanked) D2.3 – DATA COLLECTION PROTOCOL | Page 3 AUTHORS - CONTRIBUTORS Name Organization Miriam Cabrita Roessingh Research and Development (RRD) Mattienne van der Kamp Roessingh Research and Development (RRD) Harm op den Akker Roessingh Research and Development (RRD) Lex van Velsen Roessingh Research and Development (RRD) Willeke van Staalduinen Cáritas Diocesana de Coimbra (CDC) Natália Machado Cáritas Diocesana de Coimbra (CDC) Tom Thomsen Arhus Kommune (CAT) Otilia Kocsis University of Patras (UPAT) João Quintas Instituto Pedro Nunes (IPN) André Pardal Instituto Pedro Nunes (IPN) Hugo Marcos Instituto Pedro Nunes (IPN) Dimitrios Amaxilatis Spark Works ITC (SPARKS) Nikos Tsironis Spark Works ITC (SPARKS) PEER REVIEWERS Name Organization Carina Dantas Cáritas Diocesana de Coimbra (CDC) Otilia Kocsis University of Patras (UPAT) Nikos Fazakis University of Patras (UPAT) Charalampos Vassiliou BYTE D2.3 – DATA COLLECTION PROTOCOL | Page 4 REVISION HISTORY Version Date Author/Organisation Modifications 0.1 08.04.2019 RRD Table of Contents Revised Table of Contents 0.2 23.04.2019