D Disse Erta Ation N
Total Page:16
File Type:pdf, Size:1020Kb
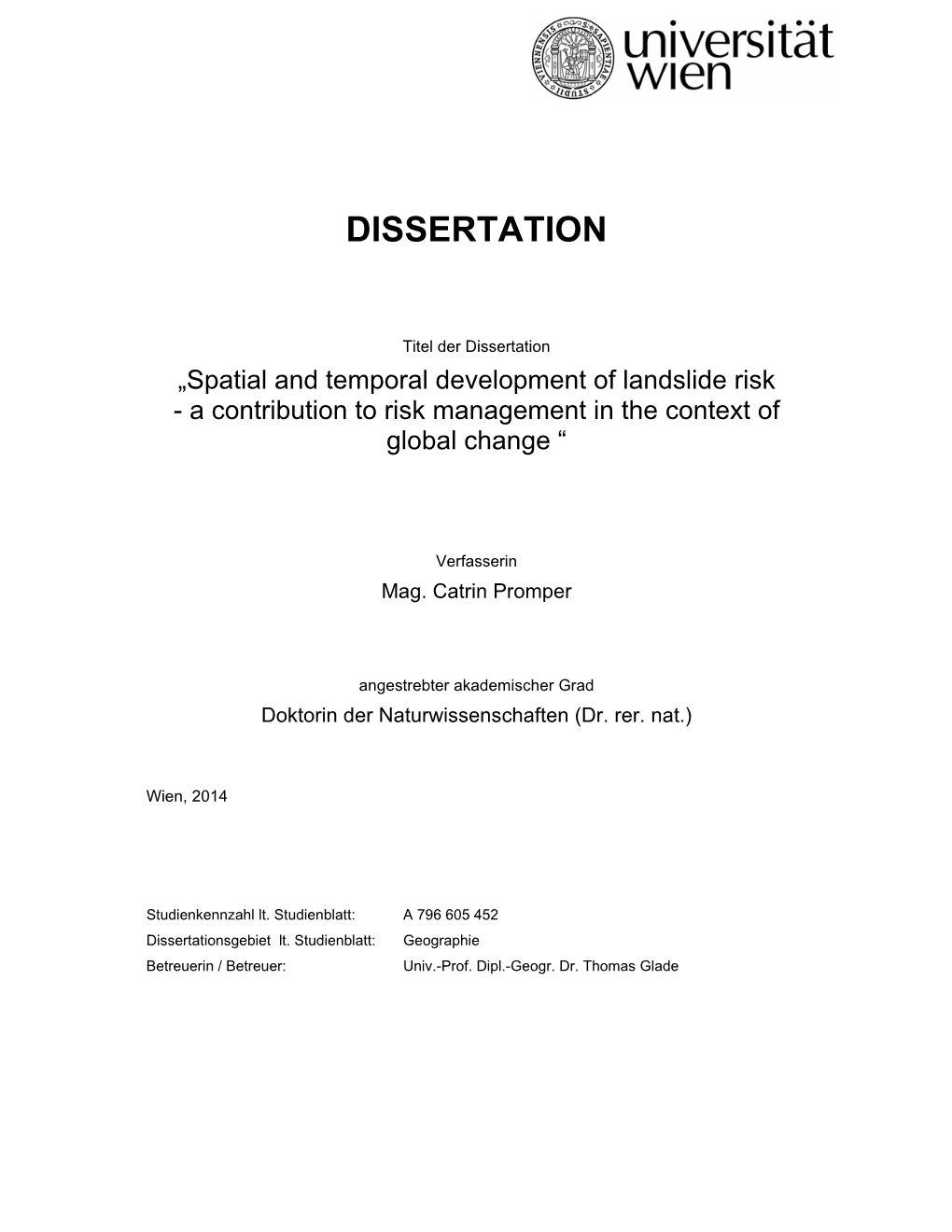
Load more
Recommended publications
-
For People Who Love Early Maps Early Love Who People for 143 No
143 INTERNATIONAL MAP COLLECTORS’ SOCIETY WINTER 2015 No.143 FOR PEOPLE WHO LOVE EARLY MAPS JOURNAL ADVERTISING Index of Advertisers 4 issues per year Colour B&W Altea Gallery 57 Full page (same copy) £950 £680 Half page (same copy) £630 £450 Art Aeri 47 Quarter page (same copy) £365 £270 Antiquariaat Sanderus 36 For a single issue 22 Full page £380 £275 Barron Maps Half page £255 £185 Barry Lawrence Ruderman 4 Quarter page £150 £110 Flyer insert (A5 double-sided) £325 £300 Collecting Old Maps 10 Clive A Burden 2 Advertisement formats for print Daniel Crouch Rare Books 58 We can accept advertisements as print ready artwork saved as tiff, high quality jpegs or pdf files. Dominic Winter 36 It is important to be aware that artwork and files Frame 22 that have been prepared for the web are not of sufficient quality for print. Full artwork Gonzalo Fernández Pontes 15 specifications are available on request. Jonathan Potter 42 Advertisement sizes Kenneth Nebenzahl Inc. 47 Please note recommended image dimensions below: Kunstantiquariat Monika Schmidt 47 Full page advertisements should be 216 mm high Librairie Le Bail 35 x 158 mm wide and 300–400 ppi at this size. Loeb-Larocque 35 Half page advertisements are landscape and 105 mm high x 158 mm wide and 300–400 ppi at this size. The Map House inside front cover Quarter page advertisements are portrait and are Martayan Lan outside back cover 105 mm high x 76 mm wide and 300–400 ppi at this size. Mostly Maps 15 Murray Hudson 10 IMCoS Website Web Banner £160* The Observatory 35 * Those who advertise in the Journal may have a web banner on the IMCoS website for this annual rate. -
James Hutton's Reputation Among Geologists in the Late Eighteenth and Nineteenth Centuries
The Geological Society of America Memoir 216 Revising the Revisions: James Hutton’s Reputation among Geologists in the Late Eighteenth and Nineteenth Centuries A. M. Celâl Şengör* İTÜ Avrasya Yerbilimleri Enstitüsü ve Maden Fakültesi, Jeoloji Bölümü, Ayazağa 34469 İstanbul, Turkey ABSTRACT A recent fad in the historiography of geology is to consider the Scottish polymath James Hutton’s Theory of the Earth the last of the “theories of the earth” genre of publications that had begun developing in the seventeenth century and to regard it as something behind the times already in the late eighteenth century and which was subsequently remembered only because some later geologists, particularly Hutton’s countryman Sir Archibald Geikie, found it convenient to represent it as a precursor of the prevailing opinions of the day. By contrast, the available documentation, pub- lished and unpublished, shows that Hutton’s theory was considered as something completely new by his contemporaries, very different from anything that preceded it, whether they agreed with him or not, and that it was widely discussed both in his own country and abroad—from St. Petersburg through Europe to New York. By the end of the third decade in the nineteenth century, many very respectable geologists began seeing in him “the father of modern geology” even before Sir Archibald was born (in 1835). Before long, even popular books on geology and general encyclopedias began spreading the same conviction. A review of the geological literature of the late eighteenth and the nineteenth centuries shows that Hutton was not only remembered, but his ideas were in fact considered part of the current science and discussed accord- ingly. -
Doctor Werner Schwerdtfeger a Short Biography
Doctor Werner Schwerdtfeger A Short Biography The Wetterflieger Project The Wetterflieger Project – Werner Schwerdtfeger Table of Contents Preface .......................................................................................................................................................... 1 Early Years ..................................................................................................................................................... 2 Beginnings ................................................................................................................................................. 2 Researcher, Aeronaut, Teacher ................................................................................................................ 4 Warrior Years ................................................................................................................................................ 7 Weather Reconnaissance Meteorologist .................................................................................................. 9 Zentral Wetterdienst Gruppe ................................................................................................................. 13 Chef, Zentral Wetterdienst Gruppe .................................................................................................... 14 Normandy and the D-Day Forecast ..................................................................................................... 16 Ardennes Counteroffensive Forecast ................................................................................................ -
Masters Thesis
Distributed modelling of the energy and mass-balance at the surface of Langenferner, Ortles-Cevedale Group/Italy Diploma Thesis submitted to the Faculty of Geo- and Atmospheric Science University of Innsbruck presented by: Stephan Peter Galos supervised by: Priv.-Doz. Dr. Thomas Mölg Innsbruck, September 2010 für Peter... Abstract In order to study the spatial distribution of surface mass balance and the related surface energy fluxes of an alpine glacier, a physically based 2D-model was applied to Langenferner, Ortles-Cevedale Group/Northern Italy for the period 2004-2008. The model was driven by meteorological data from six permanent automatic weather stations in the vicinity of the glacier. Meteorological data from the stations which are maintained by the Hydrographic Office of the Autonomous Province of Bolzano were extrapolated to the glacier surface using simple transfer functions. The energy and mass balance model was optimized using data from an automatic weather station which was temporarily installed nearby the glacier. To validate the results, the model output was compared to stake measurements and snow probing data from the glacier. Modelled annual mean specific mass balance showed a sufficient accordance to the measurements at Langenferner except for the hydrological year 2007/2008. The considerable underestimation of melt ablation in this year could be attributed to deficiencies in the employed albedo parameterization. Model results revealed that the spatial distribution of surface mass balance at Langenferner is strongly dependent on shortwave net radiation while other surface energy fluxes showed less influence on the spatial pattern. In order to test the sensitivity of the model to changes in selected model parameters as well as the meteorological input data, a series of sensitivity tests were performed. -
Przegląd Geograficzny T. 82 Z. 3 (2010) Rozwój Geografii Fizycznej
S P R A W O Z D A N I A PRZEGLĄD GEOGRAFICZNY 2010, 82, 3, s. 455–474 Rozwój geografii fizycznej na Uniwersytecie w Wiedniu Development of physical geography at Vienna University JAN SZUPRYCZYŃSKI Instytut Geografii i Przestrzennego Zagospodarowania im. S. Leszczyckiego PAN, 87-100 Toruń, ul. Kopernika 19; [email protected] Zarys treści. Przedstawiony został rozwój geografii fizycznej na Uniwersytecie w Wiedniu, od powołania pierwszej katedry w 1851 roku aż do chwili obecnej. Scharakteryzowano sylwetki naukowe wszystkich kierowników katedr. Słowa kluczowe: Austria, Uniwersytet Wiedeński, F.F. Simony, A. Penck, E. Brückner, F. Machatschek, J. Sölch, H. Spreitzer, J. Fink, H. Fischer. Wprowadzenie Uniwersytet w Wiedniu powstał w 1365 r. Jest zatem o rok młodszy od Uni- wersytetu w Krakowie. W roku 1894 w Austrii przeprowadzono ważną i głęboką reformę szkolnictwa. Reforma ta objęła zarówno zakres programów nauczania, jak i reorganizację struktury szkolnictwa, w tym szeroką rozbudowę kierunków studiów uniwersy- teckich (Bernleithner, 1965; Fischer, 1995). W wyniku tych reform utworzo- no również Austriacką Akademię Nauk. Wśród 40 powołanych członków było dwóch geografów: Adrian Edler von Balbi i Carl Freiherr von Hügel. Ten drugi został pierwszym prezydentem Akademii (Lichtenberger, 1988). Reformatorski trend w szkolnictwie wykorzystał Friedrich Simony – kustosz Muzeum Przyrodniczego w Klagenfurcie (Klagenfurter Naturhistoriche Museum) i wystąpił z propozycją utworzenia geografii na Uniwersytecie Wie- deńskim. W owym czasie miał on już poważne osiągnięcia w badaniach geo- graficznych Alp, głównie w zakresie geomorfologii i limnologii. Pierwszym pro- fesorem w zakresie geografii przy nowo utworzonej katedrze geografii w 1851 r. został właśnie Friedrich Simony (Bernleithner, 1963; Fischer, 1993). Simony http://rcin.org.pl 456 Jan Szupryczyński na szeroką skalę rozwinął badania środowiskowe, był też wybitnym kartografem i utalentowanym malarzem (Kretschmer, 1996). -
Dialektik Der Erschließung: the German–Austrian Alps Between Exploration and Exploitation
humanities Article Dialektik der Erschließung: The German–Austrian Alps between Exploration and Exploitation Sean Ireton School of Languages, Literatures, and Cultures, University of Missouri, Columbia, MO 65211, USA; [email protected] Abstract: Focusing on the so-called Nördliche Kalkalpen or Northern Limestone Alps of Germany and Austria, I will discuss how human interaction with these mountains during the age of the Anthropocene shifts from scientific and athletic exploration to commercial and industrial exploitation. More specifically, I will examine travel narratives by the nineteenth-century mountaineers Friedrich Simony and Hermann von Barth, juxtaposing their respective experiences in diverse Alpine subranges with the environmental history of those regions. This juxtaposition harbors a deeper paradox, one that can be formulated as follows: Whereas Simony and Barth both rank as historically important Erschließer of the German and Austrian Alps, having explored their crags and glaciers in search of somatic adventure and geoscientific knowledge, these very sites of rock and ice were about to become so erschlossen by modernized tourism that one wonders where the precise boundaries between individual-based discovery and technology-driven development lie. In other words, during the nineteenth century a kind of Dialektik der Erschließung (a variation on Horkheimer and Adorno’s Dialektik der Aufklärung) manifests itself in the increasing anthropogenic alteration of the Alps. Keywords: Anthropocene; Alps; Friedrich Simony; Hermann von Barth 1. Introduction Citation: Ireton, Sean. 2021. Dialektik der Erschließung: The German– In the original version of Das abenteuerliche Herz from 1929, Ernst Jünger reminisces on Austrian Alps between Exploration his ascent of the Zugspitze (2962 m/9718 ft.), Germany’s highest mountain, in the following and Exploitation. -
Worth Knowing
worth knowing 2018 Activity Report Hohe Tauern National Park Official notification. Delivered by Post.at www.hohetauern.at 1 Contents Photo: © Roman Türk CALOPLACA ISIDIIGERA Lichens are a group of organisms that is generally overlooked. However, these symbiotic beings of fungi and algae characterise the outward appearance of the vegetation in many biotopes, especially in the semi-natural areas of the Hohe Tauern National Park. In the scientific publications of the Hohe Tauern National Park, in the volume dedicated to lichens, 200 of the most important species are portrayed along with an introduction to the diversity of lichen shapes and life. Legal notice: (Source: Flechten. Wissenschaftliche Schriften.2016. Hohe Tauern National Park Council Secretariat. Sold in bookshops by: Tyrolia-Verlag) Media proprietor, editor, publisher: Kärntner Nationalparkfonds Hohe Tauern Döllach 14, 9843 Grosskirchheim Salzburger Nationalparkfonds Hohe Tauern Gerlos Strasse 18, 5730 Mittersill Tiroler Nationalparkfonds Hohe Tauern Kirchplatz 2, 9971 Matrei in Osttirol Table of contents Verein des Sekretariates des Nationalparkrates Hohe Tauern Kirchplatz 2, 9971 Matrei in Osttirol Editorial staff and responsible for the content: National Park Directors: Mag. Peter Rupitsch, DI Hermann Stotter, DI Wolfgang Urban, MBA 4 Preface 48 Alpine partnerships Project management and coordination: Mag.a Helene Mattersberger 6 Data & facts 50 Public relations Cover photo: Grosser Geiger and Obersulzbachkees glacier © NPHT/Tobias 8 Overview 52 Tourism Kaser 14 Nationalparks Austria 54 Association of the Friends Design: vorauerfriends communications gmbh, Thalheim a Graphic design: 08/16 grafik, mag. eva scheidweiler, Lienz – Salzburg 17 International matters 57 Organisation Printed by: Oberdruck GmbH, Stribach 18 Natural resource management 64 Budget 24 Science & research 65 Outlook Legal notice 32 Preservation of the cultural landscape 66 Contacts Although every care has been taken, printing errors and mistakes cannot be ruled out. -
Geography in Austria
Belgeo Revue belge de géographie 1 | 2004 Special issue : 30th International Geographical Congress Geography in Austria Heinz Faßmann Electronic version URL: http://journals.openedition.org/belgeo/10056 DOI: 10.4000/belgeo.10056 ISSN: 2294-9135 Publisher: National Committee of Geography of Belgium, Société Royale Belge de Géographie Printed version Date of publication: 31 March 2004 Number of pages: 17-34 ISSN: 1377-2368 Electronic reference Heinz Faßmann, « Geography in Austria », Belgeo [Online], 1 | 2004, Online since 17 October 2013, connection on 10 December 2020. URL : http://journals.openedition.org/belgeo/10056 ; DOI : https:// doi.org/10.4000/belgeo.10056 This text was automatically generated on 10 December 2020. Belgeo est mis à disposition selon les termes de la licence Creative Commons Attribution 4.0 International. Geography in Austria 1 Geography in Austria1 Heinz Faßmann Introduction 1 In Austria, geography is a well-established discipline. The first chair at a university was founded in 1851, and the Austrian Geographical Society came into existence in 1856. During the 19th century geography played a major role for the general public. Both the exploration of the world and the scientific explanation of landscape structures were fundamental objectives then. The discipline’s outlook was broad and served as a sort of umbrella for developing earth sciences. By now, more than 150 years later, foci have changed in many ways. All parts of the world have been explored, the formation and structures of “landscapes” can be explained, and many formerly included disciplines became independent. Nevertheless, geography as one of the last broad and integrative disciplines is still thriving, well institutionalized and highly relevant for certain issues in the public sphere. -
Helfricht Kay 2009 Dipl.Pdf
Veränderungen des Massenhaushaltes am Hallstätter Gletscher seit 1856 Diplomarbeit eingereicht am INSTITUT FÜR METEOROLOGIE UND GEOPHYSIK, LEOPOLD-FRANZENS-UNIVERSITÄT INNSBRUCK zur Erlangung des akademischen Grades MAGISTER DER NATURWISSENSCHAFTEN KAY HELFRICHT NOVEMBER 2009 "...Es ist eine bekannte Thatsache, dass, wie fast alle Wissenschaften, so insbesondere die naturhistorischen Disciplinen und die mit ihnen vielfach verknüpfte Erdkunde während der letzten Deciennien sich in einer Weise erweitert und vertieft haben, wie dies wohl kaum in einem zweiten gleich langem Zeitraume der vergangenen Jahrhunderte der Fall gewesen sein dürfte. Der Grund dieses beschleunigten Fortschrittes ist wohl zunächst darin zu suchen, dass die moderne Forschung sich immer mehr dem einzelnen, enger begrenzten zuwendet, dasselbe bis ins äußerste Detail verfolgt und erst aus den auf solchem Wege gewonnenen Erkenntnissen allgemeinere Schlüsse zieht." (Friedrich Simony 1895) i ii Blick vom Schöberl auf den Hallstätter Gletscher am 13. Juli 2008. iii iv v Abstract Hallstätter Glacier is the northernmost glacier of Austria. Appendant to the nor- thern Limestone Alps, the glacier is located at 47◦28’50“ N, 13◦36’50“ E in the Dachstein-region. At the same time with its advance linked to the Little Ice Age (LIA), research on changes in size and mass of Hallstätter glacier was started in 1842 by Friedrich Simony. He observed and documented the glacier retreat related to its last maximum extension in 1856. In addition, Hallstätter Glacier is a subject to scientific research to date. In this thesis methods and results of ongoing mass balance measurements are pre- sented and compared to long term volume changes and meteorological observations. The current mass balance monitoring programm using the direct glaciological method was started 2006. -
P Pan American Institute of Geography and History
Although commercial and government mapmakers benefi ted from ever wider paper-making machines, the market for large sheets of high-grade paper was domi- nated by book manufacturers, who typically printed signatures of sixteen, thirty-two, or sixty-four pages on P the same sheet. The substantial growth in newspaper circulation in the late nineteenth century followed the Pan American Institute of Geography and History. development of steam-powered rotary web presses that See Societies, Cartographic: Latin America printed directly onto a continuous roll (web) of news- print, manufactured inexpensively from wood pulp. Un- til the rise of online cartography in the 1990s, news and Paper. Paper’s importance throughout the twentieth weather maps distributed on cheap, acidic newsprint, century as a medium for reproducing, disseminating, which readily yellowed, outnumbered all other carto- and storing cartographic information is matched only graphic images. by its neglect in the literatures of practical cartography Paper for printing maps was typically ordered in com- and post-Enlightenment map history. Hundreds of ar- paratively small lots according to specifi cations that re- ticles have addressed the future of the paper map, but its fl ected the intended use. Aeronautical and nautical charts, transport medium seems to have eluded scholarly scru- for instance, had to accommodate frequent annotation, tiny, except among archivists and librarians concerned and paper for the latter had to resist humidity. Military with preservation (McIlwaine 1990). This short entry maps intended for fi eld use were printed on waterproof offers a few general insights about the kinds of paper paper, whereas paper for atlases and double-sided map used in map printing as well as structural changes in the sheets had to be relatively opaque.