Journal of Development and Agricultural Economics
Total Page:16
File Type:pdf, Size:1020Kb
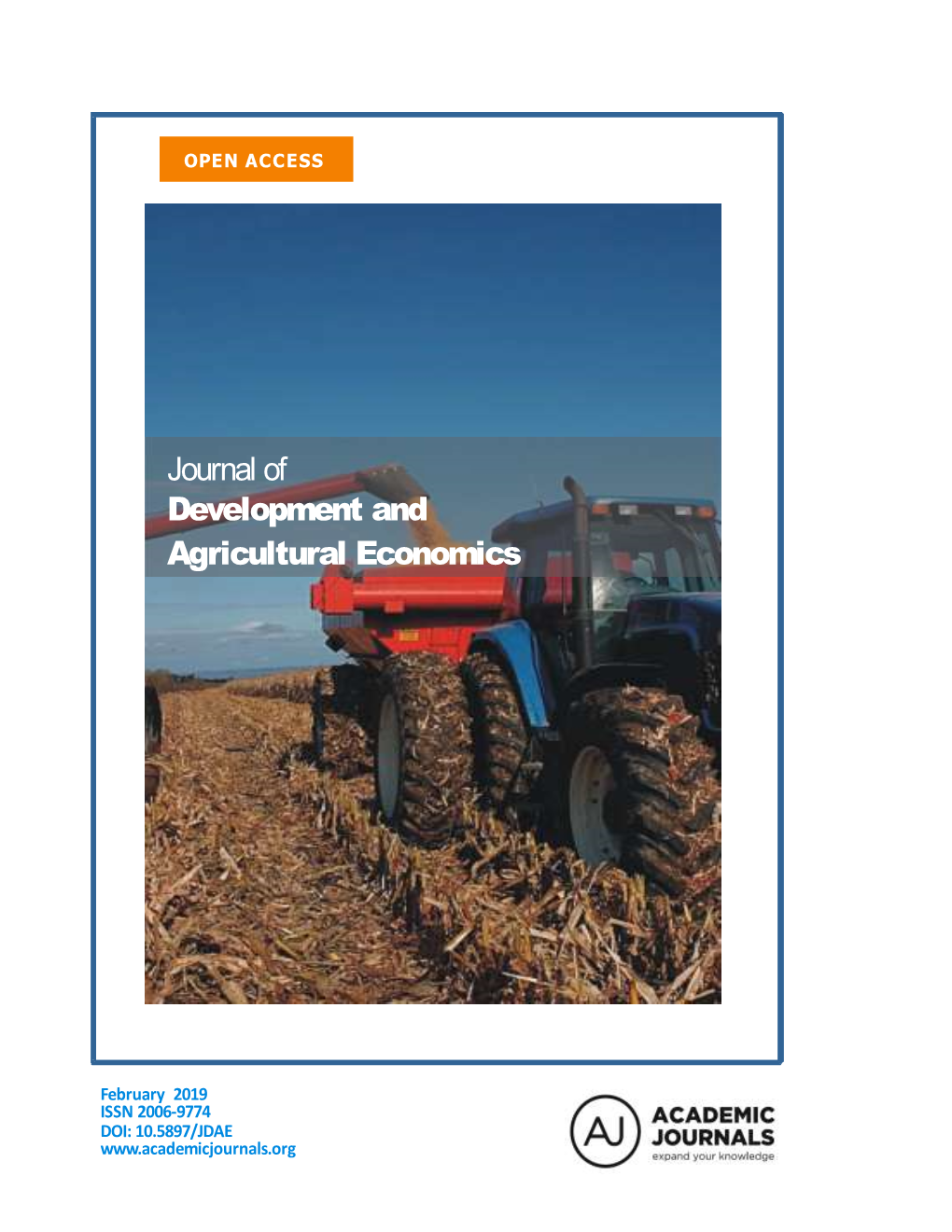
Load more
Recommended publications
-
Download/Documents/AFR2537302021ENGLISH.PDF
“I DON’T KNOW IF THEY REALIZED I WAS A PERSON” RAPE AND OTHER SEXUAL VIOLENCE IN THE CONFLICT IN TIGRAY, ETHIOPIA Amnesty International is a movement of 10 million people which mobilizes the humanity in everyone and campaigns for change so we can all enjoy our human rights. Our vision is of a world where those in power keep their promises, respect international law and are held to account. We are independent of any government, political ideology, economic interest or religion and are funded mainly by our membership and individual donations. We believe that acting in solidarity and compassion with people everywhere can change our societies for the better. © Amnesty International 2021 Except where otherwise noted, content in this document is licensed under a Creative Commons Cover photo: © Amnesty International (Illustrator: Nala Haileselassie) (attribution, non-commercial, no derivatives, international 4.0) licence. https://creativecommons.org/licenses/by-nc-nd/4.0/legalcode For more information please visit the permissions page on our website: www.amnesty.org Where material is attributed to a copyright owner other than Amnesty International this material is not subject to the Creative Commons licence. First published in 2021 by Amnesty International Ltd Peter Benenson House, 1 Easton Street London WC1X 0DW, UK Index: AFR 25/4569/2021 Original language: English amnesty.org CONTENTS 1. EXECUTIVE SUMMARY 5 2. METHODOLOGY 8 3. BACKGROUND 9 4. SEXUAL VIOLENCE AGAINST WOMEN AND GIRLS IN TIGRAY 12 GANG RAPE, INCLUDING OF PREGNANT WOMEN 12 SEXUAL SLAVERY 14 SADISTIC BRUTALITY ACCOMPANYING RAPE 16 BEATINGS, INSULTS, THREATS, HUMILIATION 17 WOMEN SEXUALLY ASSAULTED WHILE TRYING TO FLEE THE COUNTRY 18 5. -
Starving Tigray
Starving Tigray How Armed Conflict and Mass Atrocities Have Destroyed an Ethiopian Region’s Economy and Food System and Are Threatening Famine Foreword by Helen Clark April 6, 2021 ABOUT The World Peace Foundation, an operating foundation affiliated solely with the Fletcher School at Tufts University, aims to provide intellectual leadership on issues of peace, justice and security. We believe that innovative research and teaching are critical to the challenges of making peace around the world, and should go hand-in- hand with advocacy and practical engagement with the toughest issues. To respond to organized violence today, we not only need new instruments and tools—we need a new vision of peace. Our challenge is to reinvent peace. This report has benefited from the research, analysis and review of a number of individuals, most of whom preferred to remain anonymous. For that reason, we are attributing authorship solely to the World Peace Foundation. World Peace Foundation at the Fletcher School Tufts University 169 Holland Street, Suite 209 Somerville, MA 02144 ph: (617) 627-2255 worldpeacefoundation.org © 2021 by the World Peace Foundation. All rights reserved. Cover photo: A Tigrayan child at the refugee registration center near Kassala, Sudan Starving Tigray | I FOREWORD The calamitous humanitarian dimensions of the conflict in Tigray are becoming painfully clear. The international community must respond quickly and effectively now to save many hundreds of thou- sands of lives. The human tragedy which has unfolded in Tigray is a man-made disaster. Reports of mass atrocities there are heart breaking, as are those of starvation crimes. -
The War in Tigray and the Challenges Faced by the United Nations
The War in Tigray and the Challenges Faced by the United Nations 1. Introduction The war in Tigray and the consequent humanitarian catastrophe are being closely followed by prominent global news outlets. According to the United Nations the lives of 4.5 million Tigrayans (Tegaru) are already endangered by the war and the dislocation it has caused. UN representatives, aid agencies and states have repeatedly urged the Government of Ethiopia to restore the supply of electricity and other public services and to establish a humanitarian corridor for the delivery of aid. The response of the Ethiopian Government has not been encouraging. Many fear that a genocide is already underway, probably on an even larger scale than that seen in Rwanda in 1994, since Tigray has been deliberately cut off from the outside world for more than one hundred days. UN offices and officials have highlighted the magnitude of this crisis time and again. Unfortunately, the political response, especially from the Security Council, has so far been disappointing. This study shines a light on the nature of this war, how it is perceived by others, and what the challenges facing the United Nations are. Tigray is one of the eight states of Ethiopia. It is surrounded by Eritrea to the north, Sudan to the west, and the Ethiopian states of Amhara and Afar to the south and east, respectively. Around 97% of Tigrayans are adherents of Coptic Christian Orthodox religion. Tigrayans are also, more or less, united by a common language (Tigrigna), culture, tradition and psychological make-up, very much like most European nations. -
Obstacles to Regional Trade in the Horn of Africa: Borders, Markets, and Production
Obstacles to Regional Trade in the Horn of Africa: Borders, Markets, and Production by Laura Hammond Clark University February 2003 Funded by USAID/OFDA Under USDA/USAID RSSA # AOT-R-00-95-00085-00 ii Table of Contents Foreword........................................................................................................................................... v Acknowledgements ........................................................................................................................vii Acronyms......................................................................................................................................... ix Photos .............................................................................................................................................. xi 1.1 The Geopolitics of Borders .................................................................................................... 2 1.2 Plenty of Food in Some Places, Not Enough in Others......................................................... 3 2. Eritrea Food Picture...................................................................................................................... 5 2.1 2002 Agricultural season........................................................................................................ 7 2.2 Refugee repatriation ............................................................................................................... 7 2.3 Eritrean Government’s “Struggle for Development” ........................................................... -
19 April 2021 Excellency, We Have the Honour to Address You in Our
PALAIS DES NATIONS • 1211 GENEVA 10, SWITZERLAND Mandates of the Special Rapporteur on the human rights of internally displaced persons; the Working Group on Arbitrary Detention; the Special Rapporteur on the situation of human rights in Eritrea; the Special Rapporteur on extrajudicial, summary or arbitrary executions; the Special Rapporteur on the right to food; the Special Rapporteur on adequate housing as a component of the right to an adequate standard of living, and on the right to non-discrimination in this context; the Special Rapporteur on trafficking in persons, especially women and children; and the Special Rapporteur on the human rights to safe drinking water and sanitation REFERENCE: AL ETH 1/2021 19 April 2021 Excellency, We have the honour to address you in our capacities as Special Rapporteur on the human rights of internally displaced persons; Working Group on Arbitrary Detention; Special Rapporteur on the situation of human rights in Eritrea; Special Rapporteur on extrajudicial, summary or arbitrary executions; Special Rapporteur on the right to food; Special Rapporteur on adequate housing as a component of the right to an adequate standard of living, and on the right to non-discrimination in this context; Special Rapporteur on trafficking in persons, especially women and children; and Special Rapporteur on the human rights to safe drinking water and sanitation, pursuant to Human Rights Council resolutions 41/15, 42/22, 44/1, 44/5, 32/8, 43/14, 44/4 and 42/5. In this connection, we would like to bring to the attention of your Excellency’s Government information we have received concerning allegations related to violations of human rights and international humanitarian law committed in the context of the conflict in the Tigray region of Ethiopia, including deliberate attacks against civilians and summary executions, indiscriminate attacks, sexual violence, arbitrary detention, destruction and looting of civilian property, and displacement of ethnic Tigrayans. -
ETHIOPIA - TIGRAY REGION HUMANITARIAN UPDATE Situation Report Last Updated: 14 Jan 2021
ETHIOPIA - TIGRAY REGION HUMANITARIAN UPDATE Situation Report Last updated: 14 Jan 2021 HIGHLIGHTS (15 Jan 2021) The security situation in Tigray Region remains dire with reports of sporadic fighting and population movement in search of safety, particularly in rural areas. In addition to hampered physical access into many parts of Tigray, mobile network and access to internet remain cut-off except in some areas in the south and west of the region. The humanitarian situation is severe as people have now lived through nearly two and half months of conflict without adequate access to food, water and health services. Deeply dependent on imports of goods, Tigray has The boundaries and names shown and the designations been cut-off from trading since November. This, used on this map do not imply official endorsement or acceptance by the United Nations. © OCHA coupled with the impact of insecurity on the harvest, left many at risk of hunger. Despite the challenging security environment and bureaucratic obstacles, humamitarians continue to deliver limited assistance in areas where access has been granted by authorities. KEY FIGURES FUNDING (2020) CONTACTS Saviano Abreu 950,000 1.3M $1.3B $704.9M Communications Team Leader, People in need of aid Projected additional Required Received Regional Office for Southern & Eastern before the conflict people to need aid Africa A n [email protected] d ! , r j 56% y e r e r j r 58,334 $40.3M ! Progress o d Alexandra de Sousa Refugess in Sudan Unmet requirements S n A Deputy Head of Office, OCHA Ethiopia since 7 November for the Response Plan [email protected] FTS: https://fts.unocha.org/appeals/9 36/summary BACKGROUND (15 Jan 2021) SITUATION OVERVIEW https://reports.unocha.org/en/country/ethiopia/ Page 1 of 10 Downloaded: 15 Jan 2021 ETHIOPIA - TIGRAY REGION HUMANITARIAN UPDATE Situation Report Last updated: 14 Jan 2021 The security situation in Tigray Region remains dire, with reports of sporadic fighting and population movement in search of safety, particularly in rural areas. -
Environmental and Social Impact Assessment Report
ENVIRONMENTAL AND SOCIAL IMPACT ASSESSMENT REPORT FOR THE PROPOSED BAEKER AND MAI KADRA STAPLE FOOD PROCESSING ZONE (SCPZ) PROMOTING SUSTAINABLE AGRICULTURAL VALUE CHAINS, ETHIOPIA GCF ENVIRONMENTAL AND SOCIAL IMPACT ASSESSMENT WESTERN TIGRAY REGION, ETHIOPIA October, 2020 Report produced by: Zereu Girmay Environment Consultancy (ZGEC) Liberia Street, Block 015/014B, Lideta, Woreda 08, Addis Ababa, Ethiopia In collaboration with WSP/UNOPS On behalf of: UNIDO and Tigray Industrial Parks Development Corporation, Federal Democratic Republic of Ethiopia Preamble This ESIA report was an Initial document prepared in July 2018 for the Baeker IAIP and Maikadra RTC to fulfill the National EIA requirements and AfDB ESS. The present ESIA report was submitted to the Ministry of Environment, Forest and Climate Change (MoEFCC) in early 2018 for review and approval, following which it was approved by the stated National competent authority and has been issued with environmental clearance certificate to precede in late 2018. The review and approval process carried by the competent authority involved public disclosure and information dissemination of the ESIA findings contained in the document before issuance of the environmental clearance. Valuable comments were also provided by the competent authority to be considered during the project implementation phases. The present review and update process of the ESIA carried during October 2020 was initiated to integrate the proposed project activities to be supported by the GCF funds. Efforts were also made to update the ESIA by addressing aspects that occurred in the IAIP and RTC during the past two years of implementation. The ESIA and RAP reports of IAIP and RTC have been prepared comprehensively covering the development of all infrastructure components and operational activities that will take place during the construction and operation phases of the IAIP and RTC project. -
March 2021 Children in Need Of
Ethiopia Country Office Humanitarian Situation Report Includes results from Tigray Response Situation in Numbers © UNICEF Ethiopia/2021/Demissew Bizuwerk 12.5 million Reporting Period: March 2021 children in need of humanitarian assistance 1 Highlights (HNO 2021) • In the past three months, UNICEF has reached a total of 1,583,723 people with safe water supply for personal use through the rehabilitation of non-functional water systems, water 23.5 million people in need treatment, and water trucking throughout the country. Of these, (HNO 2021) 641,976 people have been reached in Tigray Region. • Some 38,758 in people in Tigray were reached through the 22 active Mobile Health and Nutrition Teams (MHNTs), out of whom 2.3 million2 11,594 were children who received medical consultations, internally displaced people nutrition services, and immunization. (DTM, 2021) • Since the beginning of its response in Tigray, UNICEF has delivered 1,093.4 metric tons of multi-sectoral supplies to nine partners (including regional bureaus) with a total value of US$2.5 805,164 million. registered refugees • In February, 34,883 new cases of Severe Acute Malnutrition (UNHCR, 31 March 2021) (SAM) were admitted for treatment throughout the country. Out of these, 48 per cent were in Oromia, 26.7 percent in Somali, and 4.3 per cent in Tigray. The cure rate was 90.9 per cent, death rate was 0.2 per cent and defaulter rate was 1.85 per cent. The UNICEF HAC Appeal rest were medical transfers or non-respondent children. 2021 US$ 188 million UNICEF’s Response and Funding -
Situation Report EEPA HORN No. 55 - 14 January 2021
Situation Report EEPA HORN No. 55 - 14 January 2021 Europe External Programme with Africa is a Belgium-based Centre of Expertise with in-depth knowledge, publications, and networks, specialised in issues of peace building, refugee protection and resilience in the Horn of Africa. EEPA has published extensively on issues related to movement and/or human trafficking of refugees in the Horn of Africa and on the Central Mediterranean Route. It cooperates with a wide network of Universities, research organisations, civil society and experts from Ethiopia, Eritrea, Kenya, Djibouti, Somalia, Sudan, South Sudan, Uganda and across Africa. Key in-depth publications can be accessed on the website. Reported war situation (as confirmed per 13 January) - The chief commander of one of the Eritrean divisions fighting in the Eastern front in Tigray has been captured alive by Tigray regional forces. - ENDF states it has killed three members of the leadership of TPLF who held high office in Ethiopia: Seyoum Mesfin, foreign minister of Ethiopia from 1991 until 2010; Abay Tsehaye, former Federal Affairs Minister and Asmelash Woldesellassie, ex-parliamentary chief whip of the Ethiopian Parliament. - The Ethiopian Government is arresting former retired Tigrayan officials and their spouses. Many of those are said to be arrested from their houses in Mekelle. Official reports make it appear as if they were captured in battle. It is reported that this is not true. - It is reported from Eritrea that Sebhat Nega, the retired co-founder of TPLF, was arrested in his house in Mekelle, then taken to the Mai Idaga prison near Dekemhare in Eritrea. -
ETHIOPIA - TIGRAY REGION HUMANITARIAN UPDATE Situation Report Last Updated: 6 Jan 2021
ETHIOPIA - TIGRAY REGION HUMANITARIAN UPDATE Situation Report Last updated: 6 Jan 2021 HIGHLIGHTS (7 Jan 2021) The security situation in Tigray remains volatile with a gradual improvement in some areas in the Eastern and Western Zones, although insecurity & bureaucratic constraints remain. The two joint Government-humanitarian partners’ assessment missions that deployed on 20 December are completed. Both missions have witnessed a dire humanitarian situation with poor access to services and limited livelihoods. According to both assessment missions, food supplies are very limited, widespread looting is reported and insecurity is high. The boundaries and names shown and the designations used on this map do not imply official endorsement or acceptance by the United Nations. © OCHA OCHA continues to closely work with Government counterparts to streamline the cargo/assessment clearance mechanism, and enable a quicker process (within 48 hours). KEY FIGURES FUNDING (2020) CONTACTS Saviano Abreu 950,000 1.3M $1.3B $704.9M Communications Team Leader, People in need of aid Projected additional Required Received Regional Office for Southern & Eastern before the conflict people to need aid Africa A n [email protected] d ! , r j 56% y e r e r j r 55,500 $63.4M ! Progress o d Alexandra de Sousa Refugess in Sudan Needed for S n A Deputy Head of Office, OCHA Ethiopia since 7 November Preparedness Plan [email protected] FTS: https://fts.unocha.org/appeals/9 36/summary BACKGROUND (7 Jan 2021) SITUATION OVERVIEW Humanitarian workers have been able to access areas that were so far inaccessible, particularly in cities. However, localized fighting and insecurity continues, with fighting reported in rural areas and in the peripheries of Mekelle, Shiraro and Shire among other locations, as of last week. -
Esia Report for the Proposed Baeker Iaip and Mai Kadra Rtc
March 2018 December 2017 ESIA REPORT FOR THE PROPOSED BAEKER IAIP AND MAI KADRA RTC ENVIRONMENTAL AND SOCIAL IMPACT ASSESSMENT WESTERN TIGRAY REGION, ETHIOPIA Tigray Region IPDC TYPE OF DOCUMENT (PUBLIC)(DRAFT) DATE: MARCH 2018 ESIA REPORT Proposed Baeker IAIP and Mai Kadra RTC, Tigray Western Tigray Region Page i TABLE OF 1 INTRODUCTION ..................................... 1-1 CONTENTS 1.1 Background to the Project ................................. 1-1 1.2 Land Tenure and Land Use ................................ 1-4 1.3 Purpose of This Report (ESIA Report) .............. 1-5 1.4 Structure of this Report ...................................... 1-5 1.5 Details of the ESIA Project Team ....................... 1-8 1.6 Details of the Applicant and Environmental Assessment Practitioner .................................... 1-9 1.7 Assumptions and Limitations ............................ 1-9 2 PROJECT DESCRIPTION ...................... 2-1 2.1 Baeker IAIP ........................................................... 2-1 2.2 MaI Kadra RTC ..................................................... 2-9 2.3 Phases of the Proposed Project ...................... 2-14 2.4 Project Status .................................................... 2-15 3 NEED AND DESIRABILTY...................... 3-1 4 PROJECT ALTERNATIVES .................... 4-1 4.1 Introduction .......................................................... 4-1 4.2 Consideration of Alternatives ............................ 4-1 4.3 Comparison of Alternatives ............................... 4-2 5 LEGAL -
Socio-Economic Assessment
SOCIO-ECONOMIC ASSESSMENT Tigray IAIP and RTC Report Produced by: WSP in collaboration with Zereu Girmay Environment Consultancy (ZGEC) DATE: DECEMBER 2017 Socio-Economic Assessment – Tigray IAIP & RTC Draft December 2017 TABLE OF CONTENTS 1 INTRODUCTION .......................................................................... 1 1.1 SOCIAL AREAS OF INFLUENCE (AOI) – SOCIAL STUDY AREA ................. 1 1.2 ADMINISTRATIVE AND DEMOGRAPHIC ENVIRONMENT ............................. 1 1.3 MIGRATION PATTERNS ................................................................................... 2 1.4 LAND USE, LIVELIHOOD AND VULNERABILITY ........................................... 3 1.5 WOMEN AND LAND OWNERSHIP ................................................................... 4 1.6 EDUCATION ....................................................................................................... 4 1.7 HOUSEHOLD INCOME AND EXPENDITURE .................................................. 5 1.8 EXISTING INFRASTRUCTURE ......................................................................... 5 1.9 NATIONAL HOLIDAYS ...................................................................................... 5 2 SOCIO-ECONOMIC PROFILE OF PEOPLE IN THE PROJECT AREA (BASED ON WSP SURVEY) .......................... 7 2.1 METHODOLOGY ................................................................................................ 7 2.2 GENDER AND AGE ........................................................................................... 7 2.3 EDUCATION ......................................................................................................