What Constitutes Credible Evidence of Effectiveness?
Total Page:16
File Type:pdf, Size:1020Kb
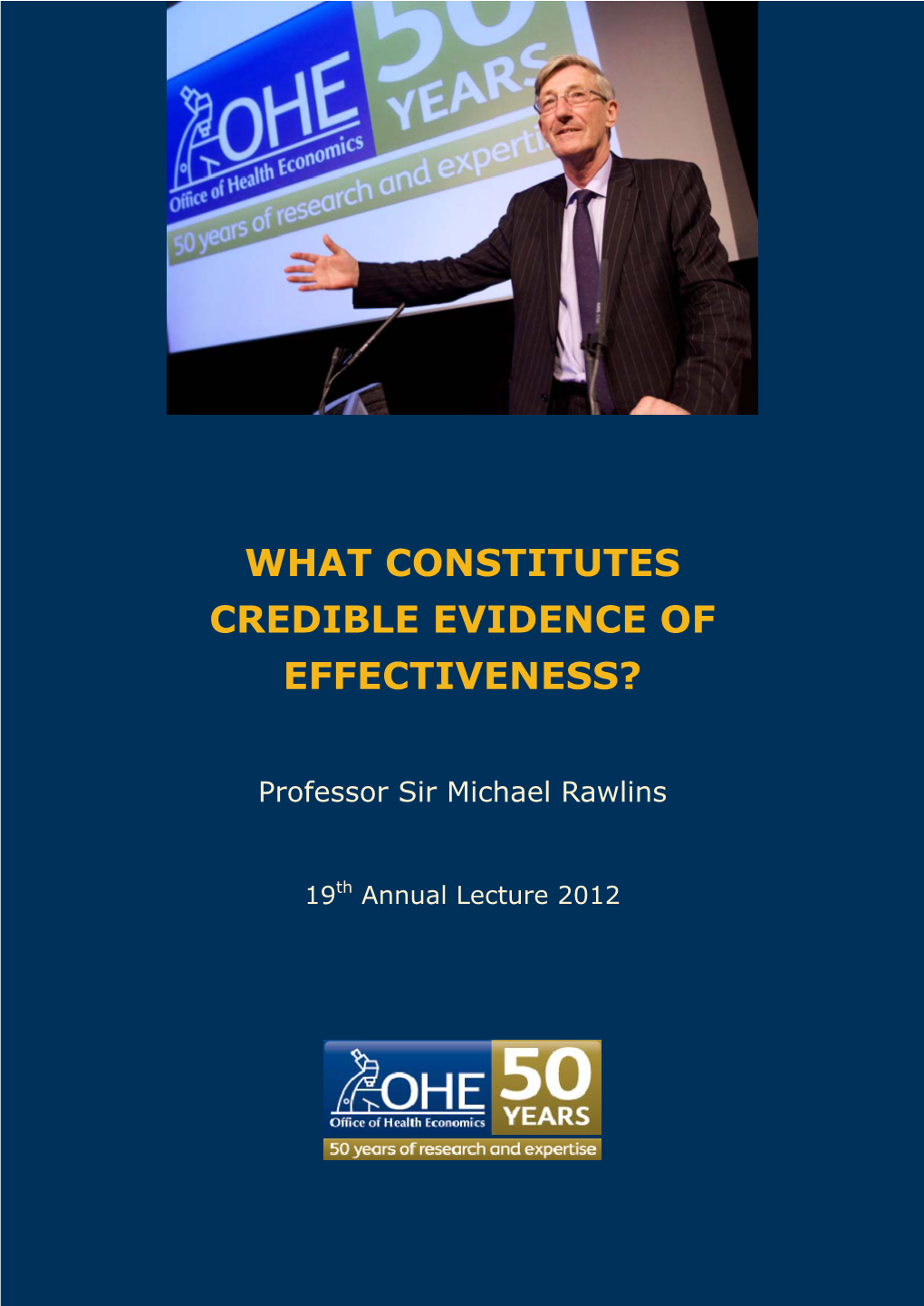
Load more
Recommended publications
-
Applications and Issues in Assessment
meeting of the Association of British Neurologists in April vessels: a direct comparison between intravenous and intra-arterial DSA. 1992. We thank Professor Charles Warlow for his helpful Cl/t Radiol 1991;44:402-5. 6 Caplan LR, Wolpert SM. Angtography in patients with occlusive cerebro- comments. vascular disease: siews of a stroke neurologist and neuroradiologist. AmcincalsouraalofNcearoradiologv 1991-12:593-601. 7 Kretschmer G, Pratschner T, Prager M, Wenzl E, Polterauer P, Schemper M, E'uropean Carotid Surgery Trialists' Collaboration Group. MRC European ct al. Antiplatelet treatment prolongs survival after carotid bifurcation carotid surgerv trial: interim results for symptomatic patients with severe endarterectomv. Analysts of the clinical series followed by a controlled trial. (70-90()',) or tvith mild stenosis (0-299',Y) carotid stenosis. Lantcet 1991 ;337: AstniSuirg 1990;211 :317-22. 1 235-43. 8 Antiplatelet Trialists' Collaboration. Secondary prevention of vascular disease 2 North American Svmptotnatic Carotid Endarterectomv Trial Collaborators. by prolonged antiplatelet treatment. BM7 1988;296:320-31. Beneficial effect of carotid endarterectomv in svmptomatic patients with 9 Murie JA, Morris PJ. Carotid endarterectomy in Great Britain and Ireland. high grade carotid stenosis. VEngl 7,1Med 1991;325:445-53. Br7Si(rg 1986;76:867-70. 3 Hankev CJ, Warlows CP. Svmptomatic carotid ischaemic events. Safest and 10 Murie J. The place of surgery in the management of carotid artery disease. most cost effective way of selecting patients for angiography before Hospital Update 1991 July:557-61. endarterectomv. Bt4 1990;300:1485-91. 11 Dennis MS, Bamford JM, Sandercock PAG, Warlow CP. Incidence of 4 Humphrev PRD, Sandercock PAG, Slatterv J. -
What Statistics Can and Can't Tell Us About Ourselves | the New Yorker
What Statistics Can and Canʼt Tell Us About Ourselves | The New Yorker 2019-09-04, 1005 Books September 9, 2019 Issue What Statistics Can and Can’t Tell Us About Ourselves In the era of Big Data, we’ve come to believe that, with enough information, human behavior is predictable. But number crunching can lead us perilously wrong. By Hannah Fry September 2, 2019 https://www.newyorker.com/magazine/2019/09/09/what-statistics…3&utm_medium=email&utm_term=0_c9dfd39373-f791742ba3-42227231 Page 1 of 15 What Statistics Can and Canʼt Tell Us About Ourselves | The New Yorker 2019-09-04, 1005 Making individual predictions from collective characteristics is a risky business. Illustration by Ben Wiseman 0"00 / 23"27 Audio: Listen to this article. To hear more, download Audm for iPhone or Android. arold Eddleston, a seventy-seven-year-old from Greater Manchester, H was still reeling from a cancer diagnosis he had been given that week https://www.newyorker.com/magazine/2019/09/09/what-statistics…3&utm_medium=email&utm_term=0_c9dfd39373-f791742ba3-42227231 Page 2 of 15 What Statistics Can and Canʼt Tell Us About Ourselves | The New Yorker 2019-09-04, 1005 when, on a Saturday morning in February, 1998, he received the worst possible news. He would have to face the future alone: his beloved wife had died unexpectedly, from a heart attack. Eddleston’s daughter, concerned for his health, called their family doctor, a well-respected local man named Harold Shipman. He came to the house, sat with her father, held his hand, and spoke to him tenderly. -
Note for the Record: Consultation on Clinical Trial Design for Ebola Virus Disease (EVD)
Note for the record: Consultation on Clinical Trial Design for Ebola Virus Disease (EVD) A group of independent scientific experts was convened by the WHO for the purpose of evaluating a proposed clinical trial design for investigational therapeutics for Ebola virus disease (EVD) during the current outbreak, 26 May 2018 Experts Sir Michael Jacobs (Chair), Dr Rick Bright, Dr Marco Cavaleri, Dr Edward Cox, Dr Natalie Dean, Dr William Fischer, Dr Thomas Fleming, Dr Elizabeth Higgs, Dr Peter Horby, Dr Philip Krause, Dr Trudie Lang, Dr Denis Malvy, Sir Richard Peto, Dr Peter Smith, Dr Marianne Van der Sande, Dr Robert Walker, Dr David Wohl, Dr Alan Young. There are many pathogens for which there is no proven specific treatment. For some pathogens, there are treatments that have shown promising safety and activity in the laboratory and in relevant animal models but have not yet been evaluated fully for safety and efficacy in humans. In the context of an outbreak characterized by high mortality rates, the Monitored Emergency Use of Unregistered Interventions (MEURI)1 framework is a means to provide access to promising but unproven investigational therapies, but is not a means to evaluate reliably whether these compounds are actually beneficial to patients or not. In light of the current Ebola Zaire DRC outbreak with a high case fatality rate, the WHO convened an independent expert panel to evaluate investigational therapeutics for MEURI use2, and that panel affirmed the importance of moving to appropriate clinical trials as soon as possible. Against this background, a clinical trial design has been proposed with a view to generating reliable evidence about safety and efficacy. -
JSM 2017 in Baltimore the 2017 Joint Statistical Meetings in Baltimore, Maryland, Which Included the CONTENTS IMS Annual Meeting, Took Place from July 29 to August 3
Volume 46 • Issue 6 IMS Bulletin September 2017 JSM 2017 in Baltimore The 2017 Joint Statistical Meetings in Baltimore, Maryland, which included the CONTENTS IMS Annual Meeting, took place from July 29 to August 3. There were over 6,000 1 JSM round-up participants from 52 countries, and more than 600 sessions. Among the IMS program highlights were the three Wald Lectures given by Emmanuel Candès, and the Blackwell 2–3 Members’ News: ASA Fellows; ICM speakers; David Allison; Lecture by Martin Wainwright—Xiao-Li Meng writes about how inspirational these Mike Cohen; David Cox lectures (among others) were, on page 10. There were also five Medallion lectures, from Edoardo Airoldi, Emery Brown, Subhashis Ghoshal, Mark Girolami and Judith 4 COPSS Awards winners and nominations Rousseau. Next year’s IMS lectures 6 JSM photos At the IMS Presidential Address and Awards session (you can read Jon Wellner’s 8 Anirban’s Angle: The State of address in the next issue), the IMS lecturers for 2018 were announced. The Wald the World, in a few lines lecturer will be Luc Devroye, the Le Cam lecturer will be Ruth Williams, the Neyman Peter Bühlmann Yuval Peres 10 Obituary: Joseph Hilbe lecture will be given by , and the Schramm lecture by . The Medallion lecturers are: Jean Bertoin, Anthony Davison, Anna De Masi, Svante Student Puzzle Corner; 11 Janson, Davar Khoshnevisan, Thomas Mikosch, Sonia Petrone, Richard Samworth Loève Prize and Ming Yuan. 12 XL-Files: The IMS Style— Next year’s JSM invited sessions Inspirational, Mathematical If you’re feeling inspired by what you heard at JSM, you can help to create the 2018 and Statistical invited program for the meeting in Vancouver (July 28–August 2, 2018). -
The American Statistician
This article was downloaded by: [T&F Internal Users], [Rob Calver] On: 01 September 2015, At: 02:24 Publisher: Taylor & Francis Informa Ltd Registered in England and Wales Registered Number: 1072954 Registered office: 5 Howick Place, London, SW1P 1WG The American Statistician Publication details, including instructions for authors and subscription information: http://www.tandfonline.com/loi/utas20 Reviews of Books and Teaching Materials Published online: 27 Aug 2015. Click for updates To cite this article: (2015) Reviews of Books and Teaching Materials, The American Statistician, 69:3, 244-252, DOI: 10.1080/00031305.2015.1068616 To link to this article: http://dx.doi.org/10.1080/00031305.2015.1068616 PLEASE SCROLL DOWN FOR ARTICLE Taylor & Francis makes every effort to ensure the accuracy of all the information (the “Content”) contained in the publications on our platform. However, Taylor & Francis, our agents, and our licensors make no representations or warranties whatsoever as to the accuracy, completeness, or suitability for any purpose of the Content. Any opinions and views expressed in this publication are the opinions and views of the authors, and are not the views of or endorsed by Taylor & Francis. The accuracy of the Content should not be relied upon and should be independently verified with primary sources of information. Taylor and Francis shall not be liable for any losses, actions, claims, proceedings, demands, costs, expenses, damages, and other liabilities whatsoever or howsoever caused arising directly or indirectly in connection with, in relation to or arising out of the use of the Content. This article may be used for research, teaching, and private study purposes. -
Sir Richard Doll, Epidemiologist – a Personal Reminiscence with a Selected Bibliography
British Journal of Cancer (2005) 93, 963 – 966 & 2005 Cancer Research UK All rights reserved 0007 – 0920/05 $30.00 www.bjcancer.com Obituary Sir Richard Doll, epidemiologist – a personal reminiscence with a selected bibliography The death of Richard Doll on 24 July 2005 at the age of 92 after a short illness ended an extraordinarily productive life in science for which he received widespread recognition, including Fellowship of The Royal Society (1966), Knighthood (1971), Companionship of Honour (1996), and many honorary degrees and prizes. He is unique, however, in having seen both universal acceptance of his work demonstrating smoking as the main cause of the most common fatal cancer in the world and the relative success of strategies to reduce the prevalence of the habit. In 1950, 80% of the men in Britain smoked but this has now declined to less than 30%. Richard Doll qualified in medicine at St Thomas’ Hospital in 1937, but his epidemiological career began after service in the Second World War when he worked with Francis Avery Jones at the Central Middlesex Hospital on occupational factors in the aetiology of peptic ulceration. The completeness of Doll’s tracing of previously surveyed men so impressed Tony Bradford Hill that he offered him a post in the MRC Statistical Research Unit to investigate the causes of lung cancer. For the representations of Percy Stocks (Chief Medical Officer to the Registrar General) and Sir Ernest Kennaway had prevailed against the then commonly held view that the marked rise in lung cancer deaths in Britain since 1900 was due only to improved diagnosis. -
Whoseжlegacyжwillжreadersж Celebrateжthisжyear?
BMJ GROUP AWARDS •ЖReviewЖtheЖshortlistsЖforЖallЖcategoriesЖatЖhttp://bit.ly/aUWoE5 Sir George Alleyne •ЖListenЖtoЖtheЖawardsЖlaunchЖpodcastЖatЖbmj.com/podcasts A highly respected figure in global health, George Alleyne has played LIFETIME ACHIEVEMENT AWARD a large part in tackling HIV and non-communicable disease and WhoseЖlegacyЖwillЖreadersЖ is an energetic promoter of health equality across the world. Currently the chancellor of the University celebrateЖthisЖyear? of the West Indies, Sir George is also the former director of the Pan Annabel FerrimanЖintroducesЖthisЖyear’sЖawardЖandЖ American Health Organization. Adrian O’DowdЖrevealsЖtheЖshortlistedЖcandidates Born in Barbados, he graduated in medicine from the University When Belgian senator Marleen Temmerman called on women in Belgium of the West Indies in 1957 and to refuse to have sex with their partners until the country’s politicians continued his postgraduate ended eight months of wrangling and formed a government, few people studies in the United Kingdom and effect on national economies. in the UK had heard of her. But readers of the BMJ were in the know and the United States. During more Sir George has served on various unsurprised. than a decade of original research, committees including the For Professor Temmerman, an obstetrician and gynaecologist, had he produced 144 publications in scientific and technical advisory won the BMJ Group’s Lifetime Achievement Award last April. In that case, scientific journals, which qualified committee of the World Health it was not for suggesting a “crossed leg strike” to end political deadlock him at just 40 years of age to be Organization Tropical Research (a solution advocated by the women of Greece in Aristophanes’ play appointed professor of medicine Programme and the Institute of Lysistrata) but for her services to women’s health in Belgium and Kenya. -
Statistical Tips for Interpreting Scientific Claims
Research Skills Seminar Series 2019 CAHS Research Education Program Statistical Tips for Interpreting Scientific Claims Mark Jones Statistician, Telethon Kids Institute 18 October 2019 Research Skills Seminar Series | CAHS Research Education Program Department of Child Health Research | Child and Adolescent Health Service ResearchEducationProgram.org © CAHS Research Education Program, Department of Child Health Research, Child and Adolescent Health Service, WA 2019 Copyright to this material produced by the CAHS Research Education Program, Department of Child Health Research, Child and Adolescent Health Service, Western Australia, under the provisions of the Copyright Act 1968 (C’wth Australia). Apart from any fair dealing for personal, academic, research or non-commercial use, no part may be reproduced without written permission. The Department of Child Health Research is under no obligation to grant this permission. Please acknowledge the CAHS Research Education Program, Department of Child Health Research, Child and Adolescent Health Service when reproducing or quoting material from this source. Statistical Tips for Interpreting Scientific Claims CONTENTS: 1 PRESENTATION ............................................................................................................................... 1 2 ARTICLE: TWENTY TIPS FOR INTERPRETING SCIENTIFIC CLAIMS, SUTHERLAND, SPIEGELHALTER & BURGMAN, 2013 .................................................................................................................................. 15 3 -
A Cancer Control Interview With: Sir Richard Peto
CANCER CONTROL PLANNING A CANCER CONTROL INTERVIEW WITH: SIR RICHARD PETO Sir Richard Peto is Professor of Medical Statistics & Epidemiology at the University of Oxford, United Kingdom. In 1975, he set up the Clincial Trial Service Unit in the Medical Sciences Division of Oxford University, of which he and Rory Collins are now co-directors. Professor Peto's work has included studies of the causes of cancer in general, and of the effects of smoking in particular, and the establishment of large-scale randomised trials of the treatment of heart disease, stroke, cancer and a variety of other diseases. He has been instrumental in introducing combined "meta-analyses" of results from related trials that achieve uniquely reliable assessment of treatment effects. He was elected a Fellow of the Royal Society of London in 1989, and was knighted (for services to epidemiology and to cancer prevention) in 1999. Cancer Control: How should the global target of a 25% reduction country would die before they were 70; now it is 18%. in premature mortality from non-communicable diseases (NCDs) by 2025 be interpreted? Cancer Control: NCD advocates place great emphasis on the Sir Richard Peto: I would define “premature death” as death relative burden of non-communicable diseases compared with during the middle age range of 35 to 69 years. It’s not that communicable diseases. Are they right to do so? deaths after 70 don’t matter – I’m after 70 myself and I’m Sir Richard Peto: I don’t like these things that say “More still enjoying life – but if you are going to do things to reduce people die from NCDs than from communicable disease and NCDs then it is going to be to reduce NCD mortality in your therefore NCDs are more important”. -
A Kernel Log-Rank Test of Independence for Right-Censored Data
A kernel log-rank test of independence for right-censored data Tamara Fern´andez∗ Arthur Gretton University College London University College London [email protected] [email protected] David Rindt Dino Sejdinovic University of Oxford University of Oxford [email protected] [email protected] November 18, 2020 Abstract We introduce a general non-parametric independence test between right-censored survival times and covariates, which may be multivariate. Our test statistic has a dual interpretation, first in terms of the supremum of a potentially infinite collection of weight-indexed log-rank tests, with weight functions belonging to a reproducing kernel Hilbert space (RKHS) of functions; and second, as the norm of the difference of embeddings of certain finite measures into the RKHS, similar to the Hilbert-Schmidt Independence Criterion (HSIC) test-statistic. We study the asymptotic properties of the test, finding sufficient conditions to ensure our test correctly rejects the null hypothesis under any alternative. The test statistic can be computed straightforwardly, and the rejection threshold is obtained via an asymptotically consistent Wild Bootstrap procedure. Extensive simulations demonstrate that our testing procedure generally performs better than competing approaches in detecting complex non-linear dependence. 1 Introduction Right-censored data appear in survival analysis and reliability theory, where the time-to-event variable one is interested in modelling may not be observed fully, but only in terms of a lower bound. This is a common occurrence in clinical trials as, usually, the follow-up is restricted to the duration of the study, or patients may decide to withdraw from the study. -
STATISTICAL SCIENCE Volume 31, Number 4 November 2016
STATISTICAL SCIENCE Volume 31, Number 4 November 2016 ApproximateModelsandRobustDecisions..................James Watson and Chris Holmes 465 ModelUncertaintyFirst,NotAfterwards....................Ingrid Glad and Nils Lid Hjort 490 ContextualityofMisspecificationandData-DependentLosses..............Peter Grünwald 495 SelectionofKLNeighbourhoodinRobustBayesianInference..........Natalia A. Bochkina 499 IssuesinRobustnessAnalysis.............................................Michael Goldstein 503 Nonparametric Bayesian Clay for Robust Decision Bricks ..................................................Christian P. Robert and Judith Rousseau 506 Ambiguity Aversion and Model Misspecification: An Economic Perspective ................................................Lars Peter Hansen and Massimo Marinacci 511 Rejoinder: Approximate Models and Robust Decisions. .James Watson and Chris Holmes 516 Filtering and Tracking Survival Propensity (Reconsidering the Foundations of Reliability) .......................................................................Nozer D. Singpurwalla 521 On Software and System Reliability Growth and Testing. ..............FrankP.A.Coolen 541 HowAboutWearingTwoHats,FirstPopper’sandthendeFinetti’s?..............Elja Arjas 545 WhatDoes“Propensity”Add?...................................................Jane Hutton 549 Reconciling the Subjective and Objective Aspects of Probability ...............Glenn Shafer 552 Rejoinder:ConcertUnlikely,“Jugalbandi”Perhaps..................Nozer D. Singpurwalla 555 ChaosCommunication:ACaseofStatisticalEngineering...............Anthony -
Asymptotically Efficient Rank Invariant Test Procedures Author(S): Richard Peto and Julian Peto Source: Journal of the Royal Statistical Society
Asymptotically Efficient Rank Invariant Test Procedures Author(s): Richard Peto and Julian Peto Source: Journal of the Royal Statistical Society. Series A (General), Vol. 135, No. 2 (1972), pp. 185-207 Published by: Wiley for the Royal Statistical Society Stable URL: http://www.jstor.org/stable/2344317 Accessed: 21-04-2017 17:23 UTC JSTOR is a not-for-profit service that helps scholars, researchers, and students discover, use, and build upon a wide range of content in a trusted digital archive. We use information technology and tools to increase productivity and facilitate new forms of scholarship. For more information about JSTOR, please contact [email protected]. Your use of the JSTOR archive indicates your acceptance of the Terms & Conditions of Use, available at http://about.jstor.org/terms Royal Statistical Society, Wiley are collaborating with JSTOR to digitize, preserve and extend access to Journal of the Royal Statistical Society. Series A (General) This content downloaded from 171.65.37.237 on Fri, 21 Apr 2017 17:23:40 UTC All use subject to http://about.jstor.org/terms J. R. Statist. Soc. A, 185 (1972), 135, Part 2, p. 185 Asymptotically Efficient Rank Invariant Test Procedures By RICHARD PETO AND JULIAN PETO Radcliffe Infirmary, Institute of Psychiatry Oxford University University of London [Read before the ROYAL STATISTICAL SOCIETY on Wednesday, January 19th, 1972, the President Professor G. A. BARNARD in the Chair] SUMMARY Asymptotically efficient rank invariant test procedures for detecting differences between two groups of independent observations are derived. These are generalized to test between two groups of independent censored observations, to test between many groups of observations, and to test between groups after allowance for the effects of concomitant variables.