Informamon Visualizamon
Total Page:16
File Type:pdf, Size:1020Kb
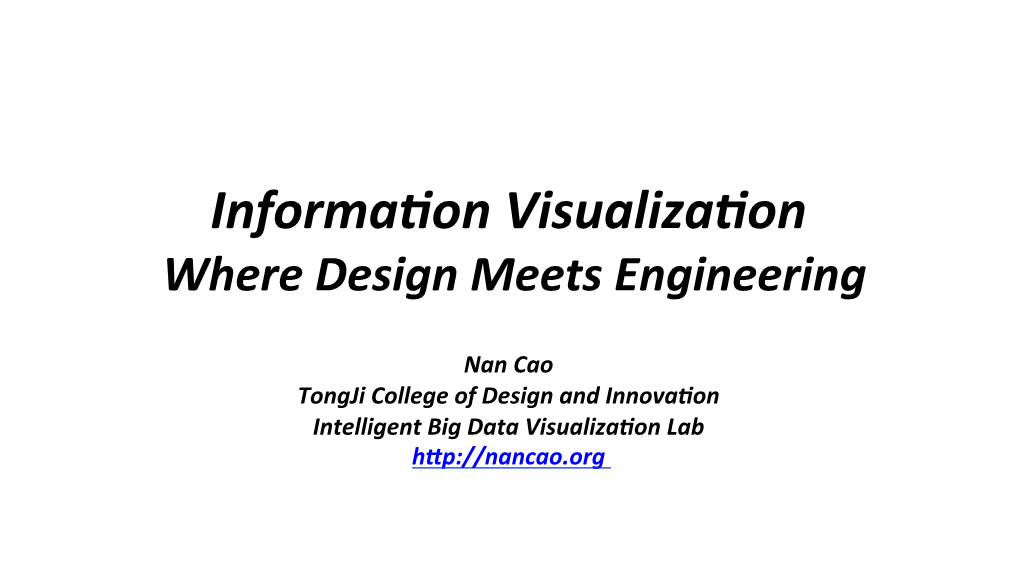
Load more
Recommended publications
-
Teachingatrisk: Progress & Potholes
Teachingat Risk: Progress & Potholes FINAL REPORT SPRING 2006 The Teaching Commission LOUIS V. GERSTNER, JR. VARTAN GREGORIAN Chairman President The Teaching Commission The Carnegie Corporation of New York Former Chairman and CEO BEVERLY L. HALL IBM Superintendent Atlanta Public Schools ARLENE ACKERMAN Superintendent JAMES B. HUNT, JR. San Francisco Unified School District Former Governor North Carolina ROY E. BARNES Former Governor FRANK KEATING Georgia Former Governor Oklahoma RICHARD I. BEATTIE Chairman RICHARD KRASNO Simpson Thacher & Bartlett LLP Executive Director The William R. Kenan, Jr., Charitable Trust BARBARA BUSH ELLEN CONDLIFFE LAGEMANN KENNETH I. CHENAULT Charles Warren Professor of the History of Chairman and CEO American Education American Express Company Harvard University PHILIP M. CONDIT W. JAMES MCNERNEY, JR. Former Chairman and CEO Chairman, President, and CEO The Boeing Company The Boeing Company JOHN DOERR SCOTT E. PAINTER Partner AP Coordinator and Teacher Kleiner Perkins Caufield & Byers Project GRAD Atlanta and South Atlanta High School MATTHEW GOLDSTEIN Chancellor RICHARD W. RILEY The City University of New York Former U.S. Secretary of Education Former Governor South Carolina THE FINAL REPORT Teaching at Risk: Progress and Potholes THE TEACHING COMMISSION Infographics by Nigel Holmes © 2006 THE TEACHING COMMISSION All Rights Reserved 2 FINAL REPORT Dedicated to R. GAYNOR MCCOWN 1960-2005 AND SANDRA FELDMAN 1939-2005 Teachers, Reformers, and Leaders 3 THE TEACHING COMMISSION 4 About The Teaching Commission stablished and chaired by Louis V.Gerstner, Jr., the former chair- man of IBM, the Teaching Commission has sought to improve Estudent performance and close the nation’s dangerous achievement gap by transforming the way in which America’s public school teachers are prepared, recruited, retained, and rewarded. -
An Investigation Into the Graphic Innovations of Geologist Henry T
Louisiana State University LSU Digital Commons LSU Doctoral Dissertations Graduate School 2003 Uncovering strata: an investigation into the graphic innovations of geologist Henry T. De la Beche Renee M. Clary Louisiana State University and Agricultural and Mechanical College Follow this and additional works at: https://digitalcommons.lsu.edu/gradschool_dissertations Part of the Education Commons Recommended Citation Clary, Renee M., "Uncovering strata: an investigation into the graphic innovations of geologist Henry T. De la Beche" (2003). LSU Doctoral Dissertations. 127. https://digitalcommons.lsu.edu/gradschool_dissertations/127 This Dissertation is brought to you for free and open access by the Graduate School at LSU Digital Commons. It has been accepted for inclusion in LSU Doctoral Dissertations by an authorized graduate school editor of LSU Digital Commons. For more information, please [email protected]. UNCOVERING STRATA: AN INVESTIGATION INTO THE GRAPHIC INNOVATIONS OF GEOLOGIST HENRY T. DE LA BECHE A Dissertation Submitted to the Graduate Faculty of the Louisiana State University and Agricultural and Mechanical College in partial fulfillment of the requirements for the degree of Doctor of Philosophy in The Department of Curriculum and Instruction by Renee M. Clary B.S., University of Southwestern Louisiana, 1983 M.S., University of Southwestern Louisiana, 1997 M.Ed., University of Southwestern Louisiana, 1998 May 2003 Copyright 2003 Renee M. Clary All rights reserved ii Acknowledgments Photographs of the archived documents held in the National Museum of Wales are provided by the museum, and are reproduced with permission. I send a sincere thank you to Mr. Tom Sharpe, Curator, who offered his time and assistance during the research trip to Wales. -
Meisho Zue and the Mapping of Prosperity in Late Tokugawa Japan
Meisho Zue and the Mapping of Prosperity in Late Tokugawa Japan Robert Goree, Wellesley College Abstract The cartographic history of Japan is remarkable for the sophistication, variety, and ingenuity of its maps. It is also remarkable for its many modes of spatial representation, which might not immediately seem cartographic but could very well be thought of as such. To understand the alterity of these cartographic modes and write Japanese map history for what it is, rather than what it is not, scholars need to be equipped with capacious definitions of maps not limited by modern Eurocentric expectations. This article explores such classificatory flexibility through an analysis of the mapping function of meisho zue, popular multivolume geographic encyclopedias published in Japan during the eighteenth and nineteenth centuries. The article’s central contention is that the illustrations in meisho zue function as pictorial maps, both as individual compositions and in the aggregate. The main example offered is Miyako meisho zue (1780), which is shown to function like a map on account of its instrumental pictorial representation of landscape, virtual wayfinding capacity, spatial layout as a book, and biased selection of sites that contribute to a vision of prosperity. This last claim about site selection exposes the depiction of meisho as a means by which the editors of meisho zue recorded a version of cultural geography that normalized this vision of prosperity. Keywords: Japan, cartography, Akisato Ritō, meisho zue, illustrated book, map, prosperity Entertaining exhibitions arrayed on the dry bed of the Kamo River distracted throngs of people seeking relief from the summer heat in Tokugawa-era Kyoto.1 By the time Osaka-based ukiyo-e artist Takehara Shunchōsai (fl. -
Useful Junk? the Effects of Visual Embellishment on Comprehension and Memorability of Charts Scott Bateman, Regan L
CHI 2010: Graphs April 10–15, 2010, Atlanta, GA, USA Useful Junk? The Effects of Visual Embellishment on Comprehension and Memorability of Charts Scott Bateman, Regan L. Mandryk, Carl Gutwin, Aaron Genest, David McDine, Christopher Brooks Department of Computer Science, University of Saskatchewan, Saskatoon, Saskatchewan, Canada [email protected], [email protected], [email protected], [email protected], [email protected], [email protected] ABSTRACT Despite these minimalist guidelines, many designers Guidelines for designing information charts often state that include a wide variety of visual embellishments in their the presentation should reduce ‘chart junk’ – visual charts, from small decorations to large images and visual embellishments that are not essential to understanding the backgrounds. One well-known proponent of visual data. In contrast, some popular chart designers wrap the embellishment in charts is the graphic artist Nigel Holmes, presented data in detailed and elaborate imagery, raising the whose work regularly incorporates strong visual imagery questions of whether this imagery is really as detrimental to into the fabric of the chart [7] (e.g., Figure 1). understanding as has been proposed, and whether the visual embellishment may have other benefits. To investigate these issues, we conducted an experiment that compared embellished charts with plain ones, and measured both interpretation accuracy and long-term recall. We found that people’s accuracy in describing the embellished charts was no worse than for plain charts, and that their recall after a two-to-three-week gap was significantly better. Although we are cautious about recommending that all charts be produced in this style, our results question some of the premises of the minimalist approach to chart design. -
EMILY JOHNSON/CATALYST SHORE Tue, Jun 17−Sun, Jun 22 Times and Locations Vary
Northrop Presents EMILY JOHNSON/CATALYST SHORE Tue, Jun 17−Sun, Jun 22 Times and Locations Vary Emily Johnson/Catalyst in SHORE. Photo © Cameron Wittig. WELCOME Dear Northrop Dance Lovers, Photo © James Everest We are pleased to share the world premiere of SHORE, the newest work NORTHROP PRESENTS from Emily Johnson/Catalyst, and the culmination of all of the events of our Northrop Grand Reopening. EMILY JOHNSON/CATALYST SHORE is the final work in a trilogy by this amazing Minnesota-based artist, and Northrop has been honored to present both The Thank-you Bar and Niicugni— the first two works in this trilogy—in our previous seasons. These have been SHORE thoughtful and provoking works by an artist who is truly pushing the boundaries Emily Johnson of what dance and performance can mean. Artistic Director Being able to present SHORE as the closing event of our season is a perfect Ain Gordon example of the transformation that Northrop has undergone. SHORE joins Director together Northrop’s resident partners in very meaningful ways: James Everest Emily Johnson is a 2013 McKnight Choreography Artist Fellow (housed within Lead Collaboration, Music and Sound Direction Northrop), as well as a fellow of the University of Minnesota’s Institute for Advanced Study (one of Northrop’s new resident partners). The IAS is also Aretha Aoki, Krista Langberg, Nona Marie Invie, Fletcher Barnhill, Julia Bither Northrop Director Christine Tschida. Collaborative Team Photo by Patrick O’Leary. home to the River Life Program, whose theme of the river and shore is one that Johnson’s work explores. Emily Johnson/Catalyst has also worked with another resident partner, the University Honors Program, on the volunteerism activities as part of SHORE. -
C 2013 EAFIT University. All Rights Reserved
. c 2013 EAFIT University. All rights reserved. 1 Geometry and Topology Extraction and Visualization from Scalar and Vector Fields John Congote EAFIT UNIVERSITY COLLEGE OF ENGINEERING DOCTORAL PROGRAM IN ENGINEERING MEDELLIN, COLOMBIA 2013 3 DOCTORAL PUBLICATION COPENDIUM ON Geometry and Topology Extraction and Visualization from Scalar and Vector Fields DOCTORAL STUDENT John Congote ADVISOR Prof. Dr. Eng. Oscar Ruiz CO-ADVISOR Dr. Eng. Jorge Posada DISSERTATION Submitted in partial fulfillment of the requirements for the degree of Doctor of Philosophy in Engineering in the College of Engineering of the EAFIT University EAFIT UNIVERSITY COLLEGE OF ENGINEERING DOCTORAL PROGRAM IN ENGINEERING MEDELLIN, COLOMBIA JUNE 2013 5 Major Field of Study Computer Graphics Dissertation GEOMETRY AND TOPOLOGY EXTRACTION AND The College of Engineering of VISUALIZATION FROM SCALAR AND VECTOR FIELDS EAFIT University Dissertation Committee Chairperson announces the Prof. Dr. Ing. Oscar Ruiz. Universidad EAFIT, Colombia final examination of Directors of Dissertation Research John Edgar Congote Calle Prof. Dr. Ing. Oscar Ruiz. Universidad EAFIT, Colombia Dr. Ing. Jorge León Posada. VICOMTECH Institute, Spain for the degree of Doctor of Philosophy in Engineering Examining Committee Prof. Dr. Ing. Diego A. Acosta Universidad EAFIT, Colombia Friday, June 07, 2013 Dr. Ing. Aitor Moreno at 10:00 am VICOMTECH Institute, Spain Room 27 – 103 Prof. Dr. Ing. Juan G. Lalinde Medellin Campus Universidad EAFIT, Colombia President of the Board of the Doctoral Program in Engineering Dean Alberto Rodriguez Garcia COLOMBIA This examination is open to the public ABSTRACT This doctoral work contributes in several major aspects: (1) Doctoral Supervisor Prof. Dr. Eng. Oscar E. Ruiz Extraction of geometrical and topological information of 1- and Professor Oscar Ruiz (Tunja, Colombia 1961) obtained 2-manifolds present in static and video imaging. -
Nigel Holmes Characterizes the Opposite End of the Spectrum, Which Supports the Heavy Use of Illustration and Decoration to Embellish Information Design (Figure 1.5)
Contents Introduction A Brief History of Infographics The Purpose of This Book What This Book is Not A Note on Terminology How to Use This Book Chapter 1: Importance and Efficacy: Why Our Brains Love Infographics Varied Perspectives on Information Design: A Brief History Objectives of Visualization Appeal Comprehension Retention Chapter 2: Infographic Formats: Choosing The Right Vehicle For Your Message Static Infographics Motion Graphics Interactive Infographics Chapter 3: The Visual Storytelling Spectrum: An Objective Approach Understanding The Visual Storytelling Spectrum Chapter 4: Editorial Infographics What Are Editorial Infographics? Origins of Editorial Infographics Editorial Infographic Production Chapter 5: Content Distribution: Sharing Your Story Posting On Your Site Distributing Your Content Patience Pays Dividends Chapter 6: Brand-Centric Infographics “About Us” Pages Product Instructions Visual Press Releases Presentation Design Annual Reports Chapter 7: Data Visualization Interfaces A Case For Visualization in User Interfaces Dashboards Visual Data Hubs Chapter 8: What Makes A Good Infographic? Utility Soundness Beauty Chapter 9: Information Design Best Practices Illustration Data Visualization Chapter 10: The Future of Infographics Democratized Access to Creation Tools Socially Generative Visualizations Problem Solving Becoming A Visual Company Further Infographic Goodness Thank You Index Copyright © 2012 by Column Five Media. All rights reserved. Published by John Wiley & Sons, Inc., Hoboken, New Jersey. Published simultaneously -
Master Thesis SC Final
The Potential Role for Infographics in Science Communication By: Laura Mol (2123177) Biomedical Sciences Master Thesis Communication specialization (9 ECTS) Vrije Universiteit Amsterdam Under supervision of dr. Frank Kupper, Athena Institute, Vrije Universiteit Amsterdam November 2011 Cover art: ‘Nonsensical Infograhics’ by Chad Hagen (www.chadhagen.com) "Tell me and I'll forget; show me and I may remember; involve me and I'll understand" - Chinese proverb - 2 Index !"#$%&'$()))))))))))))))))))))))))))))))))))))))))))))))))))))))))))))))))))))))))))))))))))))))))))))))))))))))))))))))))))))))))))))))))(*! "#!$%&'()*+&,(%())))))))))))))))))))))))))))))))))))))))))))))))))))))))))))))))))))))))))))))))))))))))))))))))))))))))))))))))))))))(+! ,),! !(#-.%$(-/#$.%0(.1(#'/23'2('.4453/'&$/.3())))))))))))))))))))))))))))))))))))))))))))))))))))))))))))))(6! ,)7! 8#/39(/4&92#(/3(#'/23'2('.4453/'&$/.3()))))))))))))))))))))))))))))))))))))))))))))))))))))))))))))))))(:! -#!$%.(/'012,+3()))))))))))))))))))))))))))))))))))))))))))))))))))))))))))))))))))))))))))))))))))))))))))))))))))))))))))))))))))(,;! 7),! <3$%.=5'$/.3())))))))))))))))))))))))))))))))))))))))))))))))))))))))))))))))))))))))))))))))))))))))))))))))))))))))))))))))))))(,;! 7)7! >/#$.%0()))))))))))))))))))))))))))))))))))))))))))))))))))))))))))))))))))))))))))))))))))))))))))))))))))))))))))))))))))))))))))))))(,,! 7)?! @/112%23$(AB2423$#())))))))))))))))))))))))))))))))))))))))))))))))))))))))))))))))))))))))))))))))))))))))))))))))))))))))(,:! 7)*! C5%D.#2()))))))))))))))))))))))))))))))))))))))))))))))))))))))))))))))))))))))))))))))))))))))))))))))))))))))))))))))))))))))))))))(,:! -
Behind the Scenes
©Lonely Planet Publications Pty Ltd 438 Behind the Scenes SEND US YOUR FEEDBACK We love to hear from travellers – your comments keep us on our toes and help make our books better. Our well-travelled team reads every word on what you loved or loathed about this book. Although we cannot reply individually to your submissions, we always guarantee that your feed- back goes straight to the appropriate authors, in time for the next edition. Each person who sends us information is thanked in the next edition – the most useful submissions are rewarded with a selection of digital PDF chapters. Visit lonelyplanet.com/contact to submit your updates and suggestions or to ask for help. Our award-winning website also features inspirational travel stories, news and discussions. Note: We may edit, reproduce and incorporate your comments in Lonely Planet products such as guidebooks, websites and digital products, so let us know if you don’t want your comments reproduced or your name acknowledged. For a copy of our privacy policy visit lonelyplanet.com/ privacy. Tamara Decaluwe, Terence Boley, Thomas Van OUR READERS Loock, Tim Elliott, Ylwa Alwarsdotter Many thanks to the travellers who used the last edition and wrote to us with help- ful hints, useful advice and interesting WRITER THANKS anecdotes: Alex Wharton, Amy Nguyen, Andrew Selth, Simon Richmond Angela Tucker, Anita Kuiper, Annabel Dunn, An- Many thanks to my fellow authors and the fol- nette Lüthi, Anthony Lee, Bernard Keller, Carina lowing people in Yangon: William Myatwunna, Hall, Christina Pefani, Christoph Knop, Chris- Thant Myint-U, Edwin Briels, Jessica Mudditt, toph Mayer, Claudia van Harten, Claudio Strep- Jaiden Coonan, Tim Aye-Hardy, Ben White, parava, Dalibor Mahel, Damian Gruber, David Myo Aung, Marcus Allender, Jochen Meissner, Jacob, Don Stringman, Elisabeth Schwab, Khin Maung Htwe, Vicky Bowman, Don Wright, Elisabetta Bernardini, Erik Dreyer, Florian James Hayton, Jeremiah Whyte and Jon Boos, Gabriella Wortmann, Garth Riddell, Gerd Keesecker. -
CSE 564: Visualization
CSE 564: Visualization The Views of Edward Tufte (and Some Others) Klaus Mueller Computer Science Department Stony Brook University Seminal Books by Edward Tufte Standard literature for every visualization enthusiast • written 1983, 1990, 1997, 2006 Edward Tufte Well recognized for his writings on information design • a pioneer in the field of data visualization • taught information design at Princeton University • now a professor at Yale University Popularized concept of “small multiples” • aka trellis chart or panel chart • similar charts of same scale + axes • allows them to be easily compared • use multiple views to show different partitions of a dataset Small Multiples – Historical Reference E. Muybridge’s Horses in Motion (1886) • proofed for the first time that horses CAN have all 4 legs in the air • work was also foundational to the development of the motion picture Small Multiples – Historical Reference FA Walker’s census charts (1870) • population is broken down by state and then occupation, including a count of those attending school • also has tree maps! Edward Tufte Also popularized “sparklines” • small integrative visualizations Sparklines inspired “word size visualizations” • charts or graphs tightly integrated into text or even computer code Tufte on Graphical Excellence The Need for Visualization: Anscombe Quartet The Need for Visualization: Anscombe Quartet John Snow: London Cholera Map (1854) Age-Adjusted Cancer Rates (by County) 21,000 numbers 3056 counties 7 numbers per county: - size (4) - location (2) - cancer rate -
Infographics As Images: Meaningfulness Beyond Information †
Proceedings Infographics as Images: Meaningfulness beyond Information † Valeria Burgio *and Matteo Moretti Faculty of Design and Art. Libera Università di Bolzano; Piazza Università, 39100 Bolzano Bozen, Italy; [email protected] * Correspondence: [email protected] † Presented at the International and Interdisciplinary Conference IMMAGINI? Image and Imagination between Representation, Communication, Education and Psychology, Brixen, Italy, 27–28 November 2017. Published: 10 November 2017 Abstract: What kind of images are data visualizations? Are they mere abstract transformations of numerical data? Should they reduce the phenomenal world into a set of pre-codified shapes? Or can they represent natural phenomena through figurative strategies? What is the boundary between useless decoration, narrative illustration and helpful visual metaphors? Through post-design reflections on a visual journalism project, the paper focuses on the context-dependent role of images in data visualization. Keywords: data visualization; visual journalism; illustration and decoration; abstraction and pictographic communication 1. Introduction What kind of images are data visualizations? Are they mere abstract transformations of numerical data? Should they reduce the phenomenal world into a set of pre-codified shapes? Or can they represent natural phenomena through figurative strategies? Where does the boundary lie between useless decoration, narrative illustration and helpful visual metaphors? Since the theoretical work and practical implementation of Otto Neurath [1,2], much has been argued about the necessary balance between scientific accuracy and communicational efficiency in data visualization. Since then, how to translate quantitative information into readable graphs has been the terrain for debate between the advocates of dryness and objectivity [3–5] and the defenders of a more playful and inventive approach [6,7]. -
Thesis (6.228Mb)
Visualizing Information Graphics How to design effective charts and graphs Mariana C. Mora Cano | Master of Fine Arts in Integrated Design | Thesis Project, Fall 2011 Visualizing Information Graphics How to design effective charts and graphs Mariana C. Mora Cano | Master of Fine Arts in Integrated Design |Thesis Project, Fall 2011 Table of Contents Overview.................................................................................................... 4 Objectives.................................................................................................. 5 Audience.................................................................................................... 6 Market....................................................................................................... 7 Process...................................................................................................... 8 Project components.................................................................................. 9 Site map........................................................................................... 9 Design elements............................................................................... 10 Web site features............................................................................... 11 Distribution............................................................................................... 18 Bibliography.............................................................................................. 19 Appendix..................................................................................................