UNIVERSITY of MYSORE Estd
Total Page:16
File Type:pdf, Size:1020Kb
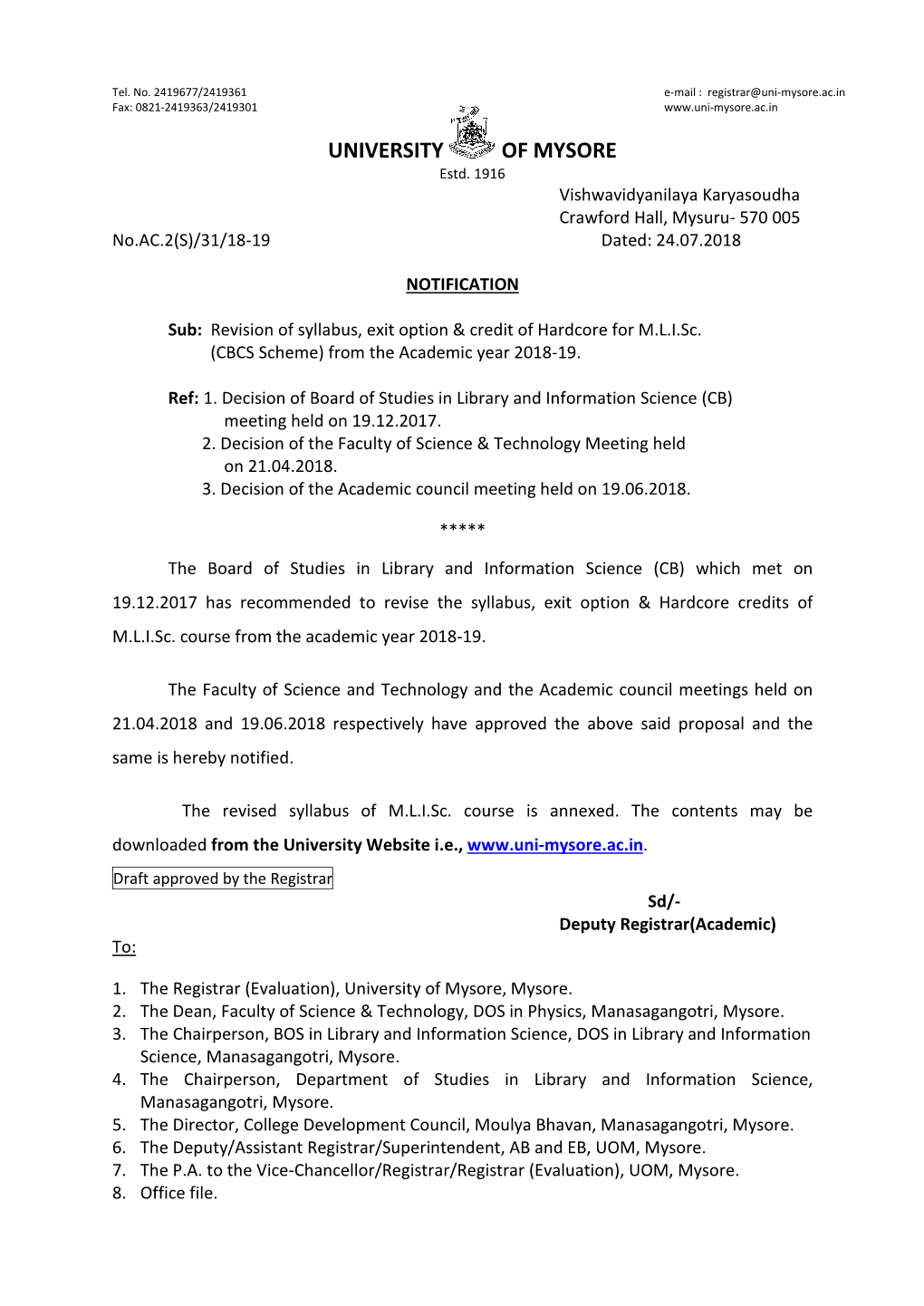
Load more
Recommended publications
-
Web Query Classification and URL Ranking Based on Click Through Approach
S.PREETHA et al, International Journal of Computer Science and Mobile Computing Vol.2 Issue. 10, October- 2013, pg. 138-144 Available Online at www.ijcsmc.com International Journal of Computer Science and Mobile Computing A Monthly Journal of Computer Science and Information Technology ISSN 2320–088X IJCSMC, Vol. 2, Issue. 10, October 2013, pg.138 – 144 RESEARCH ARTICLE Web Query Classification and URL Ranking Based On Click through Approach S.PREETHA1, K.N Vimal Shankar2 PG Scholar1, Asst. Professor2 Department of Computer Science & Engineering1, 2 V.S.B. Engineering College, Karur, India1, 2 [email protected], [email protected] Abstract― In a web based application; different users may have different search goals when they submit it to a search engine. For a broad-topic and ambiguous query it is difficult. Here we propose a novel approach to infer user search goals by analyzing search engine query logs. This is typically shown in cases such as these: Different users have different backgrounds and interests. However, effective personalization cannot be achieved without accurate user profiles. We propose a framework that enables large-scale evaluation of personalized search. The goal of personalized IR (information retrieval) is to return search results that better match the user intent. First, we propose a framework to discover different user search goals for a query by clustering the proposed feedback sessions. Feedback sessions are getting constructed from user click-through logs and can efficiently reflect the information needs of users. Second, we propose an approach to generate pseudo-documents to better represent the feedback sessions for clustering. -
Classifying User Search Intents for Query Auto-Completion
Classifying User Search Intents for Query Auto-Completion Jyun-Yu Jiang Pu-Jen Cheng Department of Computer Science Department of Computer Science University of California, Los Angeles National Taiwan University California 90095, USA Taipei 10617, Taiwan [email protected] [email protected] ABSTRACT completion is to accurately predict the user's intended query The function of query auto-completion1 in modern search with only few keystrokes (or even without any keystroke), engines is to help users formulate queries fast and precisely. thereby helping the user formulate a satisfactory query with Conventional context-aware methods primarily rank candi- less effort and less time. For instance, users can avoid mis- date queries2 according to term- and query- relationships to spelling and ambiguous queries with query completion ser- the context. However, most sessions are extremely short. vices. Hence, how to capture user's search intents for pre- How to capture search intents with such relationships be- cisely predicting the query one is typing is very important. The context information, such as previous queries and comes difficult when the context generally contains only few 3 queries. In this paper, we investigate the feasibility of dis- click-through data in the same session , is usually utilized covering search intents within short context for query auto- to capture user's search intents. It also has been exten- completion. The class distribution of the search session (i.e., sively studied in both query completion [1, 21, 35] and sug- gestion [18, 25, 37]. Previous work primarily focused on cal- issued queries and click behavior) is derived as search in- 2 tents. -
Crawling Frontier Controls
Nutch – ApacheCon US '09 Web-scale search engine toolkit search Web-scale Today and tomorrow Today Apache Andrzej Białecki [email protected] Nutch – ApacheCon US '09 • • • Questions answers and future Nutch present and solutions)some (and Challenges Nutchworkflow: Nutcharchitecture overview general Web in crawling project the About Searching Crawling Setup Agenda 2 Nutch – ApacheCon US '09 • • Collections typically 1 mln - 200 mln documents mln Collections -typically 200 1 mln search mostly vertical in operation, installations Many Spin-offs: (sub-project Lucene) of Apache project since 2004 Mike Cafarella creator, and Lucene bythe Cutting, Doug 2003 in Founded Content type detection and parsing Tika → Map-Reduce and distributed → Hadoop FS Apache Nutch project 3 Nutch – ApacheCon US '09 4 Nutch – ApacheCon US '09 first, random Traversal: depth- breadth-first, edges, the follow listsas Oftenadjacency represented (neighbor) <alabels: href=”..”>anchor Edge text</a> Edges (links): hyperlinks like <a href=”targetUrl”/> Nodes (vertices):URL-s identifiers as unique 6 2 8 1 3 Web as a directed graph 5 4 7 9 7 →3, 4, 8, 9 5 →6, 9 1 →2, 3, 4, 5, 6 5 Nutch – ApacheCon US '09 … What's in a search engine? a fewa things may surprisethat you! 6 Nutch – ApacheCon US '09 Injector -links(in/out) - Web graph pageinfo Search engine building blocks Scheduler Updater Crawling frontierCrawling controls Crawler repository Content Searcher Indexer Parser 7 Nutch – ApacheCon US '09 Robust API and integration options Robust APIintegration and Full-text&indexer search engine processingdata framework Scalable Robustcontrols frontier crawling processing (parsing, content filtering) Plugin-based crawler distributed multi-threaded, Multi-protocol, modular: highly Plugin-based, graph) (web link database and database Page − − − − Support Support for search distributed or Using Lucene Solr Map-reduce processing Mostvia plugins be behavior can changed Nutch features at a glance 8 Hadoop foundation File system abstraction • Local FS, or • Distributed FS − also Amazon S3, Kosmos and other FS impl. -
The Google Search Engine
University of Business and Technology in Kosovo UBT Knowledge Center Theses and Dissertations Student Work Summer 6-2010 The Google search engine Ganimete Perçuku Follow this and additional works at: https://knowledgecenter.ubt-uni.net/etd Part of the Computer Sciences Commons Faculty of Computer Sciences and Engineering The Google search engine (Bachelor Degree) Ganimete Perçuku – Hasani June, 2010 Prishtinë Faculty of Computer Sciences and Engineering Bachelor Degree Academic Year 2008 – 2009 Student: Ganimete Perçuku – Hasani The Google search engine Supervisor: Dr. Bekim Gashi 09/06/2010 This thesis is submitted in partial fulfillment of the requirements for a Bachelor Degree Abstrakt Përgjithësisht makina kërkuese Google paraqitet si sistemi i kompjuterëve të projektuar për kërkimin e informatave në ueb. Google mundohet t’i kuptojë kërkesat e njerëzve në mënyrë “njerëzore”, dhe t’iu kthej atyre përgjigjen në formën të qartë. Por, ky synim nuk është as afër ideales dhe realizimi i tij sa vjen e vështirësohet me zgjerimin eksponencial që sot po përjeton ueb-i. Google, paraqitet duke ngërthyer në vetvete shqyrtimin e pjesëve që e përbëjnë, atyre në të cilat sistemi mbështetet, dhe rrethinave tjera që i mundësojnë sistemit të funksionojë pa probleme apo të përtërihet lehtë nga ndonjë dështim eventual. Procesi i grumbullimit të të dhënave ne Google dhe paraqitja e tyre në rezultatet e kërkimit ngërthen në vete regjistrimin e të dhënave nga ueb-faqe të ndryshme dhe vendosjen e tyre në rezervuarin e sistemit, përkatësisht në bazën e të dhënave ku edhe realizohen pyetësorët që kthejnë rezultatet e radhitura në mënyrën e caktuar nga algoritmi i Google. -
Web Crawling, Analysis and Archiving
Web Crawling, Analysis and Archiving Vangelis Banos Aristotle University of Thessaloniki Faculty of Sciences School of Informatics Doctoral dissertation under the supervision of Professor Yannis Manolopoulos October 2015 Ανάκτηση, Ανάλυση και Αρχειοθέτηση του Παγκόσμιου Ιστού Ευάγγελος Μπάνος Αριστοτέλειο Πανεπιστήμιο Θεσσαλονίκης Σχολή Θετικών Επιστημών Τμήμα Πληροφορικής Διδακτορική Διατριβή υπό την επίβλεψη του Καθηγητή Ιωάννη Μανωλόπουλου Οκτώβριος 2015 i Web Crawling, Analysis and Archiving PhD Dissertation ©Copyright by Vangelis Banos, 2015. All rights reserved. The Doctoral Dissertation was submitted to the the School of Informatics, Faculty of Sci- ences, Aristotle University of Thessaloniki. Defence Date: 30/10/2015. Examination Committee Yannis Manolopoulos, Professor, Department of Informatics, Aristotle University of Thes- saloniki, Greece. Supervisor Apostolos Papadopoulos, Assistant Professor, Department of Informatics, Aristotle Univer- sity of Thessaloniki, Greece. Advisory Committee Member Dimitrios Katsaros, Assistant Professor, Department of Electrical & Computer Engineering, University of Thessaly, Volos, Greece. Advisory Committee Member Athena Vakali, Professor, Department of Informatics, Aristotle University of Thessaloniki, Greece. Anastasios Gounaris, Assistant Professor, Department of Informatics, Aristotle University of Thessaloniki, Greece. Georgios Evangelidis, Professor, Department of Applied Informatics, University of Mace- donia, Greece. Sarantos Kapidakis, Professor, Department of Archives, Library Science and Museology, Ionian University, Greece. Abstract The Web is increasingly important for all aspects of our society, culture and economy. Web archiving is the process of gathering digital materials from the Web, ingesting it, ensuring that these materials are preserved in an archive, and making the collected materials available for future use and research. Web archiving is a difficult problem due to organizational and technical reasons. We focus on the technical aspects of Web archiving. -
Automatic Web Query Classification Using Labeled and Unlabeled Training Data Steven M
Automatic Web Query Classification Using Labeled and Unlabeled Training Data Steven M. Beitzel, Eric C. Jensen, David D. Lewis, Abdur Chowdhury, Ophir Frieder, David Grossman Aleksandr Kolcz Information Retrieval Laboratory America Online, Inc. Illinois Institute of Technology [email protected] {steve,ej,ophir,dagr}@ir.iit.edu {cabdur,arkolcz}@aol.com ABSTRACT We examine three methods for classifying general web queries: Accurate topical categorization of user queries allows for exact match against a large set of manually classified queries, a increased effectiveness, efficiency, and revenue potential in weighted automatic classifier trained using supervised learning, general-purpose web search systems. Such categorization and a rule-based automatic classifier produced by mining becomes critical if the system is to return results not just from a selectional preferences from hundreds of millions of unlabeled general web collection but from topic-specific databases as well. queries. The key contribution of this work is the development Maintaining sufficient categorization recall is very difficult as of a query classification framework that leverages the strengths web queries are typically short, yielding few features per query. of all three individual approaches to achieve the most effective We examine three approaches to topical categorization of classification possible. Our combined approach outperforms general web queries: matching against a list of manually labeled each individual method, particularly improves recall, and allows queries, supervised learning of classifiers, and mining of us to effectively classify a much larger proportion of an selectional preference rules from large unlabeled query logs. operational web query stream. Each approach has its advantages in tackling the web query classification recall problem, and combining the three 2. -
Semantic Matching in Search
Foundations and Trends⃝R in Information Retrieval Vol. 7, No. 5 (2013) 343–469 ⃝c 2014 H. Li and J. Xu DOI: 10.1561/1500000035 Semantic Matching in Search Hang Li Huawei Technologies, Hong Kong [email protected] Jun Xu Huawei Technologies, Hong Kong [email protected] Contents 1 Introduction 3 1.1 Query Document Mismatch ................. 3 1.2 Semantic Matching in Search ................ 5 1.3 Matching and Ranking ................... 9 1.4 Semantic Matching in Other Tasks ............. 10 1.5 Machine Learning for Semantic Matching in Search .... 11 1.6 About This Survey ...................... 14 2 Semantic Matching in Search 16 2.1 Mathematical View ..................... 16 2.2 System View ......................... 19 3 Matching by Query Reformulation 23 3.1 Query Reformulation .................... 24 3.2 Methods of Query Reformulation .............. 25 3.3 Methods of Similar Query Mining .............. 32 3.4 Methods of Search Result Blending ............. 38 3.5 Methods of Query Expansion ................ 41 3.6 Experimental Results .................... 44 4 Matching with Term Dependency Model 45 4.1 Term Dependency ...................... 45 ii iii 4.2 Methods of Matching with Term Dependency ....... 47 4.3 Experimental Results .................... 53 5 Matching with Translation Model 54 5.1 Statistical Machine Translation ............... 54 5.2 Search as Translation .................... 56 5.3 Methods of Matching with Translation ........... 59 5.4 Experimental Results .................... 61 6 Matching with Topic Model 63 6.1 Topic Models ........................ 64 6.2 Methods of Matching with Topic Model .......... 70 6.3 Experimental Results .................... 74 7 Matching with Latent Space Model 75 7.1 General Framework of Matching .............. 76 7.2 Latent Space Models ................... -
A New Algorithm for Inferring User Search Goals with Feedback Sessions
International Journal of Advanced Research in Computer Engineering & Technology (IJARCET) Volume 3 Issue 11, November 2014 A Survey on: A New Algorithm for Inferring User Search Goals with Feedback Sessions Swati S. Chiliveri, Pratiksha C.Dhande a few words to search engines. Because these queries are short Abstract— For a broad-topic and ambiguous query, different and ambiguous, how to interpret the queries in terms of a set users may have different search goals when they submit it to a of target categories has become a major research issue. search engine. The inference and analysis of user search goals can be very useful in improving search engine relevance and For example, when the query ―the sun‖ is submitted to a user experience. This paper provides search engine, some people want to locate the homepage of a an overview of the system architecture of proposed feedback United Kingdom newspaper, while some people want to session framework with their advantages. Also we have briefly discussed the literature survey on Automatic Identification of learn the natural knowledge of the sun. Therefore, it is User Goals in Web Search with their pros and cons. We have necessary and potential to capture different user search goals, presented working of various classification approaches such as user needs in information retrieval. We define user search SVM, Exact matching and Bridges etc. under the web query goals as the information on different aspects of a query that classification framework. Lastly, we have described the existing user groups want to obtain. Information need is a user’s web clustering engines such as MetaCrawler, Carrot 2, particular desire to obtain information to satisfy users need. -
Detecting Malicious Websites with Low-Interaction Honeyclients
Monkey-Spider: Detecting Malicious Websites with Low-Interaction Honeyclients Ali Ikinci Thorsten Holz Felix Freiling University of Mannheim Mannheim, Germany Abstract: Client-side attacks are on the rise: malicious websites that exploit vulner- abilities in the visitor’s browser are posing a serious threat to client security, compro- mising innocent users who visit these sites without having a patched web browser. Currently, there is neither a freely available comprehensive database of threats on the Web nor sufficient freely available tools to build such a database. In this work, we in- troduce the Monkey-Spider project [Mon]. Utilizing it as a client honeypot, we portray the challenge in such an approach and evaluate our system as a high-speed, Internet- scale analysis tool to build a database of threats found in the wild. Furthermore, we evaluate the system by analyzing different crawls performed during a period of three months and present the lessons learned. 1 Introduction The Internet is growing and evolving every day. More and more people are becoming part of the so-called Internet community. With this growth, also the amount of threats for these people is increasing. Online criminals who want to destroy, cheat, con others, or steal goods are evolving rapidly [Ver03]. Currently, there is no comprehensive and free database to study malicious websites found on the Internet. Malicious websites are websites which have any kind of content that could be a threat for the security of the clients requesting these sites. For example, a malicious website could exploit a vulnerability in the visitor’s web browser and use this to compromise the system and install malware on it. -
Most Valuable (Cited)
Top 100 Most Valuable Patent Portfolios Based on 3 million patents issued from 1-1-2005 to 3-21-2017 Methodology: 1) Identify all US-based patents and their patent citations 2) Identify most cited patents (patent must be cited > 10x as 10x is the average) 3) Aggregate all of the "most cited patents" into their Assignees and sort Patents Total Average Rank Assignee Being Cited Citations Citations/Patent 1 International Business Machines Corporation 5902 163252 27.66 2 Microsoft Corporation 4688 142191 30.33 3 Ethicon Endo-Surgery, Inc. 715 97544 136.43 4 Western Digital Technologies, Inc. 972 71947 74.02 5 Intel Corporation 2273 66689 29.34 6 Hewlett-Packard Development Company, L.P. 1922 58888 30.64 7 Tyco Healthcare Group LP 498 56695 113.85 8 Samsung Electronics Co., Ltd. 2097 53863 25.69 9 Canon Kabushiki Kaisha 1950 52703 27.03 10 Cisco Technology, Inc. 1702 51491 30.25 11 Micron Technology, Inc. 1567 48443 30.91 12 Medtronic, Inc. 1049 42652 40.66 13 Sony Corporation 1454 37963 26.11 14 Semiconductor Energy Laboratory Co., Ltd. 1177 36719 31.20 15 Apple Inc. 1057 36234 34.28 16 Hitachi, Ltd. 1314 36126 27.49 17 Western Digital (Fremont), LLC 322 36007 111.82 18 Masimo Corporation 220 35402 160.92 19 IGT 746 35063 47.00 20 Nokia Corporation 1110 34107 30.73 21 Kabushiki Kaisha Toshiba 1346 33712 25.05 22 Sun Microsystems, Inc. 982 29744 30.29 23 Matsushita Electric Industrial Co., Ltd. 1092 29719 27.22 24 General Electric Company 1301 29432 22.62 25 Silverbrook Research PTY LTD 656 25941 39.54 26 Boston Scientific SciMed, Inc. -
Improving Search Engines Via Classification
Improving Search Engines via Classi¯cation Zheng Zhu May 2011 A Dissertation Submitted to Birkbeck College, University of London in Partial Ful¯llment of the Requirements for the Degree of Doctor of Philosophy Department of Computer Science & Information Systems Birkbeck College University of London Declaration This thesis is the result of my own work, except where explicitly acknowledge in the text. Zheng Zhu i Abstract In this dissertation, we study the problem of how search engines can be improved by making use of classi¯cation. Given a user query, traditional search engines output a list of results that are ranked according to their relevance to the query. However, the ranking is independent of the topic of the document. So the results of di®erent topics are not grouped together within the result output from a search engine. This can be problematic as the user must scroll though many irrelevant results until his/her desired information need is found. This might arise when the user is a novice or has super¯cial knowledge about the domain of interest, but more typically it is due to the query being short and ambiguous. One solution is to organise search results via categorization, in particular, the classi¯cation. We designed a target testing experiment on a controlled data set, which showed that classi¯cation-based search could improve the user's search experience in terms of the numbers of results the user would have to inspect before satisfying his/her query. In our investigation of classi¯cation to organise search results, we not only consider the classi¯cation of search results, but also query classi¯cation. -
Building Bridges for Web Query Classification
Building Bridges for Web Query Classification Dou Shen†, Jian-Tao Sun‡, Qiang Yang†, Zheng Chen‡ †Department of Computer Science and Engineering, Hong Kong University of Science and Technology {dshen,qyang}@cs.ust.hk ‡Microsoft Research Asia, Beijing, P.R.China {jtsun, zhengc}@microsoft.com ABSTRACT 1. INTRODUCTION Web query classification (QC) aims to classify Web users’ With exponentially increasing information becoming avail- queries, which are often short and ambiguous, into a set of able on the Internet, Web search has become an indispens- target categories. QC has many applications including page able tool for Web users to gain desired information. Typi- ranking in Web search, targeted advertisement in response cally, Web users submit a short Web query consisting of a to queries, and personalization. In this paper, we present a few words to search engines. Because these queries are short novel approach for QC that outperforms the winning solu- and ambiguous, how to interpret the queries in terms of a tion of the ACM KDDCUP 2005 competition, whose objec- set of target categories has become a major research issue. tive is to classify 800,000 real user queries. In our approach, In this paper, we call the problem of generating a ranked list we first build a bridging classifier on an intermediate tax- of target categories from user queries the query classification onomy in an offline mode. This classifier is then used in problem, or QC for short. an online mode to map user queries to the target categories The importance of QC is underscored by many services via the above intermediate taxonomy.