Computational Prediction of Functional Abortive RNA in E. Coli
Total Page:16
File Type:pdf, Size:1020Kb
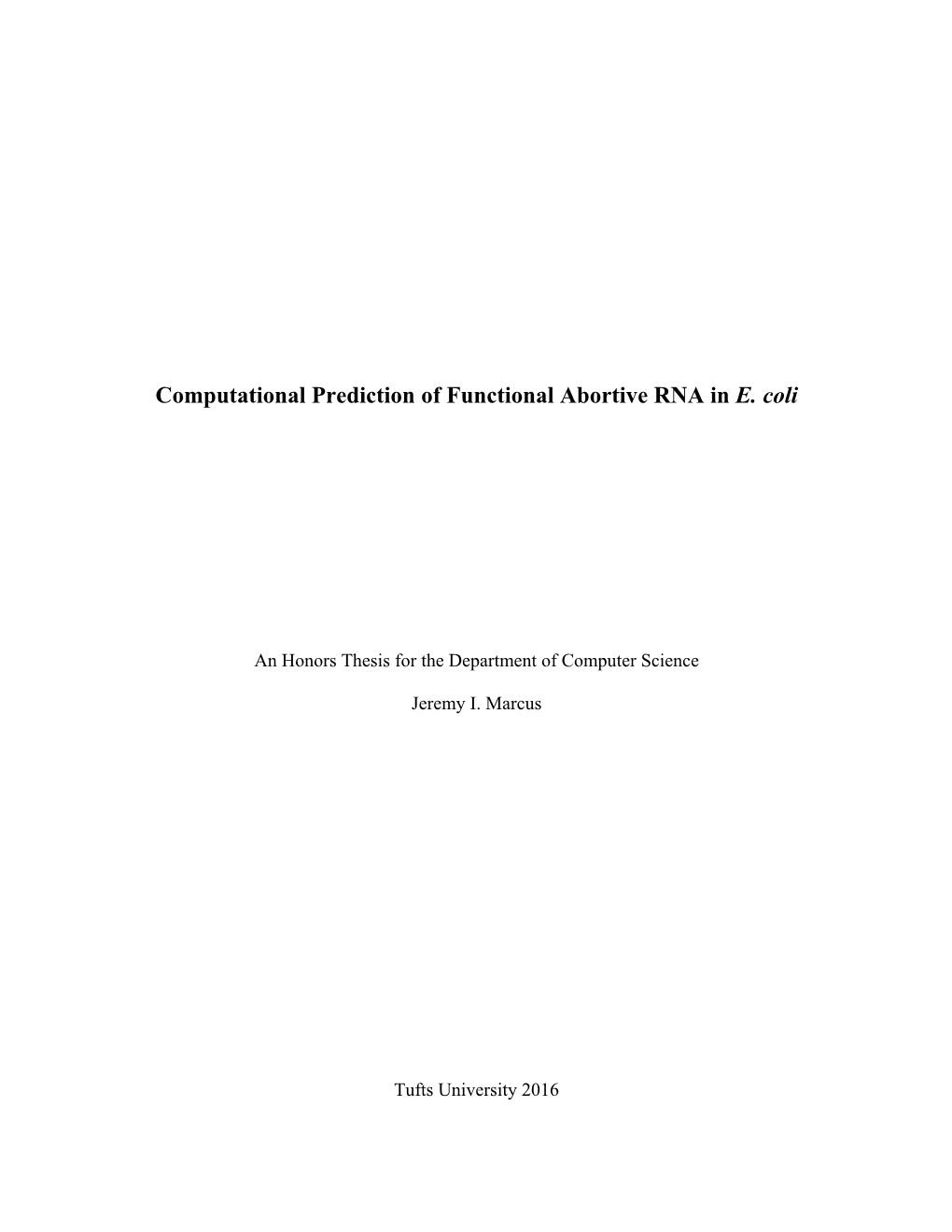
Load more
Recommended publications
-
In Vitro Comparison of Initiation Properties of Bacteriophage X Wild-Type PR and X3 Mutant Promoters (RNA Polymerase Mechanism/Abortive Initiation) DIANE K
Proc. Natl. Acad. Sci. USA Vol. 77, No. 11, pp. 6381-6385, November 1980 Biochemistry In vitro comparison of initiation properties of bacteriophage X wild-type PR and x3 mutant promoters (RNA polymerase mechanism/abortive initiation) DIANE K. HAWLEY AND WILLIAM R. MCCLURE Department of Biochemistry and Molecular Biology, Conant Laboratory, Harvard University, Cambridge, Massachusetts 02138 Communicated by Mark Ptashne, July 28,1980 ABSTRACT The in vitro initiation properties of the PR Our analysis is based on a simple two-step model of pro- promoter of bacteriophage X and of a PR mutant, x3, were moter-polymerase interaction first proposed by Zillig and his compared. Using the abortive initiation reaction, we measured coworkers (4). According to this model, RNA polymerase first the lags in the approach to a final steady-state rate when dinu- binds to the DNA in a transcriptionally inactive "closed" cleotide synthesis was initiated with RNA polymerase. These unwinds the DNA to form the lags corresponded to the average times required for the forma- complex (RPc) and subsequently tion of transcriptionally active open complexes. By measuring "open" complex (RPO) which then binds the nucleoside tri- the lags at different RNA polymerase concentrations, we could phosphates and initiates transcription (5). Formation of the open separate open complex formation into two steps, based on a complex can be described as follows: simple model in which the initial bimolecular association of free ks k2 promoter and polymerase in a closed complex is followed by an R + Pa SRPco- RPo. [1] isomerization to the open complex. The contribution of each k-1 k-2 step to the overall rate of open complex formation was quanti- tated for both promoters. -
Backtracked and Paused Transcription Initiation Intermediate of Escherichia Coli RNA Polymerase
Backtracked and paused transcription initiation intermediate of Escherichia coli RNA polymerase Eitan Lernera,1, SangYoon Chunga,1, Benjamin L. Allenb,1, Shuang Wangc,1, Jookyung Leed, Shijia W. Lua, Logan W. Grimauda, Antonino Ingargiolaa, Xavier Michaleta, Yazan Alhadida, Sergei Borukhovd, Terence R. Strickc,e,f,2, Dylan J. Taatjesb,2, and Shimon Weissa,g,h,2 aDepartment of Chemistry & Biochemistry, University of California, Los Angeles, CA 90095; bDepartment of Chemistry & Biochemistry, University of Colorado, Boulder, CO 80309; cInstitut Jacques Monod, Centre National de la Recherche Scientifique (CNRS), UMR7592, University Paris Diderot, Sorbonne Paris Cité, F-75205 Paris, France; dRowan University School of Osteopathic Medicine, Stratford, NJ 08084; eInstitut de Biologie de l’Ecole Normale Supérieure, Institut de Biologie de l’Ecole Normale Superieure (IBENS), CNRS, Inserm, Ecole Normale Supérieure, Paris Sciences et Lettres (PSL) Research University, F-75005 Paris, France; fProgramme Equipe Labellisées, Ligue Contre le Cancer, 75013 Paris, France; gMolecular Biology Institute, University of California, Los Angeles, CA 90095; and hDepartment of Physiology, University of California, Los Angeles, CA 90095 Edited by Steven M. Block, Stanford University, Stanford, CA, and approved September 13, 2016 (received for review March 30, 2016) Initiation is a highly regulated, rate-limiting step in transcription. the PRS, nascent RNA enters the RNA exit channel and tran- We used a series of approaches to examine the kinetics of RNA scription enters the elongation stage. polymerase (RNAP) transcription initiation in greater detail. Quenched In AI, the interactions between σ70 and the PRS limit the kinetics assays, in combination with gel-based assays, showed that lengths of abortive transcripts. -
Card Uses a Minor Groove Wedge Mechanism to Stabilize the RNA
1 CarD uses a minor groove wedge mechanism to stabilize the RNA 2 polymerase open promoter complex 3 4 Brian Bae1, James Chen1, Elizabeth Davis1, Katherine Leon1, Seth A. Darst1,*, 5 Elizabeth A. Campbell1,* 6 7 1The Rockefeller University, Laboratory for Molecular Biophysics, 1230 York Avenue, New York, 8 NY 10065, USA. 9 10 *Correspondence to: E-mail: [email protected], [email protected] 11 12 Present Address: Elizabeth Davis, The University of Minnesota School of Medicine, 13 420 Delaware St. SE, Minneapolis, MN 55455, USA; Katherine Leon, Department of 14 Biochemistry and Molecular Biology, University of Chicago, 929 East 57th Street, GCIS 15 W219 Chicago, IL 60637, USA. 16 17 2 18 Abstract A key point to regulate gene expression is at transcription initiation, and 19 activators play a major role. CarD, an essential activator in Mycobacterium tuberculosis, 20 is found in many bacteria, including Thermus species, but absent in Escherichia coli. To 21 delineate the molecular mechanism of CarD, we determined crystal structures of 22 Thermus transcription initiation complexes containing CarD. The structures show CarD 23 interacts with the unique DNA topology presented by the upstream double- 24 stranded/single-stranded DNA junction of the transcription bubble. We confirm that our 25 structures correspond to functional activation complexes, and extend our understanding 26 of the role of a conserved CarD Trp residue that serves as a minor groove wedge, 27 preventing collapse of the transcription bubble to stabilize the transcription initiation 28 complex. Unlike E. coli RNAP, many bacterial RNAPs form unstable promoter 29 complexes, explaining the need for CarD. -
Open Complex Scrunching Before Nucleotide Addition Accounts for The
Open complex scrunching before nucleotide addition PNAS PLUS accounts for the unusual transcription start site of E. coli ribosomal RNA promoters Jared T. Winkelmana,1, Pete Chandrangsua,1, Wilma Rossa, and Richard L. Goursea,2 aDepartment of Bacteriology, University of Wisconsin-Madison, Madison, WI 53706 Edited by Jeffrey W. Roberts, Cornell University, Ithaca, NY, and approved February 23, 2016 (received for review November 9, 2015) Most Escherichia coli promoters initiate transcription with a purine A recent single-molecule fluorescence resonance energy trans- 7 or 8 nt downstream from the –10 hexamer, but some promoters, fer study showed there can be heterogeneity in transcription including the ribosomal RNA promoter rrnB P1, start 9 nt from the bubble size in open complexes, even for an individual promoter –10 element. We identified promoter and RNA polymerase deter- (8). Thus, spontaneous bubble expansion and contraction can minants of this noncanonical rrnB P1 start site using biochemical account for multiple start sites at some promoters. The variation and genetic approaches including mutational analysis of the pro- in TSS from an individual promoter implies there is flexibility in + moter, Fe2 cleavage assays to monitor template strand positions the placement of template strand DNA by RNAP relative to the near the active-site, and Bpa cross-linking to map the path of open active site of the enzyme. complex DNA at amino acid and nucleotide resolution. We find After formation of the first phosphodiester bond during tran- that mutations in several promoter regions affect transcription scription initiation, the next 5–12 nucleotide addition steps pro- start site (TSS) selection. -
Control of Transcription Initiation*
View metadata, citation and similar papers at core.ac.uk brought to you by CORE provided by Publications of the IAS Fellows J. Biosci., Vol. 18, Number 1, March 1993, pp 13–25. © Printed in India. Control of transcription initiation* V NAGARAJA Centre for Generic Engineering, Indian Institute of Science, Bangalore 560 012, India MS received 11 June 1992; revised 23 October 1992 Abstract Mechanism of control of transcription initiation have expanded far beyond the classical operon concept. Control elements are multipartite and well separated from each other. The trans-factors bound to these sites make contacts with RNA polymerase: promoter complexes by DNA bending or looping to influence the initiation event. Activators and repressors are like two faces of the same coin and their function depends on the site of action, mode of interaction with DNA and also the nutritional status of the cell. Keywords. RNA polymerase; promoter; activator; repressor; transcription. 1. Introduction Regulation of gene expression is a fundamental event in every cell and organism. The regulatory events occur during each step of macromolecular synthesis. The control of transcription initiation is by far the major regulatory event determining whether a gene is turned 'on' or 'off'. The enzyme RNA polymerase occupies center stage during this process. A precise, productive initiation is possible only by the direct and specific interaction of the enzyme with functional promoter sequences (Mishra and Chatterji 1993). RNA polymerase is one of the conserved proteins in prokaryotes in terms of its structural organization and function. The size, composition and function of different subunits of core polymerase does not vary much in different organisms. -
The Use of Single-Molecule DNA Nanomanipulation to Study Transcription Kinetics by CHEN-YU LIU
The Use of Single-Molecule DNA Nanomanipulation to Study Transcription Kinetics By CHEN-YU LIU A dissertation submitted to the Graduate School-New Brunswick Rutgers, The State University of New Jersey In partial fulfillment of the requirements For the degree of Doctor of Philosophy Computational Biology and Biophysics in Written under the direction of Dr. Richard H. Ebright And Approved by Dr. Richard H. Ebright Dr. Ronald M. Levy Dr. Wilma K. Olson Dr. Richard D. Ludescher New Brunswick, New Jersey October, 2007 ABSTRACT OF THE DISSERTATION The Use of Single-Molecule DNA Nanomanipulation to Study Transcription Kinetics by CHEN-YU LIU Dissertation Director: Dr. Richard H. Ebright Transcription involves many reaction steps and intermediates. Many phenomena in transcription kinetics are covered by ensemble average. Single-molecule DNA nanomanipulation techniques uncover these transcription kinetic events via determination of a transcription bubble in real time. In this dissertation, we focus on the transcription kinetics of bacterial RNAP. The study of transcription kinetics in this thesis can be divided into 4 main subjects: 1) The study of abortive initiation ii mechanism: Through a single-molecule DNA nanomanipulation technique, we tested the three models proposed for the mechanism of abortive initiation - inchworming, scrunching and transient excursion - on T5 N25 promoter. Of the three models, only the scrunching model involves a change in the size of the transcription bubble during abortive initiation, which was observed by single-molecule DNA nanomanipulation technique. 2) The study of the kinetics of elongation and termination: By introducing varying transcribed region lengths into DNA templates, the kinetics of elongation and terminator rewinding were studied. -
A Novel Initiation Pathway in Escherichia Coli Transcription
bioRxiv preprint doi: https://doi.org/10.1101/042432; this version posted March 27, 2016. The copyright holder for this preprint (which was not certified by peer review) is the author/funder. All rights reserved. No reuse allowed without permission. Classification: Biological Sciences - Biochemistry A Novel Initiation Pathway in Escherichia Coli Transcription Eitan Lerner*†, SangYoon Chung*†, Benjamin L. Allen‡, Shuang Wang§, Jookyung J. Lee¶, Winson S. Lu*, Wilson L. Grimaud*, Antonino Ingargiola*, Xavier Michalet*, Yazan Alhadid*, Sergei Borukhov¶, Terence Strick§, Dylan J. Taatjes‡, Shimon Weiss*,#,||,** Affiliations: *Dept. of Chemistry & Biochemistry, University of California Los Angeles, Los Angeles, CA 90095. ‡Dept. of Chemistry & Biochemistry, University of Colorado, Boulder. §Institut Jacques Monod, Centre National de la Recherche Scientifique and University of Paris Diderot and Sorbonne Paris Cité, Paris, France. ¶Rowan University School of Osteopathic Medicine, Stratford, NJ 08084, USA. #California NanoSystems Institute, University of California Los Angeles, Los Angeles, CA 90095. ||Dept. of Physiology, University of California Los Angeles, Los Angeles, CA 90095. † Equal contributions ** Corresponding author: [email protected] bioRxiv preprint doi: https://doi.org/10.1101/042432; this version posted March 27, 2016. The copyright holder for this preprint (which was not certified by peer review) is the author/funder. All rights reserved. No reuse allowed without permission. Abstract: Initiation is a highly regulated, rate-limiting step in transcription. We employed a series of approaches to examine the kinetics of RNA polymerase (RNAP) transcription initiation in greater detail. Quenched kinetics assays, in combination with magnetic tweezer experiments and other methods, showed that, contrary to expectations, RNAP exit kinetics from later stages of initiation (e.g. -
Enhancers Predominantly Regulate Gene Expression in Vivo Via Transcription Initiation
bioRxiv preprint doi: https://doi.org/10.1101/844191; this version posted November 16, 2019. The copyright holder for this preprint (which was not certified by peer review) is the author/funder. All rights reserved. No reuse allowed without permission. Enhancers predominantly regulate gene expression in vivo via transcription initiation. Martin S. C. Larke, Takayuki Nojima, Jelena Telenius, Jacqueline A. Sharpe, Jacqueline A. Sloane- Stanley, Sue Butler, RoBert A. Beagrie, Damien J. Downes, Ron Schwessinger, A. Marieke Oudelaar, Julia Truch, Bryony Crompton, M. A. Bender, Nicholas J. Proudfoot, Douglas R. Higgs, Jim R. Hughes. ABSTRACT Gene transcription occurs via a cycle of linked events including initiation, promoter proximal pausing and elongation of RNA polymerase II (Pol II). A key question is how do transcriptional enhancers influence these events to control gene expression? Here we have used a new approach to quantify transcriptional initiation and pausing in vivo, while simultaneously identifying transcription start sites (TSSs) and pause-sites (TPSs) from single RNA molecules. When analyzed in parallel with nascent RNA-seq, these data show that differential gene expression is achieved predominantly via changes in transcription initiation rather than Pol II pausing. Using genetically engineered mouse models deleted for specific enhancers we show that these elements control gene expression via Pol II recruitment and/or initiation rather than via promoter proximal pause release. Together, our data show that enhancers, in general, control gene expression predominantly by Pol II recruitment and initiation rather than via pausing. INTRODUCTION Changes in RNA expression during cell fate decisions are made via the activation of promoters By enhancers that respond to changes in transcriptional and epigenetic programmes to regulate gene expression in time and space. -
Epigenetic Control of Ribosome Biogenesis Homeostasis Jerôme Deraze
Epigenetic control of ribosome biogenesis homeostasis Jerôme Deraze To cite this version: Jerôme Deraze. Epigenetic control of ribosome biogenesis homeostasis. Cellular Biology. Université Pierre et Marie Curie - Paris VI, 2017. English. NNT : 2017PA066342. tel-01878354 HAL Id: tel-01878354 https://tel.archives-ouvertes.fr/tel-01878354 Submitted on 21 Sep 2018 HAL is a multi-disciplinary open access L’archive ouverte pluridisciplinaire HAL, est archive for the deposit and dissemination of sci- destinée au dépôt et à la diffusion de documents entific research documents, whether they are pub- scientifiques de niveau recherche, publiés ou non, lished or not. The documents may come from émanant des établissements d’enseignement et de teaching and research institutions in France or recherche français ou étrangers, des laboratoires abroad, or from public or private research centers. publics ou privés. Université Pierre et Marie Curie Ecole doctorale : Complexité du Vivant IBPS – UMR7622 Laboratoire de Biologie du Développement UPMC CNRS Epigenetic control of developmental homeostasis and plasticity Epigenetic Control of Ribosome Biogenesis Homeostasis Par Jérôme Deraze Thèse de doctorat de Biologie Moléculaire et Cellulaire Dirigée par Frédérique Peronnet et Sébastien Bloyer Présentée et soutenue publiquement le 19 Septembre 2017 Devant un jury composé de : Pr Anne-Marie MARTINEZ Professeur Rapporteur Dr Jacques MONTAGNE Directeur de Recherche Rapporteur Dr Olivier JEAN-JEAN Directeur de Recherche Examinateur Dr Michel COHEN-TANNOUDJI Directeur de Recherche Examinateur Dr Françoise JAMEN Maître de Conférences Examinateur Dr Nicolas NEGRE Maître de Conférences Examinateur Dr Frédérique PERONNET Directrice de Recherche Directrice de thèse Pr Sébastien BLOYER Professeur Co-directeur de thèse Table of contents Table of contents .................................................................................................................................... -
The Biology of RNA-Protein Complexes
The Biology of RNA-Protein Complexes PROGRAM AND ABSTRACTS October11thͲ14th2017,ThonͲDittmerPalais,Regensburg,Germany GENERAL INFORMATION Throughout this program, the numbers next to the titles refer to corresponding oral or poster abstract numbers in the abstract section of this book. Please refrain from citing the abstracts in bibliographies. The information contained in this abstract book should be treated as personal communicationandshouldbecitedonlywiththeexpressedconsentoftheauthor. Nounauthorizedphotographyinsessions: To encourage the sharing of novel and unpublished data, taking photographs and/or videos during the scientific sessions (both oral andposters),orofpostersoutsidesessionhours,isstrictlyprohibited. Violatorsofthispolicymayhavetheirequipmentconfiscatedand/or theymaybeaskedtoleavetheconferenceandhavetheirregistration privilegesrevokedwithoutreimbursement Useofsocialmedia: Thehashtagis#SFB960.Weencourageallattendeestotweetabout the amazing science they experience at the SFB960 meeting in Regensburg.But,pleasedorespectthefollowingruleswhentweeting orusingothersocialmedia: 1. bepoliteandrespectfulofothersinallofyourmessages 2. donottransmitphotographsofslidesorpostersunderany circumstances 3. donottransmitphotographsofconferenceattendeeswithout theirexpressedconsent 4. tweetersshouldrespectrequestsofpresenterswhoasktorefrain fromtweetingthecontentoftheirtalksorposters CoverPicture:TobiasBeck Ͳ Layout:JanMedenbach Ͳ Print: Offset Haas 2 MEETING SPONSORS 3 SCIENTIFIC ORGANIZERS Prof. Dr. -
Molecular Basis of Eukaryotic Transcription
4 October 2006 Advanced information on the Nobel Prize in Chemistry 2006 Molecular basis of eukaryotic transcription ____________________________________________________________________________________________________ Information Department, Box 50005, SE-104 05 Stockholm, Sweden Phone: +46 8 673 95 00, Fax: +46 8 15 56 70, E-mail: [email protected], Website: www.kva.se Molecular basis of eukaryotic transcription The Nobel Prize in Chemistry for 2006 is awarded to Roger Kornberg for his fundamental studies of the molecular basis of eukaryotic transcription. Transcription is the process in a cell in which the genetic information stored in DNA is activated by the synthesis of complementary mRNA by enzymes called RNA polymerases. Eventually, the mRNA is translated by ribosomes into functional cell proteins. Transcription is one of the most central processes of life, and is controlled by a sophisticated and complex regulatory system. The current needs for proteins of different kinds in the cell, determine when the regulatory system triggers the activation of specific genes. Kornberg has made breakthrough progress in the molecular understanding of transcription and its regulation in eukaryotic cells. His combination of advanced biochemical techniques with structural determinations has enabled the atomic level reconstruction of RNA polymerase from yeast in isolation as well as in a number of functionally relevant complexes with template DNA, product mRNA, substrate nucleotides and regulatory proteins. Introduction The early history of eukaryotic transcription starts almost five decades ago with the discovery by Weiss and Gladstone of an RNA polymerase activity in rat liver nuclei (Weiss and Gladstone, 1959). This publication opened transcription as a field for scientific research of crucial importance for processes such as cell differentiation and the development of multicellular organisms as well as for cellular responses to external signals and stimuli. -
Mechanism of Transcription Initiation and Promoter Escape by E. Coli RNA Polymerase
Mechanism of transcription initiation and promoter escape by E. coli RNA polymerase Kate L. Hendersona,1, Lindsey C. Feltha,1, Cristen M. Molzahna,1, Irina Shkela,b, Si Wangb,2, Munish Chhabrac, Emily F. Ruffb,3, Lauren Bietera, Joseph E. Krafta,b, and M. Thomas Record Jr.a,b,c,4 aDepartment of Biochemistry, University of Wisconsin-Madison, Madison, WI 53706; bDepartment of Chemistry, University of Wisconsin-Madison, Madison, WI 53706; and cProgram in Biophysics, University of Wisconsin-Madison, Madison, WI 53706 Edited by Peter H. von Hippel, University of Oregon, Eugene, OR, and approved March 7, 2017 (received for review November 10, 2016) To investigate roles of the discriminator and open complex (OC) two residues to make an OC with the observed TSS (2); this OC is lifetime in transcription initiation by Escherichia coli RNA polymer- very unstable and short-lived. The instability of the rrnB P1 OC is α ββ’ωσ70 ase (RNAP; 2 ), we compare productive and abortive initi- the basis of the regulation of initiation at this promoter by con- λ ation rates, short RNA distributions, and OC lifetime for the PR centrations of initiating nucleotides, the feedback ligand guanosine and T7A1 promoters and variants with exchanged discriminators, tetraphosphate (ppGpp), and protein factors such as DksA (3). A all with the same transcribed region. The discriminator determines single base change (C–7G) improves the interaction of the rrnB the OC lifetime of these promoters. Permanganate reactivity of P1 discriminator with σ1.2, shifts the TSS, greatly increases OC thymines reveals that strand backbones in open regions of long- lifetime and stability, and eliminates regulation by these ligands (3, λ lived PR-discriminator OCs are much more tightly held than for 4).