Genome-Wide Association Study for Conformation Traits in Three Danish
Total Page:16
File Type:pdf, Size:1020Kb
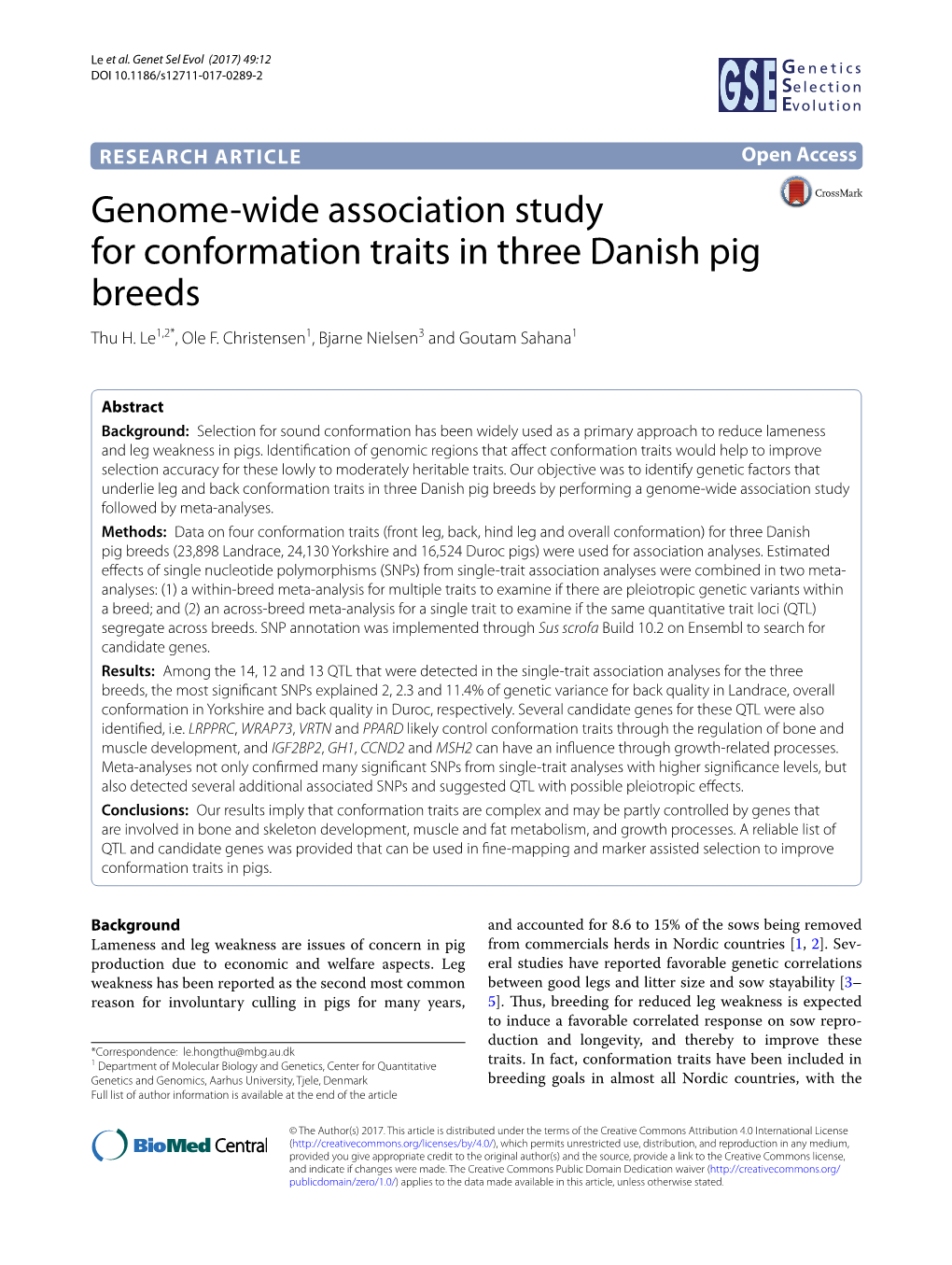
Load more
Recommended publications
-
Two Spatially Distinct Kinesin-14 Pkl1 and Klp2 Generate Collaborative
bioRxiv preprint doi: https://doi.org/10.1101/205815; this version posted October 19, 2017. The copyright holder for this preprint (which was not certified by peer review) is the author/funder. All rights reserved. No reuse allowed without permission. Two spatially distinct Kinesin-14 Pkl1 and Klp2 generate collaborative inward forces against Kinesin-5 Cut7 in S. pombe Masashi Yukawa*, Yusuke Yamada, Tomoaki Yamauchi and Takashi Toda* Laboratory of Molecular and Chemical Cell Biology, Department of Molecular Biotechnology, Graduate School of Advanced Sciences of Matter, Hiroshima University, 1-3-1 Kagamiyama, Higashi-Hiroshima, Hiroshima 739-8530, Japan. * To whom correspondence should be addressed: Tel/Fax: +81 82 424 7754; E-mail: [email protected], Tel/Fax: +81 82 424 7868; E-mail: [email protected] Running title: Inward forces exerted by Kinesin-14s Keywords: fission yeast/force generation/kinesin/mitotic bipolar spindle/spindle pole body Abbreviations used: GFP-binding protein, GBP; Msd1-Wdr8-Pkl1 complex, MWP complex; spindle pole body, SPB; temperature sensitive, ts; the γ-tubulin complex, γ-TuC 1 bioRxiv preprint doi: https://doi.org/10.1101/205815; this version posted October 19, 2017. The copyright holder for this preprint (which was not certified by peer review) is the author/funder. All rights reserved. No reuse allowed without permission. SUMMARY STATEMENT Proper force-balance generated by Kinesin-5 and Kinesin-14 is crucial for spindle bipolarity. Two fission yeast Kinesin-14s localize to different structures, thereby collaboratively producing inward forces against Kinesin-5-mediated outward force. ABSTRACT Kinesin motors play central roles in bipolar spindle assembly. -
4-6 Weeks Old Female C57BL/6 Mice Obtained from Jackson Labs Were Used for Cell Isolation
Methods Mice: 4-6 weeks old female C57BL/6 mice obtained from Jackson labs were used for cell isolation. Female Foxp3-IRES-GFP reporter mice (1), backcrossed to B6/C57 background for 10 generations, were used for the isolation of naïve CD4 and naïve CD8 cells for the RNAseq experiments. The mice were housed in pathogen-free animal facility in the La Jolla Institute for Allergy and Immunology and were used according to protocols approved by the Institutional Animal Care and use Committee. Preparation of cells: Subsets of thymocytes were isolated by cell sorting as previously described (2), after cell surface staining using CD4 (GK1.5), CD8 (53-6.7), CD3ε (145- 2C11), CD24 (M1/69) (all from Biolegend). DP cells: CD4+CD8 int/hi; CD4 SP cells: CD4CD3 hi, CD24 int/lo; CD8 SP cells: CD8 int/hi CD4 CD3 hi, CD24 int/lo (Fig S2). Peripheral subsets were isolated after pooling spleen and lymph nodes. T cells were enriched by negative isolation using Dynabeads (Dynabeads untouched mouse T cells, 11413D, Invitrogen). After surface staining for CD4 (GK1.5), CD8 (53-6.7), CD62L (MEL-14), CD25 (PC61) and CD44 (IM7), naïve CD4+CD62L hiCD25-CD44lo and naïve CD8+CD62L hiCD25-CD44lo were obtained by sorting (BD FACS Aria). Additionally, for the RNAseq experiments, CD4 and CD8 naïve cells were isolated by sorting T cells from the Foxp3- IRES-GFP mice: CD4+CD62LhiCD25–CD44lo GFP(FOXP3)– and CD8+CD62LhiCD25– CD44lo GFP(FOXP3)– (antibodies were from Biolegend). In some cases, naïve CD4 cells were cultured in vitro under Th1 or Th2 polarizing conditions (3, 4). -
Two Spatially Distinct Kinesin-14 Proteins, Pkl1 and Klp2, Generate Collaborative Inward Forces Against Kinesin-5 Cut7 in S
© 2018. Published by The Company of Biologists Ltd | Journal of Cell Science (2018) 131, jcs210740. doi:10.1242/jcs.210740 RESEARCH ARTICLE Two spatially distinct kinesin-14 proteins, Pkl1 and Klp2, generate collaborative inward forces against kinesin-5 Cut7 in S. pombe Masashi Yukawa*, Yusuke Yamada, Tomoaki Yamauchi and Takashi Toda* ABSTRACT bioinformatics analysis indicates the existence of additional groups Kinesin motors play central roles in bipolar spindle assembly. In many (Wickstead et al., 2010). In general, N-kinesins and C-kinesins eukaryotes, spindle pole separation is driven by kinesin-5, which possess microtubule plus end- and minus end-directed motilities generates outward force. This outward force is balanced by respectively, whereas M-kinesins are microtubule depolymerases. antagonistic inward force elicited by kinesin-14 and/or dynein. In As proposed originally (Vale et al., 1985), kinesin molecules act as fission yeast, two kinesin-14 proteins, Pkl1 and Klp2, play an cellular power plants by generating directional forces across opposing role against the kinesin-5 motor protein Cut7. However, microtubules through repeated ATPase cycles. how the two kinesin-14 proteins coordinate individual activities During mitosis, a number of distinct kinesin molecules engage remains elusive. Here, we show that although deletion of either pkl1 with bipolar spindle assembly, thereby ensuring coordinated or klp2 rescues temperature-sensitive cut7 mutants, deletion of only chromosome segregation; these kinesins are collectively called pkl1 can bypass the lethality caused by cut7 deletion. Pkl1 is tethered mitotic kinesins (Sharp et al., 2000b; Tanenbaum and Medema, to the spindle pole body, whereas Klp2 is localized along the spindle 2010; Yount et al., 2015). -
Símbolos, Siglas E Abreviaturas
UNIVERSIDADE FEDERAL DO RIO GRANDE DO SUL DEPARTAMENTO DE GENÉTICA PROGRAMA DE PÓS-GRADUAÇÃO EM GENÉTICA E BIOLOGIA MOLECULAR AVALIAÇÃO CITOGENÔMICA EM INDIVÍDUOS COM CARDIOPATIAS CONGÊNITAS CONOTRUNCAIS Káren Regina Silva de Souza Dissertação submetida ao Programa de Pós- Graduação em Genética e Biologia Molecular da UFRGS como requisito parcial para a obtenção do grau de Mestre em Genética e Biologia Molecular Orientadora: Profa Dra. Mariluce Riegel Porto Alegre Março de 2015 Este trabalho foi desenvolvido no Laboratório de Citogenética Molecular do Hospital de Clínicas de Porto Alegre e teve como fontes financiadoras o Conselho Nacional de Desenvolvimento Científico e Tecnológico (CNPq/476783/2013-5) e Fundação de Amparo à Pesquisa do Rio Grande do Sul (FAPERGS/Edital PQG 001/2013). AGRADECIMENTOS ______________________________________________________________________________ Agradeço, primeiramente, a minha orientadora Profa Dra. Mariluce Riegel, sem sua paciência, profissionalismo e companheirismo este trabalho não teria sido possível. Agradeço por sua orientação e compreensão durante todas as etapas deste estudo. Agradeço a meus pais, José Nilton e Hilda Regina, por seu apoio em todas as etapas da minha vida e por sempre acreditarem em mim e me proporcionarem a possibilidade de escolher o caminho que eu gostaria de trilhar. Agradeço a meu marido André, por sua paciência e amor infinito. Agradeço a minhas irmãs Carla e Helena por serem companheiras de todas as horas. Agradeço a equipe dos Laboratórios de Citogenética do Serviço de Genética Médica e de Citogenética Molecular por fazerem parte da minha rotina tornando todos os dias de trabalho mais agradáveis. A Dra. Janaina Huber, cardiologista do Instituto de Cardiologia do Rio Grande do Sul/Fundação Universitária, pelo trabalho em colaboração. -
A Prevalence of Imprinted Genes Within the Total Transcriptomes of Human Tissues and Cells
Hindawi Publishing Corporation Molecular Biology International Volume 2012, Article ID 793506, 29 pages doi:10.1155/2012/793506 Research Article A Prevalence of Imprinted Genes within the Total Transcriptomes of Human Tissues and Cells Sergey V. Anisimov1, 2 1 Research Unit of Cellular and Genetic Engineering, V. A. Almazov Federal Center for Heart, Blood & Endocrinology, Akkuratova Street 2, Saint-Petersburg 197341, Russia 2 Department of Intracellular Signaling and Transport, Institute of Cytology, Russian Academy of Sciences, Tikhoretsky Prosp. 4, Saint-Petersburg 194064, Russia Correspondence should be addressed to Sergey V. Anisimov, [email protected] Received 14 March 2012; Revised 23 June 2012; Accepted 28 June 2012 Academic Editor: Alessandro Desideri Copyright © 2012 Sergey V. Anisimov. This is an open access article distributed under the Creative Commons Attribution License, which permits unrestricted use, distribution, and reproduction in any medium, provided the original work is properly cited. Genomic imprinting is an epigenetic phenomenon that causes a differential expression of paternally and maternally inherited alleles of a subset of genes (the so-called imprinted genes). Imprinted genes are distributed throughout the genome and it is predicted that about 1% of the human genes may be imprinted. It is recognized that the allelic expression of imprinted genes varies between tissues and developmental stages. The current study represents the first attempt to estimate a prevalence of imprinted genes within the total human transcriptome. In silico analysis of the normalized expression profiles of a comprehensive panel of 173 established and candidate human imprinted genes was performed, in 492 publicly available SAGE libraries. The latter represent human cell and tissue samples in a variety of physiological and pathological conditions. -
A Dynamic Model of Gene Expression in Monocytes Reveals Differences In
Journal of Inflammation BioMed Central Research Open Access A dynamic model of gene expression in monocytes reveals differences in immediate/early response genes between adult and neonatal cells Shelley Lawrence†1, Yuhong Tang†2, M Barton Frank2, Igor Dozmorov2, Kaiyu Jiang1, Yanmin Chen1, Craig Cadwell2, Sean Turner2, Michael Centola2 and James N Jarvis*1 Address: 1Dept. of Pediatrics, Neonatal Section, University of Oklahoma College of Medicine, Oklahoma City, OK, USA and 2Arthritis & Immunology Program Oklahoma Medical Research Foundation, Oklahoma City, 73104, USA Email: Shelley Lawrence - [email protected]; Yuhong Tang - [email protected]; M Barton Frank - Bart- [email protected]; Igor Dozmorov - [email protected]; Kaiyu Jiang - [email protected]; Yanmin Chen - yanmin- [email protected]; Craig Cadwell - [email protected]; Sean Turner - [email protected]; Michael Centola - michael- [email protected]; James N Jarvis* - [email protected] * Corresponding author †Equal contributors Published: 16 February 2007 Received: 26 September 2006 Accepted: 16 February 2007 Journal of Inflammation 2007, 4:4 doi:10.1186/1476-9255-4-4 This article is available from: http://www.journal-inflammation.com/content/4/1/4 © 2007 Lawrence et al; licensee BioMed Central Ltd. This is an Open Access article distributed under the terms of the Creative Commons Attribution License (http://creativecommons.org/licenses/by/2.0), which permits unrestricted use, distribution, and reproduction in any medium, provided the original work is properly cited. Abstract Neonatal monocytes display immaturity of numerous functions compared with adult cells. Gene expression arrays provide a promising tool for elucidating mechanisms underlying neonatal immune function. -
Epigenetics of Ancient DNA
JULY-SEPTEMBER 2016 VOL. 8 № 3 (30) ISSN 2075-8251 Epigenetics of Ancient DNA ancient bone ancient DNA modern DNA STEM CELLS IN THE TREATMENT HYALURONIC ACID IN VASCULAR AND OF INSULIN-DEPENDENT IMMUNE HOMEOSTASIS DURING NORMAL DIABETES MELLITUS PREGNANCY AND PREECLAMPSIA Р. 31 Р. 59 Letter from the Editors ear readers of ActaNaturae, In the near future we intend to launch the We are delighted to bring you the 30th “electronic editorial board” system, which Dissue of the journal. will hopefully make communication between We continue to develop steadily. In 2015, authors and the editorial staff, peer review- our impact factor rose to 1.77, making Acta ers, and the Editorial Board more mobile, will Naturae third among Russian research jour- allow one to check manuscript status, to look nals in all disciplines. The credit goes to the through the comments of peer reviewers, and highly professional work of the editorial staff, to edit one’s personal data provided upon in- the Editorial Board, as well as to the increased itial registration. quality of the papers published. Gone are the We are looking forward to receiving new days when the Editorial Board had to put in a interesting manuscripts to publish with great lot of effort in attracting well-known authors pleasure! to submit articles. Today, with the portfolio See you in our next issue! full, we have an opportunity to select the sub- mitted papers more carefully and strictly. The Editorial Board VOL. 8 № 3 (30) 2016 | ACTA NATURAE | 1 INNOVATION RUSSIA Discussion club We create a dialogue between all socially active groups of people: students, scientists, lecturers, businessmen, managers, innovators, investors, designers, art critics, architects, photographs. -
Blood Transcriptome Based Biomarkers for Human Circadian
RESEARCH ARTICLE Blood transcriptome based biomarkers for human circadian phase Emma E Laing1*†, Carla S Mo¨ ller-Levet2†, Norman Poh3†, Nayantara Santhi4, Simon N Archer4, Derk-Jan Dijk4* 1Department of Microbial Sciences, School of Biosciences and Medicine, Faculty of Health and Medical Sciences, University of Surrey, Guildford, United Kingdom; 2Bioinformatics Core Facility, Faculty of Health and Medical Sciences, University of Surrey, Guildford, United Kingdom; 3Department of Computer Science, Faculty of Engineering and Physical Sciences, University of Surrey, Guildford, United Kingdom; 4Surrey Sleep Research Centre, School of Biosciences and Medicine, Faculty of Health and Medical Sciences, University of Surrey, Guildford, United Kingdom Abstract Diagnosis and treatment of circadian rhythm sleep-wake disorders both require assessment of circadian phase of the brain’s circadian pacemaker. The gold-standard univariate method is based on collection of a 24-hr time series of plasma melatonin, a suprachiasmatic nucleus-driven pineal hormone. We developed and validated a multivariate whole-blood mRNA- based predictor of melatonin phase which requires few samples. Transcriptome data were collected under normal, sleep-deprivation and abnormal sleep-timing conditions to assess robustness of the predictor. Partial least square regression (PLSR), applied to the transcriptome, *For correspondence: e.laing@ identified a set of 100 biomarkers primarily related to glucocorticoid signaling and immune surrey.ac.uk (EEL); d.j.dijk@surrey. function. Validation showed that PLSR-based predictors outperform published blood-derived 2 ac.uk (D-JD) circadian phase predictors. When given one sample as input, the R of predicted vs observed phase was 0.74, whereas for two samples taken 12 hr apart, R2 was 0.90. -
A Prevalence of Imprinted Genes Within the Total Transcriptomes of Human Tissues and Cells
Hindawi Publishing Corporation Molecular Biology International Volume 2012, Article ID 793506, 29 pages doi:10.1155/2012/793506 Research Article A Prevalence of Imprinted Genes within the Total Transcriptomes of Human Tissues and Cells Sergey V. Anisimov1, 2 1 Research Unit of Cellular and Genetic Engineering, V. A. Almazov Federal Center for Heart, Blood & Endocrinology, Akkuratova Street 2, Saint-Petersburg 197341, Russia 2 Department of Intracellular Signaling and Transport, Institute of Cytology, Russian Academy of Sciences, Tikhoretsky Prosp. 4, Saint-Petersburg 194064, Russia Correspondence should be addressed to Sergey V. Anisimov, [email protected] Received 14 March 2012; Revised 23 June 2012; Accepted 28 June 2012 Academic Editor: Alessandro Desideri Copyright © 2012 Sergey V. Anisimov. This is an open access article distributed under the Creative Commons Attribution License, which permits unrestricted use, distribution, and reproduction in any medium, provided the original work is properly cited. Genomic imprinting is an epigenetic phenomenon that causes a differential expression of paternally and maternally inherited alleles of a subset of genes (the so-called imprinted genes). Imprinted genes are distributed throughout the genome and it is predicted that about 1% of the human genes may be imprinted. It is recognized that the allelic expression of imprinted genes varies between tissues and developmental stages. The current study represents the first attempt to estimate a prevalence of imprinted genes within the total human transcriptome. In silico analysis of the normalized expression profiles of a comprehensive panel of 173 established and candidate human imprinted genes was performed, in 492 publicly available SAGE libraries. The latter represent human cell and tissue samples in a variety of physiological and pathological conditions. -
1 Supplementary Materials and Methods
SUPPLEMENTARY MATERIALS AND METHODS Genetic ablation of miR-22 fosters diet-induced obesity and NAFLD development. Monika Gjorgjieva1*, Cyril Sobolewski1*, Anne-Sophie Ay1*, Daniel Abegg2, Marta Correia de Sousa1, Dorothea Portius1, Flavien Berthou1, Margot Fournier1, Christine Maeder1, Pia Rantakari3, Fu-Ping Zhang3, Matti Poutanen3, Didier Picard4, Xavier Montet5, Serge Nef6, Alexander Adibekian2, Michelangelo Foti1,7 1Department of Cell Physiology and Metabolism, Faculty of Medicine, University of Geneva, Switzerland. 2Department of Chemistry, The Scripps Research Institute, 130 Scripps Way, Jupiter, Florida 33458, United States. 3Institute of Biomedicine, Research Centre for Integrative Physiology and Pharmacology, and Turku Center for Disease Modeling, University of Turku, Finland. 4Department of Cell Biology, Faculty of Science, University of Geneva, Switzerland. 5Department of Radiology, Faculty of Medicine, University of Geneva, Switzerland. 6Department of Genetic Medicine and Development, Faculty of Medicine, University of Geneva, Switzerland. 7Diabetes Center, Faculty of Medicine, University of Geneva, Switzerland. * MG, CS and ASA equally contributed to this work. 1 miR-22KO mice generation Targeting construct BAC clone (CH29-40010) containing the mouse Mir22 gene was obtained from BACPAC Resources Center (Children's Hospital Oakland Research Institute, Oakland (CHORI), California, USA). A 9066 bp fragment of the Mir22 gene containing exon 1 was subcloned into the minimal vector, pACYC177 (New England Biolabs, MA, USA) by Red/ET recombination method according to the manufacturer’s instructions (Gene Bridges GmbH, Germany). PCR product used in subcloning was amplified from the pACYC177-plasmid using the primers 5’- GTCAATGAAGTTCTTACGGTGACACTCAGCTTCCCAGAATGCAGCGCGCT GGCAGACCTCAGCGCTAG-3’ and 5’-AGTACCCAGTGGGAGAATGGATC TAGGTCTGGGAGAAATTGACCTTAGCTGAAGACGAAAGGGCCTC-3’ where homology arms to the Mir22 gene are indicated in bold, and sequences homologues for ampicillin resistance (amp) gene and ori of the pACYC177-plasmid are in italics. -
Epigenetic Variability in Cells of Normal Cytology Is Associated with the Risk of Future Morphological Transformation
Teschendorff et al. Genome Medicine 2012, 4:24 http://genomemedicine.com/content/4/3/24 RESEARCH Open Access Epigenetic variability in cells of normal cytology is associated with the risk of future morphological transformation Andrew E Teschendorff1*, Allison Jones2, Heidi Fiegl3, Alexandra Sargent4, Joanna J Zhuang1,2, Henry C Kitchener4 and Martin Widschwendter2* Abstract Background: Recently, it has been proposed that epigenetic variation may contribute to the risk of complex genetic diseases like cancer. We aimed to demonstrate that epigenetic changes in normal cells, collected years in advance of the first signs of morphological transformation, can predict the risk of such transformation. Methods: We analyzed DNA methylation (DNAm) profiles of over 27,000 CpGs in cytologically normal cells of the uterine cervix from 152 women in a prospective nested case-control study. We used statistics based on differential variability to identify CpGs associated with the risk of transformation and a novel statistical algorithm called EVORA (Epigenetic Variable Outliers for Risk prediction Analysis) to make predictions. Results: We observed many CpGs that were differentially variable between women who developed a non-invasive cervical neoplasia within 3 years of sample collection and those that remained disease-free. These CpGs exhibited heterogeneous outlier methylation profiles and overlapped strongly with CpGs undergoing age-associated DNA methylation changes in normal tissue. Using EVORA, we demonstrate that the risk of cervical neoplasia can be predicted in blind test sets (AUC = 0.66 (0.58 to 0.75)), and that assessment of DNAm variability allows more reliable identification of risk-associated CpGs than statistics based on differences in mean methylation levels. -
The Centriolar Satellite Protein Cfap53/Ccdc11 Facilitates the Formation of the First Zygotic Microtubule Organizing Center in the Zebrafish Embryo
bioRxiv preprint doi: https://doi.org/10.1101/2020.11.18.388652; this version posted November 18, 2020. The copyright holder for this preprint (which was not certified by peer review) is the author/funder, who has granted bioRxiv a license to display the preprint in perpetuity. It is made available under aCC-BY-ND 4.0 International license. The centriolar satellite protein Cfap53/Ccdc11 facilitates the formation of the first zygotic microtubule organizing center in the zebrafish embryo. Sven Willekers1, Federico Tessadori1, Babet van der Vaart2, Heiko Henning3, Riccardo Stucchi2,4, Maarten Altelaar4, Bernard A.J. Roelen5, Anna Akhmanova2, Jeroen Bakkers1 1. Hubrecht Institute-KNAW, Utrecht, The Netherlands, 2. Cell Biology, Neurobiology and Biophysics, Department of Biology, Faculty of Science, Utrecht University, Utrecht, the Netherlands 3. EQuine Sciences, Department Clinical Sciences, Faculty of Veterinary Medicine, Utrecht University, Utrecht, The Netherlands 4. Biomolecular Mass Spectrometry and Proteomics, Bijvoet Center for Biomolecular Research and Utrecht Institute for Pharmaceutical Sciences, Utrecht University, Utrecht, Netherlands. 5. Embryology, Anatomy and Physiology, Department Clinical Sciences, Faculty of Veterinary Medicine, Utrecht University, Utrecht, The Netherlands Corresponding author: [email protected] 1 bioRxiv preprint doi: https://doi.org/10.1101/2020.11.18.388652; this version posted November 18, 2020. The copyright holder for this preprint (which was not certified by peer review) is the author/funder, who has granted bioRxiv a license to display the preprint in perpetuity. It is made available under aCC-BY-ND 4.0 International license. Abstract In embryos from most animal species a zygotic centrosome is assembled by the centriole derived from the sperm cell and pericentriolar proteins present in the oocyte.