Prediction of Hydro-Meteorological, Meteorological and Climatological Hazards
Total Page:16
File Type:pdf, Size:1020Kb
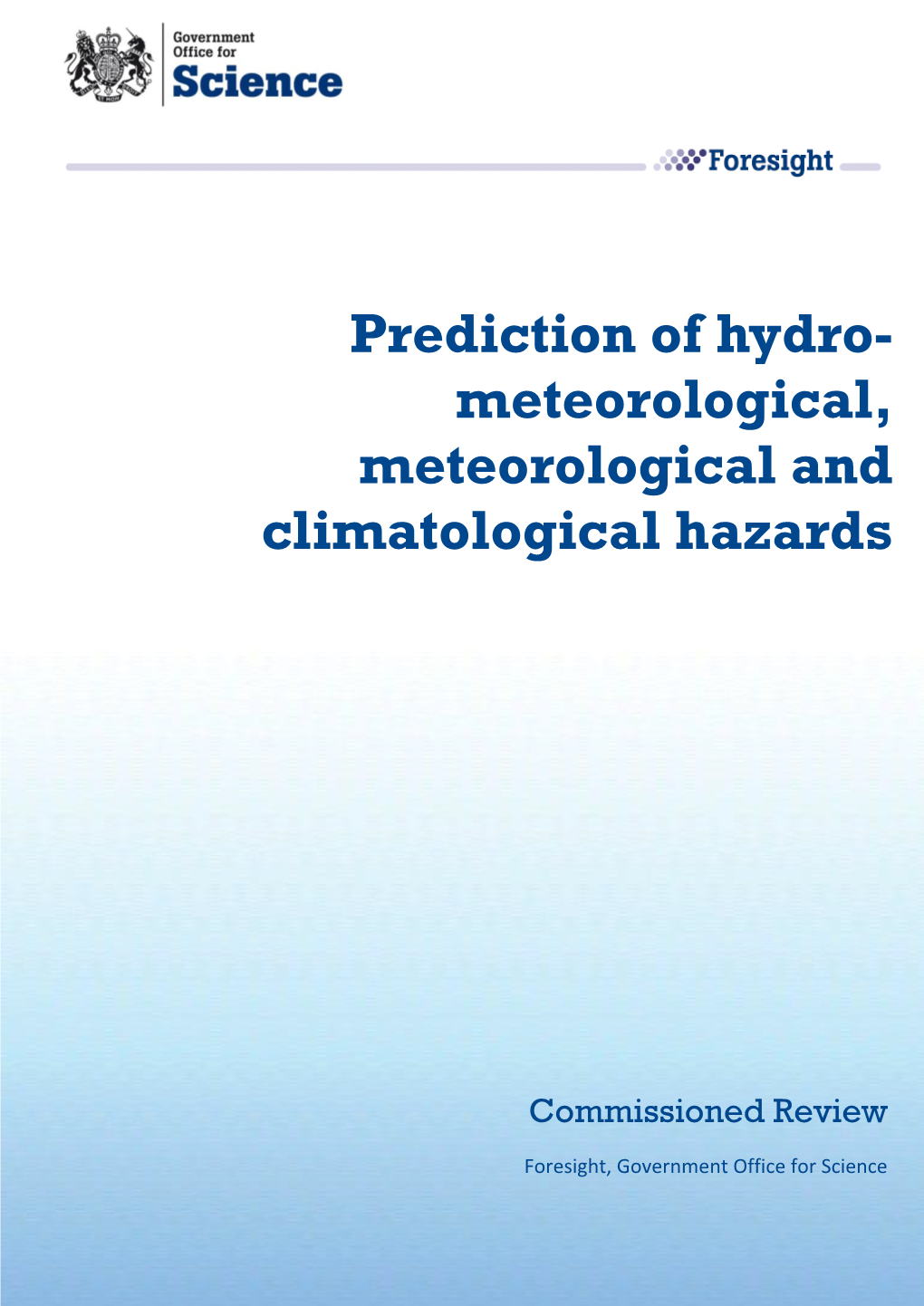
Load more
Recommended publications
-
Extended-Range Probabilistic Forecasts of Ganges and Brahmaputra Floods in Bangladesh
EXTENDED-RANGE PROBABILISTIC FORECASTS OF GANGES AND BRAHMAPUTRA FLOODS IN BANGLADESH BY PETER J. WEBSTER , JUN JIAN , THOMAS M. HO P SON , CARLOS D. HOYOS , PAULA A. AGU D ELO , HAI -RU CHANG , JU D ITH A. CURRY , ROBERT L. GROSSMAN , TIMOTHY N. PALMER , AN D A. R. SUBBIAH A new ensemble flood prediction scheme, with skill to 10 to 15 days, allowed people along the Brahmaputra to evacuate well in advance of floods in 2007/08. any of the largest rivers on the planet emanate from the Tibetan Plateau and the Himalayas (Fig. 1a), fed by glacial and snow M melting and monsoon rainfall. Nearly 25% of the global popu- lation reside in the vast agrarian societies in the Yellow, Yangtze, Mekong, Irrawaddy, Ganges, Brahmaputra, and Indus river basins, each of which is subject to periods of widespread and long-lived flooding. Flooding remains the greatest cause of death and destruc- tion in the developing world, leading to catastrophic loss of life and property. While almost every government in Asia has made substantial progress over the past two decades in saving the lives of victims of slow-onset flood disasters, such events remain relentlessly impoverishing. In India alone, an average 6 million hectares (ha) of land (approximately equivalent to the size of Texas) is inundated each year, affecting 35–40 million people (Dhar and Nandargi 2000; CWC 2008). Because the flooding occurs in the fertile flood plains of major rivers, the loss in agricultural inputs (seed, fertilizer, and pesticides) alone costs in excess of 1 billion U.S. dollars (USD; hence- forth all costs will be given in USD) in an average flood or drought event. -
Brahmaputra and the Socio-Economic Life of People of Assam
Brahmaputra and the Socio-Economic Life of People of Assam Authors Dr. Purusottam Nayak Professor of Economics North-Eastern Hill University Shillong, Meghalaya, PIN – 793 022 Email: [email protected] Phone: +91-9436111308 & Dr. Bhagirathi Panda Professor of Economics North-Eastern Hill University Shillong, Meghalaya, PIN – 793 022 Email: [email protected] Phone: +91-9436117613 CONTENTS 1. Introduction and the Need for the Study 1.1 Objectives of the Study 1.2 Methodology and Data Sources 2. Assam and Its Economy 2.1 Socio-Demographic Features 2.2 Economic Features 3. The River Brahmaputra 4. Literature Review 5. Findings Based on Secondary Data 5.1 Positive Impact on Livelihood 5.2 Positive Impact on Infrastructure 5.2.1 Water Transport 5.2.2 Power 5.3 Tourism 5.4 Fishery 5.5 Negative Impact on Livelihood and Infrastructure 5.6 The Economy of Char Areas 5.6.1 Demographic Profile of Char Areas 5.6.2 Vicious Circle of Poverty in Char Areas 6. Micro Situation through Case Studies of Regions and Individuals 6.1 Majuli 6.1.1 A Case Study of Majuli River Island 6.1.2 Individual Case Studies in Majuli 6.1.3 Lessons from the Cases from Majuli 6.1.4 Economics of Ferry Business in Majuli Ghats 6.2 Dhubri 6.2.1 A Case Study of Dhubri 6.2.2 Individual Case Studies in Dhubri 6.2.3 Lessons from the Cases in Dhubri 6.3 Guwahati 6.3.1 A Case of Rani Chapari Island 6.3.2 Individual Case Study in Bhattapara 7. -
Climate Change in the Brahmaputra Valley and Impact on Rice and Tea Productivity
CLIMATE CHANGE IN THE BRAHMAPUTRA VALLEY AND IMPACT ON RICE AND TEA PRODUCTIVITY A thesis submitted in partial fulfillment of the requirements for the award of the degree of DOCTOR OF PHILOSOPHY By Rajib Lochan Deka CENTRE FOR THE ENVIRONMENT INDIAN INSTITUTE OF TECHNOLOGY GUWAHATI GUWAHATI–781039, ASSAM, INDIA MARCH, 2013 INDIAN INSTITUTE OF TECHONOLOGY GUWAHATI Centre for the Environment Guwahati –781039 Assam India CERTIFICATE This is to certify that the thesis entitled “ Climate Change in the Brahmaputra Valley and Impact on Rice and Tea Productivity ” submitted by Mr. Rajib Lochan Deka to the Indian Institute of Technology Guwahati, for the award of the degree of Doctor of Philosophy is a record of bonafide research work carried out by him under our supervision and guidance. Mr. Deka has carried out research on the topic at the Centre for the Environment of IIT Guwahati over a period of three years and eight months and the thesis, in our opinion, is worthy of consideration for the degree of Doctor of Philosophy in accordance with the regulations of this Institute. The results contained in this thesis have not been submitted elsewhere in part or full for the award of any degree or diploma to the best of our knowledge and belief. Mrinal Kanti Dutta Chandan Mahanta Associate Professor Professor Department of Humanities and Social Sciences Department of Civil Engineering Indian Institute of Technology Guwahati Indian Institute of Technology Guwahati Guwahati-781 039, Assam, India Guwahati-781 039, Assam, India TH-1188_0865206 INDIAN INSTITUTE OF TECHONOLOGY GUWAHATI Centre for the Environment Guwahati –781039 Assam India STATEMENT I do hereby declare that the matter embodied in the thesis is a result of research work carried out by me in the Centre for the Environment, Indian Institute of Technology Guwahati, Guwahati, Assam, India. -
Download File
ARTICLE https://doi.org/10.1038/s41467-020-19795-6 OPEN Seven centuries of reconstructed Brahmaputra River discharge demonstrate underestimated high discharge and flood hazard frequency ✉ Mukund P. Rao 1,2 , Edward R. Cook1, Benjamin I. Cook3,4, Rosanne D. D’Arrigo1, Jonathan G. Palmer 5, Upmanu Lall6, Connie A. Woodhouse 7, Brendan M. Buckley1, Maria Uriarte 8, Daniel A. Bishop 1,2, Jun Jian 9 & Peter J. Webster10 1234567890():,; The lower Brahmaputra River in Bangladesh and Northeast India often floods during the monsoon season, with catastrophic consequences for people throughout the region. While most climate models predict an intensified monsoon and increase in flood risk with warming, robust baseline estimates of natural climate variability in the basin are limited by the short observational record. Here we use a new seven-century (1309–2004 C.E) tree-ring recon- struction of monsoon season Brahmaputra discharge to demonstrate that the early instru- mental period (1956–1986 C.E.) ranks amongst the driest of the past seven centuries (13th percentile). Further, flood hazard inferred from the recurrence frequency of high discharge years is severely underestimated by 24–38% in the instrumental record compared to pre- vious centuries and climate model projections. A focus on only recent observations will therefore be insufficient to accurately characterise flood hazard risk in the region, both in the context of natural variability and climate change. 1 Tree Ring Laboratory, Lamont-Doherty Earth Observatory of Columbia University, Palisades, NY 10964, USA. 2 Department of Earth and Environmental Science, Columbia University, New York, NY 10027, USA. 3 NASA Goddard Institute for Space Studies, New York, NY 10025, USA. -
Multiscale Analysis of Three Consecutive Years of Anomalous Flooding in Pakistan
Multiscale analysis of three consecutive years of anomalous flooding in Pakistan By K. L. Rasmussen1+, A. J. Hill*, V. E. Toma#, M. D. Zuluaga+, P. J. Webster#, and R. A. Houze, Jr.+ +Department of Atmospheric Sciences University of Washington Seattle, WA *Atmospheric Science Group Department of Geosciences Texas Tech University Lubbock, TX #School of Earth and Atmospheric Sciences Georgia Institute of Technology Atlanta, GA Submitted to the Quarterly Journal of the Royal Meteorological Society January 2014 Revised April 2014 Revised June 2014 1 Corresponding author: Kristen Lani Rasmussen, Department of Atmospheric Sciences, University of Washington, Box 351640, Seattle, WA 98195 E-mail address: [email protected] ABSTRACT A multiscale investigation into three years of anomalous floods in Pakistan provides insight into their formation, unifying meteorological characteristics, mesoscale storm structures, and predictability. Striking similarities between all three floods existed from planetary and large- scale synoptic conditions down to the mesoscale storm structures, and these patterns were generally well-captured with the ECMWF EPS forecast system. Atmospheric blocking events associated with high geopotential heights and surface temperatures over Eastern Europe were present during all three floods. Quasi-stationary synoptic conditions over the Tibetan plateau allowed for the formation of anomalous easterly midlevel flow across central India into Pakistan that advected deep tropospheric moisture from the Bay of Bengal into Pakistan, enabling flooding in the region. The TRMM Precipitation Radar observations show that the flood- producing storms exhibited climatologically unusual structures during all three floods in Pakistan. These departures from the climatology consisted of westward propagating precipitating systems with embedded wide convective cores, rarely seen in this region, that likely occurred when convection was organized upscale by the easterly midlevel jet across the subcontinent. -
Spatial and Seasonal Responses of Precipitation in the Ganges And
South Dakota State University Open PRAIRIE: Open Public Research Access Institutional Repository and Information Exchange Natural Resource Management Faculty Publications Department of Natural Resource Management 1-28-2015 Spatial and Seasonal Responses of Precipitation in the Ganges and Brahmaputra River Basins to ENSO and Indian Ocean Dipole Modes: Implications for Flooding and Drought M. S. Pervez G. M. Henebry South Dakota State University Follow this and additional works at: http://openprairie.sdstate.edu/nrm_pubs Part of the Geographic Information Sciences Commons, Physical and Environmental Geography Commons, and the Spatial Science Commons Recommended Citation Pervez, M. S. and Henebry, G. M., "Spatial and Seasonal Responses of Precipitation in the Ganges and Brahmaputra River Basins to ENSO and Indian Ocean Dipole Modes: Implications for Flooding and Drought" (2015). Natural Resource Management Faculty Publications. 5. http://openprairie.sdstate.edu/nrm_pubs/5 This Article is brought to you for free and open access by the Department of Natural Resource Management at Open PRAIRIE: Open Public Research Access Institutional Repository and Information Exchange. It has been accepted for inclusion in Natural Resource Management Faculty Publications by an authorized administrator of Open PRAIRIE: Open Public Research Access Institutional Repository and Information Exchange. For more information, please contact [email protected]. Nat. Hazards Earth Syst. Sci., 15, 147–162, 2015 www.nat-hazards-earth-syst-sci.net/15/147/2015/ doi:10.5194/nhess-15-147-2015 © Author(s) 2015. CC Attribution 3.0 License. Spatial and seasonal responses of precipitation in the Ganges and Brahmaputra river basins to ENSO and Indian Ocean dipole modes: implications for flooding and drought M. -
Water Resources in the Northeast
BACKGROUND PAPER NO. 2 AUGUST 2006 WATER RESOURCES IN THE NORTHEAST: STATE OF THE KNOWLEDGE BASE BY CHANDAN MAHANTA INDIAN INSTITUTE OF TECHNOLOGY, GUWAHATI, INDIA This paper was commissioned as an input to the study “Development and Growth in Northeast India: The Natural Resources, Water, and Environment Nexus” Table of contents 1. Background..........................................................................................................................................1 2. Context .................................................................................................................................................1 3. Present status of knowledge base.....................................................................................................1 4. Characteristics of the water resources of the Northeast................................................................3 4.1 General features............................................................................................................................3 4.2 Brahmaputra basin.......................................................................................................................4 4.3 Barak basin ....................................................................................................................................7 5. Water resource availability in major water bodies ........................................................................7 6. Groundwater resources .....................................................................................................................7 -
Attributing the 2017 Bangladesh Floods From
Hydrol. Earth Syst. Sci., 23, 1409–1429, 2019 https://doi.org/10.5194/hess-23-1409-2019 © Author(s) 2019. This work is distributed under the Creative Commons Attribution 4.0 License. Attributing the 2017 Bangladesh floods from meteorological and hydrological perspectives Sjoukje Philip1, Sarah Sparrow2, Sarah F. Kew1, Karin van der Wiel1, Niko Wanders3,4, Roop Singh5, Ahmadul Hassan5, Khaled Mohammed2, Hammad Javid2,6, Karsten Haustein6, Friederike E. L. Otto6, Feyera Hirpa7, Ruksana H. Rimi6, A. K. M. Saiful Islam8, David C. H. Wallom2, and Geert Jan van Oldenborgh1 1Royal Netherlands Meteorological Institute (KNMI), De Bilt, the Netherlands 2Oxford e-Research Centre, Department of Engineering Science, University of Oxford, Oxford, UK 3Department of Physical Geography, Utrecht University, Utrecht, the Netherlands 4Department of Civil and Environmental Engineering, Princeton University, Princeton, NJ, USA 5Red Cross Red Crescent Climate Centre, The Hague, the Netherlands 6Environmental Change Institute, Oxford University Centre for the Environment, Oxford, UK 7School of Geography and the Environment, University of Oxford, Oxford, UK 8Institute of Water and Flood Management, Bangladesh University of Engineering and Technology, Dhaka, Bangladesh Correspondence: Sjoukje Philip ([email protected]) and Geert Jan van Oldenborgh ([email protected]) Received: 10 July 2018 – Discussion started: 23 July 2018 Revised: 14 February 2019 – Accepted: 14 February 2019 – Published: 13 March 2019 Abstract. In August 2017 Bangladesh faced one of its worst change in discharge towards higher values is somewhat less river flooding events in recent history. This paper presents, uncertain than in precipitation, but the 95 % confidence inter- for the first time, an attribution of this precipitation-induced vals still encompass no change in risk. -
District Disaster Preparedness and Response Plan
District Disaster Preparedness and Response Plan (2019) Name of the District: Majuli (ASSAM) Telephone: +91-03775-274424 Fax: +91-03775-274475, E-Mail: [email protected] Prepared by :- District Administration. 1 Table of Contents Foreword .......................................................................................................... 2 Table of Contents ............................................................................................. 3 1 Introduction .............................................................................................. 5 1.1 Background………………………………………………………………….. 5 1.2 Importance of multi hazard management plan…………………… 7 1.3 The main features of multi hazard plan……………………………….. 7 1.4 Disaster Management Cycle………………………………………. 7 1.5 Pre Disaster or Risk Management Phase……………………….. 8 1.6 Post- Disaster or Crisis Management Phase………………………… 8 1.7 Objective of the plan………………………………………….. 8 2.1 Majuli- Administrative Profile .................................................................... 8 2.2 Disasters.................................................................................................... 9 2.3 Flood ................................................................................................... 9 2.4 Erosion ................................................................................................ 11 2.5 Earth-Quake ...................................................................................... 14 2.6 Cyclone ............................................................................................ -
Comparative Physiography of the Lower Ganges and Lower Mississippi Valleys
Louisiana State University LSU Digital Commons LSU Historical Dissertations and Theses Graduate School 1955 Comparative Physiography of the Lower Ganges and Lower Mississippi Valleys. S. Ali ibne hamid Rizvi Louisiana State University and Agricultural & Mechanical College Follow this and additional works at: https://digitalcommons.lsu.edu/gradschool_disstheses Recommended Citation Rizvi, S. Ali ibne hamid, "Comparative Physiography of the Lower Ganges and Lower Mississippi Valleys." (1955). LSU Historical Dissertations and Theses. 109. https://digitalcommons.lsu.edu/gradschool_disstheses/109 This Dissertation is brought to you for free and open access by the Graduate School at LSU Digital Commons. It has been accepted for inclusion in LSU Historical Dissertations and Theses by an authorized administrator of LSU Digital Commons. For more information, please contact [email protected]. COMPARATIVE PHYSIOGRAPHY OF THE LOWER GANGES AND LOWER MISSISSIPPI VALLEYS A Dissertation Submitted to the Graduate Faculty of the Louisiana State University and Agricultural and Mechanical College in partial fulfillment of the requirements for the degree of Doctor of Philosophy in The Department of Geography ^ by 9. Ali IJt**Hr Rizvi B*. A., Muslim University, l9Mf M. A*, Muslim University, 191*6 M. A., Muslim University, 191*6 May, 1955 EXAMINATION AND THESIS REPORT Candidate: ^ A li X. H. R iz v i Major Field: G eography Title of Thesis: Comparison Between Lower Mississippi and Lower Ganges* Brahmaputra Valleys Approved: Major Prj for And Chairman Dean of Gri ualc School EXAMINING COMMITTEE: 2m ----------- - m t o R ^ / q Date of Examination: ACKNOWLEDGMENT The author wishes to tender his sincere gratitude to Dr. Richard J. Russell for his direction and supervision of the work at every stage; to Dr. -
Situation Analysis on Climate Change
Situation Analysis on Climate Change INTERNATIONAL UNION FOR CONSERVATION OF NATURE Asia Regional Office 63 Sukhumvit Soi 39 Bangkok 10110, Thailand Tel: +66 2 662 4029 Fax: +66 2 662 4389 www.iucn.org/asia Bangladesh Country Office House 16, Road 2/3, Banani Dhaka 1213, Bangladesh Tel: +8802 9890423 Fax: +8802 9892854 www.iucn.org/bangladesh India Country Office 2nd Floor, 20 Anand Lok, August Kranti Marg, New Delhi 110049, India Tel/Fax: +91 11 4605 2583 www.iucn.org/india DIALOGUE FOR SUSTAINABLE MANAGEMENT OF TRANS-BOUNDARY WATER REGIMES IN SOUTH ASIA Ecosystems for Life • Inland Navigation 1 Situation Analysis on Climate Change K Shreelakshmi Chandan Mahanta Nandan Mukherjee and Malik Fida Abdullah Khan Nityananda Chakravorty Ecosystems for Life: A Bangladesh-India Initiative Ecosystems for Life • Inland Navigation 1 The designation of geographical entities in this book, and the presentation of the material, do not imply the expression of any opinion whatsoever on the part of IUCN concerning the legal status of any country, territory, administration, or concerning the delimitation of its frontiers or boundaries. The views expressed in this publication are authors’ personal views and do not necessarily reflect those of IUCN. This initiative is supported by the Minister for European Affairs and International Cooperation, the Netherlands. Published by: IUCN Asia Regional Office; IUCN Bangladesh Country Office; IUCN India Country Office; IUCN Gland, Switzerland Copyright: © 2012 IUCN, International Union for Conservation of Nature and Natural Resources Reproduction of this publication for educational or other non-commercial purposes is authorized without prior written permission from the copyright holder provided the source is fully acknowledged. -
A Review of Atmospheric and Land Surface Processes with Emphasis on flood Generation in the Southern Himalayan Rivers
Science of the Total Environment 556 (2016) 98–115 Contents lists available at ScienceDirect Science of the Total Environment journal homepage: www.elsevier.com/locate/scitotenv Review A review of atmospheric and land surface processes with emphasis on flood generation in the Southern Himalayan rivers A.P. Dimri a,⁎, R.J. Thayyen b, K. Kibler c,A.Stantond, S.K. Jain b,D.Tullosd, V.P. Singh e a School of Environmental Sciences, Jawaharlal Nehru University, New Delhi, India b National Institute of Hydrology, Roorkee, Uttarakhand, India c University of Central Florida, Orlando, FL, USA d Water Resources Engineering, Oregon State University, Corvallis, OR, USA e Department of Biological and Agricultural Engineering, and Zachry Department of Civil Engineering, Texas A & M University, College Station, TX, USA HIGHLIGHTS • Floods in the southern rim of the Indian Himalayas are a major cause of loss of life, property, crops, infrastructure, etc. • In the recent decade extreme precipitation events have led to numerous flash floods in and around the Himalayan region. Sporadic case-based studies have tried to explain the mechanisms causing the floods. • However, in some of the cases, the causative mechanisms have been elusive. • The present study provides an overview of mechanisms that lead to floods in and around the southern rim of the Indian Himalayas. article info abstract Article history: Floods in the southern rim of the Indian Himalayas are a major cause of loss of life, property, crops, infrastructure, Received 9 January 2016 etc. They have long term socio-economic impacts on the habitat living along/across the Himalayas. In the recent Received in revised form 29 February 2016 decade extreme precipitation events have led to numerous flash floods in and around the Himalayan region.