Applied Bayesian Modeling and Causal Inference from Incomplete-Data Perspectives an Essential Journey with Donald Rubin’S Statistical Family
Total Page:16
File Type:pdf, Size:1020Kb
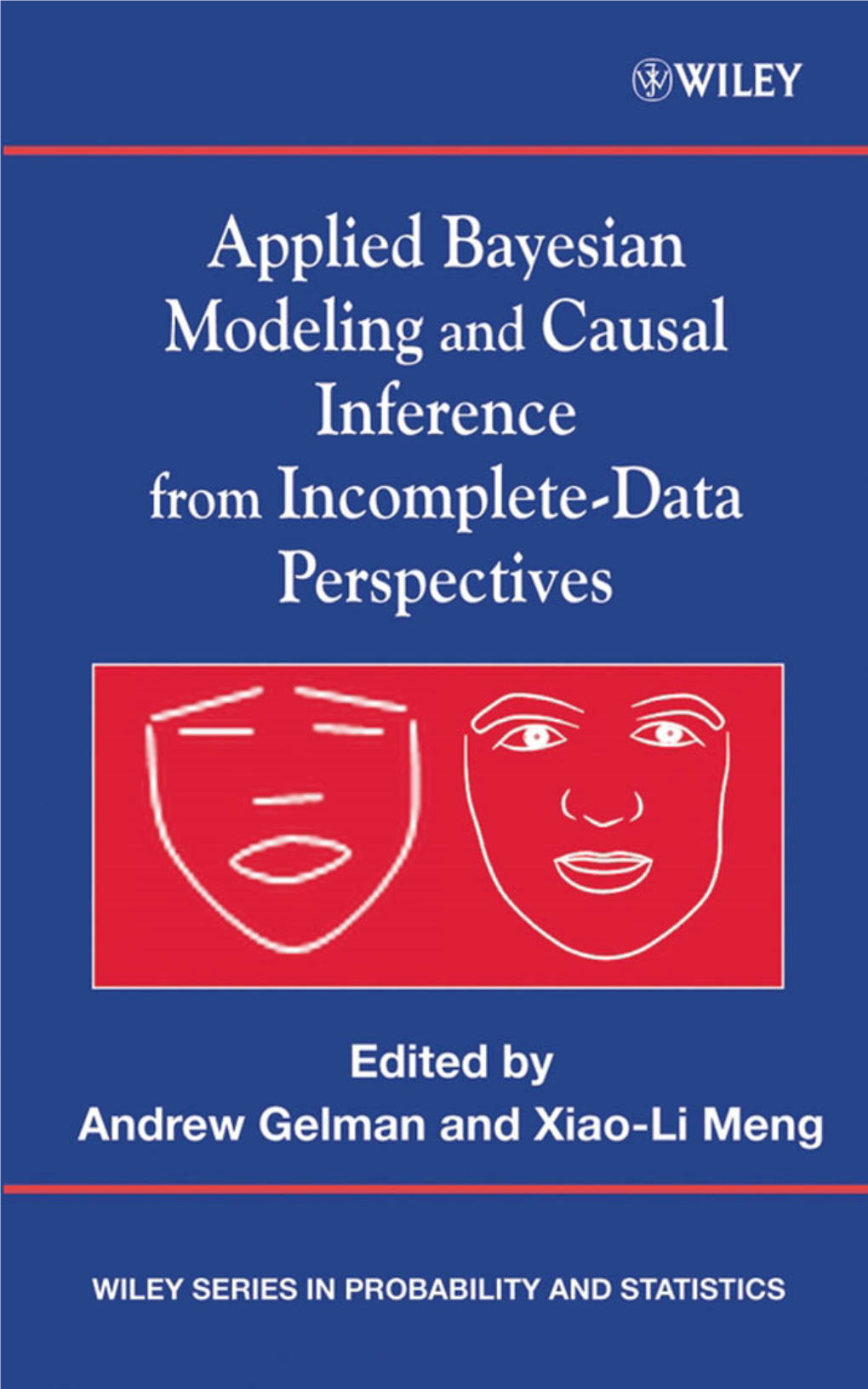
Load more
Recommended publications
-
1 the University of Chicago the Harris School of Public
THE UNIVERSITY OF CHICAGO THE HARRIS SCHOOL OF PUBLIC POLICY PPHA 421: APPLIED ECONOMETRICS II Spring 2016: Mondays and Wednesdays 10:30 – 11:50 pm, Room 140C Instructor: Professor Koichiro Ito 157 Harris School [email protected] Office hours: Mondays 3-4pm TA: Katherine Goulde: [email protected] Course Description The goal of this course is for students to learn a set of statistical tools and research designs that are useful in conducting high-quality empirical research on topics in applied microeconomics and related fields. Since most applied economic research examines questions with direct policy implications, this course will focus on methods for estimating causal effects. This course differs from many other econometrics courses in that it is oriented towards applied practitioners rather than future econometricians. It therefore emphasizes research design (relative to statistical technique) and applications (relative to theoretical proofs), though it covers some of each. Prerequisites PPHA42000 (Applied Econometrics I) is the prerequisite for this course. Students should be familiar with graduate school level probability and statistics, matrix algebra, and the classical linear regression model at the level of PPHA420. In the Economics department, the equivalent level of preparation would be the 1st year Ph.D. econometrics coursework. In general, I do not recommend taking this course if you have not taken PPHA420: Applied Econometrics I or a Ph.D. level econometrics coursework. This course is a core course for Ph.D. students and MACRM students at Harris School. Those who are not in the Harris Ph.D. program, the MACRM program, or the economics Ph.D. program need permission from the instructor to take the course. -
Donald Rubin Three-Day Course in Causality 5 to 7 February 2020 At
Donald Rubin Three-day Course in Causality 5 to 7 February 2020 at Waldhotel Doldenhorn, Kandersteg About the Course Most questions in social and biomedical sciences are causal in nature: what would happen to individuals, or to groups, if part of their environment were changed? In the course, the world-renowned expert Donald Rubin (Prof. em. Harvard) presents statistical methods for studying such questions. The course will follow his book Causal Inference for Statistics, Social, and Biomedical Sciences (Cambridge University Press, 2016) and starts with the notion of potential outcomes, each corresponding to the outcome that would be realized if a subject were exposed to a particular treatment or regime. In this approach, causal effects are comparisons of such potential outcomes. The fundamental problem of causal inference is that we can only observe one of the potential outcomes for a particular subject. Prof. Rubin will discuss how randomized experiments allow us to assess causal effects and then turn to observational studies. They lay out the assumptions needed for causal inference and describe the leading analysis methods, including matching, propensity-score methods, and instrumental variables. Many detailed applications will be shown, with special focus on practical aspects for the empirical researcher. About the Lecturer Donald B. Rubin is Professor Emeritus of Statistics, Harvard University, where he has been professor from 1984 to 2018 and department chair for fifteen years. Since 2018 he is professor at the Yau Mathematical Sciences Center, Tsinghua University, China, and Murray Shusterman Senior Research Fellow at the Department of Statistical Science, Fox School of Business, Temple University, Philadelphia. -
Theory with Applications to Voting and Deliberation Experiments!
Randomized Evaluation of Institutions: Theory with Applications to Voting and Deliberation Experiments Yves Atchadey and Leonard Wantchekonz June 24, 2009 Abstract We study causal inference in randomized experiments where the treatment is a decision making process or an institution such as voting, deliberation or decentralized governance. We provide a statistical framework for the estimation of the intrinsic e¤ect of the institution. The proposed framework builds on a standard set-up for estimating causal e¤ects in randomized experiments with noncompliance (Hirano-Imbens-Rubin-Zhou [2000]). We use the model to re- analyze the e¤ect of deliberation on voting for programmatic platforms in Benin (Wantchekon [2008]), and provide practical suggestions for the implementation and analysis of experiments involving institutions. 1 Introduction Randomized experiments are a widely accepted approach to infer causal relations in statis- tics and the social sciences. The idea dates back at least to Neyman (1923) and Fisher (1935) and has been extended by D. Rubin and coauthors (Rubin (1974), Rubin (1978), Rosenbaum and Rubin (1983)) to observational studies and to other more general experimental designs. In this approach, causality is de…ned in terms of potential outcomes. The causal e¤ect of a treatment, say Treatment 1 (compared to another treatment, Treatment 0) on the variable Y and on the statistical unit i is de…ned as Y i(1) Y i(0) (or its expected value) where Y i(j) is the value we would observe on unit i if it receives Treatment j. The estimation of this e¤ect is problematic because unit i cannot be given both Treatment 1 and Treatment 0. -
Arxiv:1805.08845V4 [Stat.ML] 10 Jul 2021 Outcomes Such As Images, Sequences, and Graphs
Counterfactual Mean Embeddings Krikamol Muandet∗ [email protected] Max Planck Institute for Intelligent Systems Tübingen, Germany Motonobu Kanagaway [email protected] Data Science Department, EURECOM Sophia Antipolis, France Sorawit Saengkyongam [email protected] University of Copenhagen Copenhagen, Denmark Sanparith Marukatat [email protected] National Electronics and Computer Technology Center National Science and Technology Development Agency Pathumthani, Thailand Abstract Counterfactual inference has become a ubiquitous tool in online advertisement, recommen- dation systems, medical diagnosis, and econometrics. Accurate modelling of outcome distri- butions associated with different interventions—known as counterfactual distributions—is crucial for the success of these applications. In this work, we propose to model counter- factual distributions using a novel Hilbert space representation called counterfactual mean embedding (CME). The CME embeds the associated counterfactual distribution into a reproducing kernel Hilbert space (RKHS) endowed with a positive definite kernel, which allows us to perform causal inference over the entire landscape of the counterfactual distri- bution. Based on this representation, we propose a distributional treatment effect (DTE) which can quantify the causal effect over entire outcome distributions. Our approach is nonparametric as the CME can be estimated under the unconfoundedness assumption from observational data without requiring any parametric assumption about the underlying dis- tributions. We also establish a rate of convergence of the proposed estimator which depends on the smoothness of the conditional mean and the Radon-Nikodym derivative of the un- derlying marginal distributions. Furthermore, our framework allows for more complex arXiv:1805.08845v4 [stat.ML] 10 Jul 2021 outcomes such as images, sequences, and graphs. -
The Neyman-Rubin Model of Causal Inference and Estimation Via Matching Methods∗
The Neyman-Rubin Model of Causal Inference and Estimation via Matching Methods∗ Forthcoming in The Oxford Handbook of Political Methodology, Janet Box-Steffensmeier, Henry Brady, and David Collier, eds. Jasjeet S. Sekhon † 11/16/2007 (15:57) ∗I thank Henry Brady, Wendy Tam Cho, David Collier and Roc´ıo Titiunik for valuable comments on earlier drafts, and David Freedman, Walter R. Mebane, Jr., Donald Rubin and Jonathan N. Wand for many valuable discussions on these topics. All errors are my responsibility. †Associate Professor, Travers Department of Political Science, Survey Research Center, UC Berkeley. [email protected], HTTP://sekhon.berkeley.edu/, 510.642.9974. “Correlation does not imply causation” is one of the most repeated mantras in the social sciences, but its full implications are sobering and often ignored. The Neyman-Rubin model of causal inference helps to clarify some of the issues which arise. In this chapter, the model is briefly described, and some consequences of the model are outlined for both quantitative and qualitative research. The model has radical implications for work in the social sciences given current practices. Matching methods, which are usually motivated by the Neyman-Rubin model, are reviewed and their properties discussed. For example, applied researchers are often surprised to learn that even if the selection on observables assumption is satisfied, the commonly used matching methods will generally make even linear bias worse unless specific and often implausible assumptions are satisfied. Some of the intuition of matching methods, such as propensity score matching, should be familiar to social scientists because they share many features with Mill’s methods, or canons, of inference. -
To Adjust Or Not to Adjust? Sensitivity Analysis of M-Bias and Butterfly-Bias
To Adjust or Not to Adjust? Sensitivity Analysis of M-Bias and Butterfly-Bias The Harvard community has made this article openly available. Please share how this access benefits you. Your story matters Citation Ding, Peng, and Luke W. Miratrix. 2015. “To Adjust or Not to Adjust? Sensitivity Analysis of M-Bias and Butterfly-Bias.” Journal of Causal Inference 3 (1) (January 1). doi:10.1515/jci-2013-0021. Published Version 10.1515/jci-2013-0021 Citable link http://nrs.harvard.edu/urn-3:HUL.InstRepos:25207409 Terms of Use This article was downloaded from Harvard University’s DASH repository, and is made available under the terms and conditions applicable to Open Access Policy Articles, as set forth at http:// nrs.harvard.edu/urn-3:HUL.InstRepos:dash.current.terms-of- use#OAP Page 1 of 24 Journal of Causal Inference 1 2 3 4 5 6 7 Abstract 8 9 “M-Bias”, as it is called in the epidemiological literature, is the bias intro- 10 11 duced by conditioning on a pretreatment covariate due to a particular “M-Structure” 12 between two latent factors, an observed treatment, an outcome, and a “collider”. 13 This potential source of bias, which can occur even when the treatment and the out- 14 come are not confounded, has been a source of considerable controversy. We here 15 16 present formulae for identifying under which circumstances biases are inflated or 17 reduced. In particular, we show that the magnitude of M-Bias in Gaussian linear 18 structural equationFor models Review tends to be relatively Only small compared to confounding 19 bias, suggesting that it is generally not a serious concern in many applied settings. -
1 the University of Chicago the Harris School of Public
THE UNIVERSITY OF CHICAGO THE HARRIS SCHOOL OF PUBLIC POLICY PPHA 42100: APPLIED ECONOMETRICS II Spring 2020: Tuesday and Thursday 9:30 – 10:50 am Instructor: Professor Koichiro Ito 157 Harris School [email protected] Office hours: Thursday 11-noon TA: TBA Office hours: TBA Course Description The goal of this course is for students to learn a set of statistical tools and research designs that are useful in conducting high-quality empirical research on topics in applied microeconomics and related fields. Since most applied economic research examines questions with direct policy implications, this course will focus on methods for estimating causal effects. This course differs from many other econometrics courses in that it is oriented towards applied practitioners rather than future econometricians. It therefore emphasizes research design (relative to statistical technique) and applications (relative to theoretical proofs), though it covers some of each. Prerequisites • PPHA420 (Applied Econometrics I) is the prerequisite for this course. Students should be familiar with PhD-level probability and statistics, matrix algebra, and the classical linear regression model at the level of PPHA420. In the Economics department, the equivalent level of preparation would be the 1st year Ph.D. econometrics coursework. • In general, I do not recommend taking this course if you have not taken PPHA420 or a Ph.D. level econometrics coursework. This course is a core course for Ph.D. students and MACRM students at Harris School. Therefore, although the course name 1 is Applied Econometrics, we'll cover a lot of theoretical econometrics with intensive math. Your problem sets and exams will be based on these materials. -
Causality Causality Refers to the Relationship
Causality Causality refers to the relationship between events where one set of events (the effects) is a direct consequence of another set of events (the causes). Causal inference is the process by which one can use data to make claims about causal relationships. Since inferring causal relationships is one of the central tasks of science, it is a topic that has been heavily debated in philosophy, statistics, and the scientific disciplines. In this article, we review the models of causation and tools for causal inference most prominent in the social sciences, including regularity approaches, associated with David Hume, and counterfactual models, associated with Jerzy Neyman, Donald Rubin, and David Lewis, among many others. One of the most notable developments in the study of causation is the increasing unification of disparate methods around a common conceptual and mathematical language that treats causality in counterfactual terms---i.e., the Neyman- Rubin model. We discuss how counterfactual models highlight the deep challenges involved in making the move from correlation to causation, particularly in the social sciences where controlled experiments are relatively rare. Regularity Models of Causation Until the advent of counterfactual models, causation was primarily defined in terms of observable phenomena. It was philosopher David Hume in the eighteenth century who began the modern tradition of regularity models of causation by defining causation in terms of repeated “conjunctions” of events. In An Enquiry into Human Understanding (1751), Hume argued that the labeling of two particular events as being causally related rested on an untestable metaphysical assumption. Consequently, Hume argued that causality could only be adequately defined in terms of empirical regularities involving classes of events. -
Donald Rubin∗ Andrew Gelman† 3 Oct 2017
Donald Rubin∗ Andrew Gelmany 3 Oct 2017 Donald Rubin (1943{) is a statistician who has made major contributions in statistical mod- eling, computation, and the foundations of causal inference. He is best known, perhaps, for the EM algorithm (a mathematical framework for iterative optimization, which has been useful for mixture models, hierarchical regression, and many other problems for which closed-form solutions are unavailable); multiple imputation as a method for accounting for uncertainty in statistical analysis with missing data; a Bayesian formulation of instrumental-variables analysis in economics; propensity scores for controlling for multiple predictors in observational studies; and, especially, the potential-outcomes framework of causal inference. Causal inference is central to social science. The effect of an intervention on an individual i (which could be a person, a firm, a school, a country, or whatever particular entity is being affected by the treatment) is defined as the difference in the outcome yi, comparing what would have happened under the intervention to what would have happened under the control. If these T C T C potential outcomes are labeled as yi and yi , then the causal effect for that individual is yi − yi . 0 1 But for any given individual i, we can never observe both potential outcomes yj and yj , thus the causal effect is impossible to directly measure. This is commonly referred to as the fundamental problem of causal inference, and it is at the core of modern economics and policy analysis. Resolutions to the fundamental problem of causal inference are called \identification strate- gies"; examples include linear regression, nonparametric regression, propensity score matching, instrumental variables, regression discontinuity, and difference in differences. -
ASA 1999 JSM Teaching Causal Inference Dr. Donald Rubin in Experiments and Observational Studies
ASA 1999 JSM Teaching Causal Inference Dr. Donald Rubin In Experiments and Observational Studies TEACHING CAUSAL INFERENCE IN EXPERIMENTS AND OBSERVATIONAL STUDIES Donald B. Rubin, Harvard University I Oxford St., 6th fl., Cambridge, MA 02138 Key Words: potential outcomes, Rubin Causal Model (RCM), Fisherian inference, Neymanian inference, Bayesian inference, experiments, observational studies, instrumental variables, noncompliance, statistical education Inference for causal effects is a critical activity in many branches of science and public policy. The field of statistics is the one field most suited to address such problems, whether from designed experiments or observational studies. Consequently, it is arguably essential that departments of statistics teach courses in causal inference to both graduate and undergraduate students. This presentation will discuss some aspects of such courses based on: a graduate level course taught at Harvard for a half dozen years, sometimes jointly with the Department of Economics (with Professor Guido Imbens, now at UCLA), and current plans for an undergraduate core course at Harvard University. An expanded version of this brief document will outline the courses' contents more completely. Moreover, a textbook by Imbens and Rubin, due to appear in 2000, will cover the basic material needed in both courses. The current course at Harvard begins with the definition of causal effects through potential outcomes. Causal estimands are comparisons of the outcomes that would have been observed under different exposures of units to treatments. This approach is commonly referred to as 'Rubin's Causal Model - RCM" (Holland, 1986), but the formal notation in the context of randomization-based inference in randomized experiments goes back to Neyman (1923), and the intuitive idea goes back centuries in various literatures; see also Fisher (1918), Tinbergen (1930) and Haavelmo (1944). -
^^.^-3 Causal Diagrams for Empirical Research C^-J-H Di^^I^)
^^. ^-3 Biometrika (1995), 82,4, pp. 669-710 Printed in Great Britain Causal diagrams for empirical research C^-j-h di^^i^) BY JUDEA PEARL Cognitive Systems Laboratory, Computer Science Department, University of California, Los Angeles, California 90024, U.S.A. SUMMARY The primary aim of this paper is to show how graphical models can be used as a mathematical language for integrating statistical and subject-matter information. In par- ticular, the paper develops a principled, nonparametric framework for causal inference, in which diagrams are queried to determine if the assumptions available are sufficient for identifying causal effects from nonexperimental data. If so the diagrams can be queried to produce mathematical expressions for causal effects in terms of observed distributions; otherwise, the diagrams can be queried to suggest additional observations or auxiliary experiments from which the desired inferences can be obtained. Some key words: Causal inference; Graph model; Structural equations; Treatment effect. 1. INTRODUCTION The tools introduced in this paper are aimed at helping researchers communicate quali- tative assumptions about cause-effect relationships, elucidate the ramifications of such assumptions, and derive causal inferences from a combination of assumptions, experi- ments, and data. The basic philosophy of the proposed method can best be illustrated through the classi- cal example due to Cochran (Wainer, 1989). Consider an experiment in which soil fumi- gants, X, are used to increase oat crop yields, Y, by controlling the eelworm population, Z, but may also have direct effects, both beneficial and adverse, on yields beside the control of eelworms. We wish to assess the total effect of the fumigants on yields when this study is complicated by several factors. -
Potential Outcome and Directed Acyclic Graph Approaches to Causality: Relevance for Empirical Practice in Economics∗
Potential Outcome and Directed Acyclic Graph Approaches to Causality: Relevance for Empirical Practice in Economics∗ Guido W. Imbensy August 2019 Abstract In this essay I discuss potential outcome and graphical approaches to causality, and their relevance for empirical work in economics. I review some of the work on directed acyclic graphs, including the recent \The Book of Why," ([Pearl and Mackenzie, 2018]). I also discuss the potential outcome framework developed by Rubin and coauthors, building on work by Neyman. I then discuss the relative merits of these approaches for empirical work in economics, focusing on the questions each answer well, and why much of the the work in economics is closer in spirit to the potential outcome framework. ∗I am grateful for help with the graphs by Michael Pollmann and for comments by Alberto Abadie, Jason Abaluck, Josh Angrist, Gary Chamberlain, Stephen Chaudoin, Rebecca Diamond, Dean Eckles, Ernst Fehr, Avi Feller, Paul Goldsmith-Pinkham, Chuck Manski, Paul Milgrom, Evan Munro, Franco Perrachi, Michael Pollmann, Jasjeet Sekhon, Samuel Skoda, Korinayo Thompson, and Heidi Williams. They are not responsible for any of the views expressed here. yProfessor of Economics, Graduate School of Business, and Department of Economics, Stanford University, SIEPR, and NBER, [email protected]. 1 Introduction Causal Inference (CI) in observational studies has been an integral part of econometrics since its start as a separate field in the 1920s and 1930s. The simultaneous equations methods developed by [Tinbergen, 1930], [Wright, 1928], and [Haavelmo, 1943] and their successors in the context of supply and demand settings were from the beginning, and continue to be, explicitly focused on causal questions.