In Sluggish Cognitive Tempo?
Total Page:16
File Type:pdf, Size:1020Kb
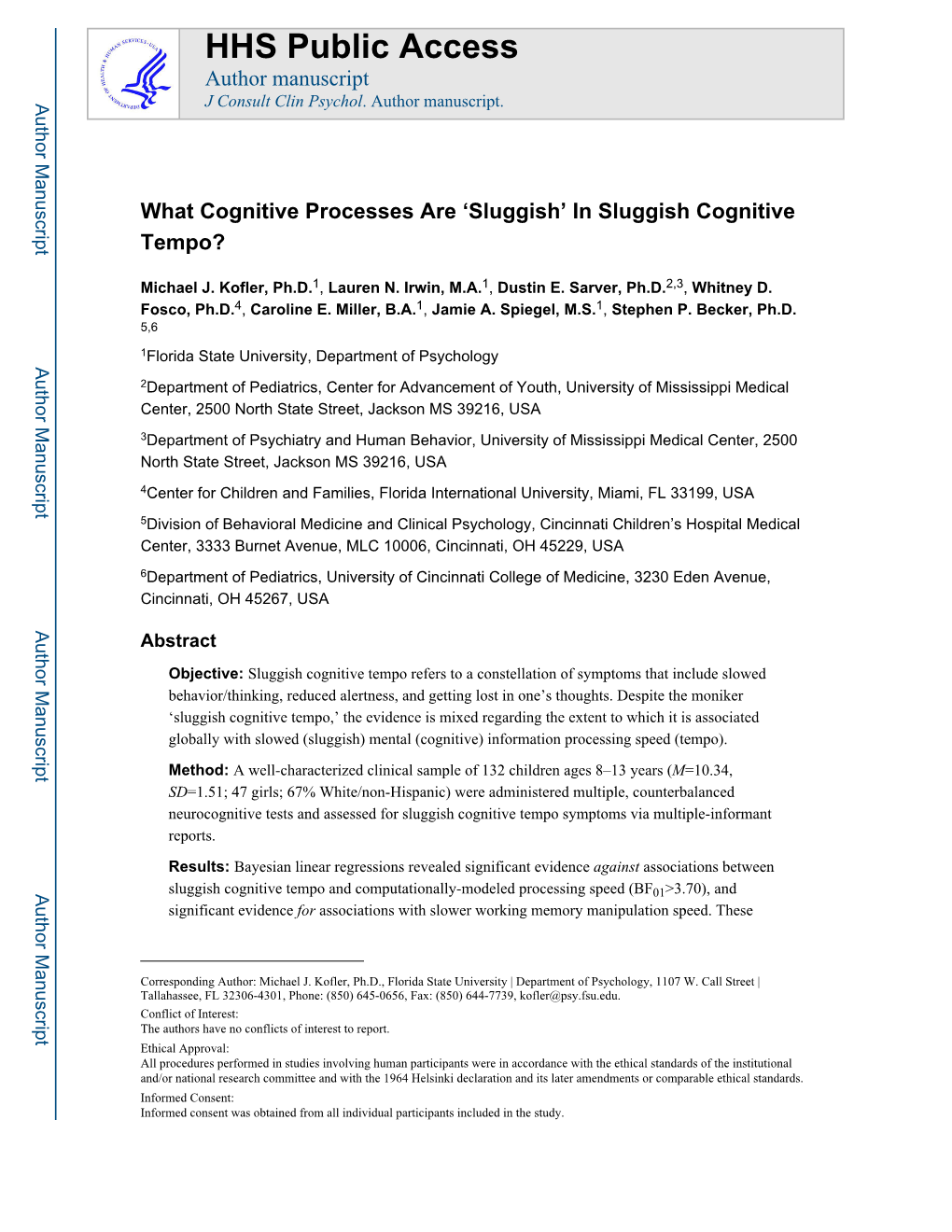
Load more
Recommended publications
-
Attention-Deficit/Hyperactivity Disorder Predominantly Inattentive
brain sciences Review Attention-Deficit/Hyperactivity Disorder Predominantly Inattentive Subtype/Presentation: Research Progress and Translational Studies Ike C. de la Peña 1,* , Michael C. Pan 2,3, Chau Giang Thai 1 and Tamara Alisso 1 1 Department of Pharmaceutical and Administrative Sciences, Loma Linda University School of Pharmacy, Loma Linda, CA 92350, USA; [email protected] (C.G.T.); [email protected] (T.A.) 2 Department of Psychology, Korea University, Seoul 02841, Korea; [email protected] 3 Division of Social Sciences, University of the Philippines Visayas Tacloban College, Tacloban 6500, Philippines * Correspondence: [email protected]; Tel.: +1-909-651-5995; Fax: +1-909-558-0446 Received: 23 April 2020; Accepted: 9 May 2020; Published: 14 May 2020 Abstract: Research on the predominantly inattentive attention-deficit/hyperactivity disorder (ADHD-PI) subtype/presentation is important given its high prevalence, but paradoxically it is under-recognized and undertreated. The temporal stability of the inattention symptom could impact the high worldwide prevalence of ADHD-PI. Some evidence suggests differences in the nature of attentional deficit in ADHD-PI vs. that in other subtypes. Impairments in neuropsychological, neurocognitive, and social functioning are also evident in ADHD-PI, which could be specific to the subtype (e.g., processing speed, social perception, and skills), or differ from others in severity. Neuroimaging studies have also revealed ADHD-PI-specific neuropathological abnormalities and those that are shared with other subtypes. ADHD-PI is highly comorbid with learning and internalizing (e.g., anxiety and depression) disorders. There is no solid evidence for ADHD-PI-specific genetic etiologies and differential responses of subtypes to ADHD medications. -
Honing in on the Social Difficulties Associated with Sluggish Cognitive Tempo in Children: Withdrawal, Peer Ignoring, and Low Engagement
Journal of Clinical Child & Adolescent Psychology ISSN: 1537-4416 (Print) 1537-4424 (Online) Journal homepage: http://www.tandfonline.com/loi/hcap20 Honing in on the Social Difficulties Associated With Sluggish Cognitive Tempo in Children: Withdrawal, Peer Ignoring, and Low Engagement Stephen P. Becker, Annie A. Garner, Leanne Tamm, Tanya N. Antonini & Jeffery N. Epstein To cite this article: Stephen P. Becker, Annie A. Garner, Leanne Tamm, Tanya N. Antonini & Jeffery N. Epstein (2017): Honing in on the Social Difficulties Associated With Sluggish Cognitive Tempo in Children: Withdrawal, Peer Ignoring, and Low Engagement, Journal of Clinical Child & Adolescent Psychology, DOI: 10.1080/15374416.2017.1286595 To link to this article: http://dx.doi.org/10.1080/15374416.2017.1286595 Published online: 13 Mar 2017. Submit your article to this journal Article views: 55 View related articles View Crossmark data Full Terms & Conditions of access and use can be found at http://www.tandfonline.com/action/journalInformation?journalCode=hcap20 Download by: [University of Iowa Libraries] Date: 31 March 2017, At: 09:20 Journal of Clinical Child & Adolescent Psychology, 00(00), 1–10, 2017 Copyright © 2017 Society of Clinical Child & Adoloscent Psychology ISSN: 1537-4416 print/1537-4424 online DOI: 10.1080/15374416.2017.1286595 Honing in on the Social Difficulties Associated With Sluggish Cognitive Tempo in Children: Withdrawal, Peer Ignoring, and Low Engagement Stephen P. Becker Division of Behavioral Medicine and Clinical Psychology, Cincinnati Children’s Hospital Medical Center and Department of Pediatrics, University of Cincinnati College of Medicine Annie A. Garner Department of Psychology, Saint Louis University Leanne Tamm Division of Behavioral Medicine and Clinical Psychology, Cincinnati Children’s Hospital Medical Center and Department of Pediatrics, University of Cincinnati College of Medicine Tanya N. -
7Th World Congress on ADHD: from Child to Adult Disorder
ADHD Atten Def Hyp Disord (2019) 11(Suppl 1):S1–S89 https://doi.org/10.1007/s12402-019-00295-7 ABSTRACTS Ó Springer-Verlag GmbH Austria, part of Springer Nature 2019 7th World Congress on ADHD: From Child to Adult Disorder 25th–28th April, Lisbon Portugal Editors: Manfred Gerlach, Wu¨rzburg Peter Riederer, Wu¨rzburg Andreas Warnke, Wu¨rzburg Luis Rohde, Porto Alegre 123 S2 ABSTRACTS Introduction Dear Colleagues and Friends, We are pleased to have received more than 180 poster abstracts as well as more than 100 poster abstracts from young scientists and clinicians (\ 35 years) who applied for our Young Scientists’ Award. Of all abstracts submitted by our young colleagues, the Scientific Programme Committee has selected the best eight. The authors have been invited to give a presentation as part of our two Young Scientist Award Sessions and to receive a prize money in the amount of 500 Euros. With this approach, we intend to highlight the importance of original scientific contributions, especially from our young colleagues. In this volume, the abstracts of our two Young Scientist Award Sessions come first, followed by regular poster abstracts. These have been organized by topics: Aetiology, Autism Spectrum Disorders, Co-morbidity, Diagnosis, Electrophysiology, Epidemiology, Experimental Models, Genetics, Neuroimaging, Non-pharmacological Treatment, Pathophysiology, Pharmacological Treatment, Quality of Life/Caregiver Burden, Substance Use Disorders and Miscellaneous. Submitted abstracts have not been modified in any way. Please, do not just read the selected poster abstracts, we also encourage you to actively discuss and share your ideas with our young colleagues. Finally, we would like thank all our speakers, contributors and sponsors of our 7th World Congress on ADHD: from Childhood to Adult Disease, and welcome you to join—what we are sure will be—a very enjoyable and highly informative event. -
Treatment-Resistant Attention-Deficit Hyperactivity Disorder: Clinical
Review Treatment-resistant Attention-deficit Hyperactivity Disorder: Clinical Significance, Concept, and Management Mu‑Hong Chen, M.D., Ph.D.1,2, Kai‑Lin Huang, M.D.1,2, Ju‑Wei Hsu, M.D.1,2,*, Shih‑Jen Tsai, M.D.1,2,* 1Department of Psychiatry, Taipei Veterans General Hospital, 2Division of Psychiatry, School of Medicine, National Yang‑Ming University, Taipei, Taiwan Abstract Background: Attention-deficit hyperactivity disorder (ADHD) is the most commonly diagnosed neurodevelopmental disorder known to cause impairment across the lifespan. ADHD was ranked as approximately the 50th leading cause of global years lived with disability for children, coming in ahead of diabetes, meningitis, and intellectual disability. About 20%–40% of patients with ADHD would not achieve the treatment response and symptomatic remission, increasing future risks of substance abuse, suicidal behavior, and premature mortality. However, there is no standard consensus for defining treatment resistance in ADHD.Method: In this systematic review, we intend to focus on treatment-resistant ADHD in the aspects of disease definition, psychopathology, pathophysiology, and treatment. Results: We suggest that the more ideal strategy of defining treatment resistance should consider the improvement of ADHD symptoms and the global functioning simultaneously. Psychiatric comorbidities (i.e. destructive behavior disorders and mood disorders), physical comorbidities (i.e. epilepsy), and psychosocial adversities (i.e. parental psychopathology and poor family functioning) should be the first to be assessed in the evaluation of treatment response or resistance. The optimal medication adjustment or the combination of medications and psychotherapy may be the potential therapeutic strategy for treatment-resistant ADHD. Conclusion: Further studies would be necessary to elucidate the underlying mechanisms of treatment-resistant ADHD and to research the novel treatment strategies for ADHD. -
Sluggish Cognitive Tempo: Current Knowledge and Future Directions
Research RESEARCH BRIEFS Sluggish Cognitive Tempo: Current Knowledge and Future Directions by Lisa Jacobson, PhD, NCSP Do you find yourself saying, “Come on! Hurry up!” multiple times each day? Maybe she is your daughter or he is a student in your classroom. She is the last one to com- plete her work, turn in that test, or even get her things together to go home. Even when he is paying attention, he just seems to need longer than anyone else to get the job done. Neither child is particularly hyperactive. Could this be ADHD? Something else? in interest in better characterizing the be- almost sixty percent of those with SCT The something else might be sluggish havioral characteristics of sluggish cogni- also met criteria for diagnosis of ADHD cognitive tempo (SCT). A growing body of tive tempo and determining whether and and close to forty percent of those meet- evidence suggests that SCT is a distinct be- to what degree symptoms are distinct from ing the diagnostic symptom threshold for havioral pattern, or phenotype, that is both ADHD and other childhood disorders. ADHD also had SCT. Other studies have overlapping and yet distinct from ADHD similarly found that approximately thirty and other childhood disorders. Identified Measuring SCT symptoms to sixty percent of youth with ADHD inat- during field trials for the fourth edition of SCT symptoms are usually measured with tentive type show high levels of SCT.These the Diagnostic and Statistical Manual of behavior rating scales, typically completed numbers suggest that a large proportion Mental Disorders (DSM-IV), SCT is char- by parents or teachers. -
Sluggish Cognitive Tempo (SCT) (AKA) Crichton Syndrome Severity Will Be Specified
Adult AD/HD Update for the Practicing Clinician Kevin T. Blake, Ph.D., P.L.C. Tucson, Arizona Southwest Continuing Education Kevin T. Blake, Ph.D., P.L.C. www.drkevintblake.com 1 All Rights Reserved In the effort to comply with the appropriate boards/associations, I declare that I do have affiliations with or financial interest in a commercial organization that could pose a conflict of interest with my presentation. Adult AD/HD Update for the Practicing Clinician Kevin T. Blake, Ph.D., P.L.C. owns shares in the following companies: Amgen, Inc. Johnson & Johnson, Inc. Southwest Continuing Education Kevin T. Blake, Ph.D., P.L.C. www.drkevintblake.com 2 All Rights Reserved “DSM®, DSM-IV-TR®, and DSM-5® are registered trademarks of the American Psychiatric Association. The American Psychiatric Association is not affiliated with nor endorses this seminar.” Kevin T. Blake, Ph.D., P.L.C. www.drkevintblake.com 3 All Rights Reserved What is a Disorder? • A disorder is a harmful dysfunction of a naturally selected mechanism. Wakefield, J.C. (1999). Evolutionary Versus Prototype Analysis of the Concept of Disorder. Journal of Abnormal Psychology, 108 (3), pp. 374-399. • It must cause a dysfunction in a trait every human develops and create impairment in a major life activity. Barkley, R.A. (2006). Attention-Deficit Hyperactivity Disorder, Third Edition. New York, NY: Guilford, p. 86, 92-93. Kevin T. Blake, Ph.D., P.L.C. www.drkevintblake.com 4 All Rights Reserved What is a Developmental Disorder? It is disorder characterized by a significant delay in the rate a normal human trait develops in an individual. -
NIH Public Access Author Manuscript Alcohol Clin Exp Res
NIH Public Access Author Manuscript Alcohol Clin Exp Res. Author manuscript; available in PMC 2014 January 01. NIH-PA Author ManuscriptPublished NIH-PA Author Manuscript in final edited NIH-PA Author Manuscript form as: Alcohol Clin Exp Res. 2013 January ; 37(Suppl 1): E338–E346. doi:10.1111/j.1530-0277.2012.01886.x. Prenatal Alcohol Exposure, ADHD, and Sluggish Cognitive Tempo Diana M. Graham, B.A.1, Nicole Crocker, M.A.1, Benjamin N. Deweese, B.A.1, Scott C. Roesch, Ph.D.2, Claire D. Coles, Ph.D.3,4, Julie A. Kable, Ph.D.4, Philip A. May, Ph.D.5,6, Wendy O. Kalberg, M.A.6, Elizabeth R. Sowell, Ph.D.7,8, Kenneth Lyons Jones, M.D.9, Edward P. Riley, Ph.D.1,2, Sarah N. Mattson, Ph.D.1,2, and the CIFASD* 1Center for Behavioral Teratology, San Diego State University, San Diego, CA 92120 2Department of Psychology, San Diego State University, San Diego, CA 92182 3Department of Psychiatry and Behavior Sciences, Emory University School of Medicine, Atlanta, GA 30322 4Department of Pediatrics, Emory University School of Medicine, Atlanta, GA 30322 5Department of Nutrition, Gillings School of Global Public Health, University of North Carolina Nutrition Research Institute, Kannapolis, NC 28081 6Center on Alcoholism, Substance Abuse and Addictions, The University of New Mexico, Albuquerque, NM 87131 7Developmental Cognitive Neuroimaging Laboratory, Department of Pediatrics, Keck School of Medicine, University of Southern California, Los Angeles, CA 90027 8Division of Research on Children, Youth, and Families, Department of Pediatrics, Children’s Hospital Los Angeles 9University of California, San Diego, School of Medicine, Department of Pediatrics, San Diego, CA 92093 Abstract Background—Children with heavy prenatal alcohol exposure often meet criteria for attention- deficit/hyperactivity disorder (ADHD). -
Transcranial Direct Current Stimulation on Dorsolateral
Transcranial Direct Current Stimulation on Dorsolateral Prefrontal Cortex as a possible tool to improve Attention in a healthy sample and how Mind Wandering, Sluggish Cognitive Tempo and multiple Psychopathological variables are related. Miquel Puigserver Ferrer Master’s Thesis Master’s degree in General Health Psychology at the UNIVERSITAT DE LES ILLES BALEARS 2018-2019 Date: September 14th, 2019 UIB Master’s Thesis Supervisor: Mateu Servera Barceló. ii Abstract Objectives: The present study has been divided in two parts. The aim of the first study was to find if anodal transcranial direct current stimulation (tDCS) over the right dorsolateral prefrontal cortex (DLPFC) in young healthy adults could enhance some attentional variables. The objective of the second study was to analyze the relationship between Mind Wandering (MW) and Sluggish Cognitive Tempo (SCT), and with some psychiatric symptoms. Methodology: We administrated a sociodemographic formulary which also included three scales (Adult Concentration Inventory (ACI), Mind Excessively Wandering Scale (MEWS) and DSM-5 Self-Rated Level 1 Cross-Cutting Symptom Measure — Adult (CCSM)), to a communitarian sample (n=43) of young adults. Ten of those forty-three participants were included in the tDCS study, where they received a single session of active (n=5) or sham (n=5) tDCS. Before and after the stimulation participants performed Conners’ Continnuous Performance Test (CPT-3) and Attention Network Task (ANT). Results: Those participants who received active tDCS uniquely showed a large and significant reduction of the variability of block change reaction time in the CPT-3 task. No significant differences were found in the three attentional networks, although the sham group showed a decrease in the efficiency of the executive network. -
Resources for Attention Deficit Hyperactivity Disorder (ADHD) Developed by Centers for Family Change
Resources for Attention Deficit Hyperactivity Disorder (ADHD) Developed by Centers for Family Change http://www.centersforfamilychange.com Web Resources for ADHD in Children, Adolescents and Adults The following websites have been reviewed by our staff and have been found to offer sound information on ADHD. We have listed only 6 websites because in our view these websites are all excellent resources, which provide extensive information on ADHD. These websites are scientific; the information provided is research based. Moreover, none of them are “selling” services in a way that would compromise their objectivity, a problem with many existing websites. http://www.russellbarkley.org/factsheets.html This is Barkley’s own site, with a specific link to his "Fact Sheets" on ADHD. While this site heavily promotes Barkley's books, lectures and professional activities his "Fact sheets" are a very solid source of information on ADHD, from the leading expert in the field. Fact Sheets include: a general fact sheet on ADHD, a discussion of classroom management strategies, a discussion of executive functioning, and a new discussion of "sluggish cognitive tempo" and a second type of attention deficit disorder. There is also an internet lecture, by Dr. Barkley, designed for parents, which is well worth viewing. This lecture, along with a number of other interesting lectures on ADHD by Dr. Barkley, can be found at: http://www.adhdlectures.com/index.php. http://www.drthomasebrown.com/ Tom Brown, Ph.D., offers a slightly different model of ADHD than Russell Barkley. His emphasis on and discussion of Executive Functioning is both illuminating and thought provoking. -
Do Symptoms of Sluggish Cognitive Tempo Independently Predict Non-Medical Use Of
Do Symptoms of Sluggish Cognitive Tempo Independently Predict Non-Medical Use of Prescription Stimulants in a College Student Sample? by Jessica Gayleard A thesis submitted to the Graduate Faculty of Auburn University in partial fulfillment of the requirements for the Degree of Master of Science Auburn, Alabama May 5, 2019 Copyright 2018 by Jessica Gayleard Approved by Steven K. Shapiro, Ph.D., Chair, Associate Professor of Psychology Joseph R. Bardeen, Ph.D., Assistant Professor of Psychology Chris Correia, Ph.D., Professor of Psychology Abstract As evidence for the construct of SCT continues to grow, it is important for further research to continue investigating how symptoms of SCT may impact functioning in college students. The current study examined whether SCT symptoms in college students are predictive of nonmedical use of prescription stimulants (NMUPS), above and beyond those of commonly comorbid disorders, including symptoms of ADHD, depression, and anxiety. Participants (N = 1142) were undergraduate college students attending a public, Southeastern university who completed an online survey. Prevalence of NUMPS at least once in the students’ lifetime or over the past 12 months was 19% and 13%, respectively. SCT was moderately correlated with ADHD and internalizing symptoms. NMUPS was modestly correlated with ADHD, internalizing symptoms, and SCT. Using hierarchical regression models, ADHD-Inattention and depressive symptoms often significantly and uniquely predicted NMUPS, but the strength or consistency of these findings was dependent upon the timespan over which participants reported their use and whether NMUPS was analyzed based on use/nonuse or frequency of use. SCT did not uniquely contribute to the prediction of NMUPS but often influenced the unique prediction of previously entered clinical variables for the final models. -
Sluggish Cognitive Tempo) Russell A
Barkley 1 Concentration Deficit Disorder (Sluggish Cognitive Tempo) Russell A. Barkley, Ph.D. Clinical Professor of Psychiatry and Pediatrics Medical University of South Carolina This Fact Sheet is based on a chapter to appear in the next edition of Dr. Barkley’s textbook, Attention Deficit Hyperactivity Disorder: A Handbook for Diagnosis and Treatment (4th edition). New York: Guilford Press. In press; expected publication date – December 2014. This chapter reviews the evidence for a second attention disorder that is distinct from yet overlaps with ADHD. Although this condition has been called Sluggish Cognitive Tempo (SCT) since the 1980s, I have recently recommended that the name be changed to Concentration Deficit Disorder (CDD) for various reasons, not the least of which is that it can be viewed by the public as pejorative, derogatory, or frankly offensive (Barkley, 2014; Saxbe & Barkley, 2014). While some prior reviewers of the evidence have suggested that the disorder be called attention deficit disorder, or ADD (Diamond, 2005; Milich & Roberts, 2012), and many clinicians have adopted this term for people who are primarily inattentive and have little or no evidence of hyperactive or impulsive behavior, it is not advisable to do so. Not the least reason for which is that ADD is the older term for ADHD dating back to DSM-III in 1980 and so resurrecting it as the name for a second attention disorder merely unnecessary creates confusion between these conditions which, as will be shown below, are quite different in a number of important features. The term SCT also implies that the neurocognitive dysfunction underlying the condition is well-known and supported by empirical evidence, and this is very far from the case at the moment. -
Sluggish Cognitive Tempo and Its Neurocognitive, Social and Emotive
Mueller et al. Journal of Molecular Psychiatry 2014, 2:5 http://www.jmolecularpsychiatry.com/content/2/1/5 JMP REVIEW Open Access Sluggish cognitive tempo and its neurocognitive, social and emotive correlates: a systematic review of the current literature Anna Katharina Mueller1, Lara Tucha1*, Janneke Koerts1, Yvonne Groen1, Klaus W Lange2 and Oliver Tucha1 Abstract Objectives: Since the elimination of items associated with Sluggish Cognitive Tempo (SCT) during the transition from DSM-III to DSM-IV from the diagnostic criteria of Attention-deficit Hyperactivity Disorder (ADHD), interest in SCT and its associated cognitive as well as emotional and social consequences is on the increase. The current review discusses recent findings on SCT in clinical as well as community based ADHD populations. The focus is further on clinical correlates of SCT in populations different from ADHD, SCT’s genetic background, SCT’s association with internalizing and other behavioral comorbidities, as well as SCT’s association with social functioning and its treatment efficacy. Method: A systematic review of empirical studies on SCT in ADHD and other pathologies in PsycInfo, SocIndex, Web of Science and PubMed using the key terms “Sluggish Cognitive Tempo”, “Cognitive Tempo”, “Sluggish Tempo” was performed. Thirty-two out of 63 studies met inclusion criteria and are discussed in the current review. Results/Conclusion: From the current literature, it can be concluded that SCT is a psychometrically valid construct with additive value in the clinical field of ADHD, oppositional defiant disorder (ODD), internalizing disorders and neuro-rehabilitation. The taxonomy of SCT has been shown to be far from consistent across studies; however, the impact of SCT on individuals’ functioning (e.g., academic achievement, social interactions) seems remarkable.