Is Football (Soccer) an Indicator of Development at the International Level
Total Page:16
File Type:pdf, Size:1020Kb
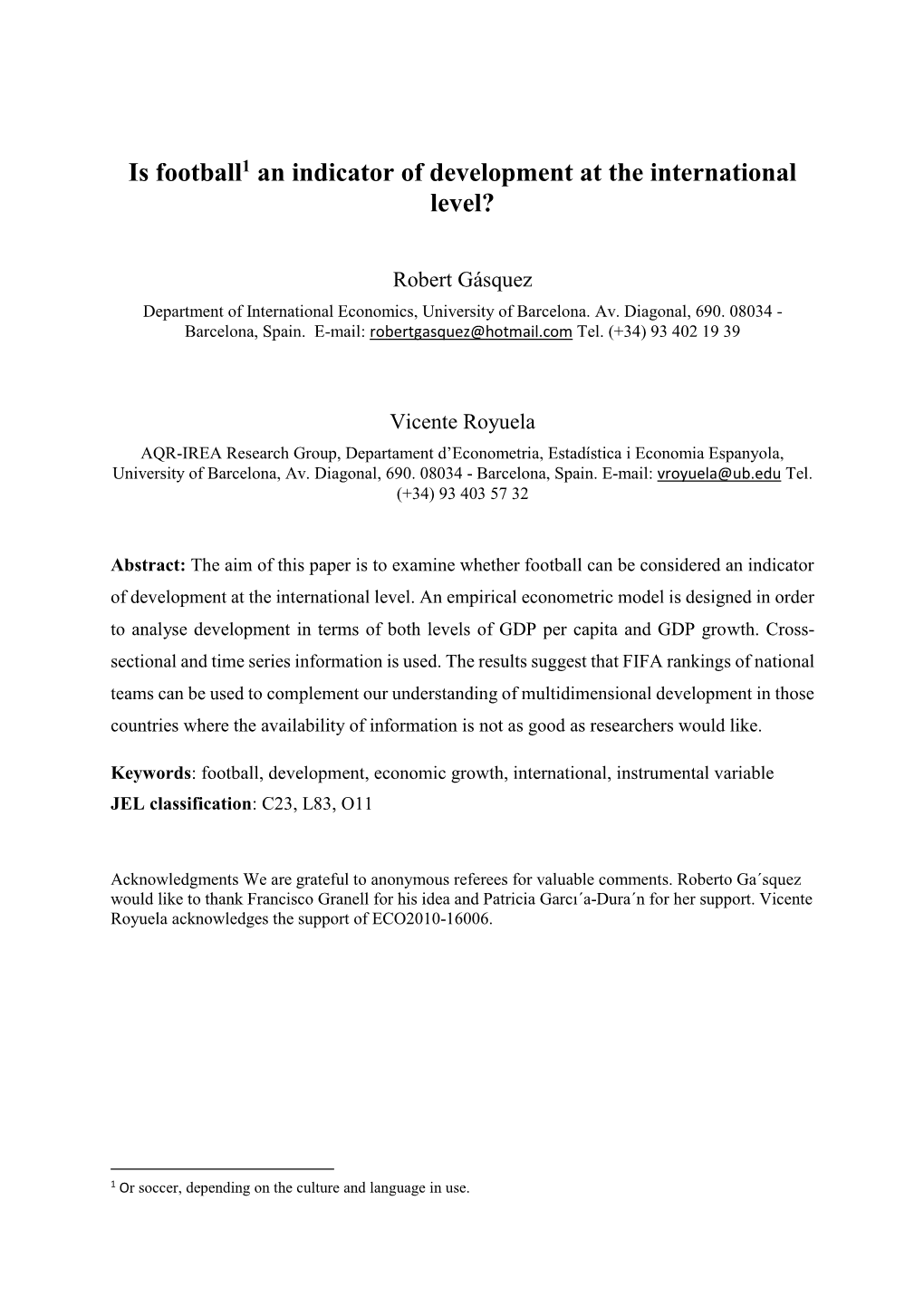
Load more
Recommended publications
-
Debate No 23 of 2009
MAURITIUS FOURTH NATIONAL ASSEMBLY FIRST SESSION Debate No. 23 of 2009 Sitting of Tuesday 21 July 2009 The Assembly met in the Assembly House, Port Louis at 11.30 a.m. The National Anthem was played (Mr Speaker in the Chair) CONTENTS ANNOUNCEMENTS PAPERS LAID QUESTIONS (Oral) MOTION STATEMENT BY MINISTER BILLS (Public) ADJOURNMENT QUESTIONS (Written) MAURITIUS -------------- Fourth National Assembly --------------- First Session Debate No. 23 of 2009 Sitting of Tuesday 21 July 2009 ANNOUNCEMENTS CARDINAL JEAN MARGÉOT - DEATH Mr Speaker: Hon. Members, before we start with the business of the day, I will kindly invite you to stand up to observe one minute of silence in memory of the late Cardinal Jean Margéot who passed away on Friday 17 July 2009. Members observed a minute of silence. THE FINANCE (MISCELLANEOUS PROVISIONS) BILL – POINT OF ORDER - RULING Mr Speaker: Hon. Members, at the sitting of the House last Tuesday, the hon. Leader of the Opposition, following the introduction of the Finance (Miscellaneous Provisions) Bill at First Reading raised a point of order. The point of order may be summarised as follows – (a) the Parliamentary Practice so far has been that the Minister of Finance presents the Bill, that is, the Finance (Miscellaneous Provisions) Bill in the House and, after listening to him, the Leader of the Opposition stands up and replies in the light of what he has said; (b) in this Finance Bill, there are issues that have nothing to do with the Ministry of Finance; (c) he made specific reference to the proposed amendments to the Bail Act, the Certificate of Morality Act, the Law Reform Commission Act, Tourism and so on, and (d) the hon. -
Women's Football, Europe and Professionalization 1971-2011
Women’s Football, Europe and Professionalization 1971-2011 A Project Funded by the UEFA Research Grant Programme Jean Williams Senior Research Fellow International Centre for Sports History and Culture De Montfort University Contents: Women’s Football, Europe and Professionalization 1971- 2011 Contents Page i Abbreviations and Acronyms iii Introduction: Women’s Football and Europe 1 1.1 Post-war Europes 1 1.2 UEFA & European competitions 11 1.3 Conclusion 25 References 27 Chapter Two: Sources and Methods 36 2.1 Perceptions of a Global Game 36 2.2 Methods and Sources 43 References 47 Chapter Three: Micro, Meso, Macro Professionalism 50 3.1 Introduction 50 3.2 Micro Professionalism: Pioneering individuals 53 3.3 Meso Professionalism: Growing Internationalism 64 3.4 Macro Professionalism: Women's Champions League 70 3.5 Conclusion: From Germany 2011 to Canada 2015 81 References 86 i Conclusion 90 4.1 Conclusion 90 References 105 Recommendations 109 Appendix 1 Key Dates of European Union 112 Appendix 2 Key Dates for European football 116 Appendix 3 Summary A-Y by national association 122 Bibliography 158 ii Women’s Football, Europe and Professionalization 1971-2011 Abbreviations and Acronyms AFC Asian Football Confederation AIAW Association for Intercollegiate Athletics for Women ALFA Asian Ladies Football Association CAF Confédération Africaine de Football CFA People’s Republic of China Football Association China ’91 FIFA Women’s World Championship 1991 CONCACAF Confederation of North, Central American and Caribbean Association Football CONMEBOL -
Governance Relationships in Football Between Management and Labour Roitman - Governance Relationships Marston, C
Building on the two prior CIES governance studies, this is the third FIFA-mandated research analysing governance relationships in football. This book focuses on those Editions CIES between football’s employers (clubs, leagues and even NAs) and its labour force. Based on a sample of forty countries across all six confederations and questionnaires from players’ associations, leagues and national associations, this research surveys and compares the diverse ‘management-labour’ approaches and scenarios in both men and women’s professional football worldwide. GOVERNANCE RELATIONSHIPS The authors place a special focus on players’ associations and highlight the variety of IN FOOTBALL BETWEEN structures found world-wide. The findings here contribute to a better understanding MANAGEMENT AND LABOUR of the systems, models and relationships in place around the globe when it comes to PLAYERS, CLUBS, LEAGUES & NATIONAL ASSOCIATIONS ‘management’ and ‘labour’. This book explores the representation of Kevin Tallec Marston, Camille Boillat & Fernando Roitman players within decision-making structures at club, league and national association level as well as the regulatory contexts and negotiation instruments linking players and management - such as collaborative agreements/MoUs, CBAs, minimum contract requirements and dispute resolution. In addition, this study provides a first ever global exploration of some of the inner workings of players’ associations and an overview of the key issues in professional football from the player’s perspective. The final chapter offers several models and frameworks illustrating the governance relationships between players and management. All three authors work at the International Centre for Sport Studies (CIES). Kevin Tallec Marston earned his PhD in history and works as research fellow and academic projects manager. -
Annual Report 2014 Dear Shareholder
FINANCIAL STATEMENTS 34- 30 80 INDEPENDENT DIRECTORS AUDITORS’ AND SENIOR REPORT 81- MANAGEMENT 83 PROFILES SE CRET ARY’S REP 29 ORT 84 OTHER STATUTORY NOTICE DISCLOSURES TO 26- SHARE 27 HOLDERS CON TENTS AUTOMATIC SYSTEMS LTD. ANNUAL REPORT 2014 DEAR SHAREHOLDER, Your Board of Directors is pleased to present the Annual Report of Automatic Systems Ltd for the year ended 31 December 2014. This report was approved by the Board of Directors on 24 March 2015. The financial statements of the Company are set out on page 34 to 80 and the Auditors’ Report is on page 30. As a shareholder, you are invited to attend the Annual Meeting of the Company which will be held as follows: DATE FRIDAY 29 MAY 2015 TIME 15 00HRS VENUE THE MAURITIUS TURF CLUB, CHAMP DE MARS, PORT LOUIS The notice of the Annual Meeting can be viewed on page 84 of the Annual Report. Kind regards, Eric Espitalier Noel Jean Hardy Chairman Director 02 AUTOMATIC SYSTEMS LTD. > ANNUAL REPORT 2014 BOARD AND COMMITTEES MANAGEMENT ADMINISTRATION Board OF DIRECTORS REGISTERED OFFICE C/o Box Office Ltd Chairman 2nd Floor, Nautica Commercial Centre, and Independent Director Royal Road, Black River M. A. Eric Espitalier Noël COMPANY SECRETARY Executive Directors Box Office Ltd M. L. Jean Hardy 2nd Floor, Nautica Commercial Centre, J. O. Guillaume Hardy (Managing Director) Royal Road, Black River (appointed as Managing Director on 18/03/2014) REGISTRY Non-Executive Directors AND TRANSFER OFFICE Hervé Henry ECS Secretaries Ltd Michel J. L. Nairac 3rd Floor, Labama House, John A. Stuart Sir William Newton Street, Port Louis Independent Directors AUDITOR Ravindra Chetty PricewaterhouseCoopers O. -
Football Diplomacy Research Fellow Tel
POLICY BRIEF November 2005 ANTHONY BUBALO Football Diplomacy Research Fellow Tel. +61 2 8238 9140 [email protected] What is the problem? While Australian governments have successfully built pragmatic ties with Asian leaders, a popular dimension to our engagement with Asia has in many respects been missing. This didn’t matter greatly in the past, but today public opinion is increasingly a factor in foreign policy. Governments must influence individuals as well as elites to address global problems such as terrorism and disease and ‘branding’ has become critical to a state’s ability to attract trade, investment and international political support. But a new opportunity to deepen people-to-people links with Asia has arrived in the form of Australia’s recent admission into the Asian Football Confederation. For the first time, Australia will have a significant sporting relationship with Asia. The question is, how can Australia best use this opportunity to enhance its regional image and engagement? What should be done? Here are five ideas for how government, business and the broader community might leverage Australia’s new sporting relationship with Asia: • Football Federation Australia (FFA) should work with business and government to establish a ‘Football Asia Council’ to coordinate commercial, cultural, and public diplomacy programs with Australian participation in Asian football competitions. • FFA, business and government should launch a coordinated effort through this Council to develop football-based tourism and travel. • Austrade should establish a ‘Football Business Club Australia’ to facilitate commercial networking opportunities during matches between Australian and LOWY INSTITUTE FOR Asian teams. FFA should also consider initiating a tri-nations series with Japan INTERNATIONAL POLICY and South Korea, providing an opportunity for greater commercial, cultural, and 31 Bligh Street political engagement with these key countries. -
Off Pitch: Football's Financial Integrity Weaknesses, and How to Strengthen
Off Pitch: Football’s financial integrity weaknesses, and how to strengthen them Matt Andrews and Peter Harrington CID Working Paper No. 311 January 2016 Copyright 2016 Andrews, Matt; Harrington, Peter; and the President and Fellows oF Harvard College Working Papers Center for International Development at Harvard University Off Pitch: Football’s financial integrity weaknesses, and how to strengthen them Matt Andrews and Peter Harrington1 Abstract Men’s professional football is the biggest sport in the world, producing (by our estimate) US $33 billion a year. All is not well in the sector, however, with regular scandals raising questions about the role of money in the sport. The 2015 turmoil around FIFA is obviously the most well known example, creating a crisis in confidence in the sector. This study examines these questions, and the financial integrity weaknesses they reveal; it also offers ideas to strengthen the weaknesses. The study argues that football’s financial integrity weaknesses extend far beyond FIFA. These weaknesses have emerged largely because the sector is dominated by a small elite of clubs, players and owners centered in Europe’s top leagues. The thousands of clubs beyond this elite have very little resources, constituting a vast base of ‘have-nots’ in football’s financial pyramid. This pyramid developed in recent decades, fuelled by concentrated growth in new revenue sources (like sponsorships, and broadcasting). The growth has also led to increasingly complex transactions—in player transfers, club ownership and financing (and more)—and an expansion in opportunities for illicit practices like match-fixing, money laundering and human trafficking. We argue that football’s governing bodies – including FIFA – helped establish this pyramid. -
Cabinet Decisions Taken on 11 December
Page 1 of 5 CABINET DECISIONS – 11 DECEMBER 2009 1. Cabinet has taken note of the outcome of the mission of Dr the Hon Navinchandra Ramgoolam, GCSK, FRCP, Prime Minister, from 27 to 29 November 2009, to the Republic of Trinidad and Tobago where he attended the Commonwealth Heads of Government Meeting (CHOGM). He was accompanied, amongst others, by Dr the Hon Arvin Boolell, Minister of Foreign Affairs, Regional Integration and International Trade. The special theme of CHOGM 2009 was “Partnering for a more Equitable and Sustainable Future”. CHOGM adopted The Port of Spain Communiqué which encompasses the promotion of Tolerance, Respect and Understanding, Disarmament and Arms Control, Terrorism, Combating Piracy and Corruption, Human Rights, World Economic Situation, Reform of International Institutions, Trade and Investment Issues, Sustainable Development, Energy and Food Security, Challenges facing Small States, Health, Education, Gender and Youth Issues and Bridging the Digital Divide. The Meeting also adopted the Port of Spain Climate Change Consensus: The Commonwealth Climate Change Declaration. Mauritius played a leading role in the debates, as well as in the drafting of the Declaration on Climate Change. In his intervention on the issue of Climate Change, the Prime Minister brought out the high vulnerability and specificities of Small Island Developing States (SIDS) and the need for urgent action to enable them to build their resilience to be able to withstand the inevitable impacts of climate change and pursue sustainable development. He also highlighted that local initiatives could be nullified and achievements swept out if proper attention was not given to food, water, land and energy security. -
Development Through Football Sustaining the Potential of the First African World Cup
Conference Report Development through Football Sustaining the potential of the first African World Cup 23 – 24 April 2010 Vienna www.footballfordevelopment.net Conference Report Development through Football Sustaining the potential of the first African World Cup Table of contents Opening Session 4 Key note address 6 Opening Addresses 7 Plenary Session 1 10 Conference Day Two 23 Closing Plenary Session 25 Vienna Action Plan 28 List of Participants 29 Project Partners & Associates 30 IMPRINT © 2010 | Publisher: VIDC – Vienna Institute for International Dialogue and Cooperation, Möllwaldplatz 5/3, A-1040 Vienna, Austria, Tel. +43 171 33594, Fax +43 171 33594-73, E-Mail: [email protected] | www.vidc.org, www.fairplay.or.at | Written and collected by Phil Vasili | Editors: Bella Bello Bitugu, Kurt Wachter | Photos: Stefan Radvanyi / www.picturedb.at, Max Moser, Kurt Wachter / FairPlay-vidc | Graphic design: typothese.at / m.zinner grafik, Sanja Jelic This report has been produced with the financial assistance of the European Union. The content of this report are the sole responsibility of VIDC and the respective project partners and can under no circumstances be regarded as reflecting the position of the European Union. 2 Football for Development Preface Sports in general but football in particular have become phe- fan initiatives, players’ unions, sport organisations and media, nomenons that have huge potentials as channels and tools for academic institutions as well as governmental bodies including social development due to the huge number of people from di- national development agencies, United Nations and European verse backgrounds that they appeal to. They are fertile grounds Union institutions, from 27 different European and African to be used as platform to be used as social and development countries. -
Our Elite Group of Players Gear up for 1 Week's Training with FC Barcelon
AfrAsia Barcelona Club – From 1,850 to a final 24! Our elite group of players gear up for 1 week’s training with FC Barcelona in Spain! Saturday 28th June AfrAsia Bank, Football First and McDonald’s today announced the names of the top 24 players selected from last week’s final trial at the New George V Stadium, ending the suspense of our young footballers from across the nation. Hon. Satyaprakash (Devanand) Ritoo, Minister of Youth & Sports was present to personally congratulate the players and their parents. With a total of 1,850 participants having been evaluated in Mauritius and Rodrigues, both organisers and partners are proud of the success of this event, a first on the island and a stepping stone for the future phases of this pioneering football program. “The last month has been hard work but very rewarding for us all to see the talented young Mauritian footballers. It would have not been possible without the involvement of our sponsors, coaches, and registration staff, plus the student volunteers from Le Bocage International School. It motivates all of the staff to see the skills and commitment the boys and parents have to improve the football in Mauritius. I would also like to thank all the boys who took part in this first national selection process, they should not feel that they failed as to us at Football First they were all winners for participating. There will be more options in the very near future to improve their skills when we open the doors for the Football First coaching courses starting in September. -
Business Plan:“Casa Do Benfica De Maputo”
BUSINESS PLAN:“CASA DO BENFICA DE MAPUTO” Miguel Luís Carrilho Fortes da Cunha Project Submitted as partial requirement for the conferral of Master in International Management Supervisor: Prof. Renato Pereira, Prof. Auxiliar Convidado, ISCTE Business School, Departamento de Marketing, Operações e Gestão Geral September 2019 “CASA DO BENFICA DE MAPUTO” “CASA DE DO BENFICA Da Miguel CunhaCarrilho Luís Fortes BUSINESSPLAN: Business plan: “Casa do Benfica de Maputo” Abstract "Fado, Football and Fátima" are the three words that have always characterized our people. It's an expression that nobody knows where it came from, but the truth is that the vast majority of the Portuguese associate it with the period in which we were ruled by a dictatorship. Today we can say that, especially, football is still very present in Portuguese culture, and a great example is the number of television shows related to the "king" sport that our country broadcasts every day. That's why we've managed to understand that there's a market to explore, both in Portugal and in the former Portuguese colonies, where there is still a cultural and sporting connection. The "Casas do Benfica", throughout their history, have always had a very simple purpose: to bring together the different benefactors in a place where everyone could meet and "share their benevolence" even if it was not possible for them to go to the stadium. To this end, these small facilities began to be built in different parts of the country, where there could be a community of supporters and sympathizers of Sport Lisboa e Benfica. -
2016 Relatório E Contas Annual Report
2016 RELATÓRIO E CONTAS ANNUAL REPORT Prestes a completar uma década da Reversão da Hidroeléctrica de Cahora Bassa, olhamos para o futuro com orgulho no caminho percorrido. Juntos atingimos recordes históricos de produção de energia limpa e sustentável para fazer o país avançar. Um ponto de viragem num percurso iniciado em 1975, que afirmou a excelência da HCB no desenvolvimento de Moçambique. As we approach the tenth anniversary of the Hidroeléctrica de Cahora Bassa dam ownership reversion, we look to the future with pride in our achievements. Together we have set historical records for production of clean and sustainable energy to power the country's progress. A ENERGIA A turning point in a journey that QUE NOS FAZ started in 1975, that affirmed AVANÇAR the excellence of HCB. POWER THAT MOVE US FORWARD PORMENOR DO MURAL COMEMORATIVO DO 5º ANIVERSÁRIO DA REVERSÃO DETAIL OF THE MURAL COMMEMORATING THE 5TH ANNIVERSARY OF THE OWNERSHIP REVERSION 2016 RELATÓRIO E CONTAS ANNUAL REPORT Prestes a completar uma década da Reversão da Hidroeléctrica de Cahora Bassa, olhamos para o futuro com orgulho no caminho percorrido. Juntos atingimos recordes históricos de produção de energia limpa e sustentável para fazer o país avançar. Um ponto de viragem num percurso iniciado em 1975, que afirmou a excelência da HCB no desenvolvimento de Moçambique. As we approach the tenth anniversary of the Hidroeléctrica de Cahora Bassa dam ownership reversion, we look to the future with pride in our achievements. Together we have set historical records for production of clean and sustainable energy to power the country's progress. -
The Worldwide Diffusion of Football: Temporal and Spatial Perspectives
1 The Worldwide Diffusion of Football: Temporal and Spatial Perspectives John S. Hill, John Vincent, & Matthew Curtner-Smith, University of Alabama Abstract The purpose of this study is to evaluate how football evolved from a village activity in rural England to become the world’s premier sport played in 208 countries. First, its diffusion in England is evaluated over time as well as how it spread spatially across the nation. Second, soccer’s diffusion worldwide is analyzed both temporally and geographically to assess the rate at which the sport spread and how its adoption moved from nation to nation across the major regions of the world. Principal findings were that 40 years was the time taken for soccer to take root nationally and regionally; and that spatially, east-west diffusion occurred first across regions before its spread northwards and southwards. The principal reasons for its adoption worldwide were the game’s simplicity, flexibility, and its appeal to common global values of teamwork, fair play, and equality. Globalization synergies are key factors accounting for the sport’s worldwide popularity. Introduction Globalization became dominant in the 20th century as world nations began to interact economically with greater frequency, exchanging commodities, goods, and technologies. But while commercial transactions were its driving force, there were also cultural exchanges as Western influences infiltrated to foreign countries to affect country cultures (Hill, 2009). One of these influences was sport, and of all Western cultural exports, sport has perhaps become the most significant for three reasons. First, in a world characterized by increasing industrialization and competition, sport has emerged as a key safety valve for relieving the social and economic stress induced by increasingly competitive societies (Madeiro, 2007).