HFES Europe Proceedings
Total Page:16
File Type:pdf, Size:1020Kb
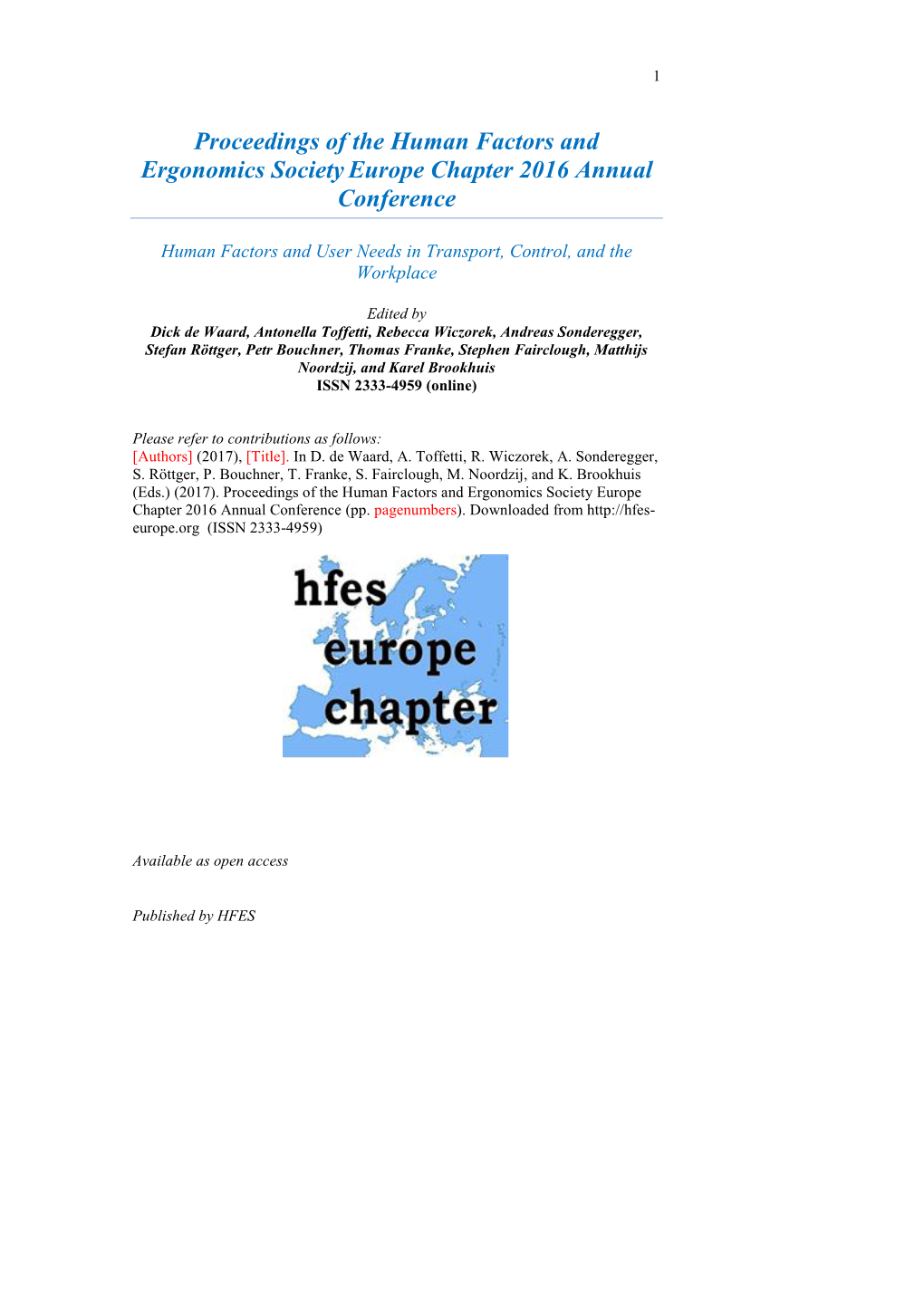
Load more
Recommended publications
-
HFES Europe Proceedings
University of Groningen Proceedings of the Human Factors and Ergonomics Society Europe Chapter 2016 Annual Conference de Waard, Dick; Toffetti, Antonella; Wiczorek, Rebecca; Sonderegger, Andreas; Röttger, Stefan; Bouchner, Petr; Franke, Thomas; Fairclough, Stephen; Noordzij, Matthijs; Brookhuis, Karel IMPORTANT NOTE: You are advised to consult the publisher's version (publisher's PDF) if you wish to cite from it. Please check the document version below. Document Version Publisher's PDF, also known as Version of record Publication date: 2017 Link to publication in University of Groningen/UMCG research database Citation for published version (APA): de Waard, D., Toffetti, A., Wiczorek, R., Sonderegger, A., Röttger, S., Bouchner, P., Franke, T., Fairclough, S., Noordzij, M., & Brookhuis, K. (Eds.) (2017). Proceedings of the Human Factors and Ergonomics Society Europe Chapter 2016 Annual Conference: Human Factors and User Needs in Transport, Control, and the Workplace. (Proceedings of the Human Factors and Ergonomics Society Europe Chapter). HFES. http://www.hfes-europe.org/largefiles/proceedingshfeseurope2016.pdf Copyright Other than for strictly personal use, it is not permitted to download or to forward/distribute the text or part of it without the consent of the author(s) and/or copyright holder(s), unless the work is under an open content license (like Creative Commons). The publication may also be distributed here under the terms of Article 25fa of the Dutch Copyright Act, indicated by the “Taverne” license. More information can be found on the University of Groningen website: https://www.rug.nl/library/open-access/self-archiving-pure/taverne- amendment. Take-down policy If you believe that this document breaches copyright please contact us providing details, and we will remove access to the work immediately and investigate your claim. -
Is Their New Flight Simulator Package Set for Release in 2020 for Both PC and Xbox
New M Announced by Microsoft at Electronic Entertainment Expo 2019 (E3 2019) is their new flight simulator package set for release in 2020 for both PC and Xbox. We weren't expecting this announcement - given the history of Microsoft's attempts to revise its historic Microsoft Flight Simulator package (aka Microsoft Flight). This is a developing story so be sure to bookmark this page - it will be updated regularly with more information as and when it becomes available. The announcement was made via a YouTube video previewing the new sim. At first, we thought it was a hoax (like our 2014 April fools joke) however the video was verified and had been released on Microsoft's official Xbox YouTube channel. You can jump to the individual sections of this article using the links below: Official Release o Release Date o Official Pre-Order Launch Trailer Video o Editions, Pricing & Airports/Aircraft Included COVID-19 (Coronavirus) Delays X019 Update FSX Beta Global Preview Event Analysis FAQ SDK The Announcement Our Analysis What About Those Add-ons? The Next Generation Of Flight Simulation, “For You, With You!” August 8th Update: Development & Control of Flight Simulator X Insider Launch Videos o Discovery Series . World . Weather . Aerodynamics . Cockpits . Soundscape . Airports . Multiplayer Screenshots What do You Think? Release of Microsoft Flight Simulator NEW Posted 14th July 2020 Finally, after all the waiting, Microsoft Flight Simulator is set to be released on the 18th August 2020 courtesy of Xbox Game Studios and Asobo Studio. The release will be available for PC as well as Xbox Game Pass for PC (Beta). -
Free Hoi4 Download
free hoi4 download Hearts Of Iron IV: Field Marshal Edition Free Download (v1.10.4 & ALL DLC’s) Victory is at your fingertips! Your ability to lead your nation is your supreme weapon, the strategy game Hearts of Iron IV lets you take command of any nation in World War II; the most engaging conflict in world history. From the heart of the battlefield to the command center, you will guide your nation to glory and wage war, negotiate or invade. You hold the power to tip the very balance of WWII. It is time to show your ability as the greatest military leader in the world. Will you relive or change history? Will you change the fate of the world by achieving victory at all costs? How to Download & Install Hearts Of Iron IV. Click the Download button below and you should be redirected to UploadHaven. Wait 5 seconds and click on the blue ‘download now’ button. Now let the download begin and wait for it to finish. Once Hearts Of Iron IV is done downloading, right click the .zip file and click on “Extract to Hearts.of.Iron.IV.v1.10.4.zip” (To do this you must have 7-Zip, which you can get here). Double click inside the Hearts Of Iron IV folder and run the exe application. Have fun and play! Make sure to run the game as administrator and if you get any missing dll errors, look for a Redist or _CommonRedist folder and install all the programs in the folder. Hearts Of Iron IV Free Download. -
Train Simulator 2014 PDF Manual
User Manual Ruth Ivimey-Cook © RailSimulator.Com TS2014 – User Manual Contents 1 INTRODUCTION TO TRAIN SIMULATOR 2014 ...................................................... 4 2 TS2014 FEATURES ................................................................................................. 6 3 GETTING STARTED ................................................................................................. 8 3.1 Making a Start .........................................................................................................................................8 3.2 Scenario Types ....................................................................................................................................... 11 3.3 Controlling your Train ......................................................................................................................... 12 3.4 Driving ....................................................................................................................................................14 3.5 Changing Your Point of View ............................................................................................................. 15 3.6 Some other Useful Controls ................................................................................................................ 16 3.7 Driving Information ............................................................................................................................. 16 3.8 The 2D Map View ................................................................................................................................. -
Tekan Bagi Yang Ingin Order Via DVD Bisa Setelah Mengisi Form Lalu
DVDReleaseBest 1Seller 1 1Date 1 Best4 15-Nov-2013 1 Seller 1 1 1 Best2 1 1-Dec-2014 1 Seller 1 2 1 Best1 1 30-Nov-20141 Seller 1 6 2 Best 4 1 9 Seller29-Nov-2014 2 1 1 1Best 1 1 Seller1 28-Nov-2014 1 1 1 Best 1 1 9Seller 127-Nov-2014 1 1 Best 1 1 1Seller 1 326-Nov-2014 1 Best 1 1 1Seller 1 1 25-Nov-20141 Best1 1 1 Seller 1 1 1 24-Nov-2014Best1 1 1 Seller 1 2 1 1 Best23-Nov- 1 1 1Seller 8 1 2 142014Best 3 1 Seller22-Nov-2014 1 2 6Best 1 1 Seller2 121-Nov-2014 1 2Best 2 1 Seller8 2 120-Nov-2014 1Best 9 11 Seller 1 1 419-Nov-2014Best 1 3 2Seller 1 1 3Best 318-Nov-2014 1 Seller1 1 1 1Best 1 17-Nov-20141 Seller1 1 1 1 Best 1 1 16-Nov-20141Seller 1 1 1 Best 1 1 1Seller 15-Nov-2014 1 1 1Best 2 1 Seller1 1 14-Nov-2014 1 1Best 1 1 Seller2 2 113-Nov-2014 5 Best1 1 2 Seller 1 1 112- 1 1 2Nov-2014Best 1 2 Seller1 1 211-Nov-2014 Best1 1 1 Seller 1 1 1 Best110-Nov-2014 1 1 Seller 1 1 2 Best1 9-Nov-20141 1 Seller 1 1 1 Best1 18-Nov-2014 1 Seller 1 1 3 2Best 17-Nov-2014 1 Seller1 1 1 1Best 1 6-Nov-2014 1 Seller1 1 1 1Best 1 5-Nov-2014 1 Seller1 1 1 1Best 1 5-Nov-20141 Seller1 1 2 1 Best1 4-Nov-20141 1 Seller 1 1 1 Best1 14-Nov-2014 1 Seller 1 1 1 Best1 13-Nov-2014 1 Seller 1 1 1 1 13-Nov-2014Best 1 1 Seller1 1 1 Best12-Nov-2014 1 1 Seller 1 1 1 Best2 2-Nov-2014 1 1 Seller 3 1 1 Best1 1-Nov-2014 1 1 Seller 1 1 1 Best5 1-Nov-20141 2 Seller 1 1 1 Best 1 31-Oct-20141 1Seller 1 2 1 Best 1 1 31-Oct-2014 1Seller 1 1 1 Best1 1 1 31-Oct-2014Seller 1 1 1 Best1 1 1 Seller 131-Oct-2014 1 1 Best 1 1 1Seller 1 30-Oct-20141 1 Best 1 3 1Seller 1 1 30-Oct-2014 1 Best1 -
Repositório Aberto
UNIVERSIDADE ABERTA Os Jogos Digitais na Educação Brasileira: uma Análise de Artigos Científicos Luiz Claudio Peixoto de Azevedo Mestrado em Comunicação Educacional e Media Digitais 2020 UNIVERSIDADE ABERTA Os Jogos Digitais na Educação Brasileira: uma Análise de Artigos Científicos Luiz Claudio Peixoto de Azevedo Mestrado em Comunicação Educacional e Media Digitais Orientada por: Professora Doutora Lúcia Amante 2020 ii A investigação realizada no âmbito desta Dissertação está integrada nas linhas de investigação da Unidade de Investigação e Desenvolvimento - Laboratório de Educação a Distância e eLearning1 (UID 4372/FCT), da Fundação para a Ciência e Tecnologia do Ministério da Ciência, Tecnologia e Ensino Superior. 1 https://lead.uab.pt iii Resumo Introdução – Os jogos digitais são disponibilizados, com grande potencial, para realizar transformações na comunicação educacional, em contextos formais ou informais, presenciais ou a distância. Objetivo – Analisar a produção de artigos sobre jogos digitais em revistas científicas, selecionadas dentre as classificadas no arquivo da Coordenação de Aperfeiçoamento de Pessoal de Nível Superior (CAPES), fundação do Ministério da Educação do Brasil, a fim de verificar como apresentam e compreendem os games na educação brasileira. Metodologia – Revisão sistemática da literatura, ancorada na análise documental e orientada pela metodologia mista, a qual integra os enfoques qualitativo e quantitativo em um só estudo. A análise de conteúdo foi realizada com o suporte do Iramuteq, programa estatístico de análise lexical, empregado para organização dos dados. Para a composição do corpus de análise foram selecionados, por intermédio de busca no portal de periódicos da CAPES, artigos em língua portuguesa, revisados por pares, que abordam os jogos digitais, na área da educação. -
Development of Virtual Reality Technology in the Aspect of Educational Applications
DEVELOPMENT OF VIRTUAL REALITY TECHNOLOGY IN THE ASPECT OF EDUCATIONAL APPLICATIONS © 2017. This is an open access article distributed under the Creative Commons Attribution-NonCommercial license (http://creativecommons.org/licenses/by-nc/4.0/) MINIB, 2017, Vol. 26, Issue 4, p. 117–134 DEVELOPMENT OF VIRTUAL REALITY TECHNOLOGY IN THE ASPECT OF EDUCATIONAL APPLICATIONS Małgorzata Żmigrodzka, Ph.D. Polish Air Force Academy, Poland [email protected] DOI: 10.14611/minib.26.12.2017.15 Summary In the recent years we have observed the development of devices and visualizations for monitoring the activity of a user (movement and position) in a virtual environment1. Along with the growing utilization of personal computers for visualization and rapid development of computer image generation in real time, universities, following the latest trends used in science, are looking for solutions to reach students through the senses of: sight, hearing and touch2. We should take into consideration the diversity of students' styles and strategies of learning, that's why the use of virtual reality (VR) in education is a response to the characteristics of the current age. Student as a creative maker and not just a passive recipient deliberately looks for new techniques of acquiring information and thanks to them he or she can build many precious skills, among others, independence in planning or carrying out a task, or cooperating in a team. In this context it is necessary to inform students about research, which is an integral part and foundation for understanding the processes taking place in course of team work e.g.: in aviation. -
Step-By-Step Guide to Building Routes for Microsoft Train Simulator
Step-by-Step Guide to Building Routes for Microsoft Train Simulator By Michael Vone [email protected] Step-by-Step Guide to Building Routes for Microsoft Train Simulator Step-by-Step Guide To Building Routes Copyright © 2001, 2002 Michael Vone Copyright © 2001, 2002 Abacus Software, Inc 5130 Patterson St SE Grand Rapids, MI 49512 This book is copyrighted. No part of this book may be reproduced, stored in a retrieval system, or transmitted in any form or by any means, electronic, mechanical, photocopying, recording or otherwise without the prior written permission of Abacus Software. Every effort has been made to ensure complete and accurate information concerning the material presented in this book. However, Abacus Software can neither guarantee nor be held legally responsible for any mistakes in printing or faulty instructions contained in this book. The editors always appreciate receiving notice of any errors or misprints. This book contains trade names and trademarks of several companies. Any mention of these names or trademarks in this book are not intended to either convey endorsement or other associations with this book. Printed in the U.S.A. ISBN 1-55755-506-0 10 9 8 7 6 5 4 3 2nd Edition November 2002 2 Step-by-Step Guide to Building Routes for Microsoft Train Simulator Step-by-Step Guide To Building Routes Table of Contents Foreword How to Use this Guide Building "First Route": a project for the first-time user of the MSTS Editors and Tools 1. Planning a Route 1.1 Steps in designing and building a route 1.2 Designing a route 1.3 MSTS limitations 2. -
TS2020 – Class 481
TS2020 – Class 481 Typ Train Simulator 2020 - Addon Autor Benjamin Ebrecht Version 1.04 Datum 01.08.2020 Kontakt [email protected] Preface Dear Customer, thank you for your interest in our product “Die Baureihe 481”. With this Add-on you have purchased a very special realisation, as it is our very first to be based upon a vehicle model series made from scratch in the DTG Train Simulator. We have created a completely new, modular script- system to replicate the specific functions of the Berlin S-Bahn, following in an appropriately long time of development. The time was well spent: next to many very detailed characteristics like flickering lights and correctly calculated switching sparks, we also could implement entirely new systems such as an independent simulation of passengers for realistically packed trains and an extendable, fully functioning IBIS (Integrated-Board-Information-System) for the destination display and announcements. Another specialty is the fact that, in addition to the vehicle, we also managed to put a whole route into the package. Cheers to Jan Bleiß! He allowed us take the S25 part of his Add-on route “Berlin- Leipzig” and fit it to our needs for this package. Together with him, we look forward to see the fully finished S25 (to Henningsdorf); announced for 2020, with this route our vehicle will be able to fully play its potential… As usually, we have added some local colour to our scenarios and as a further addition the S-Bahn classes 475 (“Stadtbahner) and 480 (“Toaster”) are included in the package. Please note the extensive contents and complex vehicle functions of this manual. -
Printer Friendly
Sony PlayStation 2 Last Updated on October 2, 2021 Title Publisher Qty Box Man Comments .hack Vol. 1 & Vol. 2: PlayStation 2 the Best Namco Bandai Games .hack//Akushou Heni Vol. 2 Bandai .hack//frägment Bandai .hack//G.U. Vol. 1: Saitan Bandai Namco Games .hack//G.U. Vol. 1: Saitan: PlayStation 2 the Best Bandai Namco Games .hack//G.U. Vol. 2: Kimi Omou Koe Namco Bandai Games .hack//G.U. Vol. 2: Kimi Omou Koe: PlayStation 2 the Best Namco Bandai Games .hack//G.U. Vol. 3: Aruku Youna Hayasa de Namco Bandai Games .hack//G.U. Vol. 3: Aruku Youna Hayasa de : PlayStation 2 the Best Namco Bandai Games .hack//Kansen Kakudai Vol. 1 Bandai .hack//Shinshoku Osen Vol. 3 Bandai .hack//Vol. 3 X Vol. 4: PlayStation 2 the Best Namco Bandai Games .hack//Zettai Houi Vol. 4 Bandai 0 Story Enix 007: Everything or Nothing Electronic Arts 007: Everything or Nothing: EA Best Hits Electronic Arts 007: Nagusame no Houshuu Square Enix 007: Nightfire Electronic Arts 120-en no Haru: 120 Yen Stories Interchannel 18 Wheeler: American Pro Trucker Acclaim Japan 2002 FIFA World Cup Electronic Arts Victor 2006 FIFA World Cup EA Sports 2006 FIFA World Cup: FIFA Fussball-Weltmeisterschaft 2006 EA Sports 3-Nen B-Gumi Kinpachi Sensei: Densetsu no Kyoudan ni Tate! Chunsoft 3-Nen B-Gumi Kinpachi Sensei: Densetsu no Kyoudan ni Tate!: Perfect Edtion, Best Edition Chunsoft 3D Kakutou Tsukuru 2 Enterbrain 3LDK: Shiawase Ni Narouyo Princess Soft 3LDK: Shiawase Ni Narouyo: Limited Edition Princess Soft 7 Blades Konami 7 Blades: Konami the Best Konami A Ressha de Ikou 6 Artdink A.C.E. -
National Train Day Volunteers Needed
ink A Monthly Publication for and by Amtrak Employees Volume 19 • Issue 3 • April 2014 National Train Day Volunteers Needed Close Call Reporting Amtrak Business Lines Train of Thought his past March, we submitted our proposed we make of resources, both those we receive TFY 2015 budget to Congress. This year, we and those we generate. New Safe-2-Safer are requesting $1.62 billion in federal capital The FY 2015 budget will bring costs of our and operating support, an increase of approxi- other services (long-distance and state-sup- mately 16 percent over last year. ported) to light through this budgeting process. Milestone Our proposed budget redirects Both our state services and the operating profit obtained our long-distance trains are a from the Northeast Corridor valuable part of our nation’s February: (NEC) towards the planning and transportation system and I have 241,288 240,000 implementation of major multi- every reason to believe that January: year projects in the NEC such Congress will continue to provide 230,896 as replacing century old bridges the necessary funding to keep 220,000 and tunnels. NEC revenues have these services operating. It will recently exceeded operating costs be up to us then to manage them by more than $300 million a year. as efficiently as we can because Many don’t realize that much of our stakeholders, the state DOTs 200,000 2014 December: the revenue from the NEC is also and Congress expect nothing less 192,718 used to cover some of the costs of Joseph H. Boardman from us. -
PLATINUM EDITION Information
ISSUE No.1 ALL ABOUT SIMULATIONS WINTER 2011 £4.99 COVER STORY FARMING SIMULATOR 2011 GetPLATINUM the low-down on the upsEDITION and downs of farming! RAILWORKS 3 Train Simulator 2012 has arrived at a station near you! The ULTIMATE in train simulation never looked so realistic. Take a look inside and discover: Trucks & Trailers | Bus & Cable Car San Francisco London Underground | Developer interview 5 060020 475009 and more. WORLD OF SIMULATIONS - WINTER 2011 1 ADVERT Don your wellingtons and take to the farm in the ultimate edition of the iconic Farming Simulator, that really is the pick of the crop! www.excalibur-publishing.com PO Box 586 Banbury Oxfordshire OX16 6BY UK 2 WORLD OF SIMULATIONS - WINTER 2011 SPOTLIGHT 21 THOMAS LANGELOTZ …From TML studios, developers of London Underground. An in depth interview. NEWS 30 SIMULATION NEWS - Issue 1. Winter 2011 We’ve got the scoop on all of the juiciest CONTENTS sim happenings. FEATURES REVIEW 4 PLAYING WITH LIFE Dominic Sacco explains what this 44 REVIEW OF simulation malarky is all about. LATEST RELEASES Read reviews about some of the best 10 RAILWORKS 3 - simulation products currently on the market. TRAIN SIMULATOR 2012 The sequel to the award-winning BUYERS GUIDE Railworks 2 Train Simulator is arriving on a 46 BUYING INFORMATION platform near you. Check here for a fast update of what’s 14 FARMING SIMULATOR 2011 available and where to find more information. PLATINUM EDITION Astragon’s best seller goes green and SPECIFICATIONS gets the upgrade treatment. 35 CHECK THE SPECS! 36 BUS & CABLE CAR SIMULATOR - SAN FRANCISCO WELCOME!! No more queuing at dreary, damp European Party poppers are primed, bottle of bubbly is bus stops.