Human Olfaction: a View from the Top Stephen Pierzchajlo and Jonas K
Total Page:16
File Type:pdf, Size:1020Kb
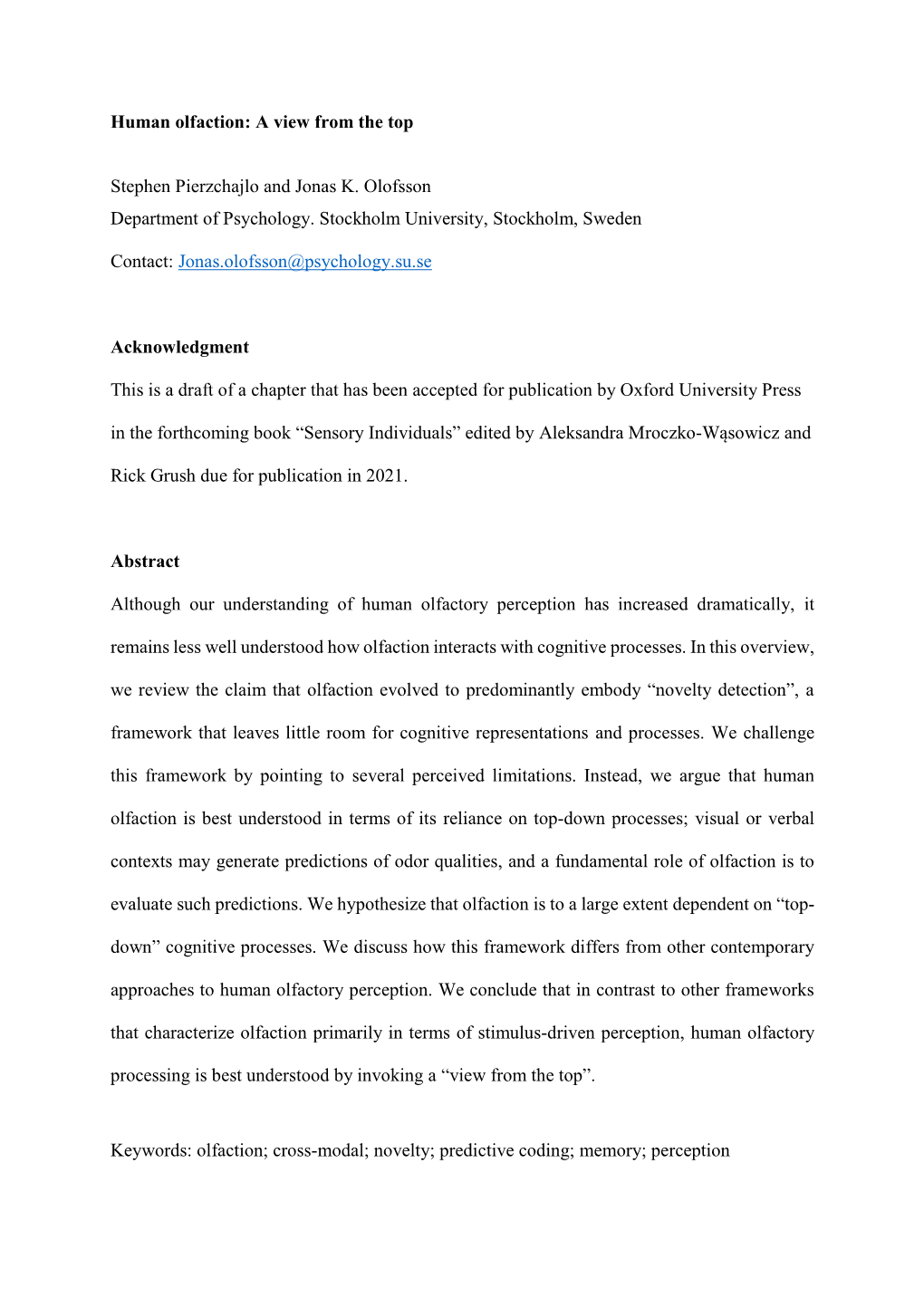
Load more
Recommended publications
-
Understanding Sensory Processing: Looking at Children's Behavior Through the Lens of Sensory Processing
Understanding Sensory Processing: Looking at Children’s Behavior Through the Lens of Sensory Processing Communities of Practice in Autism September 24, 2009 Charlottesville, VA Dianne Koontz Lowman, Ed.D. Early Childhood Coordinator Region 5 T/TAC James Madison University MSC 9002 Harrisonburg, VA 22807 [email protected] ______________________________________________________________________________ Dianne Koontz Lowman/[email protected]/2008 Page 1 Looking at Children’s Behavior Through the Lens of Sensory Processing Do you know a child like this? Travis is constantly moving, pushing, or chewing on things. The collar of his shirt and coat are always wet from chewing. When talking to people, he tends to push up against you. Or do you know another child? Sierra does not like to be hugged or kissed by anyone. She gets upset with other children bump up against her. She doesn’t like socks with a heel or toe seam or any tags on clothes. Why is Travis always chewing? Why doesn’t Sierra liked to be touched? Why do children react differently to things around them? These children have different ways of reacting to the things around them, to sensations. Over the years, different terms (such as sensory integration) have been used to describe how children deal with the information they receive through their senses. Currently, the term being used to describe children who have difficulty dealing with input from their senses is sensory processing disorder. _____________________________________________________________________ Sensory Processing Disorder -
Sensory Integration During Goal Directed Reaches: the Effects of Manipulating Target Availability
Sensory Integration During Goal Directed Reaches: The Effects of Manipulating Target Availability By Sajida Khanafer Thesis submitted to the Faculty of Graduate and Postgraduate Studies In partial fulfillment of the requirements for the degree of Master’s of Science in Human Kinetics School of Human Kinetics Health Sciences University of Ottawa Copyright © Sajida Khanafer, Ottawa, Canada, 2012 Abstract When using visual and proprioceptive information to plan a reach, it has been proposed that the brain combines these cues to estimate the object and/or limb’s location. Specifically, according to the maximum-likelihood estimation (MLE) model, more reliable sensory inputs are assigned a greater weight (Ernst & Banks, 2002). In this research we examined if the brain is able to adjust which sensory cue it weights the most. Specifically, we asked if the brain changes how it weights sensory information when the availability of a visual cue is manipulated. Twenty- four healthy subjects reached to visual (V), proprioceptive (P), or visual + proprioceptive (VP) targets under different visual delay conditions (e.g. on V and VP trials, the visual target was available for the entire reach, it was removed with the go-signal or it was removed 1, 2 or 5 seconds before the go-signal). Subjects completed 5 blocks of trials, with 90 trials per block. For 12 subjects, the visual delay was kept consistent within a block of trials, while for the other 12 subjects, different visual delays were intermixed within a block of trials. To establish which sensory cue subjects weighted the most, we compared endpoint positions achieved on V and P reaches to VP reaches. -
Making Choices While Smelling, Tasting, and Listening: the Role of Sensory (Dis)Similarity When Sequentially Sampling Products
Loyola University Chicago Loyola eCommons School of Business: Faculty Publications and Other Works Faculty Publications 1-2014 Making Choices While Smelling, Tasting, and Listening: The Role of Sensory (Dis)similarity When Sequentially Sampling Products Dipayan Biswas University of South Florida, [email protected] Lauren Labrecque Loyola University Chicago, [email protected] Donald R. Lehmann Columbia University, [email protected] Ereni Markos Suffolk University, [email protected] Follow this and additional works at: https://ecommons.luc.edu/business_facpubs Part of the Marketing Commons Recommended Citation Biswas, Dipayan; Labrecque, Lauren; Lehmann, Donald R.; and Markos, Ereni. Making Choices While Smelling, Tasting, and Listening: The Role of Sensory (Dis)similarity When Sequentially Sampling Products. Journal of Marketing, 78, : 112-126, 2014. Retrieved from Loyola eCommons, School of Business: Faculty Publications and Other Works, http://dx.doi.org/10.1509/jm.12.0325 This Article is brought to you for free and open access by the Faculty Publications at Loyola eCommons. It has been accepted for inclusion in School of Business: Faculty Publications and Other Works by an authorized administrator of Loyola eCommons. For more information, please contact [email protected]. This work is licensed under a Creative Commons Attribution-Noncommercial-No Derivative Works 3.0 License. © American Marketing Association, 2014 Dipayan Biswas, Lauren I. Labrecque, Donald R. Lehmann, & Ereni Markos Making Choices While Smelling, Tasting, and Listening: The Role of Sensory (Dis)similarity When Sequentially Sampling Products Marketers are increasingly allowing consumers to sample sensory-rich experiential products before making purchase decisions. The results of seven experimental studies (two conducted in field settings, three conducted in a laboratory, and two conducted online) demonstrate that the order in which consumers sample products and the level of (dis)similarity between the sensory cues of the products influence choices. -
The Visual System: Higher Visual Processing
The Visual System: Higher Visual Processing Primary visual cortex The primary visual cortex is located in the occipital cortex. It receives visual information exclusively from the contralateral hemifield, which is topographically represented and wherein the fovea is granted an extended representation. Like most cortical areas, primary visual cortex consists of six layers. It also contains, however, a prominent stripe of white matter in its layer 4 - the stripe of Gennari - consisting of the myelinated axons of the lateral geniculate nucleus neurons. For this reason, the primary visual cortex is also referred to as the striate cortex. The LGN projections to the primary visual cortex are segregated. The axons of the cells from the magnocellular layers terminate principally within sublamina 4Ca, and those from the parvocellular layers terminate principally within sublamina 4Cb. Ocular dominance columns The inputs from the two eyes also are segregated within layer 4 of primary visual cortex and form alternating ocular dominance columns. Alternating ocular dominance columns can be visualized with autoradiography after injecting radiolabeled amino acids into one eye that are transported transynaptically from the retina. Although the neurons in layer 4 are monocular, neurons in the other layers of the same column combine signals from the two eyes, but their activation has the same ocular preference. Bringing together the inputs from the two eyes at the level of the striate cortex provide a basis for stereopsis, the sensation of depth perception provided by binocular disparity, i.e., when an image falls on non-corresponding parts of the two retinas. Some neurons respond to disparities beyond the plane of fixation (far cells), while others respond to disparities in front of the plane of the fixation (near cells). -
Bayesian Models of Sensory Cue Integration
Bayesian models of sensory cue integration David C. Knill and Jeffrey A. Saunders 1 Center for Visual Sciences University of Rochester 274 Meliora Hall Rochester, NY 14627 Submitted to Vision Research 1E-mail address:[email protected] 1 Introduction Our senses provide a number of independent cues to the three-dimensional layout of objects and scenes. Vision, for example, contains cues from stereo, motion, texture, shading, etc.. Each of these cues provides uncertain information about a scene; however, this apparent ambiguity is mitigated by several factors. First, under normal conditions, multiple cues are available to an observer. By efficiently integrating information from all available cues, the brain can derive more accurate and robust estimates of three-dimensional geometry (i.e. positions, orientations, and shapes in three-dimensional space)[1]. Second, objects in our environment have strong statistical regularities that make cues more informative than would be the case in an unstructured environment. Prior knowledge of these regularities allows the brain to maximize its use of the information provided by sensory cues. Bayesian probability theory provides a normative framework for modeling how an observer should combine information from multiple cues and from prior knowledge about objects in the world to make perceptual inferences[2]. It also provides a framework for developing predictive theories of how human sensory systems make perceptual inferences about the world from sensory data, predictions that can be tested psychophysically. The goal of this chapter is to introduce the basic conceptual elements of Bayesian theories of perception and to illustrate a number of ways that we can use psychophysics to test predictions of Bayesian theories. -
Visual Stimulation Activities for Infants and Toddlers
National Institute for the Mentally Handicapped VISUAL STIMULATION ACTIVITIES FOR INFANTS AND TODDLERS A GUIDE TO PARENTS AND CAREGIVERS DR. AMAR Jyoihi PERShA Ms. K.R.NAWVi National Institute for the Mentally Handicapped (Ministryof Social Justice & Empowerment, Government of India) Manovikasnagar, Secunderabad - 500 009, Andhra Pradesh, INDIA. Grams : MANOVIKAS Phone : 040-27751741 Fax :040-27750198 E-mail : [email protected] Website : www.nimhindia.org VISUAL STIMULATION ACTIVITIES FOR INFANTS AND TODDLERS Authors : Dr. Amar Jyothi Persha, Ms. K.R. Nawvi Copyright 2004 National Institute for the Mentally Handicapped Secunderabad - 500 009. All rights Reserved. ISBN 81 89001 02 7 Designing Ramana Chepuri, Ramesh Chepuri, Ramaswamy, Secunderabad - 500 003. Ph : 040-55762484 Printed by : Sree Ramana Process Pvt. Ltd., Secunderabad - 500 003. Ph : 040-27811750 ,&ii1iti iiiificti icçiIii FP-1T1 (i1 1TfTftrr Icl1,'T NATIONALINSTITUTE FOR THE Dr.L. GOVINDA RAO MENTALLYHANDICAPPED irector (Ministry of Social Justice and Empowerment, Government of India) FOREWORD This book is an outcome of the project titled "Development of stimulation activities for visually impaired infants and toddlers". Studies show that there are six lakh children born with visual problem each year, among them almost 80% have a residual visual capacity. The visual system gives us a variety of information about the environment, which is necessary for learning and acquiring skills for daily living. Vision is the sense that reveals the mystery of the world to the child. The eyes are the outgrowth of the brain and they parallel the development and growth of the brain in the first few months of life. Visual system matures rapidly as the brain does in these early years of life. -
Olfaction and Emotion Content Effects for Memory of Vignettes
Current Research in Psychology 1 (1): 53-60, 2010 ISSN 1949-0178 © 2010 Science Publications Olfaction and Emotion Content Effects for Memory of Vignettes 1Jeremy W. Grabbe, 2Ann L. McCarthy, 3Charissa M. Brown and 3Arlene A. Sabo 1Department of Psychology, Plattsburgh State University, SUNY Plattsburgh, 101 Broad Street, Plattsburgh, NY 12901 2Department of Psychology, The University of Akron, OH, USA 3Department of Psychology, Plattsburgh State University, NY, USA Abstract: Problem statement: Recent research has shown a relationship between olfaction and episodic/autobiographical memory. The mnemonic theory of odor asserts that odor representation and storage is tied to memory. The Proust phenomenon suggests that specifically episodic memory is the memory component behind the mnemonic theory of olfaction. Neurological evidence demonstrates that neural structures related to emotion have connections between olfactory receptors and the episodic memory center. Approach: This study examined the role of emotion and olfaction in memory for vignettes. Participants were presented with a series of vignettes that varied by emotional content. Olfactory cues were paired with vignettes. Participants were questioned over recall of the vignettes. Results: Two experiments demonstrated a significant effect for emotion in memory performance for vignettes. The role of olfaction was not as prominent. Conclusion/Recommendations: This confirms the Proust phenomenon olfaction, namely that olfaction plays a greater role in autobiographical memory than memory for vignettes. The generalization of the Proust phenomenon to nonautobiographical memory is not supported by the results of these two studies. The authors suggest future research examining the interaction between olfaction and emotion should be directed towards autobiographical memory. Key words: Olfaction, nonautobiographical memory, sensory cues INTRODUCTION recognition memory test and the Toyota and Takagi olfactometer. -
Olfaction Modulates Ambiguous Visual Motion Perception
OPEN Smelling directions: Olfaction modulates SUBJECT AREAS: ambiguous visual motion perception HUMAN BEHAVIOUR Shenbing Kuang & Tao Zhang PERCEPTION MOTION State Key Laboratory of Brain and Cognitive Science, Institute of Psychology, Chinese Academy of Sciences, Beijing 100101, China. OLFACTORY CORTEX Senses of smells are often accompanied by simultaneous visual sensations. Previous studies have Received documented enhanced olfactory performance with concurrent presence of congruent color- or shape- 27 February 2014 related visual cues, and facilitated visual object perception when congruent smells are simultaneously present. These visual object-olfaction interactions suggest the existences of couplings between the olfactory Accepted pathway and the visual ventral processing stream. However, it is not known if olfaction can modulate visual 3 July 2014 motion perception, a function that is related to the visual dorsal stream. We tested this possibility by examining the influence of olfactory cues on the perceptions of ambiguous visual motion signals. We Published showed that, after introducing an association between motion directions and olfactory cues, olfaction could 23 July 2014 indeed bias ambiguous visual motion perceptions. Our result that olfaction modulates visual motion processing adds to the current knowledge of cross-modal interactions and implies a possible functional linkage between the olfactory system and the visual dorsal pathway. Correspondence and requests for materials n our daily life, we are constantly exposed to multiple sensory inputs from different sensory modalities of should be addressed to varying reliability. Yet we could effortless integrate these parallel sensory signals and maintain a unified T.Z. (taozhang@psych. I perception that allows us to interact with the external world. -
Anatomy and Physiology of the Afferent Visual System
Handbook of Clinical Neurology, Vol. 102 (3rd series) Neuro-ophthalmology C. Kennard and R.J. Leigh, Editors # 2011 Elsevier B.V. All rights reserved Chapter 1 Anatomy and physiology of the afferent visual system SASHANK PRASAD 1* AND STEVEN L. GALETTA 2 1Division of Neuro-ophthalmology, Department of Neurology, Brigham and Womens Hospital, Harvard Medical School, Boston, MA, USA 2Neuro-ophthalmology Division, Department of Neurology, Hospital of the University of Pennsylvania, Philadelphia, PA, USA INTRODUCTION light without distortion (Maurice, 1970). The tear–air interface and cornea contribute more to the focusing Visual processing poses an enormous computational of light than the lens does; unlike the lens, however, the challenge for the brain, which has evolved highly focusing power of the cornea is fixed. The ciliary mus- organized and efficient neural systems to meet these cles dynamically adjust the shape of the lens in order demands. In primates, approximately 55% of the cortex to focus light optimally from varying distances upon is specialized for visual processing (compared to 3% for the retina (accommodation). The total amount of light auditory processing and 11% for somatosensory pro- reaching the retina is controlled by regulation of the cessing) (Felleman and Van Essen, 1991). Over the past pupil aperture. Ultimately, the visual image becomes several decades there has been an explosion in scientific projected upside-down and backwards on to the retina understanding of these complex pathways and net- (Fishman, 1973). works. Detailed knowledge of the anatomy of the visual The majority of the blood supply to structures of the system, in combination with skilled examination, allows eye arrives via the ophthalmic artery, which is the first precise localization of neuropathological processes. -
Rapid Evolution of the Visual System: a Cellular Assay of the Retina and Dorsal Lateral Geniculate Nucleus of the Spanish Wildcat and the Domestic Cat
The Journal of Neuroscience, January 1993, 13(l): 208-229 Rapid Evolution of the Visual System: A Cellular Assay of the Retina and Dorsal Lateral Geniculate Nucleus of the Spanish Wildcat and the Domestic Cat Robert W. Williams,’ Carmen Cavada,2 and Fernando Reinoso-Suhrez* ‘Department of Anatomy and Neurobiology, College of Medicine, University of Tennessee, Memphis, Tennessee 38163 and *Departamento de Morfologia, Facultad de Medicina, Universidad Aut6noma de Madrid, 28029 Madrid, Spain The large Spanish wildcat, Fe/is silvestris tartessia, has re- and important topic, it has been difficult to study the process tained features of the Pleistocene ancestor of the modern of brain evolution in any detail. Our approach has been to domestic cat, F. catus. To gauge the direction and magnitude identify a pair of closely related living species,one from a highly of short-term evolutionary change in this lineage, we have conservative branch that has retained near identity with the compared the retina, the optic nerve, and the dorsal lateral ancestral species,and the other from a derived branch that has geniculate nucleus (LGN) of Spanish wildcats and their do- undergone rapid evolutionary change. The recent recognition mestic relatives. Retinas of the two species have the same that evolution and speciationcan occur in short bursts separated area. However, densities of cone photoreceptors are higher by long interludes of stasisprovides a sound theoretical basis in wildcat-over 100% higher in the area centralis-where- for a search for such pairs (Schindewolf, 1950; Eldredge and as rod densities are as high, or higher, in the domestic lin- Gould, 1972; Stanley, 1979; Gould and Eldredge, 1986). -
Organizing Probabilistic Models of Perception
Review Organizing probabilistic models of perception Wei Ji Ma Department of Neuroscience, Baylor College of Medicine, 1 Baylor Plaza, Houston TX 77030, USA Probability has played a central role in models of per- above, over target presence, landing location, and life span. ception for more than a century, but a look at probabi- Since this knowledge is based on sensory observations, the listic concepts in the literature raises many questions. Is probability distribution is a conditional distribution, which being Bayesian the same as being optimal? Are recent can be denoted by q(world state j observations). Bayesian models fundamentally different from classic Knowledge is not sufficient for organisms; actions are signal detection theory models? Do findings of near- needed. The wildebeest might decide whether to stay put, optimal inference provide evidence that neurons com- the badminton player whether to attempt a return, and the pute with probability distributions? This review aims to actuary what premium to set. Cost or utility is associated disentangle these concepts and to classify empirical with each combination of true world state and action, evidence accordingly. denoted by C(world state, action): if the badminton player does not attempt to return the shuttle, energy is saved, but Decision-making in an uncertain world at the cost of a point if the shuttle lands inside the court. For In order to survive and thrive, all animals must derive the observer, the expected cost of an action is a weighted knowledge about the world from sensory observations. A average over world states, with weights given by the proba- wildebeest needs to know whether a predator is hiding in the bilities of those world states given the observations: high grass, a badminton player where the shuttlecock will X EC C ; land, and an actuary how long a life insurance buyer will ðactionÞ ¼ ðworld state actionÞ world state live. -
Mapping out the Olfactory Design Space for Novel Interactions
1 SMELL SPACE: Mapping out the Olfactory Design Space for 2 Novel Interactions 3 4 SCHI Lab, School of Engineering and Informatics, University of Sussex, UK 5 EMANUELA MAGGIONI, 6 ROBERT COBDEN, SCHI Lab, School of Engineering and Informatics, University of Sussex, UK 7 DMITRIJS DMITRENKO, SCHI Lab, School of Engineering and Informatics, University of Sussex, UK 8 KASPER HORNBÆK, Department of Computer Science, University of Copenhagen, Denmark 9 MARIANNA OBRIST, SCHI Lab, School of Engineering and Informatics, University of Sussex, UK 10 The human sense of smell is powerful. However, the way we use smell as interaction modality in HCI is 11 limited. We lack a common reference point to guide designers’ choices when using smell. Here, we map out 12 an olfactory design space to provide designers with such guidance. We identified four key design features: (i) 13 chemical, (ii) emotional, (iii) spatial, and (iv) temporal. Each feature defines a building block for smell-based 14 interaction design and is grounded in a review of the relevant scientific literature. We then demonstrate 15 the design opportunities in three application cases. Each application (i.e. one desktop, two virtual reality 16 implementations) highlights the design choices alongside the implementation and evaluation possibilities in 17 using smell. We conclude by discussing how identifying those design features facilitates a healthy growth of 18 this research domain and contributes to an intermediate-level knowledge space. Finally, we discuss further 19 challenges the HCI community needs to tackle. 20 CCS Concepts: • Human-centered computing → Interaction techniques; Empirical studies in HCI. 21 Additional Key Words and Phrases: Smell; Olfactory Design Space; Chemical Sense; Scent-based Interaction 22 Design; Odour Interfaces; Smell-based Applications; Novel Interactions 23 ACM Reference Format: 24 Emanuela Maggioni, Robert Cobden, Dmitrijs Dmitrenko, Kasper Hornbæk, and Marianna Obrist.