Retrievals of the Deep Convective System Ice Cloud Microphysical Properties Using the Arm Radar and Aircraft In-Situ Measurements
Total Page:16
File Type:pdf, Size:1020Kb
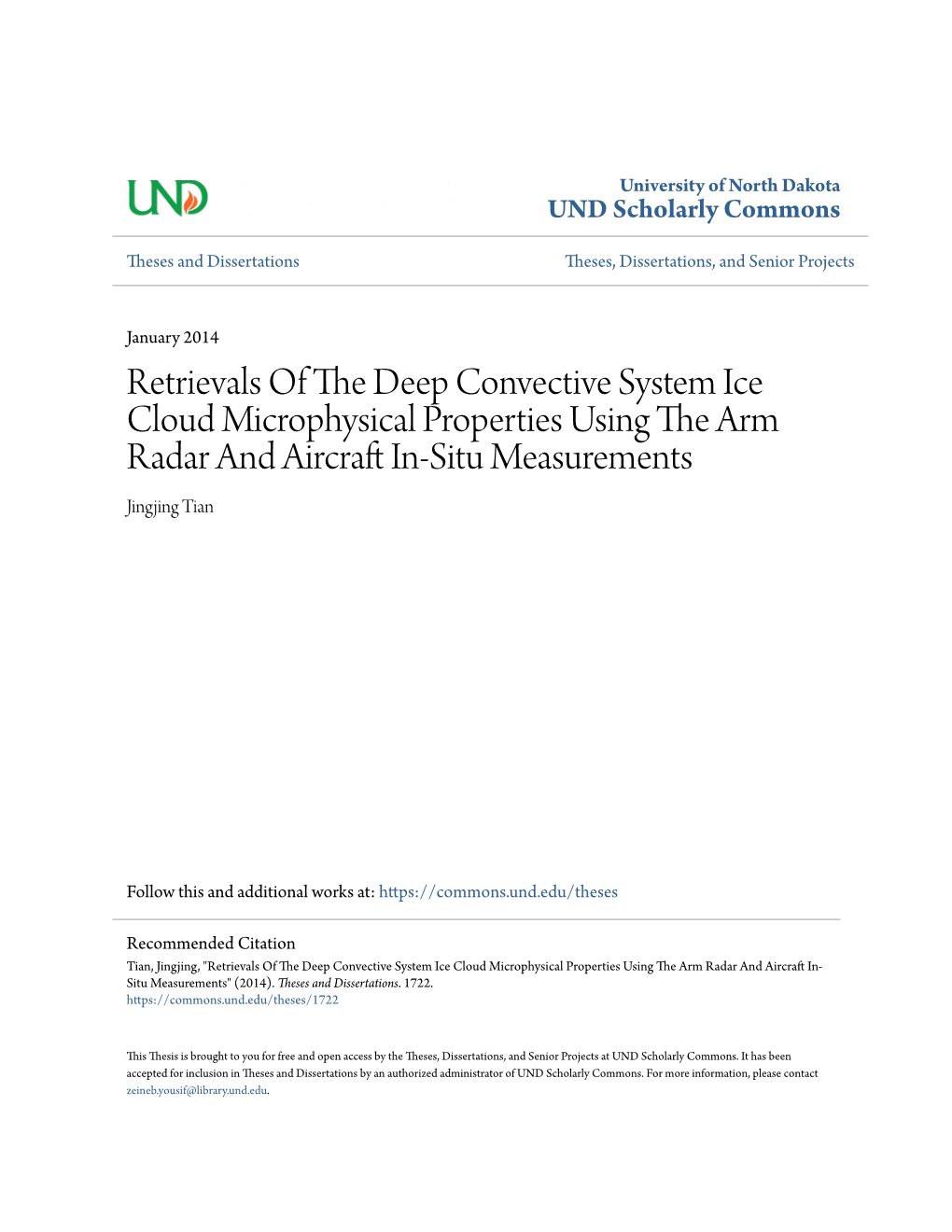
Load more
Recommended publications
-
P4b.7 Mixed-Phase Cloud Retrievals Using Doppler Radar Spectra
P4B.7 MIXED-PHASE CLOUD RETRIEVALS USING DOPPLER RADAR SPECTRA Matthew D. Shupe* NOAA Environmental Technology Laboratory, Boulder, CO 80305 Pavlos Kollias University of Miami, Miami, FL Sergey Y. Matrosov and Timothy L. Schneider NOAA Environmental Technology Laboratory, Boulder, CO 1. INTRODUCTION 2. MEASUREMENTS The radar Doppler spectrum contains a wealth of All measurements discussed here were made by information on cloud microphysical properties. Typically, ground-based remote-sensors at the CRYSTAL-FACE radar-based cloud retrievals use only the zeroth or first eastern ground site located in Miami, Florida (25° 39' N, 80° moments of the Doppler spectrum – reflectivity and mean 26' W) on 29 July, 2002. Measurements of the radar Doppler velocity – to derive quantities such as cloud water Doppler spectrum were made by a vertically-pointing, 35- content and particle characteristic size (e.g., Liou and GHz, millimeter cloud radar (MMCR). The MMCR has 45 m Sassen 1994; Matrosov et al. 2002). When using only the vertical resolution and resolves the Doppler spectrum over moments of the Doppler spectrum, important spectral the range of -4.1 to +4.1 m s -1 to 0.064 m s -1 using 128 fast information can be lost, particularly when the spectrum is Fourier transform points. We utilize one of four alternating multi-modal. Multi-modal spectra are possible when a operational modes that samples for 1.8 seconds at 35- mixture of two or more cloud particle phases, habits, or second intervals. Radar reflectivities for the half-hour long, sizes exist in the same volume (e.g., Gossard et al. -
Use of Cloud Radar Observations for Model Evaluation: a Probabilistic Approach Christian Jakob Bureau of Meteorology Research Centre, Melbourne, Australia
JOURNAL OF GEOPHYSICAL RESEARCH, VOL. 109, D03202, doi:10.1029/2003JD003473, 2004 Use of cloud radar observations for model evaluation: A probabilistic approach Christian Jakob Bureau of Meteorology Research Centre, Melbourne, Australia Robert Pincus and Ce´cile Hannay Climate Diagnostics Center, National Oceanic and Atmospheric Administration Cooperative Institute for Research in Environmental Sciences, Boulder, Colorado, USA Kuan-Man Xu NASA Langley Research Center, Hampton, Virginia, USA Received 30 January 2003; revised 21 October 2003; accepted 17 November 2003; published 5 February 2004. [1] The use of narrow-beam, ground-based active remote sensors (such as cloud radars and lidars) for long-term observations provides valuable new measurements of the vertical structure of cloud fields. These observations might be quite valuable as tests for numerical simulations, but the vastly different spatial and temporal scales of the observations and simulation must first be reconciled. Typically, the observations are averaged over time and those averages are claimed to be representative of a given model spatial scale, though the equivalence of temporal and spatial averages is known to be quite tenuous. This paper explores an alternative method of model evaluation based on the interpretation of model cloud predictions as probabilistic forecasts at the observation point. This approach requires no assumptions about statistical stationarity and allows the use of an existing, well- developed suite of analytic tools. Time-averaging and probabilistic evaluation techniques are contrasted, and their performance is explored using a set of ‘‘perfect’’ forecasts and observations extracted from a long cloud system model simulation of continental convection. This idealized example demonstrates that simple time averaging always obscures forecast skill regardless of model domain size. -
Cloud Classification and Distribution of Cloud Types in Beijing Using Ka
ADVANCES IN ATMOSPHERIC SCIENCES, VOL. 36, AUGUST 2019, 793–803 • Original Paper • Cloud Classification and Distribution of Cloud Types in Beijing Using Ka-Band Radar Data Juan HUO∗, Yongheng BI, Daren LU,¨ and Shu DUAN Key Laboratory for Atmosphere and Global Environment Observation, Chinese Academy of Sciences, Beijing, China 100029 (Received 27 December 2018; revised 15 April 2019; accepted 23 April 2019) ABSTRACT A cloud clustering and classification algorithm is developed for a ground-based Ka-band radar system in the vertically pointing mode. Cloud profiles are grouped based on the combination of a time–height clustering method and the k-means clustering method. The cloud classification algorithm, developed using a fuzzy logic method, uses nine physical parameters to classify clouds into nine types: cirrostratus, cirrocumulus, altocumulus, altostratus, stratus, stratocumulus, nimbostratus, cumulus or cumulonimbus. The performance of the clustering and classification algorithm is presented by comparison with all-sky images taken from January to June 2014. Overall, 92% of the cloud profiles are clustered successfully and the agree- ment in classification between the radar system and the all-sky imager is 87%. The distribution of cloud types in Beijing from January 2014 to December 2017 is studied based on the clustering and classification algorithm. The statistics show that cirrostratus clouds have the highest occurrence frequency (24%) among the nine cloud types. High-level clouds have the maximum occurrence frequency and low-level clouds the minimum occurrence frequency. Key words: clouds, clustering algorithm, classification algorithm, radar, cloud type Citation: Huo, J., Y. H. Bi, D. R. Lu,¨ and S. Duan, 2019: Cloud classification and distribution of cloud types in Beijing using Ka-band radar data. -
The Tropical Warm Pool International Cloud Experiment
THE TROPICAL WARM POOL INTERNATIONAL CLOUD EXPERIMENT BY PETER T. MAY, JAMES H. MATHER, GERAINT VAUGHAN, CHRISTIAN JAKOB, GREG M. MCFARQUHAR, KEITH N. BOWER, AND GERALD G. MACE A comprehensive observing campaign in Darwin, including a ship, five aircraft, and frequent soundings, is furthering weather and climate change studies through improved understanding and modeling of cloud and aerosol processes in tropical cloud systems. crucial factor in our ability to forecast future of this region and through it the global stratosphere climate change is a better representation (e.g., Russell et al. 1993; Folkins 2002). Understanding A of tropical cloud systems, and their heating and the transport of gases and particles in deep convec- radiative impacts in climate models (Stephens 2005; tion is also therefore of global importance. Del Genio and Kovari 2002; Neale and Slingo 2003; As a response to this challenge, a major field Spencer and Slingo 2003). This requires a better experiment named the Tropical Warm Pool Interna- understanding of the factors that control tropical tional Cloud Experiment (TWP-ICE), including the convection as well as an improved understanding of U.K. Aerosol and Chemical Transport in Tropical how the characteristics of the convection affect the Convection (ACTIVE) consortium, was undertaken nature of the cloud particles that are found in deep in the Darwin, Northern Australia, area in January convective anvils and tropical cirrus. Deep convec- and February 2006. The experiment was designed tion also lifts boundary layer air into the tropical to provide a comprehensive dataset for the develop- tropopause layer (TTL), affecting the composition ment of cloud remote-sensing retrievals and initial- AFFILIATIONS: MAY—Centre for Australian Weather and CORRESPONDING AUTHOR: Peter T. -
ICECAPS: New Observations of Clouds, Atmosphere, and Precipitation at Summit, Greenland Matthew Shupe David Turner Von Walden Ralf Bennartz with Contributions From: M
ICECAPS: New Observations of Clouds, Atmosphere, and Precipitation at Summit, Greenland Matthew Shupe David Turner Von Walden Ralf Bennartz With contributions from: M. Cadeddu, B. Castellani, CIRES, Univ. of Colorado & NOAA/ESRL NOAA/NSSL University of Idaho University of Wisconsin - Madison C. Cox, D. Hudak, M. Kulie, N. Miller, R. Neely, W. Neff Instrument Suite Operating at Summit Tropopause variability Atmospheric Structure As part of the NSF Arctic Observing Network, the Integrated Characterization of Energy, Clouds, Atmospheric state, and Precipitation at Summit (ICECAPS) project is providing a first look at detailed atmosphere and cloud properties over the central Greenland Ice Sheet. These observations are an important The ICECAPS twice-daily radiosonde program provides an contribution towards the IASOA network of Arctic atmospheric observatories, and offer exciting new perspectives on this unique environment. unprecedented perspective on atmospheric structure above the Instruments Measurements Geophysical Parameters Possible min T record central Greenland Ice Sheet. Millimeter Cloud Radar (MMCR) 35-GHz Doppler spectra and moments Cloud phase, microphysics, dynamics MicroPulse Lidar (MPL) 532 nm Backscatter, quasi-circular depol ratio Cloud phase, microphysics, optics • Periodic, warm, moist, and cloudy air masses advect onto the ice Cloud-Aerosol Polarization & Backscatter Lidar (CAPABL) 523 nm Backscatter, linear depol radio, diattenuation Cloud phase, microphysics, optics Warm, moist pulses sheet. There is a clear distinction between air masses containing Sonic Detection and Ranging System (SODAR) 2.1 kHz Reflectivity Boundary layer depth clouds and those without. • A temperature inversion has occurred in every ICECAPS sounding Precipitation Occurrence Sensor System (POSS) 10.5 GHz Reflectivity Snowfall rate except one. Humidity and Temp. -
12A1 the Tropical Warm Pool International Cloud Experiment (TWP-ICE)
12A1 The Tropical Warm Pool International Cloud Experiment (TWP-ICE) Peter T. May1, James H. Mather2, Geraint Vaughan3, Christian Jakob1, Greg M. McFarquhar4, Keith N. Bower3, and Gerald G. Mace5 1 Bureau of Meteorology Research Centre, Melbourne, Australia 2. Pacific Northwest National Laboratory, Richland, Washington, USA 3. School of Earth, Atmospheric and Environmental Sciences, University of Manchester, UK 4. Department of Atmospheric Sciences, University of Illinois, Illinois, USA 5. Meteorology Department, University of Utah, Utah, USA physical and chemical properties of cirrus and 1. Introduction the upper troposphere region. A crucial factor for our ability to forecast future The experiment was designed to provide a climate change is a better representation of comprehensive data set for the development of tropical convection and clouds in climate cloud remote sensing retrievals and initialising models. This requires a better understanding of and verifying a range of process-scale models, the multi-scale factors that control tropical with a view to improving the parameterisation convection as well as an improved of tropical convection and clouds in numerical understanding of how the characteristics of the weather prediction and climate models. convection affect the density and nature of the Accordingly, a surface measurement network cloud particles that are found in deep (including a research ship) of unprecedented convective anvils and tropical cirrus. Deep coverage for a tropical experiment was convection also lifts boundary-layer air into the combined with a fleet of five research aircraft to Tropical Tropopause Layer (TTL), affecting the enable in-situ and remote-sensing composition of this region and through it the measurements of clouds, meteorological global stratosphere. -
A Method to Merge WSR-88D Data with ARM SGP Millimeter Cloud Radar Data by Studying Deep Convective Systems
958 JOURNAL OF ATMOSPHERIC AND OCEANIC TECHNOLOGY VOLUME 26 A Method to Merge WSR-88D Data with ARM SGP Millimeter Cloud Radar Data by Studying Deep Convective Systems ZHE FENG,XIQUAN DONG, AND BAIKE XI Department of Atmospheric Sciences, University of North Dakota, Grand Forks, North Dakota (Manuscript received 18 June 2008, in final form 8 December 2008) ABSTRACT A decade of collocated Atmospheric Radiation Measurement Program (ARM) 35-GHz Millimeter Cloud Radar (MMCR) and Weather Surveillance Radar-1988 Doppler (WSR-88D) data over the ARM Southern Great Plains (SGP) site have been collected during the period of 1997–2006. A total of 28 winter and 45 summer deep convective system (DCS) cases over the ARM SGP site have been selected for this study during the 10-yr period. For the winter cases, the MMCR reflectivity, on average, is only 0.2 dB lower than that of the WSR-88D, with a correlation coefficient of 0.85. This result indicates that the MMCR signals have not been attenuated for ice-phase convective clouds, and the MMCR reflectivity measurements agree well with the WSR-88D, regardless of their vastly different characteristics. For the summer nonprecipitating convective clouds, however, the MMCR reflectivity, on average, is 10.6 dB lower than the WSR-88D mea- surement, and the average differences between the two radar reflectivities are nearly constant with height above cloud base. Three lookup tables with Mie calculations have been generated for correcting the MMCR signal attenuation. After applying attenuation correction for the MMCR reflectivity measurements, the averaged difference between the two radars has been reduced to 9.1 dB. -
Amf2 Facility Overview
AMF2 FACILITY OVERVIEW The following slides illustrate the basic AMF2 configuration. All systems are configurable and the foot print can be adjusted to meet the particular requirements of a deployment such as locating on ships. The modules can be distributed remotely from other AMF2 systems, taking advantage of limited space or complex configurations. Instrumentation in the OPS van is somewhat fixed but some subsystems can be removed and run independently if needed. Power requirements are only estimates and they will vary depending on the cooling and/or heating requirements of a deployment. Most systems are designed to accept 120 up to 480 VAC input power. For additional information please contact: Brad Orr Mike Ritsche (Manager) (Technical Operations Manager) 630-252-8665 (w) 630-252-1554 (w) 630-485-8004 (c) 630-777-1169 (c) [email protected] [email protected] AMF2 OPS Van Typical instrument configuration •MPL •HSRL •ASSIST II •Wind Profiler •MWR •Power Requirements: 10 KVA AMF2 General Purpose Van Typical instrument configuration •Radiosonde launch support •Ceilometer, general surface support •Guest instrument support •Instrument spare and tools •Power requirements: 10 kW Modules • 120 VAC Operation • 10-20 amps per module • Wireless communication Aerosol Observing System Current Instrument List • Aerosol Inlet/Manifold • DMT CCN-200 • Radiance PSAP • TSI Nephelometer I • TSI Nephelometer II • TSI CNC 3772 • Humidigraph • TEI O3 Analyzer • HTDMA Power requirement: 10 KVA ARM X/Ka-band Scanning Cloud radar The AMF2 radar is similar to the one above but it will have two large antennae instead of one large and one small as shown. Estimated Power requirement: 10 KVA Active Sensors Micropulse Lidar (MPL) Nd:YAG laser, 1.0 watt IR CW (30 mW pulses), 523 um, 8” telescope, eye safe Ka-band Zenith Pointing Radar (KAZR) Ka-band: 34.83–34.89 GHz, 200 W peak power High Spectral Resolution Lidar (HSRL) Nd:YAG laser, 0.6 W average power, 532 nm, 15” telescope, eye safe Radar Wind Profiler (RWP) Foreign deployments: 1283-1290 MHz, 1000 W peak power U.S. -
Validation of a Radiosonde-Based Cloud Layer Detection Method
JOURNAL OF GEOPHYSICAL RESEARCH: ATMOSPHERES, VOL. 118, 846–858, doi:10.1029/2012JD018515, 2013 Validation of a radiosonde-based cloud layer detection method against a ground-based remote sensing method at multiple ARM sites Jinqiang Zhang,1,2 Zhanqing Li,1,2,3 Hongbin Chen,1 and Maureen Cribb2 Received 19 July 2012; revised 18 December 2012; accepted 5 October 2012; published 31 January 2013. [1] Cloud vertical structure is a key quantity in meteorological and climate studies, but it is also among the most difficult quantities to observe. In this study, we develop a long-term (10 years) radiosonde-based cloud profile product for the U.S. Department of Energy’s Atmospheric Radiation Measurement (ARM) program Southern Great Plains (SGP), Tropical Western Pacific (TWP), and North Slope of Alaska (NSA) sites and a shorter-term product for the ARM Mobile Facility (AMF) deployed in Shouxian, Anhui Province, China (AMF-China). The AMF-China site was in operation from 14 May to 28 December 2008; the ARM sites have been collecting data for over 15 years. The Active Remote Sensing of Cloud (ARSCL) value-added product (VAP), which combines data from the 95-GHz W-band ARM Cloud Radar (WACR) and/or the 35-GHz Millimeter Microwave Cloud Radar (MMCR), is used in this study to validate the radiosonde-based cloud layer retrieval method. The performance of the radiosonde-based cloud layer retrieval method applied to data from different climate regimes is evaluated. Overall, cloud layers derived from the ARSCL VAP and radiosonde data agree very well at the SGP and AMF-China sites. -
Identification and Analysis of Atmospheric States and Associated Cloud Properties for Darwin, Australia S
JOURNAL OF GEOPHYSICAL RESEARCH, VOL. 117, D06204, doi:10.1029/2011JD017010, 2012 Identification and analysis of atmospheric states and associated cloud properties for Darwin, Australia S. M. Evans,1,2 R. T. Marchand,1,2 T. P. Ackerman,1,2 and N. Beagley3 Received 13 October 2011; revised 18 January 2012; accepted 30 January 2012; published 21 March 2012. [1] An iterative automated classification technique that combines European Centre for Medium-Range Weather Forecasts analysis data and vertically pointing millimeter wavelength cloud radar observations is used to identify commonly occurring atmospheric patterns or states around Darwin, Australia. The technique defines the atmospheric states by large-scale, synoptic variables such that, once defined, these states will be suitable to composite climate model output. Radar observations of clouds are used to test the statistical significance of each state and prompt the automated refinement of the states until each state produces a statistically stable and unique hydrometeor occurrence profile. The technique identifies eight atmospheric states: two monsoon states, two transition season states, and four dry season states. The two monsoon states can be identified as the active monsoon and the break monsoon. Among the dry season states, periods of isolated and suppressed convection can be identified. We use these states as the basis for compositing hydrometeor occurrence, precipitation rate, outgoing longwave radiation, and Madden-Julian Oscillation phase to further understand the meteorology of each state. Citation: Evans, S. M., R. T. Marchand, T. P. Ackerman, and N. Beagley (2012), Identification and analysis of atmospheric states and associated cloud properties for Darwin, Australia, J. -
On the Retrieval of Effective Radius with Cloud Radarsmarc…
On the Retrieval of Effective Radius with Cloud Radars Shelby Frisch1, Matthew Shupe2, Irina Djalalova2, Graham Feingold3, and Mike Poellot4 Abstract. In-situ sampling of cloud droplets by aircraft in Oklahoma in 1997 , SHEBA – 1FIREACE in 1998 and from a collection of droplet spectra measured from various locations around the world are used to evaluate the potential for a ground-based remote-sensing technique for retrieving profiles of cloud droplet effective radius. The technique uses vertically-pointing measurements from a high-sensitivity millimeter- wavelength radar to obtain height-resolved estimates of the effective radius in clouds. Although most meteorological radars lack the sensitivity to detect small cloud droplets, millimeter-wavelength Acloud@ radars provide opportunities for remotely monitoring the properties of non-precipitating clouds. These high-sensitivity radars can reveal detailed reflectivity and velocity structure of most clouds within several kilometers range. In order to put these reflectivity ad velocity measurements into usable microphysical quantities, relationships between the measured quantities and the desired quantities must be developed. This can be done through theoretical analysis, modeling, or empirical measurements. Then the problem is determining the uncertainty of each procedure in order to know which to use. A number of procedures have been developed recently to estimate the microphysical features of clouds from millimeter-wave radar observations. In this article we restrict our attention to liquid-water clouds; retrievals for ice clouds are described in other studies (e.g., Matrosov [1997]). Approaches for retrieving cloud radar reflectivity and ice and liquid water content were suggested by Liao and Sassen, 1994, which was expanded on and validated by Sassen et al, 1999 . -
High Resolution Modeling of Cloud Fields at the TWP Site with MM5
Tenth ARM Science Team Meeting Proceedings, San Antonio, Texas, March 13-17, 2000 High Resolution Modeling of Cloud Fields at the TWP Site with MM5 K. Wyser, C. Gautier, C. Jones, and W. O'Hirok Institute for Computational Earth System Science University of California, Santa Barbara Santa Barbara, California Introduction The advent of powerful computers has enabled atmospheric scientists to run weather forecast and climate models at ever increasing resolutions. Of particular interest are limited area models with high temporal and spatial resolution that can be run affordably on workstations. These models, initialized and forced at the boundaries with data from large-scale models with coarse resolution, resolve fine-scale features in the interior, a process generally known as dynamical downscaling (e.g., Murphy 1999). However, the success of a high-resolution simulation depends on a number of factors such as the coupling at the boundaries or the representation of physical processes. These processes are treated differently at different resolutions; convection, for example, is parameterized at coarse resolution but cumulus clouds are explicitly resolved at finer scales. Furthermore, the values of model parameters may be optimal at certain scales but inappropriate at others. The selection of the right combination of parameterizations is therefore a difficult task in complex numerical models of the atmosphere. Our main objective in this paper is to study the capabilities of the Pennsylvania State University (PSU)/National Center for Atmospheric Research (NCAR) mesoscale model (MM5) for regional climate simulations. We are also interested in the generation of realistic three-dimensional (3-D) distributions of clouds for separate studies with a 3-D radiative transfer model.