Analysis of Somatic Copy Number Gains in Pancreatic Ductal Adenocarcinoma Implicates ECT2 As a Candidate Therapeutic Target
Total Page:16
File Type:pdf, Size:1020Kb
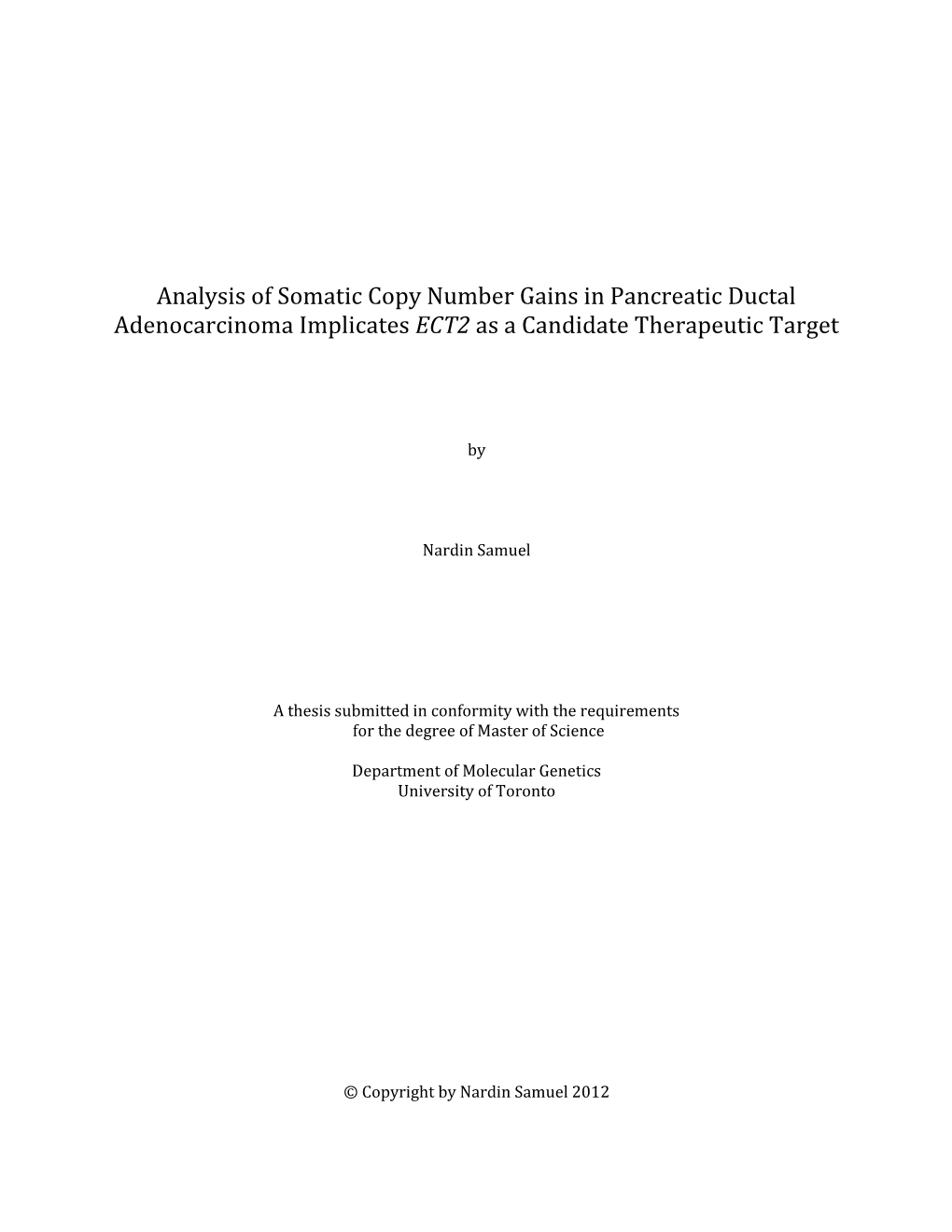
Load more
Recommended publications
-
Cytoplasmic Expression of Epithelial Cell Transforming Sequence 2 in Lung Adenocarcinoma and Its Implications for Malignant Progression
Laboratory Investigation (2019) 99:551–567 https://doi.org/10.1038/s41374-018-0142-4 ARTICLE Cytoplasmic expression of epithelial cell transforming sequence 2 in lung adenocarcinoma and its implications for malignant progression 1 2 3 1 2 2 Zeinab Kosibaty ● Yoshihiko Murata ● Yuko Minami ● Tomoko Dai ● Junko Kano ● Ryota Matsuoka ● 2 2 Noriyuki Nakano ● Masayuki Noguchi Received: 8 March 2018 / Revised: 14 August 2018 / Accepted: 20 August 2018 / Published online: 12 December 2018 © United States & Canadian Academy of Pathology 2018 Abstract Epithelial cell transforming sequence 2 (ECT2), a guanine nucleotide exchange factor, is predominantly localized in the nucleus of non-transformed cells and functions to regulate cytokinesis. ECT2 is also localized in the cytoplasm of cancer cells. Aberrant cytoplasmic expression of ECT2 is thought to drive tumor growth and invasion. In this study, we investigated the cytoplasmic expression of ECT2 and its prognostic and biological significance in lung adenocarcinoma. Western blotting of cellular fractions from the nucleus and cytoplasm was performed to determine the subcellular localization of ECT2 in lung adenocarcinoma cell lines. The cytoplasmic expression of ECT2 in 167 lung fi 1234567890();,: 1234567890();,: adenocarcinomas was evaluated by immunohistochemistry and its clinical signi cance was examined using Kaplan–Meier curves and Cox regression analysis. Scraping cytology specimens of 13 fresh lung adenocarcinomas were used to assess the subcellular localization of ECT2 and its phosphorylation at Thr790 (P-ECT2(T790)). We found that ECT2 was localized in both the nucleus and cytoplasm of lung adenocarcinoma cell lines and tumor tissues. Cytoplasmic expression of ECT2 was detected by immunohistochemistry in 83 (50%) of the lung adenocarcinomas, and was found to increase during cancer progression. -
In Silico Prediction of High-Resolution Hi-C Interaction Matrices
ARTICLE https://doi.org/10.1038/s41467-019-13423-8 OPEN In silico prediction of high-resolution Hi-C interaction matrices Shilu Zhang1, Deborah Chasman 1, Sara Knaack1 & Sushmita Roy1,2* The three-dimensional (3D) organization of the genome plays an important role in gene regulation bringing distal sequence elements in 3D proximity to genes hundreds of kilobases away. Hi-C is a powerful genome-wide technique to study 3D genome organization. Owing to 1234567890():,; experimental costs, high resolution Hi-C datasets are limited to a few cell lines. Computa- tional prediction of Hi-C counts can offer a scalable and inexpensive approach to examine 3D genome organization across multiple cellular contexts. Here we present HiC-Reg, an approach to predict contact counts from one-dimensional regulatory signals. HiC-Reg pre- dictions identify topologically associating domains and significant interactions that are enri- ched for CCCTC-binding factor (CTCF) bidirectional motifs and interactions identified from complementary sources. CTCF and chromatin marks, especially repressive and elongation marks, are most important for HiC-Reg’s predictive performance. Taken together, HiC-Reg provides a powerful framework to generate high-resolution profiles of contact counts that can be used to study individual locus level interactions and higher-order organizational units of the genome. 1 Wisconsin Institute for Discovery, 330 North Orchard Street, Madison, WI 53715, USA. 2 Department of Biostatistics and Medical Informatics, University of Wisconsin-Madison, Madison, WI 53715, USA. *email: [email protected] NATURE COMMUNICATIONS | (2019) 10:5449 | https://doi.org/10.1038/s41467-019-13423-8 | www.nature.com/naturecommunications 1 ARTICLE NATURE COMMUNICATIONS | https://doi.org/10.1038/s41467-019-13423-8 he three-dimensional (3D) organization of the genome has Results Temerged as an important component of the gene regulation HiC-Reg for predicting contact count using Random Forests. -
Cell Division Symmetry Control and Cancer Stem Cells
AIMS Molecular Science, 7(2): 82–98. DOI: 10.3934/molsci.2020006 Received: 15 February 2020 Accepted: 26 April 2020 Published: 06 May 2020 http://www.aimspress.com/journal/Molecular Review Cell division symmetry control and cancer stem cells Sreemita Majumdar and Song-Tao Liu* Department of Biological Sciences, University of Toledo, Toledo, OH 43606, USA * Correspondence: Email: [email protected]; Tel: +14195307853. Table S1. Genes encoding polarity and fate-determinant proteins involved in asymmetric cell division. C. elegans1 D. melanogaster 1 Mammals1 Description2 Associated with/ Interactors 3 Cellular Localization (mammalian cell)4 Serine/threonine protein microtubule-associated protein cell membrane, peripheral and lateral, par-1 par-1 MARK1/2/3/4 kinase MAPT/TAU cytoplasm, dendrite RING, Lipid binding par-2 - - domain PDZ for membrane, cell junction, adherens junction, cell cortex, par-3 baz PARD3 Oligomerization domain at actin, PARD6 endomembrane system, NTD Continued on next page 2 C. elegans1 D. melanogaster 1 Mammals1 Description2 Associated with/ Interactors 3 Cellular Localization (mammalian cell)4 Serine/threonine-protein nucleus, mitochondria, cytoplasm, par-4 Lkb1 STK11/LKB1 STRAD complex kinase membrane 14-3-3 domain binding par-5 14-3-3 YWHAB phosphoserine/ adapter to many proteins cytoplasm phosphothreonine motif cell membrane, centriolar satellite, actin par-6 par-6 PARD6A/B/G PB1, CRIB, PDZ PARD3 cytoskeleton,centrosome, cytoplasm ,ruffles PARD3, and a PARD6 protein PB1, AGC-Kinase (PARD6A, PARD6B or PARD6G) pkc-3 aPKC PRKCI/Z domain, DAG binding, cytoplasm, nucleus, membrane and a GTPase protein (CDC42 or Zinc finger domain RAC1), LLGL1,ECT2 LRR and PDZ protein Cadherin, Scrib-APC-beta-catenin nucleoplasm, basolateral plasma membrane, let-413 scrib SCRIB family. -
The Rac Gtpase in Cancer: from Old Concepts to New Paradigms Marcelo G
Published OnlineFirst August 14, 2017; DOI: 10.1158/0008-5472.CAN-17-1456 Cancer Review Research The Rac GTPase in Cancer: From Old Concepts to New Paradigms Marcelo G. Kazanietz1 and Maria J. Caloca2 Abstract Rho family GTPases are critical regulators of cellular func- mislocalization of Rac signaling components. The unexpected tions that play important roles in cancer progression. Aberrant pro-oncogenic functions of Rac GTPase-activating proteins also activity of Rho small G-proteins, particularly Rac1 and their challenged the dogma that these negative Rac regulators solely regulators, is a hallmark of cancer and contributes to the act as tumor suppressors. The potential contribution of Rac tumorigenic and metastatic phenotypes of cancer cells. This hyperactivation to resistance to anticancer agents, including review examines the multiple mechanisms leading to Rac1 targeted therapies, as well as to the suppression of antitumor hyperactivation, particularly focusing on emerging paradigms immune response, highlights the critical need to develop ther- that involve gain-of-function mutations in Rac and guanine apeutic strategies to target the Rac pathway in a clinical setting. nucleotide exchange factors, defects in Rac1 degradation, and Cancer Res; 77(20); 5445–51. Ó2017 AACR. Introduction directed toward targeting Rho-regulated pathways for battling cancer. Exactly 25 years ago, two seminal papers by Alan Hall and Nearly all Rho GTPases act as molecular switches that cycle colleagues illuminated us with one of the most influential dis- between GDP-bound (inactive) and GTP-bound (active) forms. coveries in cancer signaling: the association of Ras-related small Activation is promoted by guanine nucleotide exchange factors GTPases of the Rho family with actin cytoskeleton reorganization (GEF) responsible for GDP dissociation, a process that normally (1, 2). -
Characterization of Genomic Copy Number Variation in Mus Musculus Associated with the Germline of Inbred and Wild Mouse Populations, Normal Development, and Cancer
Western University Scholarship@Western Electronic Thesis and Dissertation Repository 4-18-2019 2:00 PM Characterization of genomic copy number variation in Mus musculus associated with the germline of inbred and wild mouse populations, normal development, and cancer Maja Milojevic The University of Western Ontario Supervisor Hill, Kathleen A. The University of Western Ontario Graduate Program in Biology A thesis submitted in partial fulfillment of the equirr ements for the degree in Doctor of Philosophy © Maja Milojevic 2019 Follow this and additional works at: https://ir.lib.uwo.ca/etd Part of the Genetics and Genomics Commons Recommended Citation Milojevic, Maja, "Characterization of genomic copy number variation in Mus musculus associated with the germline of inbred and wild mouse populations, normal development, and cancer" (2019). Electronic Thesis and Dissertation Repository. 6146. https://ir.lib.uwo.ca/etd/6146 This Dissertation/Thesis is brought to you for free and open access by Scholarship@Western. It has been accepted for inclusion in Electronic Thesis and Dissertation Repository by an authorized administrator of Scholarship@Western. For more information, please contact [email protected]. Abstract Mus musculus is a human commensal species and an important model of human development and disease with a need for approaches to determine the contribution of copy number variants (CNVs) to genetic variation in laboratory and wild mice, and arising with normal mouse development and disease. Here, the Mouse Diversity Genotyping array (MDGA)-approach to CNV detection is developed to characterize CNV differences between laboratory and wild mice, between multiple normal tissues of the same mouse, and between primary mammary gland tumours and metastatic lung tissue. -
A Computational Approach for Defining a Signature of Β-Cell Golgi Stress in Diabetes Mellitus
Page 1 of 781 Diabetes A Computational Approach for Defining a Signature of β-Cell Golgi Stress in Diabetes Mellitus Robert N. Bone1,6,7, Olufunmilola Oyebamiji2, Sayali Talware2, Sharmila Selvaraj2, Preethi Krishnan3,6, Farooq Syed1,6,7, Huanmei Wu2, Carmella Evans-Molina 1,3,4,5,6,7,8* Departments of 1Pediatrics, 3Medicine, 4Anatomy, Cell Biology & Physiology, 5Biochemistry & Molecular Biology, the 6Center for Diabetes & Metabolic Diseases, and the 7Herman B. Wells Center for Pediatric Research, Indiana University School of Medicine, Indianapolis, IN 46202; 2Department of BioHealth Informatics, Indiana University-Purdue University Indianapolis, Indianapolis, IN, 46202; 8Roudebush VA Medical Center, Indianapolis, IN 46202. *Corresponding Author(s): Carmella Evans-Molina, MD, PhD ([email protected]) Indiana University School of Medicine, 635 Barnhill Drive, MS 2031A, Indianapolis, IN 46202, Telephone: (317) 274-4145, Fax (317) 274-4107 Running Title: Golgi Stress Response in Diabetes Word Count: 4358 Number of Figures: 6 Keywords: Golgi apparatus stress, Islets, β cell, Type 1 diabetes, Type 2 diabetes 1 Diabetes Publish Ahead of Print, published online August 20, 2020 Diabetes Page 2 of 781 ABSTRACT The Golgi apparatus (GA) is an important site of insulin processing and granule maturation, but whether GA organelle dysfunction and GA stress are present in the diabetic β-cell has not been tested. We utilized an informatics-based approach to develop a transcriptional signature of β-cell GA stress using existing RNA sequencing and microarray datasets generated using human islets from donors with diabetes and islets where type 1(T1D) and type 2 diabetes (T2D) had been modeled ex vivo. To narrow our results to GA-specific genes, we applied a filter set of 1,030 genes accepted as GA associated. -
Renal Cell Neoplasms Contain Shared Tumor Type–Specific Copy Number Variations
The American Journal of Pathology, Vol. 180, No. 6, June 2012 Copyright © 2012 American Society for Investigative Pathology. Published by Elsevier Inc. All rights reserved. http://dx.doi.org/10.1016/j.ajpath.2012.01.044 Tumorigenesis and Neoplastic Progression Renal Cell Neoplasms Contain Shared Tumor Type–Specific Copy Number Variations John M. Krill-Burger,* Maureen A. Lyons,*† The annual incidence of renal cell carcinoma (RCC) has Lori A. Kelly,*† Christin M. Sciulli,*† increased steadily in the United States for the past three Patricia Petrosko,*† Uma R. Chandran,†‡ decades, with approximately 58,000 new cases diag- 1,2 Michael D. Kubal,§ Sheldon I. Bastacky,*† nosed in 2010, representing 3% of all malignancies. Anil V. Parwani,*†‡ Rajiv Dhir,*†‡ and Treatment of RCC is complicated by the fact that it is not a single disease but composes multiple tumor types with William A. LaFramboise*†‡ different morphological characteristics, clinical courses, From the Departments of Pathology* and Biomedical and outcomes (ie, clear-cell carcinoma, 82% of RCC ‡ Informatics, University of Pittsburgh, Pittsburgh, Pennsylvania; cases; type 1 or 2 papillary tumors, 11% of RCC cases; † the University of Pittsburgh Cancer Institute, Pittsburgh, chromophobe tumors, 5% of RCC cases; and collecting § Pennsylvania; and Life Technologies, Carlsbad, California duct carcinoma, approximately 1% of RCC cases).2,3 Benign renal neoplasms are subdivided into papillary adenoma, renal oncocytoma, and metanephric ade- Copy number variant (CNV) analysis was performed on noma.2,3 Treatment of RCC often involves surgical resec- renal cell carcinoma (RCC) specimens (chromophobe, tion of a large renal tissue component or removal of the clear cell, oncocytoma, papillary type 1, and papillary entire affected kidney because of the relatively large size of type 2) using high-resolution arrays (1.85 million renal tumors on discovery and the availability of a life-sus- probes). -
H4K16 Acetylation Marks Active Genes and Enhancers of Embryonic Stem Cells, but Does Not Alter Chromatin Compaction
Downloaded from genome.cshlp.org on October 5, 2021 - Published by Cold Spring Harbor Laboratory Press H4K16 acetylation marks active genes and enhancers of embryonic stem cells, but does not alter chromatin compaction Gillian Taylor1, Ragnhild Eskeland2, Betül Hekimoglu-Balkan1, Madapura M. Pradeepa1* and Wendy A Bickmore1* 1 MRC Human Genetics Unit, MRC Institute of Genetics and Molecular Medicine at University of Edinburgh, Crewe Road, Edinburgh EH4 2XU, UK 2Current address: Department of Molecular Biosciences, University of Oslo, N-0316 Oslo, Norway *Correspondence to: W. Bickmore or M.M. Pradeepa, MRC Human Genetics Unit, MRC IGMM, Crewe Road, Edinburgh EH4 2XU, UK Tel: +44 131 332 2471 Fax: +44 131 467 8456 Email:[email protected] or [email protected] Running head: H4K16 acetylation and long-range genome regulation Keywords: Chromatin compaction, embryonic stem cells, fluorescence in situ hybridization, histone acetylation, long-range regulation, 1 Downloaded from genome.cshlp.org on October 5, 2021 - Published by Cold Spring Harbor Laboratory Press Abstract Compared with histone H3, acetylation of H4 tails has not been well studied, especially in mammalian cells. Yet, H4K16 acetylation is of particular interest because of its ability to decompact nucleosomes in vitro and its involvement in dosage compensation in flies. Here we show that, surprisingly, loss of H4K16 acetylation does not alter higher-order chromatin compaction in vivo in mouse embryonic stem cells (ESCs). As well as peaks of acetylated H4K16 and Kat8/MOF histone acetyltransferase at the transcription start sites of expressed genes, we report that acetylation of H4K16 is a new marker of active enhancers in ESCs and that some enhancers are marked by H3K4me1, Kat8 and H4K16ac but not by acetylated H3K27 or p300/EP300, suggesting that they are novel EP300 independent regulatory elements. -
A CRISPR-Based Screen for Hedgehog Signaling Provides Insights Into Ciliary Function and Ciliopathies
ARTICLES https://doi.org/10.1038/s41588-018-0054-7 A CRISPR-based screen for Hedgehog signaling provides insights into ciliary function and ciliopathies David K. Breslow 1,2,7*, Sascha Hoogendoorn 3,7, Adam R. Kopp2, David W. Morgens4, Brandon K. Vu2, Margaret C. Kennedy1, Kyuho Han4, Amy Li4, Gaelen T. Hess4, Michael C. Bassik4, James K. Chen 3,5* and Maxence V. Nachury 2,6* Primary cilia organize Hedgehog signaling and shape embryonic development, and their dysregulation is the unifying cause of ciliopathies. We conducted a functional genomic screen for Hedgehog signaling by engineering antibiotic-based selection of Hedgehog-responsive cells and applying genome-wide CRISPR-mediated gene disruption. The screen can robustly identify factors required for ciliary signaling with few false positives or false negatives. Characterization of hit genes uncovered novel components of several ciliary structures, including a protein complex that contains δ -tubulin and ε -tubulin and is required for centriole maintenance. The screen also provides an unbiased tool for classifying ciliopathies and showed that many congenital heart disorders are caused by loss of ciliary signaling. Collectively, our study enables a systematic analysis of ciliary function and of ciliopathies, and also defines a versatile platform for dissecting signaling pathways through CRISPR-based screening. he primary cilium is a surface-exposed microtubule-based approach. Indeed, most studies to date have searched for genes that compartment that serves as an organizing center for diverse either intrinsically affect cell growth or affect sensitivity to applied Tsignaling pathways1–3. Mutations affecting cilia cause ciliopa- perturbations16–23. thies, a group of developmental disorders including Joubert syn- Here, we engineered a Hh-pathway-sensitive reporter to enable drome, Meckel syndrome (MKS), nephronophthisis (NPHP), and an antibiotic-based selection platform. -
Noelia Díaz Blanco
Effects of environmental factors on the gonadal transcriptome of European sea bass (Dicentrarchus labrax), juvenile growth and sex ratios Noelia Díaz Blanco Ph.D. thesis 2014 Submitted in partial fulfillment of the requirements for the Ph.D. degree from the Universitat Pompeu Fabra (UPF). This work has been carried out at the Group of Biology of Reproduction (GBR), at the Department of Renewable Marine Resources of the Institute of Marine Sciences (ICM-CSIC). Thesis supervisor: Dr. Francesc Piferrer Professor d’Investigació Institut de Ciències del Mar (ICM-CSIC) i ii A mis padres A Xavi iii iv Acknowledgements This thesis has been made possible by the support of many people who in one way or another, many times unknowingly, gave me the strength to overcome this "long and winding road". First of all, I would like to thank my supervisor, Dr. Francesc Piferrer, for his patience, guidance and wise advice throughout all this Ph.D. experience. But above all, for the trust he placed on me almost seven years ago when he offered me the opportunity to be part of his team. Thanks also for teaching me how to question always everything, for sharing with me your enthusiasm for science and for giving me the opportunity of learning from you by participating in many projects, collaborations and scientific meetings. I am also thankful to my colleagues (former and present Group of Biology of Reproduction members) for your support and encouragement throughout this journey. To the “exGBRs”, thanks for helping me with my first steps into this world. Working as an undergrad with you Dr. -
Functional Diversification of the Nleg Effector Family in Enterohemorrhagic Escherichia Coli
Functional diversification of the NleG effector family in enterohemorrhagic Escherichia coli Dylan Valleaua, Dustin J. Littleb, Dominika Borekc,d, Tatiana Skarinaa, Andrew T. Quailea, Rosa Di Leoa, Scott Houlistone,f, Alexander Lemake,f, Cheryl H. Arrowsmithe,f, Brian K. Coombesb, and Alexei Savchenkoa,g,1 aDepartment of Chemical Engineering and Applied Chemistry, University of Toronto, Toronto, ON M5S 3E5, Canada; bDepartment of Biochemistry and Biomedical Sciences, Michael G. DeGroote Institute for Infectious Disease Research, McMaster University, Hamilton, ON L8S 4K1, Canada; cDepartment of Biophysics, University of Texas Southwestern Medical Center, Dallas, TX 75390; dDepartment of Biochemistry, University of Texas Southwestern Medical Center, Dallas, TX 75390; ePrincess Margaret Cancer Centre, University of Toronto, Toronto, ON M5G 2M9, Canada; fDepartment of Medical Biophysics, University of Toronto, Toronto, ON M5G 1L7, Canada; and gDepartment of Microbiology, Immunology and Infectious Diseases, University of Calgary, Calgary, AB T2N 4N1, Canada Edited by Ralph R. Isberg, Howard Hughes Medical Institute and Tufts University School of Medicine, Boston, MA, and approved August 15, 2018 (receivedfor review November 6, 2017) The pathogenic strategy of Escherichia coli and many other gram- chains. Depending on the length and nature of the polyubiquitin negative pathogens relies on the translocation of a specific set of chain, it posttranslationally regulates the target protein’s locali- proteins, called effectors, into the eukaryotic host cell during in- zation, activation, or degradation. Ubiquitination is a multistep fection. These effectors act in concert to modulate host cell pro- process which begins with the ubiquitin-activating enzyme (E1) cesses in favor of the invading pathogen. Injected by the type III using ATP to “charge” ubiquitin, covalently binding the ubiquitin secretion system (T3SS), the effector arsenal of enterohemorrhagic C terminus by a thioester linkage. -
Fine-Scale Survey of X Chromosome Copy Number Variants and Indels Underlying Intellectual Disability
ARTICLE Fine-Scale Survey of X Chromosome Copy Number Variants and Indels Underlying Intellectual Disability Annabel C. Whibley,1 Vincent Plagnol,1,2 Patrick S. Tarpey,3 Fatima Abidi,4 Tod Fullston,5,6 Maja K. Choma,1 Catherine A. Boucher,1 Lorraine Shepherd,1 Lionel Willatt,7 Georgina Parkin,7 Raffaella Smith,3 P. Andrew Futreal,3 Marie Shaw,8 Jackie Boyle,9 Andrea Licata,4 Cindy Skinner,4 Roger E. Stevenson,4 Gillian Turner,9 Michael Field,9 Anna Hackett,9 Charles E. Schwartz,4 Jozef Gecz,5,6,8 Michael R. Stratton,3 and F. Lucy Raymond1,* Copy number variants and indels in 251 families with evidence of X-linked intellectual disability (XLID) were investigated by array comparative genomic hybridization on a high-density oligonucleotide X chromosome array platform. We identified pathogenic copy number variants in 10% of families, with mutations ranging from 2 kb to 11 Mb in size. The challenge of assessing causality was facilitated by prior knowledge of XLID-associated genes and the ability to test for cosegregation of variants with disease through extended pedigrees. Fine-scale analysis of rare variants in XLID families leads us to propose four additional genes, PTCHD1, WDR13, FAAH2, and GSPT2,as candidates for XLID causation and the identification of further deletions and duplications affecting X chromosome genes but without apparent disease consequences. Breakpoints of pathogenic variants were characterized to provide insight into the underlying mutational mechanisms and indicated a predominance of mitotic rather than meiotic events. By effectively bridging the gap between karyotype-level investigations and X chromosome exon resequencing, this study informs discussion of alternative mutational mechanisms, such as non- coding variants and non-X-linked disease, which might explain the shortfall of mutation yield in the well-characterized International Genetics of Learning Disability (IGOLD) cohort, where currently disease remains unexplained in two-thirds of families.