Three Essays on Regulatory, Market, and Estimation Risk
Total Page:16
File Type:pdf, Size:1020Kb
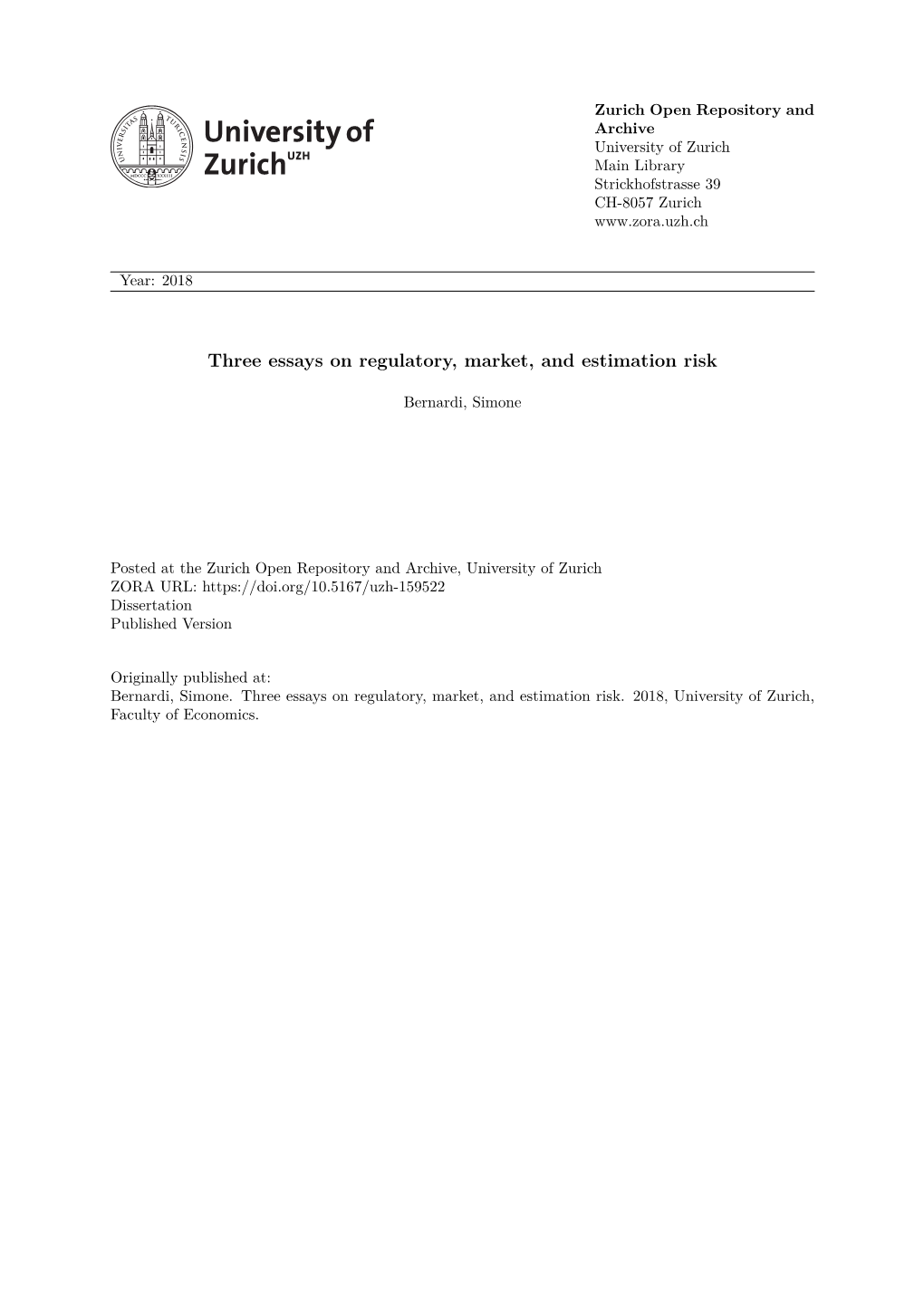
Load more
Recommended publications
-
Ascom Enters Into a Syndicated Loan Agreement with Swiss Banks
MEDIA RELEASE Ascom enters into a syndicated loan agreement with Swiss banks Ascom enters into a syndicated loan agreement of CHF 60 million over four years with a Swiss bank consortium. Ascom and a bank consortium consisting of UBS Switzerland AG (lead), Baar, Switzerland Credit Suisse (Switzerland) Ltd., Zuger Kantonalbank, and Zürcher Kantonalbank signed a syndicated loan agreement for a period of four 20 November 2020 years. The syndicate banks are committed to make available to Ascom a senior revolving loan facility in an aggregate amount equal to CHF Daniel Lack 60 million, which serves general corporate purposes of the Group. The Senior VP Legal & Communications / IR credit facility includes customary financial covenants. Ascom Group Media Office +41 41 544 78 10 Moreover, the syndicate banks may make available to Ascom, on an [email protected] uncommitted basis, additional loan facilities in an aggregate amount equal to CHF 20 million for financing specific commercial projects. With this syndicated loan facility, Ascom will replace the existing bilateral credit facilities with two banks entered on 31 March 2020. About Ascom Ascom is a global solutions provider focused on healthcare ICT and mobile workflow solutions. The vision of Ascom is to close digital information gaps allowing for the best possible decisions – anytime and anywhere. Ascom’s mission is to provide mission-critical, real-time solutions for highly mobile, ad hoc, and time-sensitive environments. Ascom uses its unique product and solutions portfolio and software architecture capabilities to devise integration and mobilization solutions that provide truly smooth, complete, and efficient workflows for healthcare as well as for industry and retail sectors. -
Die Kantonalbanken in Zahlen
Kennziffern und Adressen Die Kantonalbanken der Kantonalbanken in Zahlen Kennzahlen der 24 Kantonalbanken Eckdaten der 24 Kantonalbanken Angaben per 31.12.2018 (inkl. Tochtergesellschaften) Angaben per 31.12.2018 Kantonalbank Gründungsjahr Bilanzsumme Geschäftsstellen Personalbestand Kantonalbank Rechts- Dotations-/ PS-Kapital Kotierung Staats- in Mio. CHF teilzeitbereinigt form Aktienkapital in Mio. CHF SIX garantie in Mio. CHF Aargauische Kantonalbank 1913 28‘351 31 708 Aargauische Kantonalbank örK 200 - - ja Appenzeller Kantonalbank 1899 3‘365 4 81 Appenzeller Kantonalbank örK 30 - - ja Banca dello Stato del Cantone Ticino 1915 14‘322 21 444 Banca dello Stato del Cantone Ticino örK 430 - - ja Banque Cantonale de Fribourg 1892 22‘927 27 382 Banque Cantonale de Fribourg örK 70 - - ja Banque Cantonale de Genève 1816 23‘034 28 761 Banque Cantonale de Genève AG 360 - ja nein Banque Cantonale du Jura 1979 3‘152 12 122 Banque Cantonale du Jura AG 42 - ja ja Banque Cantonale du Valais 1917 16‘122 45 471 Banque Cantonale du Valais AG 158 - ja ja Banque Cantonale Neuchâteloise 1883 10‘847 12 285 Banque Cantonale Neuchâteloise örK 100 - - ja Banque Cantonale Vaudoise 1845 47‘863 72 1‘896 Banque Cantonale Vaudoise AG 86 - ja nein Basellandschaftliche Kantonalbank 1864 25‘341 22 689 Basellandschaftliche Kantonalbank örK 160 57 ja ja Basler Kantonalbank 1899 44‘031 46 1‘238 Basler Kantonalbank örK 304 50 ja ja Berner Kantonalbank 1834 30‘589 60 1‘000 Berner Kantonalbank AG 186 - ja nein Glarner Kantonalbank 1884 5‘982 6 191 Glarner Kantonalbank AG -
A Primer on DOJ's Swiss Bank Program
1012 Broad Street, 2nd Fl Bloomfield, NJ 07003 Tel (973) 783-7000 Fax (973) 338-3955 www.DeBlisLaw.com HIGH-STAKES TAX DEFENSE & COMPLEX CRIMINAL DEFENSE A Primer on DOJ’s Swiss Bank Program a. Background Information From the outside looking in, it is easy to accuse those who are unfamiliar with the Department of Justice’s Swiss Bank Program as living under a rock. But that is presumptuous when you are a tax attorney who is immersed in this work every day. Let’s begin with some background information. The Swiss Bank Program was unveiled on August 29, 2013. It provides a path for Swiss banks to resolve potential criminal liabilities in the United States. In order to participate, Swiss banks had to take the “bull by its horns” and notify the Department of Justice by December 31, 2013 that they had reason to believe that they had committed tax-related criminal crimes in connection with unreported U.S.-related accounts. In other words, they had to “eat crow.” Banks already under criminal investigation for shady banking activities (along with any individuals who work for such banks) were deemed ineligible from participating in the program. In order to be eligible for a non-prosecution agreement, banks must do the following: (1) Make a complete disclosure of their cross-border activities; (2) Provide detailed information on an account-by-account basis for accounts in which U.S. taxpayers have a direct or indirect interest; (3) Cooperate in treaty requests for account information; (4) Provide detailed information as to other banks that transferred funds into secret accounts or that accepted funds when secret accounts were closed; (5) Agree to close accounts of accountholders who fail to come into compliance with U.S. -
Stabilität Im System? Datenkassation – Pilotprojekt Innovation@Van Lanschot 1 Wird Mit SHKB Umgesetzt 6 8
INSIGHT DEZEMBER 2017 Marktperspektive Finnova Consulting Finnova Community Stabilität im System? Datenkassation – Pilotprojekt Innovation@Van Lanschot 1 wird mit SHKB umgesetzt 6 8 MARKTPERSPEKTIVE LIEBE LESERIN, LIEBER LESER Als führender Bankensoftwarehersteller sehen STABILITÄT wir unsere primäre Aufgabe darin, die Banken und Finanzdienstleister in ihrer Leistungserstellung zu unterstützen. Kundennutzen zu generieren im IM SYSTEM? Kontext von Digitalisierung, Automatisierung, In- dus-trialisierung und Compliance-Vorgaben ist in der Tat eine echte Herausforderung. Die IFZ Retail-Banking-Studie 20171 bestätigt, dass die Hier setzt die diesjährige IFZ Retail-Banking- Zufriedenheit der Kunden von Schweizer Retailbanken Studie an. Sie hat die Zufriedenheit der Bankkun- im Wesentlichen auf den drei Faktoren «Preis/Leistung», den mit der Leistung ihrer Bank gemessen und ver- «Transparenz2» und «Kundenwertschätzung» basiert und glichen. Dies gibt uns wertvolle Hinweise – wir die Bereitschaft der Kunden, die Bankbeziehung zu wech- beschäftigen uns laufend mit der Frage, wo und seln, im einprozentigen Bereich konstant tief ist. Heureka wie wir über die ganze integrierte Wertschöp- oder Dilemma? Wie sind diese Erkenntnisse zu werten? fungskette hinweg Mehrwerte für die Bank und Ein Blick hinter die Kulissen und ein paar Gedanken zur ihre Kunden generieren können. möglichen Zukunft. «Von der Wiege bis zur Bahre», so sollen die per- sonenbezogenen Daten geschützt sein. Wie wird Dass die Aufspaltung der Wertschöpfungsketten im Banking aber sichergestellt, -
Terms of Business of the Swiss National Bank
Terms of Business 2019 Table of contents 1 General conditions 1.1 Purpose and scope of application 1 1.2 Exclusion of an obligation to contract 1 1.3 Conflict with other terms of business 1 1.4 Formal requirements for the SNB’s contracting partners 2 1.5 Recording of telephone conversations 2 1.6 Authority to sign on behalf of the SNB 2 1.7 Authority to sign on behalf of the contracting partners 3 1.8 Communications from the SNB 3 1.9 Liability of the SNB 3 1.10 Rights of lien and setoff 4 1.11 Charges 4 1.12 Place of performance 4 1.13 Notice of termination 5 1.14 Business hours 5 1.15 Applicable law and jurisdiction 5 1.16 Amendments to Terms of Business and conditions 5 1.17 General provision on data protection 6 1.18 Publication of data for specific bank services 6 2 Payment transactions 2.1 Admission to the giro system 7 2.2 Sight deposit account conditions 7 2.3 Cheque transactions 8 2.4 Collection 8 2.5 Cash transactions 8 3 Repo transactions 3.1 General 10 3.2 Open market operations 10 3.3 Standing facilities 10 4 Foreign exchange and gold transactions 4.1 Foreign exchange transactions 12 4.2 Gold operations 12 5 Custody services 5.1 Purchase and sale of custody assets 13 5.2 Custody and administration of custody assets 13 Annexes I List of head offices and agencies of the Swiss National Bank 15 II Instruction sheets applicable in conjunction with these Terms of Business 16 Swiss National Bank Secretariat General P.O. -
Editorial Announcement
SIX Management AG Editorial announcement Selnaustrasse 30 Postfach 1758 CH-8021 Zürich www.six-group.com 2 March 2016 Media Relations: T +41 58 399 2227 F +41 58 499 2710 [email protected] Pay using Paymit at some small merchants from today The first shops and restaurants now accept Paymit, the most used mobile payment app in Switzerland. The one-month pilot phase for the first Paymit solution for cashless, mobile payments is starting today. From today, you can use Paymit to make mobile and cashless payments in selected shops and restaurants. The new solution is particularly suitable for merchants and service providers that previously only accepted cash, such as small cafes, take-aways, market stalls or unstaffed points of sale. With Paymit, merchants and their customers have a simple, reliable option for cashless payments even if there is no card terminal. The pilot phase for merchants will run from now until the end of March 2016. Shop or restaurant owners that are interested can register from now via the website www.paymit.com. After SIX carries out a check, they receive a QR code as a sticker, which they can attach to their point of sale. To make a payment, the customer scans this QR code with his Paymit app, enters the amount and then pays. Mobile payments make cash handling far easier for smaller merchants. Domino’s Pizza, Dieci, Coppolini, Il Caffè and the Student Union (SHSG) of the University of St. Gallen are among the first pilot merchants. To activate the new merchant function, all Paymit apps from SIX, UBS, Zürcher Kantonalbank and Luzerner Kantonalbank are being updated. -
Finnova Banking Software End-To-End, Efficient, Open, Innovative 3
FINNOVA BANKING SOFTWARE END-TO-END, EFFICIENT, OPEN, INNOVATIVE 3 Table of contents Finnova at a glance 4 Smarter Banking 6 Finnova Banking Software’s winning formula 8 Value proposition for our customers 10 Finnova Banking Software at a glance 12 Further development of the Finnova Banking Software 20 Services 24 Finnova Channel Suite 28 Finnova Front Suite 34 Finnova Management Suite 40 Finnova Expert Suite 44 Finnova Solution Suite 58 Licence and price model 62 Lenzburg, September 2017 4 FINNOVA AT A GLANCE Finnova is a leading provider of banking software in the Swiss financial centre. We help banks and outsourcing providers to realise growth in the banking sector, even in chal- lenging times, thanks to efficient and innovative IT solutions compliant with regulatory requirements. ‘Smarter banking’ with Finnova – that is what we stand for. And that is why over 100 banks have already put their trust in us. Finnova was founded in 1974 and now employs around 400 people at its headquarters in Lenzburg and at branch offices in Chur, Seewen and Nyon. With the Finnova Banking Software, around 80 universal and 20 private banks benefit from the at- tractive total cost of ownership (TCO) of this very high-per- Today, more than 100 universal and private banks from Switzer- formance, reliable standard solution, which can be used front- land and Liechtenstein are among our customers. We have to-back for various business models thanks to its wide range evolved to become one of the leading banking software manu- of functions. facturers in the Swiss financial centre. The Finnova platform is open to software solutions devel- oped by banks or third parties such as fintechs, so that banks Our future can set themselves apart on the market in this period of digi- It is our ambition for the future to establish Finnova software talisation. -
SIX Repo Ltd - Member List As of 20 April 2021
SIX Repo Ltd - Member list as of 20 April 2021 Bank / Place City Aargauische Kantonalbank Aarau AEK Bank 1826 Genossenschaft Thun Allgemeine Sparkasse Oberösterreich Bankaktiengesellschaft Linz Allianz Suisse Lebensversicherungs-Gesellschaft AG, EL Wallisellen Allianz Suisse Lebensversicherungs-Gesellschaft AG, KL Wallisellen Allianz Suisse Versicherungs-Gesellschaft AG Wallisellen Austrian Anadi Bank AG Klagenfurt AXA Leben AG Einzel Winterthur AXA Leben AG Kollektiv Winterthur AXA Versicherungen AG Winterthur Baloise Bank SoBa Solothurn Banca del Ceresio SA Lugano Banca del Sempione SA Lugano Banca dello Stato del Cantone Ticino Bellinzona Banca Popolare di Sondrio (Suisse) S.A. Lugano Bank CIC (Schweiz) AG Basel Bank Cler AG Basel Bank EEK AG Bern Bank EKI Genossenschaft Interlaken Bank Frick & Co. AG Balzers Bank für Tirol und Vorarlberg AG (BTV) Innsbruck Bank J. Safra Sarasin AG Basel Bank Julius Bär & Co. AG Zürich Bank Linth LLB AG Uznach Bank Thalwil Genossenschaft Thalwil Bank Vontobel AG Zürich Banque Bonhôte & Cie. SA Neuchâtel Banque Cantonale de Fribourg Fribourg Banque Cantonale de Genève Genève Banque Cantonale du Jura Porrentruy Banque Cantonale du Valais Sion Banque Cantonale Neuchâteloise Neuchâtel Banque Cantonale Vaudoise Lausanne Banque Cramer & Cie SA Genève Banque Internationale à Luxembourg SA Luxembourg Barclays Bank UK Plc London Basellandschaftliche Kantonalbank Liestal Basler Kantonalbank Basel Basler Leben AG Basel Basler Versicherung AG Basel Last update: 20 April 2021 Page: 1/4 BBVA SA Zürich Bendura Bank -
Document Vide
SIMPLIFIED PROSPECTUS Tracker Certificate Underlyings: Swiss cantonal banks’ shares Redemption: Open-ended This structured product is not a collective investment within the meaning of the Swiss Federal Investment Fund Act. It does not require approval from the Swiss Financial Market Supervisory Authority (FINMA) and is not subject to FINMA supervision. Investors are also exposed to the risk of insolvency of the Issuer. This Certificate is actively managed on a discretionary basis and has a dynamic structure. 1. PRODUCT DESCRIPTION Details Sec. No. / ISIN / Symbol 27 171 233 / CH0271712330 / No listing planned Issuer and Lead Manager Banque Cantonale Vaudoise, Lausanne, Switzerland (S&P AA/stable/A-1+) Prudential supervision BCV, Lausanne, Switzerland is subject to prudential supervision by the Swiss Financial Market Supervisory Authority (FINMA). Paying and calculation Banque Cantonale Vaudoise, Lausanne agent Investment Manager Banque Cantonale de Genève (BCGE), Geneva, Switzerland BCGE is subject to prudential supervision by FINMA. Underlying asset Basket of Swiss cantonal bank shares Management method Discretionary and dynamic Conversion ratio 1 Certificate = 1 basket Issue volume 150,000 Certificates with a reopening clause, up to a maximum of 150,000 Certificates Minimum investment 1 Certificate Base currency CHF Issue price CHF 200.00 (includes an issue margin of CHF 1.60) Reference price CHF 198.40 Distribution fees 0.40% of the issue price Initial fixing date 24 March 2015 Payment date 13 April 2015 Effective termination Open End date/redemption Redemption date The sixth working day following the termination date set by either the Investor or the Issuer (barring extraordinary market situations and emergencies). Product description This CHF-denominated Certificate comprises a selection of shares in Swiss cantonal banks and a cash component (see definition below). -
Bewilligte Banken Und Wertpapierhäuser
Bewilligte Banken und Wertpapierhäuser Name Ort Zulassung Bankart Wertpapierhaus-Art keine Wertpapierhaustätigkeit nicht-kontoführendes Wertpapierhaus Kategorie Aargauische Kantonalbank Aarau Bank Kantonalbanken Inländisches Wertpapierhaus 3 ABANCA CORPORACION BANCARIA S.A., Genève 1 Zweigniederlassung ausl. Bank Erste Zweigniederlassung ausländische X 5 Betanzos, succursale de Genève Bank acrevis Bank AG St. Gallen Bank Regionalbanken und Sparkassen Inländisches Wertpapierhaus 4 AEK BANK 1826 Genossenschaft Thun Bank Regionalbanken und Sparkassen Inländisches Wertpapierhaus 4 AFS Equity & Derivatives B.V., Amsterdam, Regensdorf Zweigniederlassung ausl. Wertpapierhaus Wertpapierhaus Erste Zweigniederlassung eines X 5 Zweigniederlassung Regensdorf ausländischen Wertpapierhauses Allfunds Bank International S.A., Luxembourg, Zurich Zürich Zweigniederlassung ausl. Bank Erste Zweigniederlassung ausländische Erste Zweigniederlassung eines 5 Branch Bank ausländischen Wertpapierhauses Alpha RHEINTAL Bank AG Heerbrugg Bank Regionalbanken und Sparkassen Inländisches Wertpapierhaus 4 Alternative Bank Schweiz AG Olten Bank Andere Banken Inländisches Wertpapierhaus 4 Appenzeller Kantonalbank Appenzell Bank Kantonalbanken Inländisches Wertpapierhaus 4 Aquila AG Zürich Bank Andere Banken Inländisches Wertpapierhaus 5 Arab Bank (Switzerland) Ltd. Genève 3 Bank Ausländisch beherrschte Banken Ausländisch beherrschte 4 Wertpapierhäuser AXION SWISS BANK SA Lugano Bank Auf Börsen, Effekten-, Vermögensverw.- Inländisches Wertpapierhaus 4 Geschäfte spez. Institute -
The Swiss Banking Websites Index Q3 2010/701
indexThe Swiss Banking Websites Index Q3 2010/701 To compile this survey we reviewed over 250 websites, using the Sitemorse automated website optimisation software (page content, templates and infrastructure) to test and rank each website. This report presents a summary of the findings and is produced in association with Supertext. www.supertext.ch We run more tests, in more depth, more often than anyone else. Our results are more accurate, more accessible and more useful than anyone else’s, and our reports are published in more places, read by more people and trusted by more organisations The Swiss Banking Websites Index Q3 2010/701 than anyone else’s. index Contents Welcome note 3 The better you tell, the better you sell 4 The top fifty 5 Time to wait 6 Fatwire - Content Integration Platform™ 7 Most improved site 8 Who’s up and who’s down 8 The bottom twenty 8 Web accessibility denied 9 92% of sites need to consider the impact 10 Welcome to the 8th issue of the Swiss Banking Websites Index. This quarterly survey provides an independent benchmark of over 250 websites, employing more than 600 tests on each page tested. The banking industry needs to improve online quality, compliance and user experience – preferably at the point of website delivery, not as an afterthought. A positive user experience is not simply a matter of beautiful design: the primary requirement is for the website to function properly and quickly. The Sitemorse tests routinely uncover all kinds of problems that can have a big impact on a visitor’s impression – such as dead links, compliance issues, non-functioning email addresses, slow pages, poor ranking in search engines, etc. -
The Rise of Bank Prosecutions Brandon L
THE YALE LAW JOURNAL FORUM M AY 23, 2016 The Rise of Bank Prosecutions Brandon L. Garrett introduction Before 2008, prosecutions of banks had been quite rare in the federal courts, and the criminal liability of banks and bankers was not a topic that received much public or scholarly attention. In the wake of the last financial crisis, however, critics have begun to ask whether prosecutors adequately held banks and bankers accountable for their crimes. Senator Jeff Merkley complained: “[A]fter the financial crisis, the [Justice] Department appears to have firmly set the precedent that no bank, bank employee, or bank executive can be prosecuted.”1 Federal judge Jed Rakoff and many others asked why prosecutors brought, with one or two low-level exceptions, no prosecutions of bankers in the wake of the 2007-2008 financial crisis and whether they were too quick to settle corporate cases by merely compelling fines and “window- dressing” compliance reforms.2 The response from the Department of Justice (DOJ) to criticism of its approach towards corporate and financial prosecutions has ranged from stern denial that it had been remiss—as when Attorney General Eric Holder announced in a video message in 2014 that “[t]here is no such thing as too big to jail” and that no financial institution “should be considered immune from prosecution”3—to reform in the face of 1. Press Release, Senator Jeff Merkley, Merkley Blasts ‘Too Big to Jail’ Policy for Lawbreaking Banks, (Dec. 13, 2012), http://www.merkley.senate.gov/news/press-releases/merkley-blasts -too-big-to-jail-policy-for-lawbreaking-banks [http://perma.cc/BY2U-GUE9].