Driven by Affect to Explore Asteroids, the Moon, and Science Education
Total Page:16
File Type:pdf, Size:1020Kb
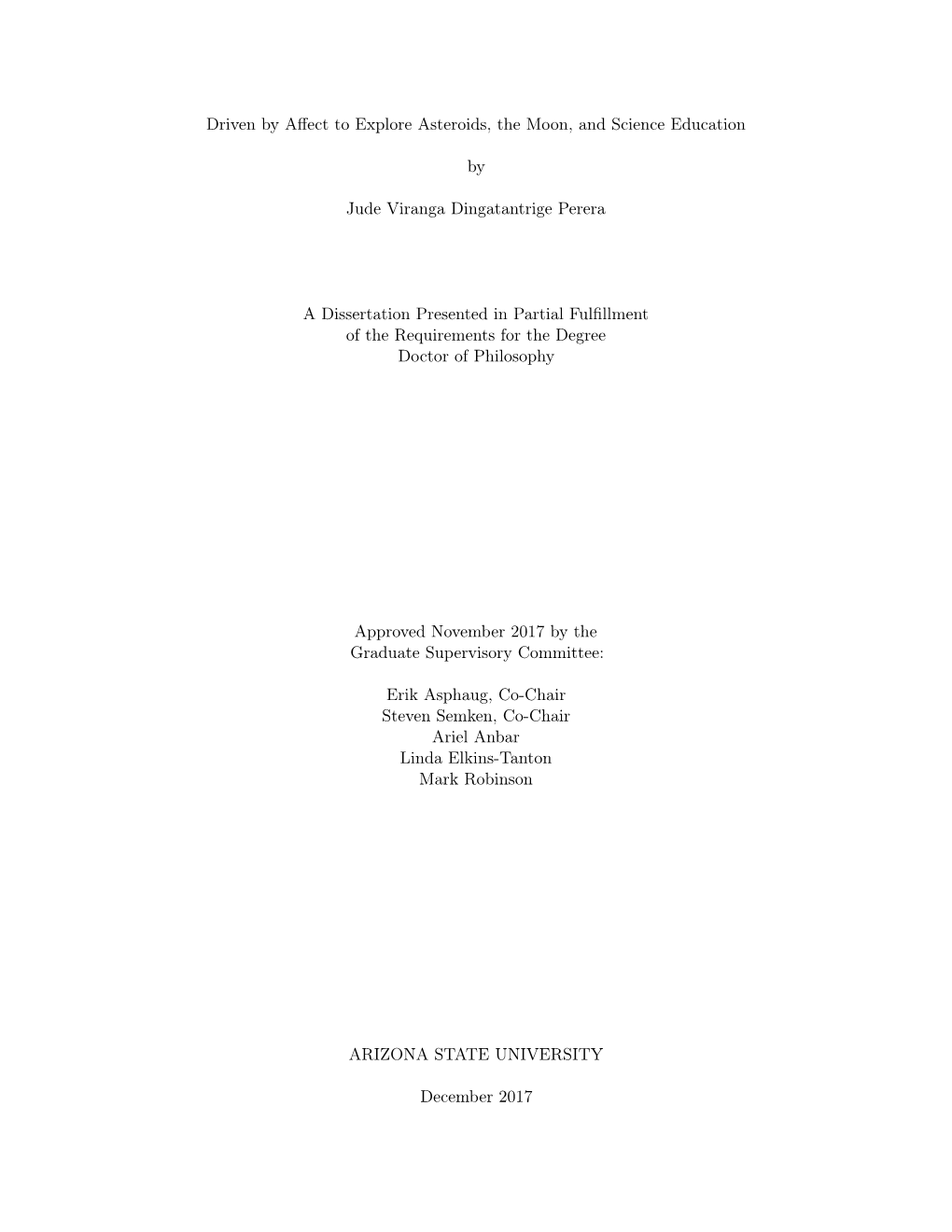
Load more
Recommended publications
-
The Futurist Moment : Avant-Garde, Avant Guerre, and the Language of Rupture
MARJORIE PERLOFF Avant-Garde, Avant Guerre, and the Language of Rupture THE UNIVERSITY OF CHICAGO PRESS CHICAGO AND LONDON FUTURIST Marjorie Perloff is professor of English and comparative literature at Stanford University. She is the author of many articles and books, including The Dance of the Intellect: Studies in the Poetry of the Pound Tradition and The Poetics of Indeterminacy: Rimbaud to Cage. Published with the assistance of the J. Paul Getty Trust Permission to quote from the following sources is gratefully acknowledged: Ezra Pound, Personae. Copyright 1926 by Ezra Pound. Used by permission of New Directions Publishing Corp. Ezra Pound, Collected Early Poems. Copyright 1976 by the Trustees of the Ezra Pound Literary Property Trust. All rights reserved. Used by permission of New Directions Publishing Corp. Ezra Pound, The Cantos of Ezra Pound. Copyright 1934, 1948, 1956 by Ezra Pound. Used by permission of New Directions Publishing Corp. Blaise Cendrars, Selected Writings. Copyright 1962, 1966 by Walter Albert. Used by permission of New Directions Publishing Corp. The University of Chicago Press, Chicago 60637 The University of Chicago Press, Ltd., London © 1986 by The University of Chicago All rights reserved. Published 1986 Printed in the United States of America 95 94 93 92 91 90 89 88 87 86 54321 Library of Congress Cataloging-in-Publication Data Perloff, Marjorie. The futurist moment. Bibliography: p. Includes index. 1. Futurism. 2. Arts, Modern—20th century. I. Title. NX600.F8P46 1986 700'. 94 86-3147 ISBN 0-226-65731-0 For DAVID ANTIN CONTENTS List of Illustrations ix Abbreviations xiii Preface xvii 1. -
Built Upon Sand: Theoretical Ideas Inspired by the Flow of Granular Materials
• Built Upon Sand: Theoretical Ideas Inspired by the Flow of Granular materials Leo P Kadanoff* The James Franck Institute The University of Chicago 5640 S. Ellis Avenue Chicago, IL 60637 USA Abstract Granulated materials, like sand and sugar and salt, are composed of many pieces which can move independently. The study of collisions and flow in these materials requires new theoretical ideas beyond those in the standard statistical mechanics, or hydrodynamics, or traditional solid mechanics. Granular materials differ from standard molecular materials in that frictional forces among grains can dissipate energy and drive the system toward frozen or glassy configurations. In experimental studies of these materials, one sees complex flow patterns similar to those of ordinary liquids, but also freezing, plasticity, and hysteresis. To explain these results, theorists have looked at mQdels based upon inelastic collisions among particles. With the aid of computer simulations of these models they have tried to build a 'statistical dynamics' of inelastic collisions. One effect seen, called inelastic collapse, is a freeZing of some of the degrees of freedom induced by an infinity of inelastic collisions. More often some degrees of freedom are partially frozen, so that we can have a rather cold clump of material in correlated motion. Conversely, thin layers of material may be mobile, while all the material around then is frozen. In these and other ways, granular motion looks different from movement in other kinds of materials. Simulations in simple geometries may also be used to ask questions like 'when does the usual Boltzmann-Gibbs-Maxwell statistical mechanics arise?', 'what are the nature of the probability distributions for forces between the grains?', and whether the system might possibly be described by uniform partial differential equations. -
Dynamics of Granular Materials . / R. P. Behringer Department Of
Dynamics of Granular Materials _ . _ / R. P. Behringer Department of Physics and Center forNonlinear and Complex Systems _/,_ Duke University Granular materials exhibit a rich variety of dynamical behavior, much of which is poorly understood. Practai-like stress chains, convection, s variety of wave dynamics, including waves which resemble capillary waves, 1/f noise, and fractlona] Brown]an motion provide examples. Work beginning at Duke will focus on gravity driven convection, mixing and gravitational collapse. Although granular materials consist of collections of interacting particles, there are important differences between the dynamics of a collections of grains and the dynamics of a collections of molecules. In particular, the ergodic hypothesis is generally invalid for granular materials, so that ordinary statistical physics does not apply. In the absence of a steady energy input, granular materials undergo a rapid collapse which is strongly influenced by the presence of gravity. Fluctuations on laboratory scales _ such quantities as the stress can be very large-as much as an order of magnitude greater than the mean. I. Introduction In this paper, I briefly given an overview of important aspects of granular flows. I then focus on recent experiments on gravity-driven convective flows. I conclude by indicating future directions. Granular materials _-3 are collections of macroscopic particles or grains typically having inelastic interactions and often surrounded by a fluid, such as air or water. The interactions between granular materials are governed chiefly by the local elastic and frictional forces between particles, or between particles and a wall. The surrounding fluid may also play an important role in the dynamics of the system. -
Inspirations Belmond Italy, Mallorca & Madeira
INSPIRATIONS BELMOND ITALY, MALLORCA & MADEIRA PLUNGE INTO OUR WORLD CELEBRATE A LEGEND ESCAPE ON UNIQUE ADVENTURES BELMOND INSPIRATIONS 2018 BELMOND INSPIRATIONS 2018 NEWS WELCOME Welcome to the exciting new season unfolding at our wonderful world of Belmond. Whether you join us in Italy, Mallorca or Madeira, expect to be amazed by the unique and truly exclusive adventures that await. Behind all our hotels are inspiring stories that celebrate what we call The Art of Belmond. This year we’re raising Bellinis galore to mark Belmond Hotel Cipriani’s first 60 years. But we’re also answering the call of the wild and heading to a Sicilian island to meet an artist at home in her mountain cave. Our heritage, authenticity—and spirit of playfulness—all come together in these and many other once-in-a-lifetime experiences. Do come and stay during what promises to be an exceptionally fun and festive year—and become part of our continuing story. I look forward to welcoming you. Robert Koren, Vice President Southern Europe, Belmond CONTENTS PAGE 3 | YOU’VE ARRIVED! PAGE 24 | GREAT EXPLORATIONS Make a glamorous new entrance Discover hidden worlds and remarkable stories—a at Belmond Reid’s Palace. rare rose in Venice, a secret Madeiran vineyard, incredible art trails in Mallorca and Tuscany. PAGE 4 | 60 YEARS A LEGEND Celebrate the diamond anniversary—and PAGE 40 | EPICUREAN ESCAPADES YOU’VE glittering history—of Belmond Hotel Cipriani. The joy of travel meets the bliss of food in these mouthwatering experiences, from Sicilian cooking ARRIVED! PAGE 10 | FOR YOUR EYES ONLY classes to a Madeiran mountain breakfast. -
Volume 64, Number 04 (April 1946) James Francis Cooke
Gardner-Webb University Digital Commons @ Gardner-Webb University The tudeE Magazine: 1883-1957 John R. Dover Memorial Library 4-1-1946 Volume 64, Number 04 (April 1946) James Francis Cooke Follow this and additional works at: https://digitalcommons.gardner-webb.edu/etude Part of the Composition Commons, Music Pedagogy Commons, and the Music Performance Commons Recommended Citation Cooke, James Francis. "Volume 64, Number 04 (April 1946)." , (1946). https://digitalcommons.gardner-webb.edu/etude/196 This Book is brought to you for free and open access by the John R. Dover Memorial Library at Digital Commons @ Gardner-Webb University. It has been accepted for inclusion in The tudeE Magazine: 1883-1957 by an authorized administrator of Digital Commons @ Gardner-Webb University. For more information, please contact [email protected]. PIETRO MASCAGNI LAURITZ MELCHIOR, sensational Wag- nerian tenor of the Metropolitan Opera Company, recently celebrated his twen- tieth anniversary with the organization. To commemorate the occasion a gala concert was arranged, in which a num- ber of his colleagues joined Mr. Melchior in singing excerpts from three of the Wagner operas. Following the concert there was a back-stage ceremony, in which all departments of the Metropol- itan, from the board of directors to the stage hands, joined in paying tribute to the distinguished tenor. AN INTERNATIONAL music festival will take place in Prague, Czechoslovakia, from May 11 to 31, in commemoration of the fiftieth birthday of the Czech Phil- harmonic Orchestra. Leonard Bernstein, composer, conductor; Samuel Barber, composer; and Eugene List, pianist, will attend, representing the U.S. cured free upon request to the National THE RESTORED Co- and Inter-American Music Week Com- lonial city of Williams- BERNARD ROGERS’ mittee, 315 Fourth Avenue, New York 10. -
Hearst Corporation Los Angeles Examiner Photographs, Negatives and Clippings--Portrait Files (A-F) 7000.1A
http://oac.cdlib.org/findaid/ark:/13030/c84j0chj No online items Hearst Corporation Los Angeles Examiner photographs, negatives and clippings--portrait files (A-F) 7000.1a Finding aid prepared by Rebecca Hirsch. Data entry done by Nick Hazelton, Rachel Jordan, Siria Meza, Megan Sallabedra, and Vivian Yan The processing of this collection and the creation of this finding aid was funded by the generous support of the Council on Library and Information Resources. USC Libraries Special Collections Doheny Memorial Library 206 3550 Trousdale Parkway Los Angeles, California, 90089-0189 213-740-5900 [email protected] 2012 April 7000.1a 1 Title: Hearst Corporation Los Angeles Examiner photographs, negatives and clippings--portrait files (A-F) Collection number: 7000.1a Contributing Institution: USC Libraries Special Collections Language of Material: English Physical Description: 833.75 linear ft.1997 boxes Date (bulk): Bulk, 1930-1959 Date (inclusive): 1903-1961 Abstract: This finding aid is for letters A-F of portrait files of the Los Angeles Examiner photograph morgue. The finding aid for letters G-M is available at http://www.usc.edu/libraries/finding_aids/records/finding_aid.php?fa=7000.1b . The finding aid for letters N-Z is available at http://www.usc.edu/libraries/finding_aids/records/finding_aid.php?fa=7000.1c . creator: Hearst Corporation. Arrangement The photographic morgue of the Hearst newspaper the Los Angeles Examiner consists of the photographic print and negative files maintained by the newspaper from its inception in 1903 until its closing in 1962. It contains approximately 1.4 million prints and negatives. The collection is divided into multiple parts: 7000.1--Portrait files; 7000.2--Subject files; 7000.3--Oversize prints; 7000.4--Negatives. -
Small Solar System Bodies As Granular Media D
Small Solar System Bodies as granular media D. Hestroffer, P. Sanchez, L Staron, A. Campo Bagatin, S. Eggl, W. Losert, N. Murdoch, E. Opsomer, F. Radjai, D. C. Richardson, et al. To cite this version: D. Hestroffer, P. Sanchez, L Staron, A. Campo Bagatin, S. Eggl, et al.. Small Solar System Bodiesas granular media. Astronomy and Astrophysics Review, Springer Verlag, 2019, 27 (1), 10.1007/s00159- 019-0117-5. hal-02342853 HAL Id: hal-02342853 https://hal.archives-ouvertes.fr/hal-02342853 Submitted on 4 Nov 2019 HAL is a multi-disciplinary open access L’archive ouverte pluridisciplinaire HAL, est archive for the deposit and dissemination of sci- destinée au dépôt et à la diffusion de documents entific research documents, whether they are pub- scientifiques de niveau recherche, publiés ou non, lished or not. The documents may come from émanant des établissements d’enseignement et de teaching and research institutions in France or recherche français ou étrangers, des laboratoires abroad, or from public or private research centers. publics ou privés. Astron Astrophys Rev manuscript No. (will be inserted by the editor) Small solar system bodies as granular media D. Hestroffer · P. S´anchez · L. Staron · A. Campo Bagatin · S. Eggl · W. Losert · N. Murdoch · E. Opsomer · F. Radjai · D. C. Richardson · M. Salazar · D. J. Scheeres · S. Schwartz · N. Taberlet · H. Yano Received: date / Accepted: date Made possible by the International Space Science Institute (ISSI, Bern) support to the inter- national team \Asteroids & Self Gravitating Bodies as Granular Systems" D. Hestroffer IMCCE, Paris Observatory, universit´ePSL, CNRS, Sorbonne Universit´e,Univ. -
New Orleans Review LOYOLA UNWERSITY VOLUME 11NUMBER3141$11.00 Cover: "Robert the Iceman" by Lee Crum L ;'O
New Orleans Review LOYOLA UNWERSITY VOLUME 11NUMBER3141$11.00 Cover: "Robert the Iceman" by Lee Crum l ;'O ,·~· New Orleans Review Fall/Winter 1984 Editors John Biguenet, Art and Literature Bruce Henricksen, Theory and Criticism John Mosier, Film, General Editor Managing Editor Sarah Elizabeth Spain Design Vilma Pesciallo Contributing Editor Raymond McGowan Founding Editor Miller Williams Advisory Editors Richard Berg Doris Betts Joseph Fichter, S.J. Dawson Gaillard Alexis Gonzales, F.S.C. John Irwin Wesley Morris Walker Percy Herman Rapaport Robert Scholes Marcus Smith Miller Williams The New Orleans Review is published by Loyola University, New Orleans, Louisiana 70118, United States. Copyright © 1984 by Loyola University. Critical essays relating to film or literature of up to ten thousand words should be prepared to conform with MLA guidelines and sent to the appropriate editor, together with a stamped, self-addressed envelope. The address is New Orleans Review, Box 195, Loyola University, New Orleans, Louisiana 70118. Fiction, poetry, photography or related artwork should be sent to the Art and Literature Editor. A stamped, self-addressed envelope should be enclosed. Reasonable care is taken in the handling of material, but no responsibility is assumed for the loss of unsolicited material. Accepted manuscripts are the property of the NOR. The New Orleans Review is published in February, May, August and November. Annual Subscription Rate: Institutions $25.00, Individuals $20.00, Foreign Subscribers $30.00. Contents listed in the PMLA Bibliography and the Index of American Periodical Verse. US ISSN 0028-6400. CONTENTS POETRY AND FICTION The Other Agency John Bovey 104 Driving Home in Winter Barbara Moore 94 It's Going to Snow. -
Overview and Current Status of Remote Sensing Applications Based on Unmanned Aerial Vehicles (Uavs)
Overview and Current Status of Remote Sensing Applications Based on Unmanned Aerial Vehicles (UAVs) Gonzalo Pajares Abstract Remotely Piloted Aircraft (RPA) is presently in continuous battery or energy system’s capabilities. There are vehicles with development at a rapid pace. Unmanned Aerial Vehicles the ability to fly at medium and high altitudes with flight dura- (UAVs) or more extensively Unmanned Aerial Systems (UAS) tions ranging from minutes to hours, i.e., from five minutes are platforms considered under the RPAs paradigm. Simulta- to 30 hours. The horizontal range of the different platforms neously, the development of sensors and instruments to be is also limited by the power of the communications system, installed onboard such platforms is growing exponentially. which should ensure contact with a ground station, again These two factors together have led to the increasing use of ranging from meters to kilometers. Communications using sat- these platforms and sensors for remote sensing applications ellite input can also be used, expanding the operational range. with new potential. Thus, the overall goal of this paper is There are several different categorizations for unmanned aerial to provide a panoramic overview about the current status platforms depending on the criterion applied (Nonami et al., of remote sensing applications based on unmanned aerial 2010). Perhaps the most extensive and current classifications platforms equipped with a set of specific sensors and instru- can be found in Blyenburgh (2014) with annual revisions. ments. First, some examples of typical platforms used in An auto platform or remotely controlled platform through remote sensing are provided. Second, a description of sensors a remote station together with a communication system, and technologies is explored which are onboard instruments including the corresponding protocol, constitutes what is specifically intended to capture data for remote sensing ap- known an Unmanned Aircraft System (UAS) (Gertler, 2012). -
Convection and Fluidization in Oscillatory Granular Flows: the Role
Eur. Phys. J. E (2015) 38:66 THE EUROPEAN DOI 10.1140/epje/i2015-15066-7 PHYSICAL JOURNAL E Colloquium Convection and fluidization in oscillatory granular flows: The role of acoustic streaming Jose Manuel Valverdea Faculty of Physics, University of Seville, Avenida Reina Mercedes s/n, 41012 Seville, Spain Received 28 January 2015 and Received in final form 13 April 2015 Published online: 30 June 2015 – c EDP Sciences / Societ`a Italiana di Fisica / Springer-Verlag 2015 Abstract. Convection and fluidization phenomena in vibrated granular beds have attracted a strong inter- est from the physics community since the last decade of the past century. As early reported by Faraday, the convective flow of large inertia particles in vibrated beds exhibits enigmatic features such as frictional weakening and the unexpected influence of the interstitial gas. At sufficiently intense vibration intensities surface patterns appear bearing a stunning resemblance with the surface ripples (Faraday waves) observed for low-viscosity liquids, which suggests that the granular bed transits into a liquid-like fluidization regime despite the large inertia of the particles. In his 1831 seminal paper, Faraday described also the development of circulation air currents in the vicinity of vibrating plates. This phenomenon (acoustic streaming) is well known in acoustics and hydrodynamics and occurs whenever energy is dissipated by viscous losses at any oscillating boundary. The main argument of the present paper is that acoustic streaming might develop on the surface of the large inertia particles in the vibrated granular bed. As a consequence, the drag force on the particles subjected to an oscillatory viscous flow is notably enhanced. -
Collection of Original Works of Art on Paper, V.D
http://oac.cdlib.org/findaid/ark:/13030/kt5w1006wm No online items Finding Aid for the Collection of Original Works of Art on Paper, v.d. Processed by UCLA Library Special Collections staff; machine-readable finding aid created by Caroline Cubé UCLA Library Special Collections UCLA Library Special Collections Room A1713, Charles E. Young Research Library Box 951575 Los Angeles, CA 90095-1575 Email: [email protected] URL: http://www.library.ucla.edu/libraries/special/scweb/ © 2003 The Regents of the University of California. All rights reserved. Finding Aid for the Collection of Original 99 1 Original Works of Art on Paper, v.d. Finding Aid for the Collection of Original Works of Art on Paper, v.d. Collection number: Original 99 UCLA Library Special Collections UCLA Library Special Collections staff Los Angeles, CA Contact Information UCLA Library Special Collections staff UCLA Library Special Collections Room A1713, Charles E. Young Research Library Box 951575 Los Angeles, CA 90095-1575 Telephone: 310/825-4988 (10:00 a.m. - 4:45 p.m., Pacific Time) Email: [email protected] URL: http://www.library.ucla.edu/libraries/special/scweb/ Processed by: UCLA Library Special Collections staff Encoded by: Caroline Cubé Online finding aid edited by: Josh Fiala, May 2003 © 2003 The Regents of the University of California. All rights reserved. Descriptive Summary Title: Collection of Original Works of Art on Paper, Date (inclusive): v.d. Collection number: Original 99 Repository: University of California, Los Angeles. Library Special Collections. Los Angeles, California 90095-1575 Physical location: Stored off-site at SRLF. -
Wildland Fire in Ecosystems: Fire and Nonnative Invasive Plants
Alaska (Producer). Available: http://akweeds.uaa.alaska.edu/ References _____________________ akweeds_ranking_page.htm [2005, January 15]. Abella, S. R.; Covington, W. W. 2004. Monitoring an Arizona Albert, M. 2000. Carpobrotus edulis. In: Bossard, C. C.; Randall, J. ponderosa pine restoration: sampling efficiency and multivari- M.; Hoshovsky, M. C., eds. Invasive plants of California’s wildlands. ate analysis of understory vegetation. Restoration Ecology. 12: Berkeley, CA: University of California Press: 90-94. 359-367. Albini F.; Amin, M. R.; Hungerford R. D.; Frandsen W. H.; Ryan, Abella, Scott. R.; MacDonald, Neil. W. 2000. Intense burns may K. C. 1996. Models for fire-driven heat and moisture transport reduce spotted knapweed germination. Ecological Restoration. in soils. Gen. Tech. Rep. INT-GTR-335. Ogden, UT: U.S. Depart- 18(2): 203-205. ment of Agriculture, Forest Service, Intermountain Reasearch Abrahamson, W. G. 1984. Species responses to fire and the Florida Station. 16 p. Lake Wales ridge. American Journal of Botany. 71: 35-43. Alexander, Janice M.; D‘Antonio, Carla M. D. 2003. Seed bank dy- Acker, Steven A. 1992. Wildfire and soil organic carbon in sage- namics of French broom in coastal California grasslands: effects brush-bunchgrass vegetation. The Great Basin Naturalist. 52(3): of stand age and prescribed burning on control and restoration. 284-287. Restoration Ecology. 11(2): 185-197. Adger, Neil; Aggarwal, Pramod; Agrawala, Shardul; [and others]. Alexander, M.; Stefner, C.; Beck, J.; Lanoville, R. 2001. New 2007. Climate Change 2007: impacts, adaptation and vulnerability. insights into the effectiveness of fuel reduction treatments on Contribution of Working Group II to the 4th assessment report of crown fire potential at the stand level.