A User-Friendly Introduction to Lebesgue Measure and Integration
Total Page:16
File Type:pdf, Size:1020Kb
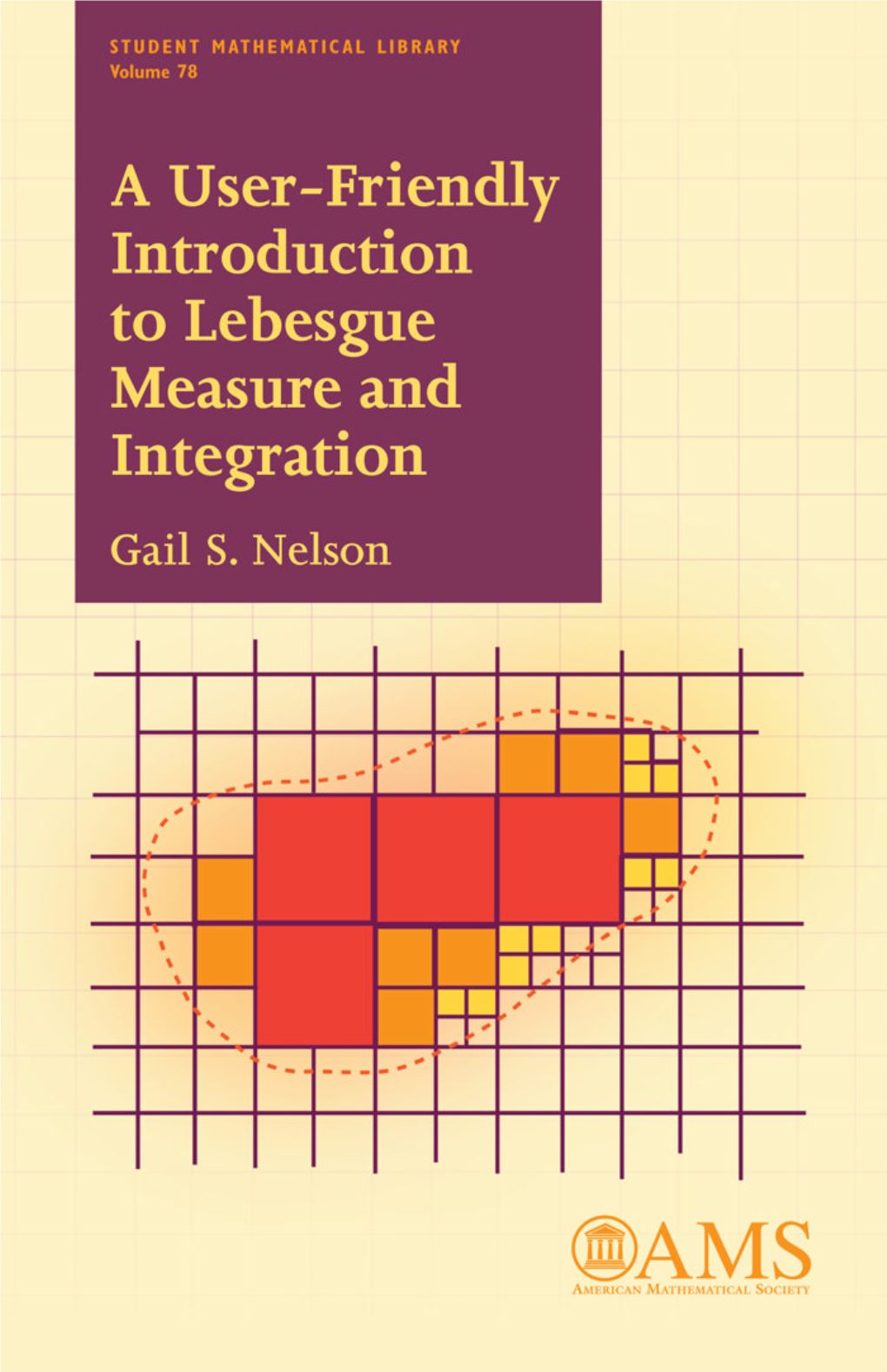
Load more
Recommended publications
-
Set Theory, Including the Axiom of Choice) Plus the Negation of CH
ANNALS OF ~,IATltEMATICAL LOGIC - Volume 2, No. 2 (1970) pp. 143--178 INTERNAL COHEN EXTENSIONS D.A.MARTIN and R.M.SOLOVAY ;Tte RockeJ~'ller University and University (.~t CatiJbrnia. Berkeley Received 2 l)ecemt)er 1969 Introduction Cohen [ !, 2] has shown that tile continuum hypothesis (CH) cannot be proved in Zermelo-Fraenkel set theory. Levy and Solovay [9] have subsequently shown that CH cannot be proved even if one assumes the existence of a measurable cardinal. Their argument in tact shows that no large cardinal axiom of the kind present;y being considered by set theorists can yield a proof of CH (or of its negation, of course). Indeed, many set theorists - including the authors - suspect that C1t is false. But if we reject CH we admit Gurselves to be in a state of ignorance about a great many questions which CH resolves. While CH is a power- full assertion, its negation is in many ways quite weak. Sierpinski [ 1 5 ] deduces propcsitions there called C l - C82 from CH. We know of none of these propositions which is decided by the negation of CH and only one of them (C78) which is decided if one assumes in addition that a measurable cardinal exists. Among the many simple questions easily decided by CH and which cannot be decided in ZF (Zerme!o-Fraenkel set theory, including the axiom of choice) plus the negation of CH are tile following: Is every set of real numbers of cardinality less than tha't of the continuum of Lebesgue measure zero'? Is 2 ~0 < 2 ~ 1 ? Is there a non-trivial measure defined on all sets of real numbers? CIhis third question could be decided in ZF + not CH only in the unlikely event t Tile second author received support from a Sloan Foundation fellowship and tile National Science Foundation Grant (GP-8746). -
Geometric Integration Theory Contents
Steven G. Krantz Harold R. Parks Geometric Integration Theory Contents Preface v 1 Basics 1 1.1 Smooth Functions . 1 1.2Measures.............................. 6 1.2.1 Lebesgue Measure . 11 1.3Integration............................. 14 1.3.1 Measurable Functions . 14 1.3.2 The Integral . 17 1.3.3 Lebesgue Spaces . 23 1.3.4 Product Measures and the Fubini–Tonelli Theorem . 25 1.4 The Exterior Algebra . 27 1.5 The Hausdorff Distance and Steiner Symmetrization . 30 1.6 Borel and Suslin Sets . 41 2 Carath´eodory’s Construction and Lower-Dimensional Mea- sures 53 2.1 The Basic Definition . 53 2.1.1 Hausdorff Measure and Spherical Measure . 55 2.1.2 A Measure Based on Parallelepipeds . 57 2.1.3 Projections and Convexity . 57 2.1.4 Other Geometric Measures . 59 2.1.5 Summary . 61 2.2 The Densities of a Measure . 64 2.3 A One-Dimensional Example . 66 2.4 Carath´eodory’s Construction and Mappings . 67 2.5 The Concept of Hausdorff Dimension . 70 2.6 Some Cantor Set Examples . 73 i ii CONTENTS 2.6.1 Basic Examples . 73 2.6.2 Some Generalized Cantor Sets . 76 2.6.3 Cantor Sets in Higher Dimensions . 78 3 Invariant Measures and the Construction of Haar Measure 81 3.1 The Fundamental Theorem . 82 3.2 Haar Measure for the Orthogonal Group and the Grassmanian 90 3.2.1 Remarks on the Manifold Structure of G(N,M).... 94 4 Covering Theorems and the Differentiation of Integrals 97 4.1 Wiener’s Covering Lemma and its Variants . -
The Fundamental Theorem of Calculus for Lebesgue Integral
Divulgaciones Matem´aticasVol. 8 No. 1 (2000), pp. 75{85 The Fundamental Theorem of Calculus for Lebesgue Integral El Teorema Fundamental del C´alculo para la Integral de Lebesgue Di´omedesB´arcenas([email protected]) Departamento de Matem´aticas.Facultad de Ciencias. Universidad de los Andes. M´erida.Venezuela. Abstract In this paper we prove the Theorem announced in the title with- out using Vitali's Covering Lemma and have as a consequence of this approach the equivalence of this theorem with that which states that absolutely continuous functions with zero derivative almost everywhere are constant. We also prove that the decomposition of a bounded vari- ation function is unique up to a constant. Key words and phrases: Radon-Nikodym Theorem, Fundamental Theorem of Calculus, Vitali's covering Lemma. Resumen En este art´ıculose demuestra el Teorema Fundamental del C´alculo para la integral de Lebesgue sin usar el Lema del cubrimiento de Vi- tali, obteni´endosecomo consecuencia que dicho teorema es equivalente al que afirma que toda funci´onabsolutamente continua con derivada igual a cero en casi todo punto es constante. Tambi´ense prueba que la descomposici´onde una funci´onde variaci´onacotada es ´unicaa menos de una constante. Palabras y frases clave: Teorema de Radon-Nikodym, Teorema Fun- damental del C´alculo,Lema del cubrimiento de Vitali. Received: 1999/08/18. Revised: 2000/02/24. Accepted: 2000/03/01. MSC (1991): 26A24, 28A15. Supported by C.D.C.H.T-U.L.A under project C-840-97. 76 Di´omedesB´arcenas 1 Introduction The Fundamental Theorem of Calculus for Lebesgue Integral states that: A function f :[a; b] R is absolutely continuous if and only if it is ! 1 differentiable almost everywhere, its derivative f 0 L [a; b] and, for each t [a; b], 2 2 t f(t) = f(a) + f 0(s)ds: Za This theorem is extremely important in Lebesgue integration Theory and several ways of proving it are found in classical Real Analysis. -
Shape Analysis, Lebesgue Integration and Absolute Continuity Connections
NISTIR 8217 Shape Analysis, Lebesgue Integration and Absolute Continuity Connections Javier Bernal This publication is available free of charge from: https://doi.org/10.6028/NIST.IR.8217 NISTIR 8217 Shape Analysis, Lebesgue Integration and Absolute Continuity Connections Javier Bernal Applied and Computational Mathematics Division Information Technology Laboratory This publication is available free of charge from: https://doi.org/10.6028/NIST.IR.8217 July 2018 INCLUDES UPDATES AS OF 07-18-2018; SEE APPENDIX U.S. Department of Commerce Wilbur L. Ross, Jr., Secretary National Institute of Standards and Technology Walter Copan, NIST Director and Undersecretary of Commerce for Standards and Technology ______________________________________________________________________________________________________ This Shape Analysis, Lebesgue Integration and publication Absolute Continuity Connections Javier Bernal is National Institute of Standards and Technology, available Gaithersburg, MD 20899, USA free of Abstract charge As shape analysis of the form presented in Srivastava and Klassen’s textbook “Functional and Shape Data Analysis” is intricately related to Lebesgue integration and absolute continuity, it is advantageous from: to have a good grasp of the latter two notions. Accordingly, in these notes we review basic concepts and results about Lebesgue integration https://doi.org/10.6028/NIST.IR.8217 and absolute continuity. In particular, we review fundamental results connecting them to each other and to the kind of shape analysis, or more generally, functional data analysis presented in the aforeme- tioned textbook, in the process shedding light on important aspects of all three notions. Many well-known results, especially most results about Lebesgue integration and some results about absolute conti- nuity, are presented without proofs. -
An Introduction to Measure Theory Terence
An introduction to measure theory Terence Tao Department of Mathematics, UCLA, Los Angeles, CA 90095 E-mail address: [email protected] To Garth Gaudry, who set me on the road; To my family, for their constant support; And to the readers of my blog, for their feedback and contributions. Contents Preface ix Notation x Acknowledgments xvi Chapter 1. Measure theory 1 x1.1. Prologue: The problem of measure 2 x1.2. Lebesgue measure 17 x1.3. The Lebesgue integral 46 x1.4. Abstract measure spaces 79 x1.5. Modes of convergence 114 x1.6. Differentiation theorems 131 x1.7. Outer measures, pre-measures, and product measures 179 Chapter 2. Related articles 209 x2.1. Problem solving strategies 210 x2.2. The Radamacher differentiation theorem 226 x2.3. Probability spaces 232 x2.4. Infinite product spaces and the Kolmogorov extension theorem 235 Bibliography 243 vii viii Contents Index 245 Preface In the fall of 2010, I taught an introductory one-quarter course on graduate real analysis, focusing in particular on the basics of mea- sure and integration theory, both in Euclidean spaces and in abstract measure spaces. This text is based on my lecture notes of that course, which are also available online on my blog terrytao.wordpress.com, together with some supplementary material, such as a section on prob- lem solving strategies in real analysis (Section 2.1) which evolved from discussions with my students. This text is intended to form a prequel to my graduate text [Ta2010] (henceforth referred to as An epsilon of room, Vol. I ), which is an introduction to the analysis of Hilbert and Banach spaces (such as Lp and Sobolev spaces), point-set topology, and related top- ics such as Fourier analysis and the theory of distributions; together, they serve as a text for a complete first-year graduate course in real analysis. -
Hausdorff Measure
Hausdorff Measure Jimmy Briggs and Tim Tyree December 3, 2016 1 1 Introduction In this report, we explore the the measurement of arbitrary subsets of the metric space (X; ρ); a topological space X along with its distance function ρ. We introduce Hausdorff Measure as a natural way of assigning sizes to these sets, especially those of smaller \dimension" than X: After an exploration of the salient properties of Hausdorff Measure, we proceed to a definition of Hausdorff dimension, a separate idea of size that allows us a more robust comparison between rough subsets of X. Many of the theorems in this report will be summarized in a proof sketch or shown by visual example. For a more rigorous treatment of the same material, we redirect the reader to Gerald B. Folland's Real Analysis: Modern techniques and their applications. Chapter 11 of the 1999 edition served as our primary reference. 2 Hausdorff Measure 2.1 Measuring low-dimensional subsets of X The need for Hausdorff Measure arises from the need to know the size of lower-dimensional subsets of a metric space. This idea is not as exotic as it may sound. In a high school Geometry course, we learn formulas for objects of various dimension embedded in R3: In Figure 1 we see the line segment, the circle, and the sphere, each with it's own idea of size. We call these length, area, and volume, respectively. Figure 1: low-dimensional subsets of R3: 2 4 3 2r πr 3 πr Note that while only volume measures something of the same dimension as the host space, R3; length, and area can still be of interest to us, especially 2 in applications. -
Measure, Integral and Probability
Marek Capinski´ and Ekkehard Kopp Measure, Integral and Probability Springer-Verlag Berlin Heidelberg NewYork London Paris Tokyo Hong Kong Barcelona Budapest To our children; grandchildren: Piotr, Maciej, Jan, Anna; Luk asz Anna, Emily Preface The central concepts in this book are Lebesgue measure and the Lebesgue integral. Their role as standard fare in UK undergraduate mathematics courses is not wholly secure; yet they provide the principal model for the development of the abstract measure spaces which underpin modern probability theory, while the Lebesgue function spaces remain the main source of examples on which to test the methods of functional analysis and its many applications, such as Fourier analysis and the theory of partial differential equations. It follows that not only budding analysts have need of a clear understanding of the construction and properties of measures and integrals, but also that those who wish to contribute seriously to the applications of analytical methods in a wide variety of areas of mathematics, physics, electronics, engineering and, most recently, finance, need to study the underlying theory with some care. We have found remarkably few texts in the current literature which aim explicitly to provide for these needs, at a level accessible to current under- graduates. There are many good books on modern probability theory, and increasingly they recognize the need for a strong grounding in the tools we develop in this book, but all too often the treatment is either too advanced for an undergraduate audience or else somewhat perfunctory. We hope therefore that the current text will not be regarded as one which fills a much-needed gap in the literature! One fundamental decision in developing a treatment of integration is whether to begin with measures or integrals, i.e. -
The Axiom of Choice and Its Implications
THE AXIOM OF CHOICE AND ITS IMPLICATIONS KEVIN BARNUM Abstract. In this paper we will look at the Axiom of Choice and some of the various implications it has. These implications include a number of equivalent statements, and also some less accepted ideas. The proofs discussed will give us an idea of why the Axiom of Choice is so powerful, but also so controversial. Contents 1. Introduction 1 2. The Axiom of Choice and Its Equivalents 1 2.1. The Axiom of Choice and its Well-known Equivalents 1 2.2. Some Other Less Well-known Equivalents of the Axiom of Choice 3 3. Applications of the Axiom of Choice 5 3.1. Equivalence Between The Axiom of Choice and the Claim that Every Vector Space has a Basis 5 3.2. Some More Applications of the Axiom of Choice 6 4. Controversial Results 10 Acknowledgments 11 References 11 1. Introduction The Axiom of Choice states that for any family of nonempty disjoint sets, there exists a set that consists of exactly one element from each element of the family. It seems strange at first that such an innocuous sounding idea can be so powerful and controversial, but it certainly is both. To understand why, we will start by looking at some statements that are equivalent to the axiom of choice. Many of these equivalences are very useful, and we devote much time to one, namely, that every vector space has a basis. We go on from there to see a few more applications of the Axiom of Choice and its equivalents, and finish by looking at some of the reasons why the Axiom of Choice is so controversial. -
Computing the Bayes Factor from a Markov Chain Monte Carlo
Computing the Bayes Factor from a Markov chain Monte Carlo Simulation of the Posterior Distribution Martin D. Weinberg∗ Department of Astronomy University of Massachusetts, Amherst, USA Abstract Computation of the marginal likelihood from a simulated posterior distribution is central to Bayesian model selection but is computationally difficult. The often-used harmonic mean approximation uses the posterior directly but is unstably sensitive to samples with anomalously small values of the likelihood. The Laplace approximation is stable but makes strong, and of- ten inappropriate, assumptions about the shape of the posterior distribution. It is useful, but not general. We need algorithms that apply to general distributions, like the harmonic mean approximation, but do not suffer from convergence and instability issues. Here, I argue that the marginal likelihood can be reliably computed from a posterior sample by careful attention to the numerics of the probability integral. Posing the expression for the marginal likelihood as a Lebesgue integral, we may convert the harmonic mean approximation from a sample statistic to a quadrature rule. As a quadrature, the harmonic mean approximation suffers from enor- mous truncation error as consequence . This error is a direct consequence of poor coverage of the sample space; the posterior sample required for accurate computation of the marginal likelihood is much larger than that required to characterize the posterior distribution when us- ing the harmonic mean approximation. In addition, I demonstrate that the integral expression for the harmonic-mean approximation converges slowly at best for high-dimensional problems with uninformative prior distributions. These observations lead to two computationally-modest families of quadrature algorithms that use the full generality sample posterior but without the instability. -
(Measure Theory for Dummies) UWEE Technical Report Number UWEETR-2006-0008
A Measure Theory Tutorial (Measure Theory for Dummies) Maya R. Gupta {gupta}@ee.washington.edu Dept of EE, University of Washington Seattle WA, 98195-2500 UWEE Technical Report Number UWEETR-2006-0008 May 2006 Department of Electrical Engineering University of Washington Box 352500 Seattle, Washington 98195-2500 PHN: (206) 543-2150 FAX: (206) 543-3842 URL: http://www.ee.washington.edu A Measure Theory Tutorial (Measure Theory for Dummies) Maya R. Gupta {gupta}@ee.washington.edu Dept of EE, University of Washington Seattle WA, 98195-2500 University of Washington, Dept. of EE, UWEETR-2006-0008 May 2006 Abstract This tutorial is an informal introduction to measure theory for people who are interested in reading papers that use measure theory. The tutorial assumes one has had at least a year of college-level calculus, some graduate level exposure to random processes, and familiarity with terms like “closed” and “open.” The focus is on the terms and ideas relevant to applied probability and information theory. There are no proofs and no exercises. Measure theory is a bit like grammar, many people communicate clearly without worrying about all the details, but the details do exist and for good reasons. There are a number of great texts that do measure theory justice. This is not one of them. Rather this is a hack way to get the basic ideas down so you can read through research papers and follow what’s going on. Hopefully, you’ll get curious and excited enough about the details to check out some of the references for a deeper understanding. -
Measure Theory and Probability
Measure theory and probability Alexander Grigoryan University of Bielefeld Lecture Notes, October 2007 - February 2008 Contents 1 Construction of measures 3 1.1Introductionandexamples........................... 3 1.2 σ-additive measures ............................... 5 1.3 An example of using probability theory . .................. 7 1.4Extensionofmeasurefromsemi-ringtoaring................ 8 1.5 Extension of measure to a σ-algebra...................... 11 1.5.1 σ-rings and σ-algebras......................... 11 1.5.2 Outermeasure............................. 13 1.5.3 Symmetric difference.......................... 14 1.5.4 Measurable sets . ............................ 16 1.6 σ-finitemeasures................................ 20 1.7Nullsets..................................... 23 1.8 Lebesgue measure in Rn ............................ 25 1.8.1 Productmeasure............................ 25 1.8.2 Construction of measure in Rn. .................... 26 1.9 Probability spaces ................................ 28 1.10 Independence . ................................. 29 2 Integration 38 2.1 Measurable functions.............................. 38 2.2Sequencesofmeasurablefunctions....................... 42 2.3 The Lebesgue integral for finitemeasures................... 47 2.3.1 Simplefunctions............................ 47 2.3.2 Positivemeasurablefunctions..................... 49 2.3.3 Integrablefunctions........................... 52 2.4Integrationoversubsets............................ 56 2.5 The Lebesgue integral for σ-finitemeasure................. -
A Measure Blowup Sam Chow∗∗∗, Gus Schrader∗∗∗∗ and J.J
A measure blowup Sam Chow∗∗∗, Gus Schrader∗∗∗∗ and J.J. Koliha∗ ∗∗∗∗ Last year Sam Chow and Gus Schrader were third-year students at the Uni- versity of Melbourne enrolled in Linear Analysis, a subject involving — among other topics — Lebesgue measure and integration. This was based on parts of the recently published book Metrics, Norms and Integrals by the lecturer in the subject, Associate Professor Jerry Koliha. In addition to three lectures a week, the subject had a weekly practice class. The lecturer introduced an extra weekly practice class with a voluntary attendance, but most stu- dents chose to attend, showing a keen interest in the subject. The problems tackled in the extra practice class ranged from routine to very challenging. The present article arose in this environment, as Sam and Gus independently attacked one of the challenging problems. Working in the Euclidean space Rd we can characterise Lebesgue measure very simply by relying on the Euclidean volume of the so-called d-cells.Ad-cell C in Rd is the Cartesian product C = I1 ×···×Id of bounded nondegenerate one-dimensional intervals Ik; its Euclidean d-volume is d the product of the lengths of the Ik. Borel sets in R are the members of the least family Bd of subsets of Rd containing the empty set, ∅, and all d-cells which are closed under countable unions and complements in Rd. Since every open set in Rd can be expressed as the countable union of suitable d-cells, the open sets are Borel, as are all sets obtained from them by countable unions and intersections, and complements.