Assessment of Mildew Levels in Wheat Samples Based on Spectral Characteristics of Bulk Grains Muhammad A
Total Page:16
File Type:pdf, Size:1020Kb
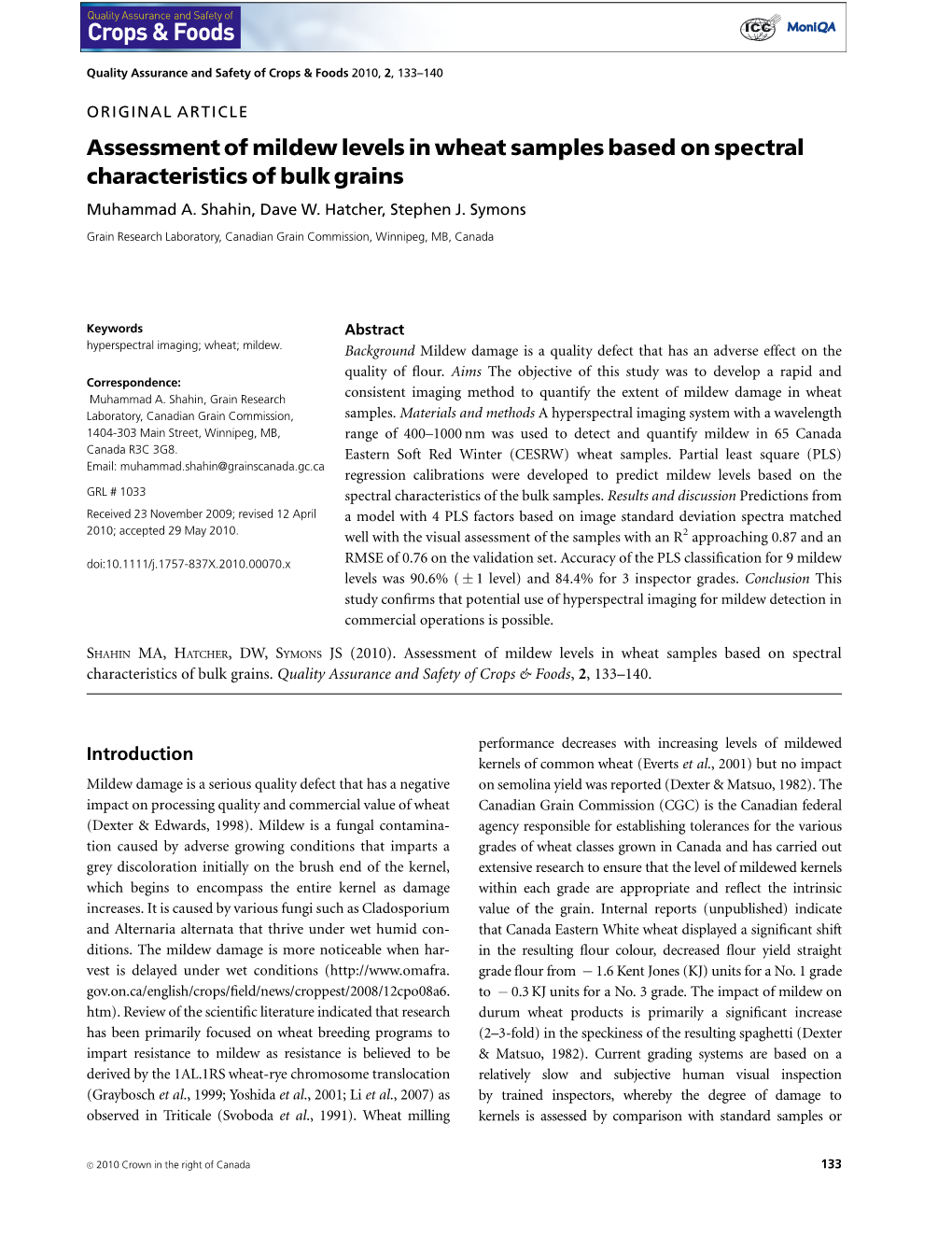
Load more
Recommended publications
-
Meeting Report the Aaronsohn-ITMI International Conference
Israel Journal of Plant Sciences Vol. 55 2007 pp. 315–319 Meeting Report The Aaronsohn-ITMI International Conference CATHERINE FEUILLET,a PETER LANGRIDGE,b AND ROBBIE WAUGHc aUMR INRA-UBP 1095, Amélioration et Santé des Plantes, Domaine de Crouelle, 234 Avenue du Brézet, 63100 Clermont-Ferrand, France bAustralian Centre for Plant Functional Genomics, University of Adelaide, SA 5064, Australia cScottish Crop Research Institute, Invergowrie, DD2 5DA, UK The Aaronsohn-ITMI international conference was held 16–20 April 2007 in Tiberias (Israel) to celebrate the 100 year anniversary of the rediscovery of wild emmer wheat by Aaron Aaronsohn in Rosh Pinna, a few kilometers away from Tiberias. The workshop, attended by more than 110 participants from 22 countries, also provided an opportunity to discuss the latest developments in Triticeae genetics and genomics. WILD EMMER WHEAT programs as a source of new alleles. Wild emmer wheat also represents a key material for studying the evolution In the opening session of the workshop, Shaul Katz (He- of wheat genomes and the impact of polyploidization brew Univ., Israel) reported on the history of the discov- on genome structure and evolution, as exemplified ery of wild emmer wheat, the wild ancestor of tetraploid by Moshe Feldman (Weizman Institute, Israel) in his wheat. This goes back to 1855 when botanists (Kotschy, lecture. There is genetic asymmetry between the A and Koernicke) from Austria came to Palestine and found a B genomes of wild emmer wheat. For example, genes sample of a cereal with ancestral features (“Urweizen”) controlling inflorescence morphology, growth habit, do- that had not been previously recorded. -
Wild Wheat to Productive Drylands: Global Scientific Practice and the Agroecological Remaking of Palestine
Geoforum 78 (2017) 43–51 Contents lists available at ScienceDirect Geoforum journal homepage: www.elsevier.com/locate/geoforum Wild wheat to productive drylands: Global scientific practice and the agroecological remaking of Palestine Omar Tesdell Department of Geography, Birzeit University, PO Box 14, Birzeit, West Bank, Palestine article info abstract Article history: This paper traces how scientific research on wheat (Triticum) worked to establish Palestine as a region Received 23 May 2016 sought for colonization. Recent work in geography has refined our understanding of agricultural expan- Received in revised form 16 November 2016 sion as an outcome of colonization, however, this work leaves the place-making capacity of agricultural Accepted 18 November 2016 research largely unexplored. My claim is that rather than a byproduct of colonization, wheat research served to remake Palestine as a biophysical region in need of improvement and colonization. I show how a shift in the plant sciences from research in taxonomy to plant breeding corresponded to an Keywords: agro-climatic shift on Palestine from an undesirable, arid region to a promising dryland agricultural Agro-climatology region. In this way, wheat research drew Palestine and the United States into a wider effort to transform Agro-ecology Colonization arid areas into agricultural drylands. Drawing on a previously unexplored episode of technical coopera- Palestine tion between researchers in the United States and Palestine, I argue that we must examine how wildness, United States native-ness, and agro-climatic suitability are scientifically constituted within and not apart from colonial Drylands conquest. In doing so, the paper calls for reconsideration within geography and political ecology of the place-making relationship between colonization and scientific practice. -
One Thousand Objects for the Microscope
WITH ILLUSTRATIONS Frederick Warne and Co., Publishers, ._t_ ~l S GTl It H{ FI T HIC EST LIBER MEUS, testES EST DEUS; SI QUIS ME QUERIT .A% HIC NOMEN ERIT. - . a>V T /. 13 I 5 ing, irlllU 11/A 111 UlUiig, *-r j .. __ Notice.—This completely New Poultry Book is the only one the Author has fully written ; all others bearing her name being only edited by her, and much anterior to this volume. Bedford Street, Strand. Frederick Warne and Co., Publishers, WARNE’S USEFUL BOOKS-Continued. Fcap. 8vo, Is. each, boards, with Practical Illustrations. Angling, and How to Angle. A Practical Guide to Bottom-Fishing, Trolling, Spinning, Fly-Fishing, and Sea-Fishing. By J. T. Burgess. Illustrated. “An excellent little volume, and full of advice the angler will treasure."— Sunday Times. “ A practical and handy guide.’’—Spectator. The Common Sea-Weeds of the British Coast and Channel Islands. With some Insight into the Microscopic Beauties of their Structure and Fructification. By Mrs. L. Lane Clarke. With Original Plates printed in Tinted Litho. “This portable cheap little manual will serve as an admirable introduction to the study of sea-weeds.”—Field. The Common Shells of the Sea-Shore. By the Rev. J. G. Wood. “ The book is so copiously illustrated, that it is impossible to find a shell which cannot be identified by reference to the engravings.”—Vide Preface. “It would be difficult to select a more pleasant sea-side companion than this.” —Observer. The Dog : Its Varieties and Management in Health and Disease. Bird-Keeping : A Practical Guide for the Management of Cage Birds. -
Food Processing and Breeding Strategies for Coeliac-Safe and Healthy Wheat Products T
Food Research International 110 (2018) 11–21 Contents lists available at ScienceDirect Food Research International journal homepage: www.elsevier.com/locate/foodres Food processing and breeding strategies for coeliac-safe and healthy wheat products T Aurélie Jouanina,b, Luud J.W.J. Gilissena, Lesley A. Boydb, James Cockramb, Fiona J. Leighb, Emma J. Wallingtonb, Hetty C. van den Broecka, Ingrid M. van der Meera, Jan G. Schaarta, ⁎ Richard G.F. Vissera, Marinus J.M. Smuldersa, a Wageningen University & Research, Wageningen, The Netherlands b NIAB, Cambridge CB3 0LE, UK ARTICLE INFO ABSTRACT Keywords: A strict gluten-free diet is currently the only treatment for the 1–2% of the world population who suffer from Gluten coeliac disease (CD). However, due to the presence of wheat and wheat derivatives in many food products, Gliadin avoiding gluten consumption is difficult. Gluten-free products, made without wheat, barley or rye, typically T cell epitope require the inclusion of numerous additives, resulting in products that are often less healthy than gluten-based Coeliac disease equivalents. Here, we present and discuss two broad approaches to decrease wheat gluten immunogenicity for Plant breeding CD patients. The first approach is based on food processing strategies, which aim to remove gliadins or all gluten Food processing fi Mutation breeding from edible products. We nd that several of the candidate food processing techniques to produce low gluten- Genome editing immunogenic products from wheat already exist. The second approach focuses on wheat breeding strategies to remove immunogenic epitopes from the gluten proteins, while maintaining their food-processing properties. A combination of breeding strategies, including mutation breeding and possibly genome editing, will be necessary to produce coeliac-safe wheat. -
IPM Update/Fungicide Resistance
IPM Update/Fungicide resistance Neil Havis, Crop Protection Lead, SRUC Introduction 2019 – temperature up on the 30 year average Introduction 2019 rainfall – wet summer (esp May and August) following a near average spring (march figures were higher than average) Introduction 2019 rainfall – wet summer- late attack from splash borne diseases Introduction 2019 sunshine – normal service resumed IPM • Work is ongoing to develop effective, sustainable and profitable IPM programmes in barley • Various projects funded by RESAS, AHDB Mains of Loirston and commercial sponsors https://voluntaryinitiative.org.uk/schemes/integrated-pest-management/ Winter barley IPM: Sow date*Cv*Fungicide • Late sown crops sown 3-4 weeks later than early sown. • 2 Varieties: Surge (res), Tower (sus) • 4 fungicide programmes: 0/1/2/3 sprays 10.5 • Greater fungicide requirement in 10 early sown + susceptible crops 9.5 9 8.5 8 Medium2 spray programme 7.5 Untreated Yield t/ha Yield Untreated 7 6.5 6 EarlyEarly LateLate EarlyEarly LateLate sown sown sown sown Surge Tower Winter barley IPM: Sow date*Cv*Fungicide 16 • Early sowing decreases GLA in disease 14 years. 12 10 • Due to RLS (both years) and also tan spot EarlyEarly sown 1 % 8 - 2019. F 6 LateLate sown 4 RLS • Susceptible variety= less GLA, more 2 disease. 0 Medium UntreatedUntreated 120 2 spray prog. 100 10 80 8 1 - F 60 EarlyEarly sown 6 40 EarlyEarly sown GLA LateLate sown % 20 4 LateLate sown Tan Spot % Tan 0 - 2 Surge Tower Surge Tower 2019 0 2018 2019 Surge Tower Fungicide treatments for all trials Trt T0 -
POWDERY MILDEW RESISTANCE of the LITHUANIAN WINTER WHEAT BREEDING MATERIAL Þilvinas Liatukas* and Vytautas Ruzgas**
PROCEEDINGS OF THE LATVIAN ACADEMY OF SCIENCES. Section B, Vol. 63 (2009), No. 1/2 (660/661), pp. 37–44. DOI: 10.2478/v10046-009-0013-x POWDERY MILDEW RESISTANCE OF THE LITHUANIAN WINTER WHEAT BREEDING MATERIAL Þilvinas Liatukas* and Vytautas Ruzgas** * Lithuanian Institute of Agriculture, Instituto al.1, Akademija, Këdainiai district, LT-58344, LITHUANIA; E-mail: [email protected] ** Lithuanian University of Agriculture, Akademija, Studentø str. 11, Kaunas district, LT-53361, LITHUANIA; E-mail: [email protected] Communicated by Îzaks Raðals At the Lithuanian Institute of Agriculture, during 2004–2006, resistance to powdery mildew of ap- proximately 1,500 winter wheat lines was assessed in check and competitive trial nurseries. Our experimental evidence showed that there were no genotypes with effective resistance single- genes among the lines tested. Effective powdery mildew resistance from start to end of vegeta- tion season depended on the quantitative resistance level. The most resistant lines were evalu- ated with a score of 2 and area under the disease progress curve (AUDPC) values ranging between 1.0–5.4. The most susceptible genotypes from the collection nurseries had score 8–9 and AUDPC values ranging between 1350–2220. The correlations between maximal disease se- verity and AUDPC values were strong (r = 0.79–0.92). Genotypes with AUDPC values up to 10 represented 93 lines or 7% in the check trial nursery and 22 lines or 9% in the competitive trial nursery. Lines evaluated with a score 4–5 and AUDPC value 100–300 dominated in 2004. In the next year the dominant genotypes had resistance scores 3–4 and AUDPC value 50–200. -
A Brief Handbook of the Diseases of Cultivated Plants in Ohio
Cornell "University OF THE flew jporfe State (Tolleoe of Horiculture \^.^r23.a Cornell University Library SB 624.03S4 diseases of cult A brief handbook of the Cornell University Library The original of this book is in the Cornell University Library. There are no known copyright restrictions in the United States on the use of the text. http://www.archive.org/details/cu31924003214529 BULLETIN OF THE Ohio Agricultural Experiment Station Number 214 ' March, 1910. A BRIEF HANDBOOK OF THE DISEASES OF CULTIVATED PLANTS IN OHIO By A. D. SELBY INTRODUCTION The idea of disease is not a simple one, though it may seem so before trying to define it. In reality the term "disease" as applied to plants, means any change in that plant toward reduced vigor, etc., from the ordinary or average behavior. To put it another way, a plant is said to be "diseased" when it shows any deviation from the ordinary or average behavior of that plant in respect to appearance, growth, color of bark, foliage, fruitfulness, time of dropping leaves or length of life; in short, when the plant fails to conform to those averages which we have established by extended observation for the species and variety in question, we say it is diseased. Under such a general definition, variegated or purple hued sports would be included, although potentially rather than actually in diminished succumb easily to parasitic attack and, as - vigor. Variegated sports later investigations show, are really suffering from enzymatic troubles. The more usual symptoms of disease are marked by evident curled or differences in the plant. -
Supporting Your Success Science Highlights 2015-17
Grains Research and Development Supporting your success Science Highlights 2015-17 - 4 - Important disclaimer The Chief Executive Officer of the Department of Agriculture and Food and the State of Western Australia accept no liability whatsoever by reason of negligence or otherwise arising from the use or release of this information or any part of it. Copyright Copyright © Western Australian Agriculture Authority, 2017 Western Australian Government materials, including website pages, documents, images and recordings, are protected by copyright law. Copyright of materials created by or for the Department of Agriculture and Food resides with the Western Australian Agriculture Authority established under the Biosecurity and Agriculture Management Act 2007. Apart from any fair dealing for the purposes of private study, research, criticism or review, as permitted under the provisions of the Copyright Act 1968, no part may be reproduced or reused for any commercial purposes whatsoever without prior written permission of the Western Australian Agriculture Authority. The department will recognise and protect the right of authors and creators as required under the Copyright Act 1968. Accessibility Copies of this document may be available in alternative formats upon request. 3 Baron-Hay Court, South Perth WA 6151 Tel: +61 (0)8 9368 3333 Email: [email protected] agric.wa.gov.au 5 Forward and Royalties for Regions Investment in science and industry development 1 key to profitable agrifood sector.......................................................................................................................... -
Septoria Leaf Spot and Glume Blotch of Wheat
Journal of the Department of Agriculture, Western Australia, Series 4 Volume 7 Number 4 1966 Article 6 1-1-1966 Septoria leaf spot and glume blotch of wheat W A. Shipton Follow this and additional works at: https://researchlibrary.agric.wa.gov.au/journal_agriculture4 Part of the Agronomy and Crop Sciences Commons, and the Plant Pathology Commons Recommended Citation Shipton, W A. (1966) "Septoria leaf spot and glume blotch of wheat," Journal of the Department of Agriculture, Western Australia, Series 4: Vol. 7 : No. 4 , Article 6. Available at: https://researchlibrary.agric.wa.gov.au/journal_agriculture4/vol7/iss4/6 This article is brought to you for free and open access by Research Library. It has been accepted for inclusion in Journal of the Department of Agriculture, Western Australia, Series 4 by an authorized administrator of Research Library. For more information, please contact [email protected], [email protected], [email protected]. PLANT DISEASES SEPTORIA LEAF SPOT AND GLUME BLOTCH OF WHEAT By W. A. SHIPTON, Ph.D., Plant Pathologist SEPTORIA leaf spot and glume blotch of wheat occur throughout Australia and are cap able of reducing crop yields. While no estimates of yield losses are yet available in Western Australia both diseases can cause serious damage to crops. Septoria leaf spot is caused by the fun Environmental Conditions Favouring the gus Septoria tritici Rob. ex Desm. and Disease glume blotch by the related Septoria Infection may take place over a wide nodorum Berk. range of temperatures. The disease is Glume blotch and Septoria leaf spot usually conspicuous in cool to mild, wet were first recorded in Western Australia conditions, but may occur in warmer in 1905 and 1924 respectively (Sutton, 1920; weather provided that there is sufficient Carne and Campbell, 1924), and now occur moisture for infection. -
Evaluation of D-Genome Synthetic Hexaploid Wheats and Advanced Derivatives for Powdery Mildew Resistance
Pak. J. Bot., 49(2): 735-743, 2017. EVALUATION OF D-GENOME SYNTHETIC HEXAPLOID WHEATS AND ADVANCED DERIVATIVES FOR POWDERY MILDEW RESISTANCE KHOLA RAFIQUE1, CHAUDHARY ABDUL RAUF1, ALVINA GUL2, HADI BUX3*, AHMAD ALI4, RABIA ASMA MEMON3, SUMAIRA FARRAKH5 AND ABDUL MUJEEB-KAZI6,7 1Department of Plant Pathology, PMAS Arid Agriculture University Rawalpindi, Pakistan 2Atta-ur-Rahman School of Applied Biosciences (ASAB), National University of Sciences and Technology (NUST), Islamabad, Pakistan 3Institute of Plant Sciences, University of Sindh, Jamshoro, Pakistan 4Center of Plant Sciences and Biodiversity, University of Swat, Pakistan 5Department of Biosciences, COMSATS Institute of Information Technology, Islamabad, Pakistan6 6Department of Botany, University of Sargodha, Sargodha, Pakistan 7Texas A&M University, Texas, USA *Corresponding author: [email protected] Abstract The present study was undertaken to characterize 32 D-genome synthetic hexaploid wheats (SHWs) of an Elite-II sub- set and their 60 advanced derivatives. At seedling stage under controlled glasshouse conditions in Murree, the SHWs showed complete resistance/ immune to susceptible disease reactions viz., two (6.25%) were immune, eighteen (56.25%) were resistant, nine (28.12%) were intermediate, whereas three (9.37%) were susceptible. The BW/SH derivatives also demonstrated complete resistance/ immune to intermediate reactions viz., 51 (85%) were immune, 5 (8.33%) were resistant while 4 (6.66%) were intermediate. Adult plant resistance (APR) evaluated at the hill off-station summer site in Kaghan was 100% in SHWs (32/32) and 80% (48/60) in BW/SH derivatives. Several genotypes provided resistance at both plant stages viz., 20 (62.5%) SHWs and 45 (75%) BW/SH derivatives. -
Effects of Temperature on Wheat-Pathogen Interactions
Effects of temperature on wheat-pathogen interactions Ruth Bryant A thesis submitted to the University of East Anglia for the degree of Doctor of Philosophy John Innes Centre September 2013 © This copy of the thesis has been supplied on condition that anyone who consults it is understood to recognise that its copyright rests with the author and that no quotation from the thesis, nor any other information derived there from, may be published without the author’s prior, written consent 1 Abstract Climate change is affecting UK agriculture, and research is needed to prepare crops for the future. Wheat is the UK’s most important crop, and needs to be protected from losses caused by disease. While direct effect of the environment on pathogen spread is often reported, effect of the environment on host defence is not. Many wheat resistance genes are temperature sensitive and these were used as a starting point to investigate defence temperature sensitivity in wheat starting with yellow rust resistance gene Yr36, previously shown to be temperature-sensitive. The effect of temperature on resistance was shown to be independent of Yr36 in breeding line UC1041, and was more likely to be due to a previously-uncharacterised background temperature sensitivity. These results suggest that temperature changes, rather than thresholds, might influence some disease resistance mechanisms. Understanding this phenomenon could enable the breeding of more stable defence in crops. In order to gain further insight into how temperature changes influence resistance, plants were grown under different thermoperiods and challenged with different types of pathogens; Results showed that resistance to multiple pathogens in one cultivar Claire was enhanced under variable temperatures, compared to constant temperatures. -
Registrations of Cultivars
REGISTRATIONS OF CULTIVARS Registration of ‘Roane’ Wheat f. sp. tritici E´ m. Marchal; syn. Blumeria graminis (DC.) E.O. Speer]. The identity of the resistance genes in Roane is not ‘Roane’ (Reg. no. CV-899, PI 612958) is a full-season, high known, but it likely inherited the gene Pm4a from IN65309C1- yielding, apically awnleted soft red winter wheat (Triticum 18-2-3-2, and also may possess Pm3a from VA 71-54-147. aestivum L.) with exceptionally high test weight and resistance Roane was resistant to 34 of 38 isolates of E. graminis in to a broad spectrum of plant pathogens and insect pests. The seedling tests of entries in the 1996 UESRWWN, conducted Virginia Agricultural Experiment Station released Roane in by USDA-ARS at Raleigh, NC. Tests conducted by the the fall of 1999. Roane wheat was named in honor of Curtis USDA-ARS Cereal Disease Laboratory in St. Paul, MN, indi- W. Roane, Professor Emeritus, Virginia Polytechnic Institute cate that Roane possesses Gene Lr11, but is susceptible in and State University, for his contributions toward the develop- the seedling stage to the most prevalent races of leaf rust ment of disease and insect resistant small grain cultivars. (caused by Puccinia triticina Eriks.). However, in field tests Roane was derived from the three-way cross of VA 71-54- Roane had an average leaf rust severity (0–9) score of 3.0, 147/‘Coker 68-15’//IN65309C1-18-2-3-2. The first two parents, compared with scores of 4.6 for Pocahontas (Lr11) and 5.4 VA 71-54-147 (CItr 17449) and Coker 68-15 (CItr 15291), are for FFR555W (Lr10), indicating that Roane possesses some also the parents of the cultivar Saluda (Starling et al., 1986).