Data Mining in Bioinformatics: Report on BIOKDD'03
Total Page:16
File Type:pdf, Size:1020Kb
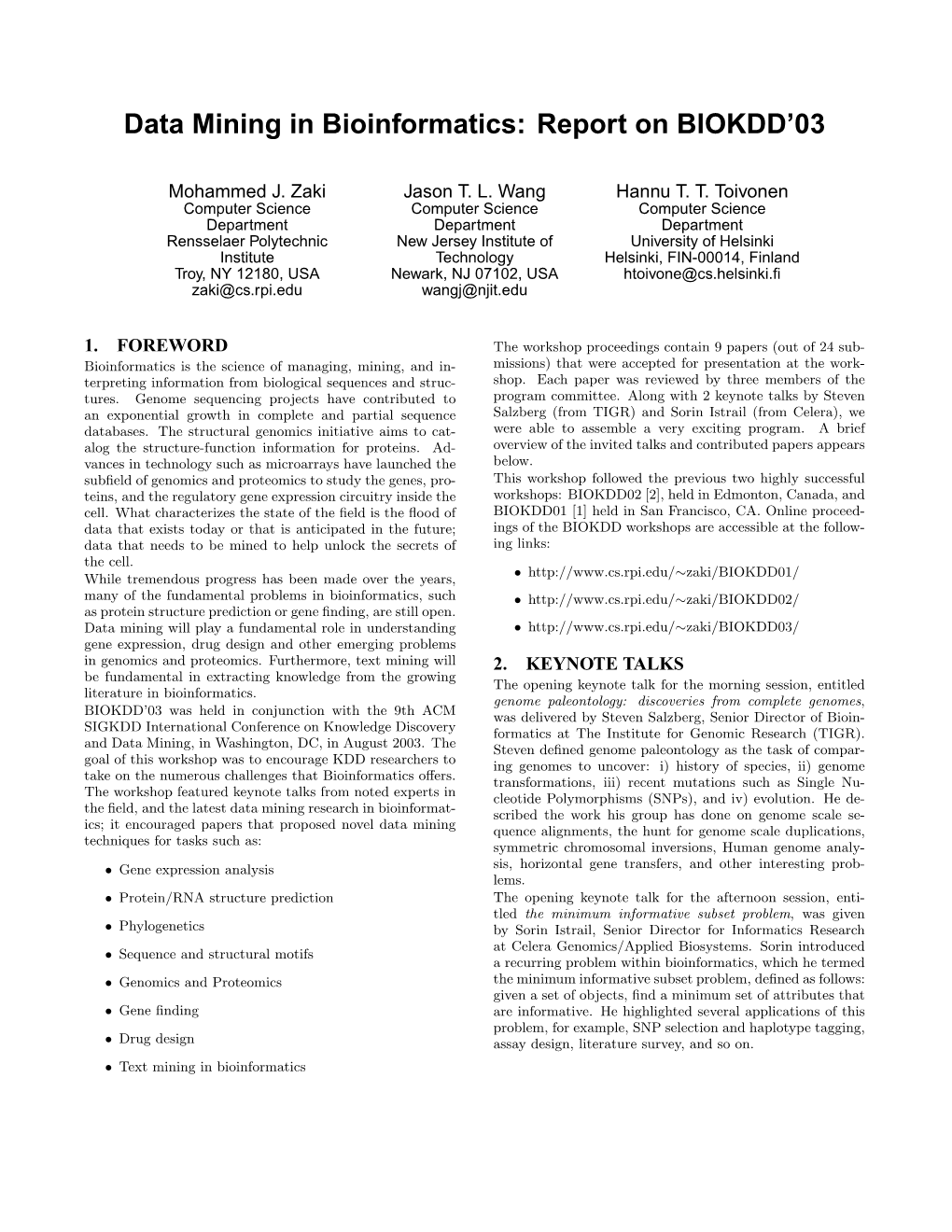
Load more
Recommended publications
-
BIOINFORMATICS Doi:10.1093/Bioinformatics/Bti144
Vol. 00 no. 0 2004, pages 1–11 BIOINFORMATICS doi:10.1093/bioinformatics/bti144 Solving and analyzing side-chain positioning problems using linear and integer programming Carleton L. Kingsford, Bernard Chazelle and Mona Singh∗ Department of Computer Science, Lewis-Sigler Institute for Integrative Genomics, Princeton University, 35, Olden Street, Princeton, NJ 08544, USA Received on August 1, 2004; revised on October 10, 2004; accepted on November 8, 2004 Advance Access publication … ABSTRACT set of possible rotamer choices (Ponder and Richards, 1987; Motivation: Side-chain positioning is a central component of Dunbrack and Karplus, 1993) for each Cα position on the homology modeling and protein design. In a common for- backbone. The goal is to choose a rotamer for each position mulation of the problem, the backbone is fixed, side-chain so that the total energy of the molecule is minimized. This conformations come from a rotamer library, and a pairwise formulation of SCP has been the basis of some of the more energy function is optimized. It is NP-complete to find even a successful methods for homology modeling (e.g. Petrey et al., reasonable approximate solution to this problem. We seek to 2003; Xiang and Honig, 2001; Jones and Kleywegt, 1999; put this hardness result into practical context. Bower et al., 1997) and protein design (e.g. Dahiyat and Mayo, Results: We present an integer linear programming (ILP) 1997; Malakauskas and Mayo, 1998; Looger et al., 2003). In formulation of side-chain positioning that allows us to tackle homology modeling, the goal is to predict the structure for a large problem sizes. -
BIOGRAPHICAL SKETCH NAME: Berger
BIOGRAPHICAL SKETCH NAME: Berger, Bonnie eRA COMMONS USER NAME (credential, e.g., agency login): BABERGER POSITION TITLE: Simons Professor of Mathematics and Professor of Electrical Engineering and Computer Science EDUCATION/TRAINING (Begin with baccalaureate or other initial professional education, such as nursing, include postdoctoral training and residency training if applicable. Add/delete rows as necessary.) EDUCATION/TRAINING DEGREE Completion (if Date FIELD OF STUDY INSTITUTION AND LOCATION applicable) MM/YYYY Brandeis University, Waltham, MA AB 06/1983 Computer Science Massachusetts Institute of Technology SM 01/1986 Computer Science Massachusetts Institute of Technology Ph.D. 06/1990 Computer Science Massachusetts Institute of Technology Postdoc 06/1992 Applied Mathematics A. Personal Statement Advances in modern biology revolve around automated data collection and sharing of the large resulting datasets. I am considered a pioneer in the area of bringing computer algorithms to the study of biological data, and a founder in this community that I have witnessed grow so profoundly over the last 26 years. I have made major contributions to many areas of computational biology and biomedicine, largely, though not exclusively through algorithmic innovations, as demonstrated by nearly twenty thousand citations to my scientific papers and widely-used software. In recognition of my success, I have just been elected to the National Academy of Sciences and in 2019 received the ISCB Senior Scientist Award, the pinnacle award in computational biology. My research group works on diverse challenges, including Computational Genomics, High-throughput Technology Analysis and Design, Biological Networks, Structural Bioinformatics, Population Genetics and Biomedical Privacy. I spearheaded research on analyzing large and complex biological data sets through topological and machine learning approaches; e.g. -
Computational Methods Addressing Genetic Variation In
COMPUTATIONAL METHODS ADDRESSING GENETIC VARIATION IN NEXT-GENERATION SEQUENCING DATA by Charlotte A. Darby A dissertation submitted to Johns Hopkins University in conformity with the requirements for the degree of Doctor of Philosophy Baltimore, Maryland June 2020 © 2020 Charlotte A. Darby All rights reserved Abstract Computational genomics involves the development and application of computational meth- ods for whole-genome-scale datasets to gain biological insight into the composition and func- tion of genomes, including how genetic variation mediates molecular phenotypes and disease. New biotechnologies such as next-generation sequencing generate genomic data on a massive scale and have transformed the field thanks to simultaneous advances in the analysis toolkit. In this thesis, I present three computational methods that use next-generation sequencing data, each of which addresses the genetic variations within and between human individuals in a different way. First, Samovar is a software tool for performing single-sample mosaic single-nucleotide variant calling on whole genome sequencing linked read data. Using haplotype assembly of heterozygous germline variants, uniquely made possible by linked reads, Samovar identifies variations in different cells that make up a bulk sequencing sample. We apply it to 13cancer samples in collaboration with researchers at Nationwide Childrens Hospital. Second, scHLAcount is a software pipeline that computes allele-specific molecule counts for the HLA genes from single-cell gene expression data. We use a personalized reference genome based on the individual’s genotypes to reveal allele-specific and cell type-specific gene expression patterns. Even given technology-specific biases of single-cell gene expression data, we can resolve allele-specific expression for these genes since the alleles are often quite different between the two haplotypes of an individual. -
Big Data, Moocs, and ... (PDF)
HHMI Constellation Studios for Science Education November 13-15, 2015 | HHMI Headquarters | Chevy Chase, MD Big Data, MOOCs, and Quantitative Education for Biologists Co-Chairs Pavel Pevzner, University of California- San Diego Sarah Elgin, Washington University Studio Objectives Discuss existing challenges in bioinformatics education with experts in computational biology and quantitative biology education, Evaluate best practices in teaching quantitative and computational biology, and Collaborate with scientist educators to develop instructional modules to support a biology curriculum that includes quantitative approaches. Friday | November 13 4:00 pm Arrival Registration Desk 5:30 – 6:00 pm Reception Great Hall 6:00 – 7:00 pm Dinner Dining Room 7:00 – 7:15 pm Welcome K202 David Asai, HHMI Cynthia Bauerle, HHMI Pavel Pevzner, University of California-San Diego Sarah Elgin, Washington University Alex Hartemink, Duke University 7:15 – 8:00 pm How to Maximize Interaction and Feedback During the Studio K202 Cynthia Bauerle and Sarah Simmons, HHMI 8:00 – 9:00 pm Keynote Presentation K202 "Computing + Biology = Discovery" Speakers: Ran Libeskind-Hadas, Harvey Mudd College Eliot Bush, Harvey Mudd College 9:00 – 11:00 pm Social The Pilot Saturday | November 14 7:30 – 8:15 am Breakfast Dining Room 8:30 – 10:00 am Lecture session 1 K202 Moderator: Pavel Pevzner 834a-854a “How is body fat regulated?” Laurie Heyer, Davidson College 856a-916a “How can we find mutations that cause cancer?” Ben Raphael, Brown University “How does a tumor evolve over time?” 918a-938a Russell Schwartz, Carnegie Mellon University “How fast do ribosomes move?” 940a-1000a Carl Kingsford, Carnegie Mellon University 10:05 – 10:55 am Breakout working groups Rooms: S221, (coffee available in each room) N238, N241, N140 1. -
ABSTRACT HISTORICAL GRAPH DATA MANAGEMENT Udayan
ABSTRACT Title of dissertation: HISTORICAL GRAPH DATA MANAGEMENT Udayan Khurana, Doctor of Philosophy, 2015 Dissertation directed by: Professor Amol Deshpande Department of Computer Science Over the last decade, we have witnessed an increasing interest in temporal analysis of information networks such as social networks or citation networks. Finding temporal interaction patterns, visualizing the evolution of graph properties, or even simply com- paring them across time, has proven to add significant value in reasoning over networks. However, because of the lack of underlying data management support, much of the work on large-scale graph analytics to date has largely focused on the study of static properties of graph snapshots. Unfortunately, a static view of interactions between entities is often an oversimplification of several complex phenomena like the spread of epidemics, informa- tion diffusion, formation of online communities, and so on. In the absence of appropriate support, an analyst today has to manually navigate the added temporal complexity of large evolving graphs, making the process cumbersome and ineffective. In this dissertation, I address the key challenges in storing, retrieving, and analyzing large historical graphs. In the first part, I present DeltaGraph, a novel, extensible, highly tunable, and distributed hierarchical index structure that enables compact recording of the historical information, and that supports efficient retrieval of historical graph snapshots. I present analytical models for estimating required storage space and snapshot retrieval times which aid in choosing the right parameters for a specific scenario. I also present optimizations such as partial materialization and columnar storage to speed up snapshot retrieval. In the second part, I present Temporal Graph Index that builds upon DeltaGraph to support version-centric retrieval such as a node’s 1-hop neighborhood history, along with snapshot reconstruction. -
Cloud Computing and the DNA Data Race Michael Schatz
Cloud Computing and the DNA Data Race Michael Schatz April 14, 2011 Data-Intensive Analysis, Analytics, and Informatics Outline 1. Genome Assembly by Analogy 2. DNA Sequencing and Genomics 3. Large Scale Sequence Analysis 1. Mapping & Genotyping 2. Genome Assembly Shredded Book Reconstruction • Dickens accidentally shreds the first printing of A Tale of Two Cities – Text printed on 5 long spools It was theIt was best the of besttimes, of times, it was it wasthe worstthe worst of of times, times, it it was was the the ageage of of wisdom, wisdom, it itwas was the agethe ofage foolishness, of foolishness, … … It was theIt was best the bestof times, of times, it was it was the the worst of times, it was the theage ageof wisdom, of wisdom, it was it thewas age the of foolishness,age of foolishness, … It was theIt was best the bestof times, of times, it was it wasthe the worst worst of times,of times, it it was the age of wisdom, it wasit was the the age age of offoolishness, foolishness, … … It was It thewas best the ofbest times, of times, it wasit was the the worst worst of times,of times, itit waswas thethe ageage ofof wisdom,wisdom, it wasit was the the age age of foolishness,of foolishness, … … It wasIt thewas best the bestof times, of times, it wasit was the the worst worst of of times, it was the age of ofwisdom, wisdom, it wasit was the the age ofage foolishness, of foolishness, … … • How can he reconstruct the text? – 5 copies x 138, 656 words / 5 words per fragment = 138k fragments – The short fragments from every copy are mixed -
BENG181/CSE 181/BIMM 181 Molecular Sequence Analysis Instructor: Pavel Pevzner
COURSE ANNOUNCEMENT FOR WINTER 2021 BENG181/CSE 181/BIMM 181 Molecular Sequence Analysis https://sites.google.com/site/ucsdcse181 Instructor: Pavel Pevzner ● phone: (858) 822-4365 ● e.mail: [email protected] ● web site: bioalgorithms.ucsd.edu Teaching Assistants: ● Andrey Bzikadze ([email protected]) ● Hsuan-lin (Charlene) Her ([email protected]) Time: 6:30-7:50 Mon/Wed, Place: online (seminar Friday 4:00-4:50 online) Zoom link for the class: https://ucsd.zoom.us/j/99782745100 Zoom link for the seminar: https://ucsd.zoom.us/j/96805484881 Office hours: PP: (Th 3-5 online), TAs (online Tue 1-2 PM and 4-5 PM or by appointment online) PP zoom link: https://ucsd.zoom.us/j/96986851791 Andrey Bzikadze zoom link: https://ucsd.zoom.us/j/94881347266 Hsuan-lin (Charlene) Her zoom link: https://ucsd.zoom.us/j/95134947264 Prerequisites: The course assumes some prior background in biology, some algorithmic culture (CSE 101 course on algorithms as a prerequisite), and some programming skills. Flipped online class. Starting in 2014, the Innovative Learning Technology Initiative (ILTI) at University of California encourages professors to transform their classes into online offerings available across various UC campuses. Dr. Pevzner is funded by the ILTI and NIH to develop new online approaches to bioinformatics education at UCSD. Since 2014, well before the COVID-19 pandemic, all lectures in this class are available online rather than presented in the classroom. Multi-university class. This class closely follows the textbook Bioinformatics Algorithms: an Active Learning Approach that has now been adopted by 140+ instructors from 40+ countries. -
John Anthony Capra
John Anthony Capra Contact Vanderbilt University e-mail: tony.capra-at-vanderbilt.edu Information Dept. of Biological Sciences www: http://www.capralab.org/ VU Station B, Box 35-1634 office: U5221 BSB/MRB III Nashville, TN 37235-1634 phone: (615) 343-3671 Research • Applying computational methods to problems in genetics, evolution, and biomedicine. Interests • Integrating genome-scale data to understand the functional effects of genetic differences between individuals and species. • Modeling evolutionary processes that drive the creation of lineage-specific traits and diseases. Academic Vanderbilt University, Nashville, Tennessee USA Employment Assistant Professor, Department of Biological Sciences August 2014 { Present Assistant Professor, Department of Biomedical Informatics February 2013 { Present Investigator, Center for Human Genetics Research Education And Gladstone Institutes, University of California, San Francisco, CA USA Training Postdoctoral Fellow, October 2009 { December 2012 • Advisor: Katherine Pollard Princeton University, Princeton, New Jersey USA Ph.D., Computer Science, June 2009 • Advisor: Mona Singh • Thesis: Algorithms for the Identification of Functional Sites in Proteins M.A., Computer Science, October 2006 Columbia College, Columbia University, New York, New York USA B.A., Computer Science, May 2004 B.A., Mathematics, May 2004 Pembroke College, Oxford University, Oxford, UK Columbia University Oxford Scholar, October 2002 { June 2003 • Subject: Mathematics Honors and Gladstone Institutes Award for Excellence in Scientific Leadership 2012 Awards Society for Molecular Biology and Evolution (SMBE) Travel Award 2012 PhRMA Foundation Postdoctoral Fellowship in Informatics 2011 { 2013 Princeton University Wu Graduate Fellowship 2004 { 2008 Columbia University Oxford Scholar 2002 { 2003 Publications Capra JA* and Kostka D*. Modeling DNA methylation dynamics with approaches from phyloge- netics. -
Steven L. Salzberg
Steven L. Salzberg McKusick-Nathans Institute of Genetic Medicine Johns Hopkins School of Medicine, MRB 459, 733 North Broadway, Baltimore, MD 20742 Phone: 410-614-6112 Email: [email protected] Education Ph.D. Computer Science 1989, Harvard University, Cambridge, MA M.Phil. 1984, M.S. 1982, Computer Science, Yale University, New Haven, CT B.A. cum laude English 1980, Yale University Research Areas: Genomics, bioinformatics, gene finding, genome assembly, sequence analysis. Academic and Professional Experience 2011-present Professor, Department of Medicine and the McKusick-Nathans Institute of Genetic Medicine, Johns Hopkins University. Joint appointments as Professor in the Department of Biostatistics, Bloomberg School of Public Health, and in the Department of Computer Science, Whiting School of Engineering. 2012-present Director, Center for Computational Biology, Johns Hopkins University. 2005-2011 Director, Center for Bioinformatics and Computational Biology, University of Maryland Institute for Advanced Computer Studies 2005-2011 Horvitz Professor, Department of Computer Science, University of Maryland. (On leave of absence 2011-2012.) 1997-2005 Senior Director of Bioinformatics (2000-2005), Director of Bioinformatics (1998-2000), and Investigator (1997-2005), The Institute for Genomic Research (TIGR). 1999-2006 Research Professor, Departments of Computer Science and Biology, Johns Hopkins University 1989-1999 Associate Professor (1996-1999), Assistant Professor (1989-1996), Department of Computer Science, Johns Hopkins University. On leave 1997-99. 1988-1989 Associate in Research, Graduate School of Business Administration, Harvard University. Consultant to Ford Motor Co. of Europe and to N.V. Bekaert (Kortrijk, Belgium). 1985-1987 Research Scientist and Senior Knowledge Engineer, Applied Expert Systems, Inc., Cambridge, MA. Designed expert systems for financial services companies. -
Graduation 2019
Department of Graduation Computer Science Celebration & Awards Dinner 2019 Evening Schedule 6:00pm Social Time 7:00pm Welcome Dr. Sanjeev Setia, Chair Department of Computer Science 7:10pm Dinner 8:00pm Presentation of Awards Dr. Sanjeev Setia, Chair Department of Computer Science Doctor of Philosophy Computer Science Indranil Banerjee Dissertation Title: Problems on Sorting, Sets and Graphs Major Professor: Dana Richards, PhD Arda Gumusalan Dissertation Title: Dynamic Modulation Scaling Enabled Real Time Transmission Scheduling For Wireless Sensor Networks Major Professor: Robert Simon, PhD Yun Guo Dissertation Title: Towards Automatically Localizing and Repairing SQL Faults Major Professors: Jeff Offut, PhD & Amihai Motro, PhD Mohan Krishnamoorthy Dissertation Title: Stochastic Optimization based on White-box Deterministic Approximations: Models, Algorithms and Application to Service Networks Major Professors: Alexander Brodsky, PhD & Daniel Menascé, PhD Arsalan Mousavian Dissertation Title: Semantic and 3D Understanding of a Scene for Robot Perception Major Professor : Jana Kosecka, PhD Zhiyun Ren Dissertation Title: Academic Performance Prediction with Machine Learning Techniques Major Professor : Huzefa Rangwala, PhD Md A. Reza Dissertation Title: Scene Understanding for Robotic Applications Major Professor : Jana Kosecka, PhD Venkateshwar Tadakamalla Dissertation Title: Analysis and Autonomic Elasticity Control for Multi-Server/Queues Under Traffic Surges in Cloud Environments Major Professor : Daniel A. Menascé, PhD Jianchao Tan -
THE BIG CHALLENGES of BIG DATA As They Grapple with Increasingly Large Data Sets, Biologists and Computer Scientists Uncork New Bottlenecks
TECHNOLOGY FEATURE THE BIG CHALLENGES OF BIG DATA As they grapple with increasingly large data sets, biologists and computer scientists uncork new bottlenecks. EMBL–EBI Extremely powerful computers are needed to help biologists to handle big-data traffic jams. BY VIVIEN MARX and how the genetic make-up of different can- year, particle-collision events in CERN’s Large cers influences how cancer patients fare2. The Hadron Collider generate around 15 petabytes iologists are joining the big-data club. European Bioinformatics Institute (EBI) in of data — the equivalent of about 4 million With the advent of high-throughput Hinxton, UK, part of the European Molecular high-definition feature-length films. But the genomics, life scientists are starting to Biology Laboratory and one of the world’s larg- EBI and institutes like it face similar data- Bgrapple with massive data sets, encountering est biology-data repositories, currently stores wrangling challenges to those at CERN, says challenges with handling, processing and mov- 20 petabytes (1 petabyte is 1015 bytes) of data Ewan Birney, associate director of the EBI. He ing information that were once the domain of and back-ups about genes, proteins and small and his colleagues now regularly meet with astronomers and high-energy physicists1. molecules. Genomic data account for 2 peta- organizations such as CERN and the European With every passing year, they turn more bytes of that, a number that more than doubles Space Agency (ESA) in Paris to swap lessons often to big data to probe everything from every year3 (see ‘Data explosion’). about data storage, analysis and sharing. -
UNIVERSITY of CALIFORNIA RIVERSIDE RNA-Seq
UNIVERSITY OF CALIFORNIA RIVERSIDE RNA-Seq Based Transcriptome Assembly: Sparsity, Bias Correction and Multiple Sample Comparison A Dissertation submitted in partial satisfaction of the requirements for the degree of Doctor of Philosophy in Computer Science by Wei Li September 2012 Dissertation Committee: Dr. Tao Jiang , Chairperson Dr. Stefano Lonardi Dr. Marek Chrobak Dr. Thomas Girke Copyright by Wei Li 2012 The Dissertation of Wei Li is approved: Committee Chairperson University of California, Riverside Acknowledgments The completion of this dissertation would have been impossible without help from many people. First and foremost, I would like to thank my advisor, Dr. Tao Jiang, for his guidance and supervision during the four years of my Ph.D. He offered invaluable advice and support on almost every aspect of my study and research in UCR. He gave me the freedom in choosing a research problem I’m interested in, helped me do research and write high quality papers, Not only a great academic advisor, he is also a sincere and true friend of mine. I am always feeling appreciated and fortunate to be one of his students. Many thanks to all committee members of my dissertation: Dr. Stefano Lonardi, Dr. Marek Chrobak, and Dr. Thomas Girke. I will be greatly appreciated by the advice they offered on the dissertation. I would also like to thank Jianxing Feng, Prof. James Borneman and Paul Ruegger for their collaboration in publishing several papers. Thanks to the support from Vivien Chan, Jianjun Yu and other bioinformatics group members during my internship in the Novartis Institutes for Biomedical Research.