Toward a Distributed Data Store for Fog Computing
Total Page:16
File Type:pdf, Size:1020Kb
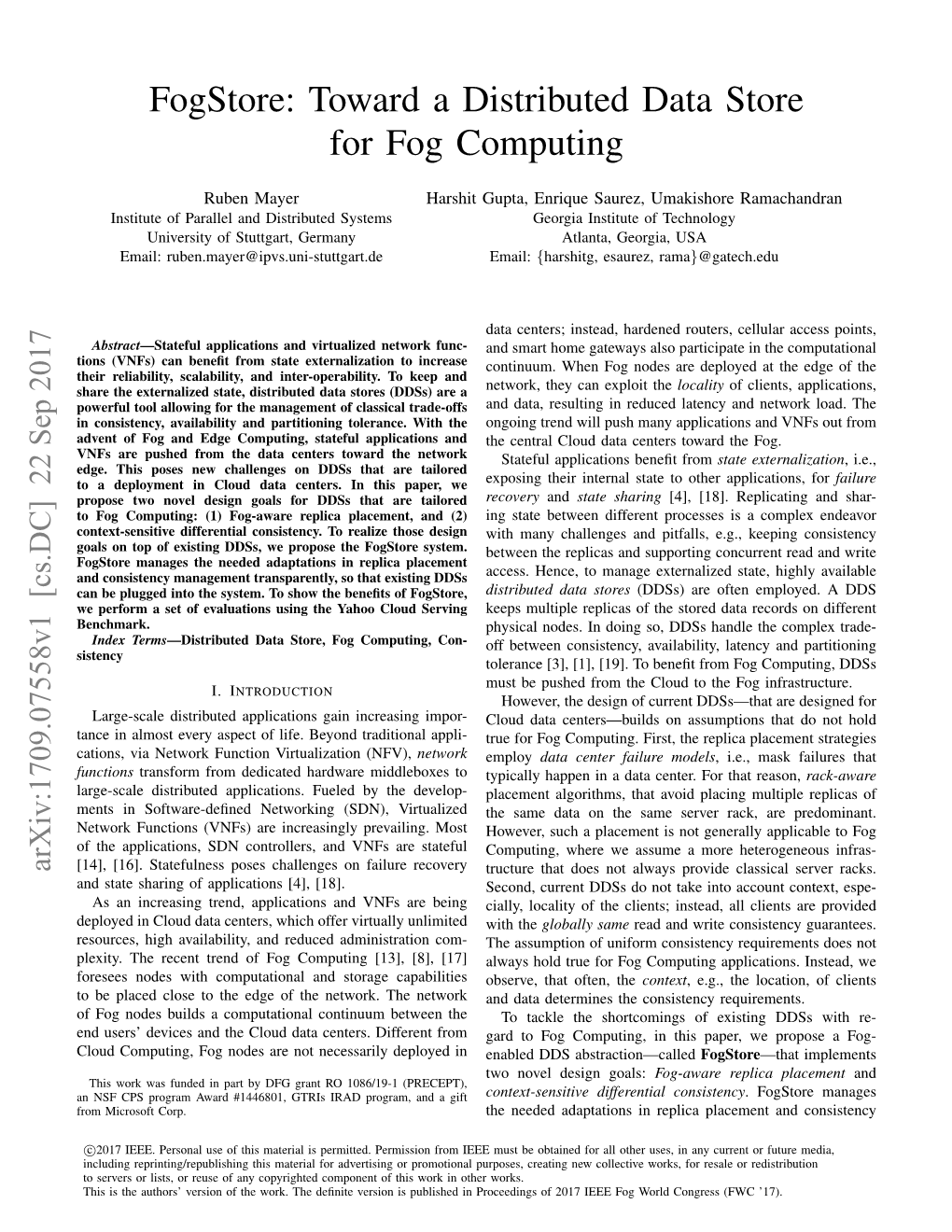
Load more
Recommended publications
-
Reflex: Remote Flash at the Performance of Local Flash
ReFlex: Remote Flash at the Performance of Local Flash Ana Klimovic Heiner Litz Christos Kozyrakis Flash Memory Summit 2017 Santa Clara, CA Stanford MAST1 ! Flash in Datacenters § NVMe Flash • 1,000x higher throughput than disk (1MIOPS) • 100x lower latency than disk (50-70usec) § But Flash is often underutilized due to imbalanced resource requirements Example Datacenter Flash Use-Case Applica(on Tier Datastore Tier Datastore Service get(k) Key-Value Store So9ware put(k,val) App App Tier Tier App Clients Servers TCP/IP NIC CPU RAM Hardware Flash 3 Imbalanced Resource Utilization Sample utilization of Facebook servers hosting a Flash-based key-value store over 6 months 4 [EuroSys’16] Flash storage disaggregation. Ana Klimovic, Christos Kozyrakis, Eno Thereska, Binu John, Sanjeev Kumar. Imbalanced Resource Utilization Sample utilization of Facebook servers hosting a Flash-based key-value store over 6 months 5 [EuroSys’16] Flash storage disaggregation. Ana Klimovic, Christos Kozyrakis, Eno Thereska, Binu John, Sanjeev Kumar. Imbalanced Resource Utilization u"lizaon Sample utilization of Facebook servers hosting a Flash-based key-value store over 6 months 6 [EuroSys’16] Flash storage disaggregation. Ana Klimovic, Christos Kozyrakis, Eno Thereska, Binu John, Sanjeev Kumar. Imbalanced Resource Utilization u"lizaon Flash capacity and bandwidth are underutilized for long periods of time 7 [EuroSys’16] Flash storage disaggregation. Ana Klimovic, Christos Kozyrakis, Eno Thereska, Binu John, Sanjeev Kumar Solution: Remote Access to Storage § Improve resource utilization by sharing NVMe Flash between remote tenants § There are 3 main concerns: 1. Performance overhead for remote access 2. Interference on shared Flash 3. Flexibility of Flash usage 8 Issue 1: Performance Overhead 4kB random read 1000 Local Flash iSCSI (1 core) 800 libaio+libevent (1core) 600 4x throughput drop 400 200 p95 read latency(us) 2× latency increase 0 0 50 100 150 200 250 300 IOPS (Thousands) • Traditional network/storage protocols and Linux I/O libraries (e.g. -
Multi-Resolution Storage and Search in Sensor Networks Deepak Ganesan University of Massachusetts Amherst
University of Massachusetts Amherst ScholarWorks@UMass Amherst Computer Science Department Faculty Publication Computer Science Series 2003 Multi-resolution Storage and Search in Sensor Networks Deepak Ganesan University of Massachusetts Amherst Follow this and additional works at: https://scholarworks.umass.edu/cs_faculty_pubs Part of the Computer Sciences Commons Recommended Citation Ganesan, Deepak, "Multi-resolution Storage and Search in Sensor Networks" (2003). Computer Science Department Faculty Publication Series. 79. Retrieved from https://scholarworks.umass.edu/cs_faculty_pubs/79 This Article is brought to you for free and open access by the Computer Science at ScholarWorks@UMass Amherst. It has been accepted for inclusion in Computer Science Department Faculty Publication Series by an authorized administrator of ScholarWorks@UMass Amherst. For more information, please contact [email protected]. Multi-resolution Storage and Search in Sensor Networks Deepak Ganesan Department of Computer Science, University of Massachusetts, Amherst, MA 01003 Ben Greenstein, Deborah Estrin Department of Computer Science, University of California, Los Angeles, CA 90095, John Heidemann University of Southern California/Information Sciences Institute, Marina Del Rey, CA 90292 and Ramesh Govindan Department of Computer Science, University of Southern California, Los Angeles, CA 90089 Wireless sensor networks enable dense sensing of the environment, offering unprecedented op- portunities for observing the physical world. This paper addresses two key challenges in wireless sensor networks: in-network storage and distributed search. The need for these techniques arises from the inability to provide persistent, centralized storage and querying in many sensor networks. Centralized storage requires multi-hop transmission of sensor data to internet gateways which can quickly drain battery-operated nodes. -
Network-Compute Co-Design for Distributed In-Memory Computing” Advisors: Prof
Network–Compute Co-Design for Distributed In-Memory Computing Alexandros Daglis Ph.D. Thesis July 6, 2018 Thesis Committee Prof. Babak Falsafi, Prof. Edouard Bugnion, thesis directors Prof. Paolo Ienne, jury president Dr. Paolo Faraboschi, external examiner Prof. James Larus, internal examiner Prof. Gurindar Sohi, external examiner Communication and Information Sciences École Polytechnique Fédérale de Lausanne Lausanne, Switzerland T¨c paideÐac êfh tὰς μὲn ûÐzac εἶnai πικράς, tὸn δὲ καρπὸn γλυκύν. — Aristotèlhc The roots of education are bitter, but the fruit is sweet. — Aristotle To my family Acknowledgements My PhD journey has undoubtedly been the most challenging and, at the same time, most rewarding so far in my life. A journey that transformed me into a better person and taught me the true value of perseverance, team work, empathy, and patience. I would not have been able to reach the finish line if it weren’t for the wonderful people around me; people who were always there to magnify the joy of the best moments; people who would always support and help me push through the hardest times. To all of these people, I owe my deepest gratitude. It is therefore apposite to start this thesis by thanking them. First and foremost, I am grateful to my advisors, Babak and Ed. Babak has been a constant source of stimulation to keep pushing myself out of my comfort zone, a practice instrumental to my success. He taught me the importance of asking the right questions and taking a step back to look at the big picture. Babak’s attention to detail and quest for perfection confirms that the "the path of virtues goes through toils". -
Succinct: Enabling Queries on Compressed Data
Succinct: Enabling Queries on Compressed Data Rachit Agarwal, Anurag Khandelwal, and Ion Stoica, University of California, Berkeley https://www.usenix.org/conference/nsdi15/technical-sessions/presentation/agarwal This paper is included in the Proceedings of the 12th USENIX Symposium on Networked Systems Design and Implementation (NSDI ’15). May 4–6, 2015 • Oakland, CA, USA ISBN 978-1-931971-218 Open Access to the Proceedings of the 12th USENIX Symposium on Networked Systems Design and Implementation (NSDI ’15) is sponsored by USENIX Succinct: Enabling Queries on Compressed Data Rachit Agarwal Anurag Khandelwal Ion Stoica UC Berkeley UC Berkeley UC Berkeley Abstract that indexes can be as much as 8× larger than the in- Succinct is a data store that enables efficient queries di- put data size. Traditional compression techniques can rectly on a compressed representation of the input data. reduce the memory footprint but suffer from degraded Succinct uses a compression technique that allows ran- throughput since data needs to be decompressed even dom access into the input, thus enabling efficient stor- for simple queries. Thus, existing data stores either re- age and retrieval of data. In addition, Succinct natively sort to using complex memory management techniques supports a wide range of queries including count and for identifying and caching “hot” data [5, 6, 26, 35] or search of arbitrary strings, range and wildcard queries. simply executing queries off-disk or off-SSD [25].In What differentiates Succinct from previous techniques either case, latency and throughput advantages of in- is that Succinct supports these queries without storing dexes drop compared to in-memory query execution. -
Consistent and Durable Data Structures for Non-Volatile Byte-Addressable Memory
Consistent and Durable Data Structures for Non-Volatile Byte-Addressable Memory Shivaram Venkataraman†∗, Niraj Tolia‡, Parthasarathy Ranganathan†, and Roy H. Campbell∗ †HP Labs, Palo Alto, ‡Maginatics, and ∗University of Illinois, Urbana-Champaign Abstract ther disk or flash. Not only are they byte-addressable and low-latency like DRAM but, they are also non-volatile. The predicted shift to non-volatile, byte-addressable Projected cost [19] and power-efficiency characteris- memory (e.g., Phase Change Memory and Memristor), tics of Non-Volatile Byte-addressable Memory (NVBM) the growth of “big data”, and the subsequent emergence lead us to believe that it can replace both disk and mem- of frameworks such as memcached and NoSQL systems ory in data stores (e.g., memcached, database systems, require us to rethink the design of data stores. To de- NoSQL systems, etc.) but not through legacy inter- rive the maximum performance from these new mem- faces (e.g., block interfaces or file systems). First, the ory technologies, this paper proposes the use of single- overhead of PCI accesses or system calls will dominate level data stores. For these systems, where no distinc- NVBM’s sub-microsecond access latencies. More im- tion is made between a volatile and a persistent copy of portantly, these interfaces impose a two-level logical sep- data, we present Consistent and Durable Data Structures aration of data, differentiating between in-memory and (CDDSs) that, on current hardware, allows programmers on-disk copies. Traditional data stores have to both up- to safely exploit the low-latency and non-volatile as- date the in-memory data and, for durability, sync the data pects of new memory technologies. -
(ASE 18 US 8,370.224 B2 Page 2
USOO8370224B2 (12) United States Patent (10) Patent No.: US 8,370.224 B2 Grewal (45) Date of Patent: Feb. 5, 2013 (54) GRAPHICAL INTERFACE FOR DISPLAY OF 6,574,779 B2 6/2003 Allen et al. ASSETS IN AN ASSET MANAGEMENT 6,581,045 B1 6/2003 Watson 6,631,552 B2 10/2003 Yamaguchi SYSTEM 6,650,346 B1 1 1/2003 Jaeger et al. 6,691,115 B2 2/2004 Mosher, Jr. et al. (75) Inventor: Ardaman Singh Grewal, Milwaukee, 6,735,752 B2 5, 2004 Manoo WI (US) 6,738,958 B2 5, 2004 Manoo 6,763,377 B1 7/2004 Belknap et al. 6,775,576 B2 8/2004 Spriggs et al. (73) Assignee: Rockwell Automation Technologies, 6,782,399 B2 8/2004 Mosher, Jr. Inc., Mayfield Heights, OH (US) 6,789,214 B1 9/2004 De Bonis-Hamelin et al. 6,806,813 B1 10/2004 Cheng et al. (*) Notice: Subject to any disclaimer, the term of this 6,847,982 B2 1/2005 Parker et al. patent is extended or adjusted under 35 6,889,096 B2 * 5/2005 Spriggs et al. .................. 7OO/17 U.S.C. 154(b) by 247 days. (Continued) (21) Appl. No.: 11/535,549 FOREIGN PATENT DOCUMENTS GB 2414568 A 11, 2005 (22) Filed: Sep. 27, 2006 WO 2006/081024 A2 8, 2006 (65) Prior Publication Data OTHER PUBLICATIONS US 2008/OO77512 A1 Mar. 27, 2008 “Gibson Petroleum Selects Couay to Add Location Intelligence to Fleet Management System.” Canada NewsWire Nov. 29, 2001 (51) Int. Cl. ProQuest Newsstand, ProQuest. -
Bluecache: a Scalable Distributed Flash-Based Key-Value Store
BlueCache: A Scalable Distributed Flash-based Key-value Store Shuotao Xuz Sungjin Leey Sang-Woo Junz Ming Liuz Jamey Hicks[ Arvindz zMassachusetts Institute of Technology yInha University [Accelerated Tech, Inc fshuotao, wjun, ml, [email protected] [email protected] [email protected] ABSTRACT KVS caches are used extensively in web infrastructures because of A key-value store (KVS), such as memcached and Redis, is widely their simplicity and effectiveness. used as a caching layer to augment the slower persistent backend Applications that use KVS caches in data centers are the ones storage in data centers. DRAM-based KVS provides fast key-value that have guaranteed high hit-rates. Nevertheless, different appli- access, but its scalability is limited by the cost, power and space cations have very different characteristics in terms of the size of needed by the machine cluster to support a large amount of DRAM. queries, the size of replies and the request rate. KVS servers are This paper offers a 10X to 100X cheaper solution based on flash further subdivided into application pools, each representing a sepa- storage and hardware accelerators. In BlueCache key-value pairs rate application domain, to deal with these differences. Each appli- are stored in flash storage and all KVS operations, including the cation pool has its own prefix of the keys and typically it does not flash controller are directly implemented in hardware. Further- share its key-value pairs with other applications. Application mixes more, BlueCache includes a fast interconnect between flash con- also change constantly, therefore it is important for applications to trollers to provide a scalable solution. -
Building Distributed Storage with Specialized Hardware
Research Collection Doctoral Thesis Building Distributed Storage with Specialized Hardware Author(s): Istvan, Zsolt Publication Date: 2018-06 Permanent Link: https://doi.org/10.3929/ethz-b-000266096 Rights / License: In Copyright - Non-Commercial Use Permitted This page was generated automatically upon download from the ETH Zurich Research Collection. For more information please consult the Terms of use. ETH Library DISS. ETH NO. 24858 Building Distributed Storage with Specialized Hardware A thesis submitted to attain the degree of DOCTOR OF SCIENCES of ETH ZURICH¨ (Dr. sc. ETH Z¨urich) presented by ZSOLT ISTVAN´ Master of Science ETH in Computer Science, ETH Z¨urich born on 08.09.1988 accepted on the recommendation of Prof. Dr. Gustavo Alonso (ETH Z¨urich), examiner Prof. Dr. Torsten Hoefler (ETH Z¨urich), co-examiner Prof. Dr. Timothy Roscoe (ETH Z¨urich), co-examiner Dr. Ken Eguro (MSR Redmond), co-examiner 2017 Abstract In an effort to keep up with increasing data sizes, applications in the datacenter are scaled out to large number of machines. Even though this allows them to tackle complex problems, data movement bottlenecks of various types appear, limiting overall performance and scalability. Pushing computation closer to the data reduces bottlenecks, and in this work we explore this idea in the context of distributed key-value stores. Efficient compute is important in the datacenter, especially at scale. Driven by the stagna- tion of single-threaded CPU performance, specialized hardware and hybrid architectures are emerging and could hold the answer for more efficient compute and data manage- ment. In this work we use Field Programmable Gate Arrays (FPGA) to break traditional trade-offs and limitations, and to explore design scenarios that were previously infeasible. -
Iot Governance, Privacy and Security Issues EUROPEAN RESEARCH CLUSTER on the INTERNET of THINGS
Internet of Things IoT Governance, Privacy and Security Issues EUROPEAN RESEARCH CLUSTER ON THE INTERNET OF THINGS January, 2015 “Just as energy is the basis of life itself, and ideas the source of innovation, so is innovation the vital spark of all human change, improvement and progress.” Ted Levitt IERC Coordinators: HINGS Ovidiu Vermesan, Coordinator IERC Cluster, [email protected] T Peter Friess, Coordinator IERC Cluster, European Commission, [email protected] Authors Gianmarco Baldini, (DG JRC-EC) Trevor Peirce (Avanta Global), Maarten Botterman (GNKS Consult) Maria Chiara Talacchini, (DG JRC-EC) NTERNET OF I Angela Pereira (DG JRC – EC) Marcus Handte (University of Duisburg-Essen), Domenico Rotondi (TXT Group), Henrich C. Pöhls (Passau University), Ovidiu Vermesan (SINTEF), Atta Baddii (University of Reading), Bertrand Copigneaux (Inno Ag), Schreckling, Daniel (Passau University), THE ON LUSTER Luca Vigano (University of Verona), C Gary Steri (DG JRC – EC) Salvatore Piccione (TXT Group), Panagiotis Vlacheas (UPRC) Vera Stavroulaki (UPRC) ESEARCH Dimitris Kelaidonis (UPRC) R Ricardo Neisse (DG JRC-EC) Elias Tragos (ICS - FORTH), Philippe Smadja (GEMALTO) Christine Hennebert (CEA LETI) Martin Serrano (National University of Ireland Galway) UROPEAN E Stefano Severi (Jacobs University) - Giuseppe Abreu (Jacobs University) Peter T. Kirstein (University College London) Socrates Varakliotis (University College London) IERC Antonio Skarmeta (University of Murcia) Contributing SDOs, Projects and Initiatives ••• 2 / 128 iCore, GAMBAS, BUTLER, CEN/CENELEC, ETSI, ISO, PROBE-IT, SPaCIoS, IoT@Work, COMPOSE, RERUM, OpenIoT, IoT6, Value-Ageing IERC Acknowledgements The IERC would like to thank the European Commission services for their support in the planning and preparation of this document. -
Zipg: a Memory-Efficient Graph Store for Interactive Queries
ZipG: A Memory-efficient Graph Store for Interactive Queries Anurag Khandelwal Zongheng Yang Evan Ye UC Berkeley UC Berkeley UC Berkeley Rachit Agarwal Ion Stoica Cornell University UC Berkeley ABSTRACT Achieving high performance for interactive user-facing queries We present ZipG, a distributed memory-efficient graph store for on large graphs, however, remains a challenging problem. serving interactive graph queries. ZipG achieves memory effi- For such interactive queries, the goal is not only to achieve ciency by storing the input graph data using a compressed rep- millisecond-level latency, but also high throughput [24, 29, 35]. resentation. What differentiates ZipG from other graph stores is When the graph data is distributed between memory and sec- its ability to execute a wide range of graph queries directly on ondary storage, potentially across multiple servers, achieving this compressed representation. ZipG can thus execute a larger these goals is particularly hard. For instance, consider the query: fraction of queries in main memory, achieving query interactivity. “Find friends of Alice who live in Ithaca”. One possible way to ZipG exposes a minimal API that is functionally rich enough execute this query is to execute two sub-queries — find friends to implement published functionalities from several industrial of Alice, and, find people who live in Ithaca — and compute the graph stores. We demonstrate this by implementing and evaluat- final result using a join or an intersection of results from two sub-queries. Such joins may be complex1, and may incur high ing graph queries from Facebook TAO, LinkBench, Graph Search 2 and several other workloads on top of ZipG. -
Towards Elastic High-Performance Geo-Distributed Storage in the Cloud
Towards Elastic High-Performance Geo-Distributed Storage in the Cloud YING LIU School of Information and Communication Technology KTH Royal Institute of Technology Stockholm, Sweden 2016 and Institute of Information and Communication Technologies, Electronics and Applied Mathematics Université catholique de Louvain Louvain-la-Neuve, Belgium 2016 KTH School of Information and Communication Technology TRITA-ICT 2016:24 SE-164 40 Kista ISBN 978-91-7729-092-6 SWEDEN Akademisk avhandling som med tillstånd av Kungl Tekniska högskolan framlägges till offentlig granskning för avläggande av doktorsexamen i Informations- och kom- munikationsteknik måndagen den 3 oktober 2016 klockan 10:00 i Sal C, Electrum, Kungl Tekniska högskolan, Kistagången 16, Kista. © Ying Liu, October 2016 Tryck: Universitetsservice US AB To my beloved Yi and my parents Xianchun and Aiping. Abstract In this thesis, we have investigated two aspects towards improving the performance of distributed stor- age systems. In one direction, we present techniques and algorithms to reduce request latency of distributed storage services that are deployed geographically. In another direction, we propose and design elasticity controllers to maintain predictable/stable performance of distributed storage systems under dynamic work- loads and platform uncertainties. On the research path towards the first direction, we have proposed a lease-based data consistency algo- rithm that allows a distributed storage system to serve read-dominant workload efficiently in a global scale. Essentially, leases are used to assert the correctness/freshness of data within a time interval. The leasing algorithm allows replicas with valid leases to serve read requests locally. As a result, most of the read requests are served with little latency. -
A Scalable Distributed Flash-Based Key-Value Store
BlueCache: A Scalable Distributed Flash-based Key-Value Store by Shuotao Xu B.S., University of Illinois at Urbana-Champaign (2012) Submitted to the Department of Electrical Engineering and Computer Science in partial fulfillment of the requirements for the degree of Master of Science in Computer Science and Engineering at the MASSACHUSETTS INSTITUTE OF TECHNOLOGY June 2016 © Massachusetts Institute of Technology 2016. All rights reserved. Author............................................................. Department of Electrical Engineering and Computer Science May 20, 2016 Certified by . Arvind Johnson Professor of Computer Science and Engineering Thesis Supervisor Accepted by. Leslie Kolodziejski Chair, Department Committee on Graduate Theses 2 BlueCache: A Scalable Distributed Flash-based Key-Value Store by Shuotao Xu Submitted to the Department of Electrical Engineering and Computer Science on May 20, 2016, in partial fulfillment of the requirements for the degree of Master of Science in Computer Science and Engineering Abstract Low-latency and high-bandwidth access to a large amount of data is a key requirement for many web applications in data centers. To satisfy such a requirement, a distributed in- memory key-value store (KVS), such as memcached and Redis, is widely used as a caching layer to augment the slower persistent backend storage (e.g. disks) in data centers. DRAM- based KVS is fast key-value access, but it is difficult to further scale the memory pool size because of cost, power/thermal concerns and floor plan limits. Flash memory offers an alternative as KVS storage media with higher capacity per dollar and less power per byte. However, a flash-based KVS software running on an x86 server with commodity SSD can- not harness the full potential device performance of flash memory, because of overheads of the legacy storage I/O stack and relatively slow network in comparision with faster flash storage.