Connecting the Countryside Via E-Commerce: Evidence from China
Total Page:16
File Type:pdf, Size:1020Kb
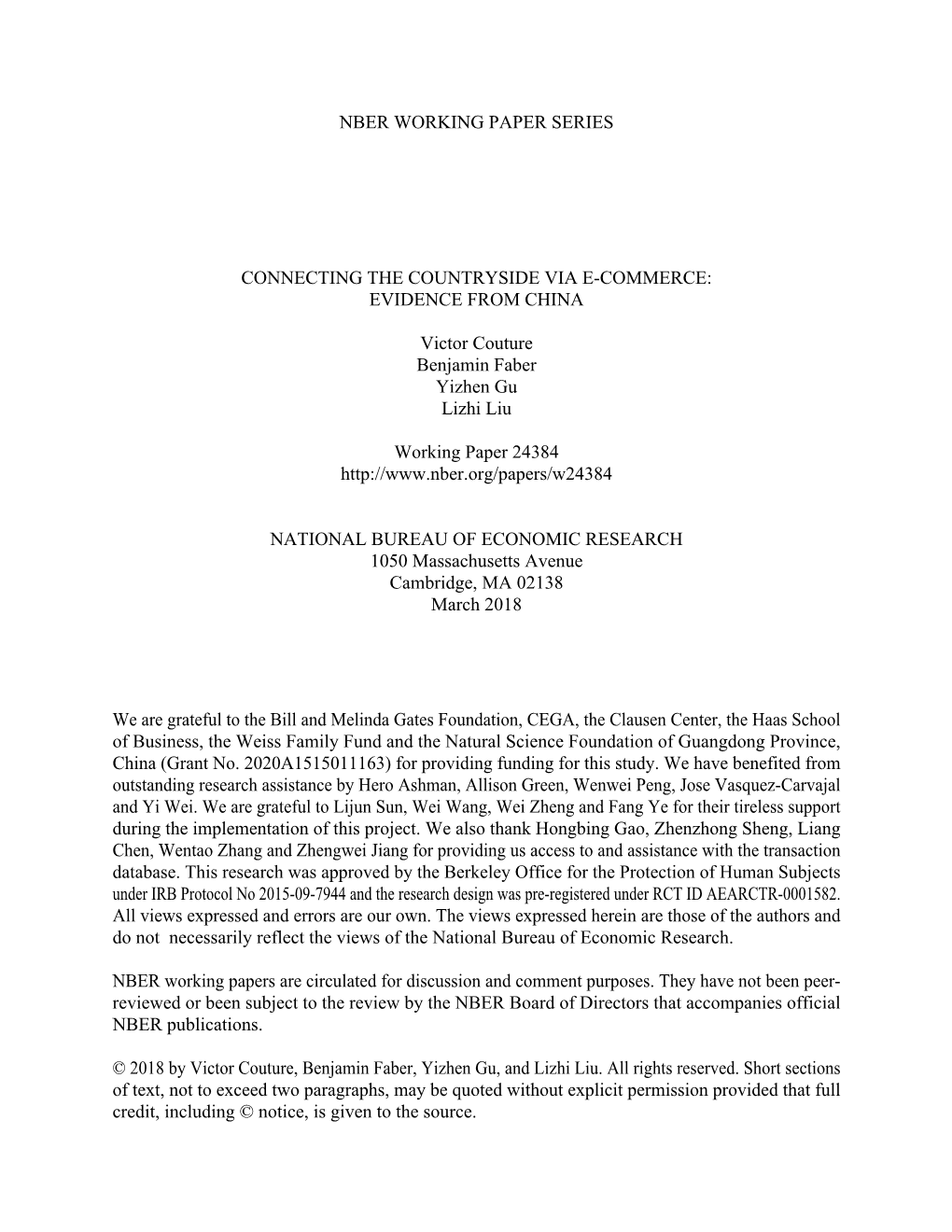
Load more
Recommended publications
-
Curious About Cryptocurrencies? Investors Need to Make Sure They Separate “Investing” from “Speculation” by Don Mcarthur, CFA®
Curious About Cryptocurrencies? Investors Need to Make Sure They Separate “Investing” from “Speculation” By Don McArthur, CFA® Bitcoin and other cryptocurrencies have received plenty of media coverage lately, and it is natural for investors to wonder about them. Even celebrities have become associated with Bitcoin publicity through social media. Interest has piqued to a point where there are even Exchange Traded Funds (ETFs) that invest in Bitcoin, giving investors the means to invest in the Futures market. After having performed in-depth research on Bitcoin and other cryptocurrencies, our position at Commerce Trust Company is that they should not currently play a role in client portfolios. As part of that research, Commerce Senior Vice President and Investment Analyst Don McArthur, CFA, put together a primer on the topic of cryptocurrencies in general. In the following commentary, he explains why Bitcoin at this stage is more about speculating than investing in something with intrinsic value. He also touches on how Blockchain networking technology not only supports cryptocurrencies, but many other industrial applications as well. We thought you would enjoy this commentary as McArthur shares his thoughts in a mind-opening Q&A. Q . What is Bitcoin and how did it start? A. Bitcoin is one of hundreds of digital currencies, or cryptocurrency, based on Blockchain technology. As an early mover, Bitcoin is by far the largest digital currency. Bitcoin was launched in 2009 by a mysterious person (or persons) known only by the pseudonym Satoshi Nakamoto. Unlike traditional currencies, which are issued by central banks, Bitcoin has no central monetary authority. -
Archaeological Observation on the Exploration of Chu Capitals
Archaeological Observation on the Exploration of Chu Capitals Wang Hongxing Key words: Chu Capitals Danyang Ying Chenying Shouying According to accurate historical documents, the capi- In view of the recent research on the civilization pro- tals of Chu State include Danyang 丹阳 of the early stage, cess of the middle reach of Yangtze River, we may infer Ying 郢 of the middle stage and Chenying 陈郢 and that Danyang ought to be a central settlement among a Shouying 寿郢 of the late stage. Archaeologically group of settlements not far away from Jingshan 荆山 speaking, Chenying and Shouying are traceable while with rice as the main crop. No matter whether there are the locations of Danyang and Yingdu 郢都 are still any remains of fosses around the central settlement, its oblivious and scholars differ on this issue. Since Chu area must be larger than ordinary sites and be of higher capitals are the political, economical and cultural cen- scale and have public amenities such as large buildings ters of Chu State, the research on Chu capitals directly or altars. The site ought to have definite functional sec- affects further study of Chu culture. tions and the cemetery ought to be divided into that of Based on previous research, I intend to summarize the aristocracy and the plebeians. The relevant docu- the exploration of Danyang, Yingdu and Shouying in ments and the unearthed inscriptions on tortoise shells recent years, review the insufficiency of the former re- from Zhouyuan 周原 saying “the viscount of Chu search and current methods and advance some personal (actually the ruler of Chu) came to inform” indicate that opinion on the locations of Chu capitals and later explo- Zhou had frequent contact and exchange with Chu. -
New Insights on Retail E-Commerce (July 26, 2017)
U.S. Department of Commerce Economics Newand Insights Statistics on Retail Administration E-Commerce Office of the Chief Economist New Insights on Retail E-Commerce Executive Summary The U.S. Census Bureau has been collecting data on retail sales since the 1950s and data on e-commerce retail sales since 1998. As the Internet has become ubiquitous, many retailers have created websites and even entire divisions devoted to fulfilling online orders. Many consumers have By turned to e-commerce as a matter of convenience or to increase the Jessica R. Nicholson variety of goods available to them. Whatever the reason, retail e- commerce sales have skyrocketed and the Internet will undoubtedly continue to influence how consumers shop, underscoring the need for good data to track this increasingly important economic activity. In June 2017, the Census Bureau released a new supplemental data table on retail e-commerce by type of retailer. The Census Bureau developed these estimates by re-categorizing e-commerce sales data from its ESA Issue Brief existing “electronic shopping” sales data according to the primary #04-17 business type of the retailer, such as clothing stores, food stores, or electronics stores. This report examines how the new estimates enhance our understanding of where consumers are shopping online and also provides an overview of trends in retail and e-commerce sales. Findings from this report include: E-commerce sales accounted for 7.2 percent of all retail sales in 2015, up dramatically from 0.2 percent in 1998. July 26, 2017 E-commerce sales have been growing nine times faster than traditional in-store sales since 1998. -
E-Commerce Integration and Economic Development: Evidence from China∗
E-Commerce Integration and Economic Development: Evidence from China∗ Victor Couture,y Benjamin Faber,z Yizhen Gu§ and Lizhi Liu{ July 2017 –Preliminary and incomplete– Abstract The number of people buying and selling products online in China has grown from practically zero in 2000 to more than 400 million by 2015. Most of this growth has occurred in cities. In this context, the Chinese government recently announced the expansion of e-commerce to the countryside as a policy priority with the objective to close the rural-urban economic divide. As part of this agenda, the government entered a partnership with a large Chinese e-commerce firm. The program invests in the necessary transport logistics to ship products to and sell prod- ucts from tens of thousands of villages that were largely unconnected to e-commerce. The firm also installs an e-commerce terminal at a central village location, where a terminal manager assists households in buying and selling products through the firm’s e-commerce platform. This paper combines a new collection of survey and transaction microdata with a randomized control trial (RCT) across villages that we implement in collaboration with the e-commerce firm. We use this empirical setting to provide evidence on the potential of e-commerce inte- gration to foster economic development in the countryside, the underlying channels and the distribution of the gains from e-commerce across households and villages. Keywords: E-commerce, trade integration, economic development, rural-urban divide JEL Classification: F63, O12, R13 ∗We are grateful to CEGA, the Clausen Center, the Haas School of Business, the Weiss Family Fund and the Bill and Melinda Gates Foundation for providing funding for this study. -
Southeast Asia
SOUTHEAST ASIA Shang Dynasty Zhou Dynasty ● Time of emergence: 1766 BC ● Time of emergence: 1046-256 BCE ● Time they were at their peak:1350 BC ● Divided into 2 different periods (Western Zhou: ● Time they were around: 1766-1122 BC 1046-771 BCE)(Eastern Zhou: 770-256 BCE) ● Time of fall: 1122 BC ● They were around for 8 centuries (800+ years) ● Time of fall: 256 BCE GEOGRAPHIC IMPACT ON SOCIETY Shang Dynasty Zhou Dynasty The Shang Dynasty controlled the North China Plain, which ● They were located west of Shang Dynasty however after corresponds to the modern day Chinese provinces of Anhui, Hebei, conquering Shang Dynasty, their borders extended as far Henan, Shandong, and Shanxi. The area that those of the Shang south as chang Jiang river and east to the Yellow sea. Dynasty lived in, under the Yellow River Valley, gave them water as These body of waters provided fertile soil for good farming well as fertile soil which helped their civilization thrive. Natural borders, and their trading increased. ● Present day location: Xi’an in Shaanxi near the Wei river such as mountains, also protected the area, making it easier to protect. and confluence of the Yellow river The Yellow River also made it easy for the people that lived there to ● They were not geographically isolated from other obtain a steady supply of water. civilizations ● They were exposed to large bodies of water POLITICAL SYSTEM AND IMPACT ON SOCIETY government Shang Dynasty Zhou Dynasty The Shang Dynasty was ruled by a ● The Zhou Dynasty ruled with a confucian social hierarchy hereditary monarchy, in which the ● The citizens were expected to follow the rules and values of confucianism government wa controlled by the king Organization: mainly, and the line of rule descended ● Had the “mandate of heaven” through the family. -
Eliminating Barriers to Internal Commerce to Facilitate Intraregional Trade
Eliminating Barriers to Internal Commerce to Facilitate Intraregional Trade Olumide Taiwo and Nelipher Moyo, Brookings Africa Growth Initiative ncreased trade between African countries holds Roads account for 80 to 90 percent of all freight and promise for shared growth and development in passenger movement in Africa. Road density is an ef- the region. However, before African countries can fective proxy of how well connected areas of a country Ifully exploit the benefits associated with increased are. Africa has a road density of only 16.8 kilometers trade with each other, they must first address the bar- per 1,000 square kilometers, compared with 37 kilo- riers to the movement of goods and people within meters per 1,000 square kilometers in other low-in- their countries. It is difficult to imagine how Africa come regions (table 1). Likewise, rail density in Africa will be able to move goods from Cape Town to Cairo is only 2.8 kilometers per 1,000 square kilometers— when it is unable to move goods from one city to an- much lower than the 3.4 kilometers per 1,000 square other within the same country. Take the case of Ke- kilometers in other low-income regions. Air travel nya: while parts of northern Kenya were experiencing within Africa continues to be more expensive per mile major food shortages in January 2011, farmers in the than intercontinental travel. Africa’s inland waterways Rift Valley had food surpluses and were imploring the present an excellent opportunity to connect cities and government to buy their excess crops before they went countries. -
The Macro-Economic Impact of E-Commerce in the EU Digital Single Market
INSTITUTE FOR PROSPECTIVE TECHNOLOGICAL STUDIES DIGITAL ECONOMY WORKING PAPER 2015/09 The Macro-economic Impact of e-Commerce in the EU Digital Single Market Melisande Cardona Nestor Duch-Brown Joseph Francois Bertin Martens Fan Yang 2015 The Macro-economic Impact of e-Commerce in the EU Digital Single Market This publication is a Working Paper by the Joint Research Centre of the European Commission. It results from the Digital Economy Research Programme at the JRC Institute for Prospective Technological Studies, which carries out economic research on information society and EU Digital Agenda policy issues, with a focus on growth, jobs and innovation in the Single Market. The Digital Economy Research Programme is co-financed by the Directorate General Communications Networks, Content and Technology It aims to provide evidence-based scientific support to the European policy-making process. The scientific output expressed does not imply a policy position of the European Commission. Neither the European Commission nor any person acting on behalf of the Commission is responsible for the use which might be made of this publication. JRC Science Hub https://ec.europa.eu/jrc JRC98272 ISSN 1831-9408 (online) © European Union, 2015 Reproduction is authorised provided the source is acknowledged. All images © European Union 2015 How to cite: Melisande Cardona, Nestor Duch-Brown, Joseph Francois, Bertin Martens, Fan Yang (2015). The Macro-economic Impact of e-Commerce in the EU Digital Single Market. Institute for Prospective Technological Studies Digital Economy Working Paper 2015/09. JRC98272 Table of Contents Abstract ............................................................................................................... 3 1. Introduction .............................................................................................. 4 2. Online trade in goods in the EU ................................................................... -
GLOSSARY of INTERNATIONAL TRADE TERMS 2016 Guide
CALIFORNIA FASHION ASSOCIATION 444 South Flower Street, 37th Floor · Los Angeles, CA 90071 ·ph. 213.688.6288 ·fax 213.688.6290 Email: [email protected] Website: www.californiafashionassociation.org GLOSSARY OF INTERNATIONAL TRADE TERMS 2016 Guide Sponsored By: Prepared by: CALIFORNIA FASHION ASSOCIATION 444 South Flower Street, 37th Floor, Los Angeles, CA 90071 Phone: 213-688-6288, Fax: 213-688-6290 [email protected] | www.californiafashionassociation.org 1 CALIFORNIA FASHION ASSOCIATION 444 South Flower Street, 37th Floor · Los Angeles, CA 90071 ·ph. 213.688.6288 ·fax 213.688.6290 Email: [email protected] Website: www.californiafashionassociation.org THE VOICE OF THE CALIFORNIA INDUSTRY The California Fashion Association is the forum organized to address the issues of concern to our industry. Manufacturers, contractors, suppliers, educational institutions, allied associations and all apparel-related businesses benefit. Fashion is the largest manufacturing sector in Southern California. Nearly 13,548 firms are involved in fashion-related businesses in Los Angeles and Orange County; it is a $49.3-billion industry. The apparel and textile industry of the region employs approximately 128,148 people, directly and indirectly in Los Angeles and surrounding counties. The California Fashion Association is the clearinghouse for information and representation. We are a collective voice focused on the industry's continued growth, prosperity and competitive advantage, directed toward the promotion of global recognition for the "Created in California" -
Achieving High Coverage for Floating-Point Code Via Unconstrained Programming
Achieving High Coverage for Floating-Point Code via Unconstrained Programming Zhoulai Fu Zhendong Su University of California, Davis, USA [email protected] [email protected] Abstract have driven the research community to develop a spectrum Achieving high code coverage is essential in testing, which of automated testing techniques for achieving high code gives us confidence in code quality. Testing floating-point coverage. code usually requires painstaking efforts in handling floating- A significant challenge in coverage-based testing lies in point constraints, e.g., in symbolic execution. This paper turns the testing of numerical code, e.g., programs with floating- the challenge of testing floating-point code into the oppor- point arithmetic, non-linear variable relations, or external tunity of applying unconstrained programming — the math- function calls, such as logarithmic and trigonometric func- ematical solution for calculating function minimum points tions. Existing solutions include random testing [14, 23], over the entire search space. Our core insight is to derive a symbolic execution [17, 24], and various search-based strate- representing function from the floating-point program, any of gies [12, 25, 28, 31], which have found their way into many whose minimum points is a test input guaranteed to exercise mature implementations [16, 39]. Random testing is easy to a new branch of the tested program. This guarantee allows employ and fast, but ineffective in finding deep semantic is- us to achieve high coverage of the floating-point program by sues and handling large input spaces; symbolic execution and repeatedly minimizing the representing function. its variants can perform systematic path exploration, but suf- We have realized this approach in a tool called CoverMe fer from path explosion and are weak in dealing with complex and conducted an extensive evaluation of it on Sun’s C math program logic involving numerical constraints. -
Digital Economy 2002
Digital Economy 2002 ECONOMICS AND STATISTICS U.S. DEPARTMENT OF COMMERCE ADMINISTRATION Economics and Statistics Administration DIGITAL ECONOMY 2002 ECONOMICS AND STATISTICS ADMINISTRATION Office of Policy Development AUTHORS Chapter I ................................................................................................................................ Lee Price [email protected] George McKittrick [email protected] Chapter II..................................................................................................................... Patricia Buckley [email protected] Sabrina Montes [email protected] Chapter III ........................................................................................................................... David Henry [email protected] Donald Dalton [email protected] Chapter IV ................................................................................................................... Jesus Dumagan [email protected] Gurmukh Gill [email protected] Chapter V ....................................................................................................................... Sandra Cooke [email protected] Chapter VI .................................................................................................................... Dennis Pastore [email protected] Chapter VII ........................................................................................................ Jacqueline Savukinas [email protected] -
Credential Foreign National Holder of ARC (Resident Visa Not Included) 1
(starting July 27, 2021 Taipei Time) Traveler Type History of Travel Eligible to Enter Taiwan? Requirement upon Arrival Departure from 1. a COVID-19 RT-PCR negative test report issued within 3 working R.O.C. (Taiwan) any international Yes days of their incoming flight's scheduled departure time National port 2. strengthened quarantine measures *note1, 2 Eligible Traveler Type Credential to Enter Requirement • ARC means R.O.C. (Taiwan) Resident Certificate Taiwan? Holder of ARC 1. Open to all purposes of entry. 1. a COVID-19 RT-PCR negative test (Resident visa not Yes 2. Migrant worker must also hold re-entry permit. report issued within 3 working included) days of their incoming flight's Foreign scheduled departure time Who obtains entry permission for emergency or humanitarian National Yes 2. strengthened quarantine reason. *note1, 2 Non-ARC holder measures All others No Note: 1. All travelers are required to undergo 14-day quarantine after arrival. All travelers are required to arrange their location of quarantine prior to arrival and follow other regulations and instructions by Taiwan Centers of Disease Control. 2. Starting 00:00 on July 18 (time of arrival in Taiwan), arriving travelers from Brazil, India, the UK, Peru, Israel, Indonesia, Bangladesh and Myanmar (including those who have visited or transited in those countries in the past 14 days) will be required to quarantine in group quarantine facilities for 14 days and undergo PCR tests for COVID-19 upon checking in at group quarantine facilities and at the end of their quarantine period. 3. Beginning 00:00 am, May 19 (departure time at the airport of departure), non- R.O.C. -
Daily Life for the Common People of China, 1850 to 1950
Daily Life for the Common People of China, 1850 to 1950 Ronald Suleski - 978-90-04-36103-4 Downloaded from Brill.com04/05/2019 09:12:12AM via free access China Studies published for the institute for chinese studies, university of oxford Edited by Micah Muscolino (University of Oxford) volume 39 The titles published in this series are listed at brill.com/chs Ronald Suleski - 978-90-04-36103-4 Downloaded from Brill.com04/05/2019 09:12:12AM via free access Ronald Suleski - 978-90-04-36103-4 Downloaded from Brill.com04/05/2019 09:12:12AM via free access Ronald Suleski - 978-90-04-36103-4 Downloaded from Brill.com04/05/2019 09:12:12AM via free access Daily Life for the Common People of China, 1850 to 1950 Understanding Chaoben Culture By Ronald Suleski leiden | boston Ronald Suleski - 978-90-04-36103-4 Downloaded from Brill.com04/05/2019 09:12:12AM via free access This is an open access title distributed under the terms of the prevailing cc-by-nc License at the time of publication, which permits any non-commercial use, distribution, and reproduction in any medium, provided the original author(s) and source are credited. An electronic version of this book is freely available, thanks to the support of libraries working with Knowledge Unlatched. More information about the initiative can be found at www.knowledgeunlatched.org. Cover Image: Chaoben Covers. Photo by author. Library of Congress Cataloging-in-Publication Data Names: Suleski, Ronald Stanley, author. Title: Daily life for the common people of China, 1850 to 1950 : understanding Chaoben culture / By Ronald Suleski.