Proceedings of the 12Th Workshop on Asian Language Resources (ALR)
Total Page:16
File Type:pdf, Size:1020Kb
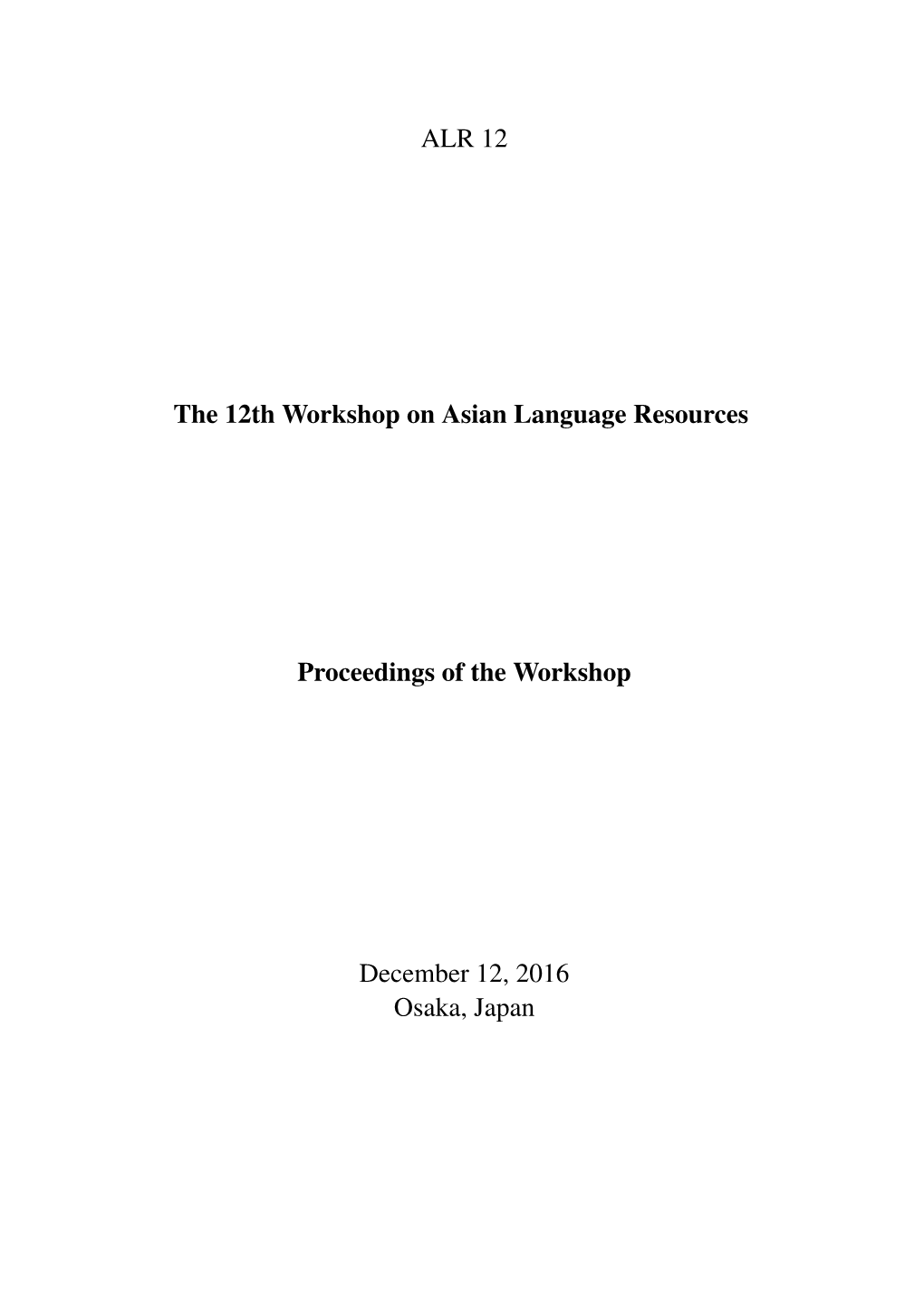
Load more
Recommended publications
-
Standards for Language Coding: the ISO 639 Family
Standards for language coding: the ISO 639 family Rebecca Guenther Library of Congress Jan. 8, 2010 ISO Standards development !! ISO consists of Technical Committees (TC) with subcommittees (SC) !! ISO language coding standards are maintained by !! TC 37/SC2 (Terminology and other language and content resources ) !! TC 46/SC4 (Information and documentation) LSA Annual Meeting 2 ISO 639 standards !! ISO 639-1: 2-character codes (136 codes) !! ISO 639-2: 3-character codes (450+) !! ISO 639-3: 3-character codes (7700+) !! ISO 639-4: principles !! ISO 639-5: 3-character codes (114) !! ISO 639-6: 4-character codes (??) LSA Annual Meeting 3 ISO 639 Joint Advisory Committee !! Established to advise the RAs for ISO 639-1 and ISO 639-2 !! Rotating chairs: Infoterm (for TC37) and Library of Congress (for TC46) !! Committee consists of 3 members of each TC, representatives of each registration authority and up to 6 observers !! Coordinates development of different parts of ISO 639 LSA Annual Meeting 4 ISO 639 language coding principles !! Language codes are not changed for stability of standard !! If a language code is retired it is not reassigned to something else !! Programming languages are not in scope !! Only deals with languages; codes from other ISO standards may be added as needed for more granularity, e.g. country codes, script codes LSA Annual Meeting 5 ISO 639-1 !! First published 1967 !! Covers major languages of the world !! Alpha-2 codes; only 676 possible combinations !! Developed for use in terminology applications !! Consists of -
Hiroshima-ICAN Academy on Nuclear Weapons and Global Security 2020
Hiroshima-ICAN Academy on Nuclear Weapons and Global Security 2020 Hiroshima - ICAN Academy on Nuclear Weapons and Global Security 2020 --- Contents --- Program Framework Profiles of Guest Educators and Coordinators Contact Persons Program Framework Introduction: Hiroshima Prefecture and the International Campaign to Abolish Nuclear Weapons ("ICAN"), the 2017 Nobel Peace Prize Laureate organization, will hold the Hiroshima-ICAN Academy on Nuclear Weapons and Global Security 2020. Through this training program, we aim to nurture global leaders to make concrete and peaceful contributions to the world. Due to the global spread of COVID-19, the Academy will be composed of two parts. Part 1 is the online learning course and webinars, and Part 2 the in-person training program to take place in Hiroshima City. The dates for Part 2 are to be confirmed after carefully examining the COVID-19 circumstances in Japan and overseas. Further information will be provided at the end of Part 1. Program Framework: The program of the Academy is designed around four themes. The first theme, “Humanitarian impact of nuclear weapons,” is a crucial component of the program, particularly given that the Academy is organized by Hiroshima Prefecture and Part 2 is physically held in Hiroshima. We believe all discussions on nuclear weapons and global security must begin here. The second theme is “Political, legal and technical aspects – nuclear weapons and global security.” Leading experts in the field will offer videos to watch and documents to read as learning materials that aim to shed light on various aspects of the theme. The third theme, “Civil society in action,” will take up a wide range of civil society efforts including education, awareness raising, and advocacy. -
Tags for Identifying Languages File:///C:/W3/International/Draft-Langtags/Draft-Phillips-Lan
Tags for Identifying Languages file:///C:/w3/International/draft-langtags/draft-phillips-lan... Network Working Group A. Phillips, Ed. TOC Internet-Draft webMethods, Inc. Expires: October 7, 2004 M. Davis IBM April 8, 2004 Tags for Identifying Languages draft-phillips-langtags-02 Status of this Memo This document is an Internet-Draft and is in full conformance with all provisions of Section 10 of RFC2026. Internet-Drafts are working documents of the Internet Engineering Task Force (IETF), its areas, and its working groups. Note that other groups may also distribute working documents as Internet-Drafts. Internet-Drafts are draft documents valid for a maximum of six months and may be updated, replaced, or obsoleted by other documents at any time. It is inappropriate to use Internet-Drafts as reference material or to cite them other than as "work in progress." The list of current Internet-Drafts can be accessed at http://www.ietf.org/ietf/1id-abstracts.txt. The list of Internet-Draft Shadow Directories can be accessed at http://www.ietf.org/shadow.html. This Internet-Draft will expire on October 7, 2004. Copyright Notice Copyright (C) The Internet Society (2004). All Rights Reserved. Abstract This document describes a language tag for use in cases where it is desired to indicate the language used in an information object, how to register values for use in this language tag, and a construct for matching such language tags, including user defined extensions for private interchange. Table of Contents 1. Introduction 2. The Language Tag 2.1 Syntax 2.2 Language Tag Sources 2.2.1 Pre-Existing RFC3066 Registrations 1 of 20 08/04/2004 11:03 Tags for Identifying Languages file:///C:/w3/International/draft-langtags/draft-phillips-lan.. -
Peace Culture Foundation Peace Culture
HIROSHIMA PEACE CULTURE FOUNDATION PEACE CULTURE Vol. 2 No.70 February 2014 (semiannual) 8th General Conference of Mayors for Peace The General Conference of Mayors for Peace, which takes what you have learned in Hiroshima”. place once every four years, was held in Hiroshima City from August 3-6 last year. Agenda I This was the 8th time that the conference has been held. A Mayor Matsui facilitated deliberations on five bills, includ- total of 305 people from 157 cities, 11 national governments ing the election of executive officials and the action plan from and 18 NGOs and other organizations from Japan and overseas 2013 to 2017. All proposals were passed without change. attended the conference, which had as its keynote theme “Toward a World Without Nuclear Weapons−Conveying the Agenda II ‘Spirit of Hiroshima and Nagasaki’ to the World”. Participants Mr. Yasuyoshi Komizo, Chairperson of this Foundation, discussed concrete initiatives to achieve the abolition of nucle- coordinated deliberations on the theme of “Future Initiatives ar weapons by 2020. for the 2020 Vision Campaign”. A report was given on activi- ties to date aiming for the abolition of nuclear weapons by Opening Ceremony 2020, and participants discussed future initiatives. At this session there were presentations on activities by the 2020 Vision Campaign Association, Japanese member cities, 2020 Vision campaigners, and Berlin University of Applied Sciences, which is running the Hiroshima-Nagasaki Peace Study Course. There were also proposals made on future pros- pects for the campaign. Agenda III Mayor Thore Vestby of Frogn City in Norway coordinated this session on the theme of “The Creation of Mayors for Peace Regional Groups and the Promotion of Joint Activities with Citizens”. -
Ethnologue: Languages of Honduras Twentieth Edition Data
Ethnologue: Languages of Honduras Twentieth edition data Gary F. Simons and Charles D. Fennig, Editors Based on information from the Ethnologue, 20th edition: Simons, Gary F. and Charles D. Fennig (eds.). 2017. Ethnologue: Languages of the World, Twentieth edition. Dallas, Texas: SIL International. Online: http://www.ethnologue.com. For personal use only Permission to distribute or reuse this work (in whole or in part) may be obtained through the Copyright Clearance Center at http://www.copyright.com. SIL International, 7500 West Camp Wisdom Road, Dallas, Texas 75236-5699 USA Web: www.sil.org, Phone: +1 972 708 7404, Email: [email protected] Ethnologue: Languages of Honduras 2 Contents List of Abbreviations 3 How to Use This Digest 4 Country Overview 6 Language Status Profile 7 Statistical Summaries 8 Alphabetical Listing of Languages 11 Language Map 14 Languages by Population 15 Languages by Status 16 Languages by Department 18 Languages by Family 19 Language Code Index 20 Language Name Index 21 Bibliography 22 Copyright © 2017 by SIL International All rights reserved. No part of this publication may be reproduced, redistributed, or transmitted in any form or by any means—electronic, mechanical, photocopying, recording, or otherwise—without the prior written permission of SIL International, with the exception of brief excerpts in articles or reviews. Ethnologue: Languages of Honduras 3 List of Abbreviations A Agent in constituent word order alt. alternate name for alt. dial. alternate dialect name for C Consonant in canonical syllable patterns CDE Convention against Discrimination in Education (1960) Class Language classification CPPDCE Convention on the Protection and Promotion of the Diversity of Cultural Expressions (2005) CSICH Convention for the Safeguarding of Intangible Cultural Heritage (2003) dial. -
Conference Agenda
25th United Nations Conference on Disarmament Issues Hiroshima, Japan 26 - 28 August, 2015 Theme: Towards the Realization of a World Free of Nuclear Weapons following the 2015 NPT Review Conference Tuesday, 25 August 2015 15:00 - 16:00 Advance Press Conference Participants: Mr. Thomas MARKRAM, Director of UNODA’s Geneva Branch, Deputy Secretary-General of the Conference on Disarmament Mr. Hidehiko YUZAKI, Governor of Hiroshima Prefecture Mr. Kazumi MATSUI, Mayor of Hiroshima City Ambassador Kazutoshi AIKAWA, Director General for Disarmament, Non- Proliferation and Science Department, Ministry of Foreign Affairs of Japan Master of ceremonies (MC): Dr. Yuriy KRYVONOS, Interim Director, United Nations Regional Center for Peace and Disarmament in Asia and the Pacific Wednesday, 26 August 2015 9:00 -13:00 Opening High-Level Session (Theme: Renewed Determination and Concrete Action towards the Realization of a World Free of Nuclear Weapons) - Video message from Mr. Ban Ki-moon, Secretary General of the United Nations - Welcoming Remarks: Mr. Kazumi MATSUI, Mayor of Hiroshima City Opening Remarks: Mr. Minoru KIUCHI, State Minister for Foreign Affairs of Japan Dr. Lassina ZERBO, Executive Secretary of the CTBTO Preparatory Commission Mr. Thomas MARKRAM, Director of UNODA’s Geneva Branch, Deputy Secretary-General of the Conference on Disarmament 9:40 – 11:20 Part 1: Towards the Realization of a World Free of Nuclear Weapons – Messages from the Sites of the Atomic Bombing - Video message from Mr. Michel DOUGLAS, UN Messenger of Peace - Moderator: Dr. Nobumasa AKIYAMA, Professor, Hitotsubashi University Panelists: Mr. William PERRY, Director of the Preventive Defense Project; Professor at Stanford University; Former Secretary of Defense Lord Des BROWNE, Vice-Chairman of the Nuclear Threat Initiative; Former UK Secretary of State for Defence Mr. -
An Analysis of Twentieth-Century Flute Sonatas by Ikuma Dan, Hikaru
Flute Repertoire from Japan: An Analysis of Twentieth-Century Flute Sonatas by Ikuma Dan, Hikaru Hayashi, and Akira Tamba D.M.A. Document Presented in Partial Fulfillment of the Requirements for the Degree Doctor of Musical Arts in the Graduate School of The Ohio State University By Daniel Ryan Gallagher, M.M. Graduate Program in Music The Ohio State University 2019 D.M.A. Document Committee: Professor Katherine Borst Jones, Advisor Dr. Arved Ashby Dr. Caroline Hartig Professor Karen Pierson 1 Copyrighted by Daniel Ryan Gallagher 2019 2 Abstract Despite the significant number of compositions by influential Japanese composers, Japanese flute repertoire remains largely unknown outside of Japan. Apart from standard unaccompanied works by Tōru Takemitsu and Kazuo Fukushima, other Japanese flute compositions have yet to establish a permanent place in the standard flute repertoire. The purpose of this document is to broaden awareness of Japanese flute compositions through the discussion, analysis, and evaluation of substantial flute sonatas by three important Japanese composers: Ikuma Dan (1924-2001), Hikaru Hayashi (1931- 2012), and Akira Tamba (b. 1932). A brief history of traditional Japanese flute music, a summary of Western influences in Japan’s musical development, and an overview of major Japanese flute compositions are included to provide historical and musical context for the composers and works in this document. Discussions on each composer’s background, flute works, and compositional style inform the following flute sonata analyses, which reveal the unique musical language and characteristics that qualify each work for inclusion in the standard flute repertoire. These analyses intend to increase awareness and performance of other Japanese flute compositions specifically and lesser- known repertoire generally. -
Who Is Who 1997
2nd Volume Convention on Climate Change Who is Who in the UNFCCC Process 1996 - 1997 FCCC Directory of Participants at Meetings of the Convention Bodies in the period July 1996 to December 1997 UN (COP2 - COP3) Contents Introduction page 3 Representatives of Countries page 5 Representatives of Observer Organizations page 259 Appendix I - Intergovernmental organizations accredited by the Conference of the Parties up to its third session page 482 Appendix II - Non-governmental organizations accredited by the Conference of the Parties up to its third session page 483 Appendix III - Alphabetical index of entries page 486 Appendix IV - Information update form page 523 1 2 Introduction This is the second volume of the Who’s Who in the UNFCCC Process. As indicated by its subtitle, this CC:INFO product is a directory of delegates and observers having attended the second or third sessions of the Conference of the Parties of the United Nations Framework Convention on Climate Change, or any of its subsidiary body meetings in between (COP2-COP3). This Who is Who was developed to provide those involved in the Climate Change process with a single, easy-to-use document, enabling them to renew or establish contact with each other. The Who is Who provides the title and contact information (e.g., institutional and e-mail addresses, direct telephone and fax numbers, etc…) for each individual, as provided to the secretariat during conference registration. Some of this information is now no longer valid, due to, e.g., new professional reassignments, including in some cases to the Climate Change Secretariat. -
Regions of Japan JETRO Toronto New Office
October 17, 2014 - vol.2 no.2 Inside this issue…. Executive Director’s Message Regions of Japan JETRO Toronto New Office Firstly, I would PM Speaks at JETRO Events JETRO encourages foreign companies looking to access like to introduce Focus on Regions of Japan the Japanese market to look beyond Tokyo and to consider myself as the new Success Stories Executive Direc- setting up in other areas of Japan. In this edition, we will Trade & Investment Report tor of JETRO focus on opportunities in the ‘Regions of Japan’. Toronto. Events & Programmes Having already GDP comparison of Japan’s regions with some countries Kazuo Nakamura lived in Canada Executive Director for about 7 years, Source: IMF“World Economic Outlook Database (April, 2012)”; “Prefectural Economic Almanac” (February, 2012), JETRO Toronto I have gained a Economic and Social Research Institute, Cabinet Office (from JETRO’s 3 Windows on Japan Presentation, 2012) (US $ billion, 2009) good understand- ing of Canada and Canadian industries. However, there is always more to learn and I look forward to my time in charge of JETRO Toronto promoting increased business ties between Canada and Japan. In other changes, we also welcomed a new Deputy Executive Director, Mr. Toshikazu Ito, and we moved our office to a new location. This edition of the newsletter will focus on the ‘Regions of Japan’. Many compa- nies looking to invest in Japan think first of setting up in Tokyo. Naturally, it is the capital of Japan and its largest city. However, there are many large regional markets where competition may be less intense and costs less. -
Policy of Cultural Affairs in Japan
Policy of Cultural Affairs in Japan Fiscal 2016 Contents I Foundations for Cultural Administration 1 The Organization of the Agency for Cultural Affairs .......................................................................................... 1 2 Fundamental Law for the Promotion of Culture and the Arts and Basic Policy on the Promotion of Culture and the Art ...... 2 3 Council for Cultural Affairs ........................................................................................................................................................ 5 4 Brief Overview of the Budget for the Agency for Cultural Affairs for FY 2016 .......................... 6 5 Commending Artistic and Related Personnel Achievement ...................................................................... 11 6 Cultural Publicity ............................................................................................................................................................................... 12 7 Private-Sector Support for the Arts and Culture .................................................................................................. 13 Policy of Cultural Affairs 8 Cultural Programs for Tokyo 2020 Olympic and Paralympic Games .................................................. 15 9 Efforts for Cultural Programs Taking into Account Changes Surrounding Culture and Arts ... 16 in Japan II Nurturing the Dramatic Arts 1 Effective Support for the Creative Activities of Performing Arts .......................................................... 17 2 -
8Th Annual Florida-Japan Summit
PRE-REGISTER NOW! Pre-registration is required for the Summit. Due to space limitations, registration will be closed when seating capacity has been Special Thanks to our Key Partner & Co-Sponsor reached. You may email your completed reg- Announcing the istration form to [email protected], or fax it to us at 786-235-8290, or mail it to us 8th Annual at: Southeast U.S./Japan Association Florida-Japan Summit P.O. Box 226647 Thanks also for additional support from: Miami, FL. 33222-6647 Yes, I wish to attend the 8th Annual Florida- Japan Summit. Please bill me for _____ regis- trations, which includes admission to both the Presented by summit presentation and networking recep- tion. (Complimentary for SEUS/Japan mem- bers; $20 for non-members; and $10 for stu- dents.) In cooperation with Consulate General of Japan in Miami Name 在マイアミ日本国総領事館 The University of West Florida Company/Organization Address November 15, 2011 City State Zip 3:00PM to 5:30PM at Phone Fax The Florida State University Email Turnbull Conference Center 555 W. Pensacola Street For more information please contact us at: Florida Delegation, Southeast U.S./Japan Association - Tallahassee, FL 32306-1640 Tel: 786-235-8289 Fax: 786-235-8290 Tel: (850) 644-2589 Email: [email protected] www.fl-seusjapan.org Jikei Group As the primary host and organizer of the Annual Florida-Japan Summit, Florida Dele- gation Southeast U.S./ T HE 8 TH A NNUAL F LORIDA - J APAN S UMMIT Japan Association is pleased to invite you to N OVEMBER 15, 2011 3:00 — 5 : 3 0 P M George Gabel join us for this impor- Chairperson tant annual event, to be The Honorable PROGRAM AGENDA The Honorable FL Delegation, held this year at The F. -
Estimation of Regional Business Cycle in Japan Using Bayesian Panel Spatial Autoregressive Probit Model∗
Estimation of Regional Business Cycle in Japan using Bayesian Panel Spatial Autoregressive Probit Model∗ Kazuhiko Kakamuy Hajime Wagoz Hisashi Tanizakix Abstract This paper considers the panel spatial autoregressive probit model from Bayesian point of view. The probit model is useful for qualitative (specifically, binary) data analyses. As it is well known, it is not easy to estimate the panel probit model by the maximum likelihood method. Therefore, in this paper, we consider the Markov chain Monte Carlo (MCMC) method to estimate the parameters as an alternative estima- tion method. Moreover, including the spatial autoregressive effect into the panel probit model we consider estimating the panel spatial autore- gressive probit model. Our model (i.e., the panel spatial autoregressive probit model) is illustrated with simulated data set and it is compared with the panel probit model. Furthermore, taking into account the ∗An earlier version of this paper was presented at the 2005 Japanese Joint Statistical Meeting at Hiroshima, the 2005 Fall meeting of Japanese Economic Association at Chuo University, MODSIM05 in Melbourne and ISBA08 in Hamilton Island. We would like to thank seminar/conference participants, especially Hideo Kozumi, Koji Miyawaki, Yasuhiro Omori, Mike K.P. So and Cathy W.S. Chen for their helpful comments and suggestions. However, responsibility for any errors remains entirely with us. This research was partially supported by the Ministry of Education, Science, Sports and Culture (Grant-in-Aid for Young Scientists (B) #20730144) for K. Kakamu and Japan Society for the Promotion of Science (Grant-in-Aid for Scientific Research (C) #18530158) for H. Tanizaki. yFaculty of Law and Economics, Chiba University, Inageku, Chiba 263-8522, Japan.