The Role of R&D and Technology Diffusion in Climate Change
Total Page:16
File Type:pdf, Size:1020Kb
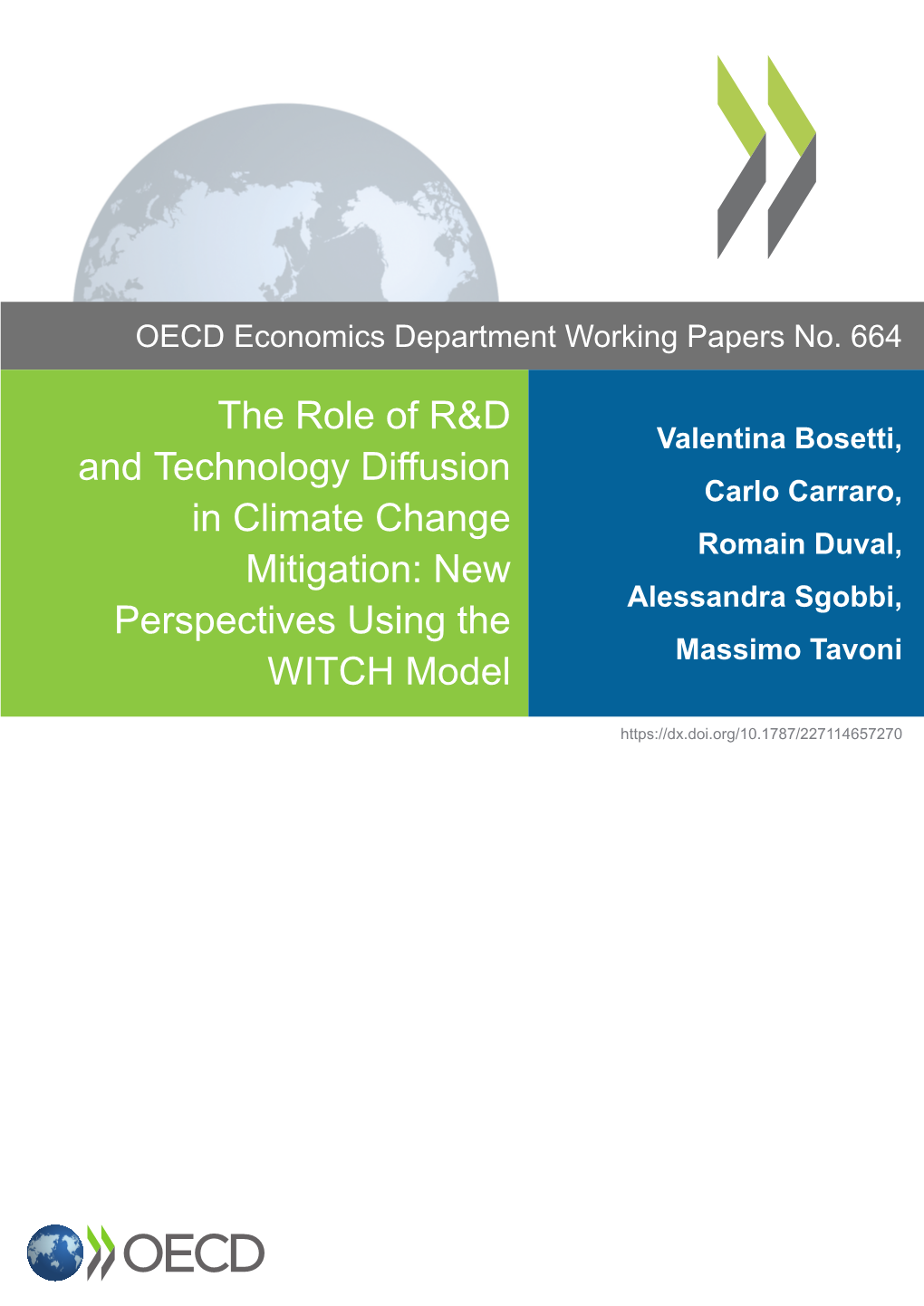
Load more
Recommended publications
-
The Right to Food and the Impact of Liquid Biofuels (Agrofuels) Photo by © FAO/18079/M
The Right to Food and the Impact of Liquid Biofuels (Agrofuels) Photo by © FAO/18079/M. Griffin RIGHT TO FOOD STUDIES Photo by © FAO/18079/M. Griffin The Right to Food and the Impact of Liquid Biofuels (Agrofuels) Asbjørn Eide FOOD AND AGRICULTURE ORGANIZATION OF THE UNITED NATIONS Rome, 2008 The designations employed and the presentation of material in this information product do not imply the expression of any opinion whatsoever on the part of the Food and Agriculture Organization of the United Nations (FAO) concerning the legal or development status of any country, territory, city or area or of its authorities, or concerning the delimitation of its frontiers or boundaries. The mention of specific companies or products of manufacturers, whether or not these have been patented, does not imply that these have been endorsed or recommended by FAO in preference to others of a similar nature that are not mentioned. ISBN 978-92-5-106174-9 All rights reserved. Reproduction and dissemination of material in this information product for educational or other non-commercial purposes are authorized without any prior written permission from the copyright holders provided the source is fully acknowledged. Reproduction of material in this information product for resale or other commercial purposes is prohibited without written permission of the copyright holders. Applications for such permission should be addressed to: Chief Electronic Publishing Policy and Support Branch Communication Division FAO Viale delle Terme di Caracalla, 00153 Rome, Italy or by e-mail to: [email protected] © FAO 2009 The Right to Food and the Impact of Liquid Biofuels (Agrofuels) Photo by © FAO/18079/M. -
Economic and Environmental Effectiveness of a Technology-Based Climate Protocol Barbara Buchner and Carlo Carraro
Economic and Environmental Effectiveness of a Technology-based Climate Protocol Barbara Buchner and Carlo Carraro NOTA DI LAVORO 61.2004 APRIL 2004 CCMP – Climate Change Modelling and Policy Barbara Buchner, Fondazione Eni Enrico Mattei Carlo Carraro, University of Venice, Fondazione Eni Enrico Mattei, CEPR, CEPS, CESifo This paper can be downloaded without charge at: The Fondazione Eni Enrico Mattei Note di Lavoro Series Index: http://www.feem.it/Feem/Pub/Publications/WPapers/default.htm Social Science Research Network Electronic Paper Collection: http://ssrn.com/abstract=XXXXXX The opinions expressed in this paper do not necessarily reflect the position of Fondazione Eni Enrico Mattei Economic and Environmental Effectiveness of a Technology-based Climate Protocol Summary The present stalemate in climate negotiations has led policy analysts and economists to explore the possible emergence of alternative climate regimes. This paper explores the idea of replacing international cooperation on greenhouse gas emission control with international cooperation on climate-related technological innovation and diffusion. This idea – recently proposed among others by Barrett (2001) and Benedick (2001) – is based on the insight that incentives to free-ride are much smaller in the case of technological cooperation than in the case of cooperation on emission control. This paper provides a first applied game theory analysis of a technology-based climate protocol by assessing: (i) the self-enforcingness (namely, the absence of incentives to free ride) of the coalition that would form when countries negotiate on climate-related technological cooperation; (ii) the environmental effectiveness of a technology-based climate protocol. The analysis is carried out by using a model in which endogenous and induced technical change are explicitly modelled and in which international technological spillovers are also quantified. -
An Analysis of Adaptation As a Response to Climate Change
COPENHAGEN CONSENSUS ON CLIMATE An Analysis of Adaptation as a Response to Climate Change Francesco Bosello, Carlo Carraro & Enrica De Cian COPENHAGEN CONSENSUS ON CLIMATE An Analysis of Adaptation as a Response to Climate Change Francesco Bosello, Carlo Carraro, and Enrica De Cian University of Venice and FEEM Acknowlwdgement: This report has been prepared for the Copenaghen Consensus 2009. AD-WITCH, the model used in this study, has been developed by FEEM in cooperation with the OECD. The authors gratefully acknowledge their financial support. They are also grateful to Shardul Agrawala, Rob Dellink, Kelly de Bruin and Richard Tol for helpful comments. Nonetheless, the views expressed in this paper are the authors’ sole responsibility. Finally, the contribution of all colleagues who worked to the development of the original WITCH model – in particular Valentina Bosetti, Emanuele Massetti, and Massimo Tavoni – is gratefully acknowledged. Corre- spondence address: Carlo Carraro, FEEM, Castello 5252, 30122 Venice, Italy. E mail: [email protected]. Copenhagen Consensus Center Copenhagen Business School Solbjerg Plads 3 DK-2000 Frederiksberg Denmark +45 3815 2255 [email protected] www.copenhagenconsensus.com COPENHAGEN CONSENSUS ON CLIMATE PREFACE ABSTRACT Climate change is likely to have relevant effects on our future socio-economic systems. It is therefore important to identify how markets and policy jointly react to expected climate change to protect our societies and well-being. This study addresses this issue by carrying out an integrated analysis of both optimal mitigation and adaptation at the global and regional level. Adaptation responses are disentangled into three different modes: reactive adaptation, proactive (or anticipatory) adaptation, and investments in innovation for adaptation purposes. -
Literally, Stories of Climate Change
NONPROFIT CIVIL SOCIETY CSR SOCIAL ENTERPRISE PHILAntHropy 18 Winds of Change 24 The Birds and the Bees: Lessons from a Social Enterprise 36 Face-Off: End-of-Life Ideas for Plastic 52 Short Fiction: Monarch Blue Edition 27 | JAN-MAR 2019 | /AsianNGO | www.asianngo.org/magazine | US$10 It’s not all doom and gloom Find nature conservation stories with a happy ending at: Table of Contents 24 the Birds and the Bees: LessOns FrOm a SociaL enterPrise 34 PhOtO FEATURE: Last Forest Enterprises is a social initiative based in South India that supports communities dependent on biodiversity for their livelihood. iMPACT traces their women and the journey, and some lessons they learned along the way. envirOnment PHOTO CREDITS Graphics, stock photos by flaticon.com, freepik.com, 123rf.com, Pixabay, Unsplash, Pexels, Ten Photos to Shake the World and Getty Images • Aadhimalai Pazhangudiyinar Producer Co. Ltd. • ABC Central Victoria: Larissa Romensky • B&T Magazine • BioCote • Canopy • Colossal • Conservation International • Digital Green 18 Winds of change 37 Face-Off: end- • Endangered Emoji/World Wide Fund For Nature • Florence Geyevu of-LiFe ideas for • Ian Kelly Jamotillo Renewable energy, despite its promise • Last Forest Enterprises of a cleaner planet, is not without its • Lensational PLastic • Misper Apawu problems. Meera Rajagopalan explores • National Wildlife Federation wind energy and its effect on bird Plastic pollution is putting countries • Sanna Lindberg in danger, yet improper waste • SDF fatalities, and how organizations such • Sasmuan Bankung Malapad Critical Habitat as Birdlife International promote clean disposal continues. iMPACT takes a Ecotourism Area (SBMCHEA) look at three possible solutions for • The Elephants & Bees Project / Lucy King energy from a biodiversity prospective. -
Technologies to Sustain Forest Resources
Technologies To Sustain Forest Resources March 1984 OTA-F-515 NTIS order #PB92-182104 Recommended Citation: Technologies to Sustain Tropical Forest Resources (Washington, D. C.: U.S. Congress, Office of Technology Assessment, OTA-F-214, March 1984). Library of Congress Catalog Card Number 84-601018 For sale by the Superintendent of Documents U.S. Government Printing Office, Washington, D.C. 20402 Foreword The United States has a stake in the sustained economic development of tropical nations for humanitarian, political, and economic reasons. To a great extent, the development of these nations depends on increasing production from their poten- tially renewable soil, forest, and water resources. But tropical forest resources, which cover nearly one-half of the tropical nations’ land, are being consumed at a rate that may make them nonrenewable. They are exploited for timber and cleared for pasture and cropland with little regard for their abilities to produce—in a long- term sustainable fashion—important goods, maintain soil productivity, regulate water regimes, or regenerate themselves. Much of the recent deforestation occurs where the new land uses cannot be sustained and it causes productivity losses that tropical nations and the world can ill afford. International recognition of the importance of tropical forests, and efforts to sustain the productivity of these resources, have increased significantly in the last decade. In 1980, the House of Representatives Committee on Foreign Affairs, Sub- committee on International Organizations, held hearings on tropical deforestation. The committee then requested the Office of Technology Assessment (OTA) to con- duct a more thorough assessment of the problem, the technologies that could help sustain tropical forest resources, and possible options for Congress. -
Observe and Replicate Natural Patterns
THE PERMACULTURE ETHICS Care of Earth Care of Humans Share the Surplus Respect Intrinsic Value THE PRINCIPLES OF PERMACULTURE from Bill Mollison & David Holmgren Observe and Interact The primary design tool is the designer. Beauty is in the eye of the beholder. Observation is a short way of saying, “Noticing the information coming from all your senses as they experience and interact with your environment, both inner and outer.” The root of this principle is the willingness to begin designing a culture based on co-creation with Nature, while acknowledging the limitations of our present cultural norm of separation from Nature. It is the willingness to Dance with Nature, learning when to interfere, when not to; learning where limitations and abundances exist, and when; and how to intermesh our human-ness into an ecosystem to meet our needs and help it work better for All. OBSERVATION PRACTICES: sit spot, meditation, breathing awareness, journaling, Nature Walks [learning about the flora and fauna that live around you], Herb Walks [learning about wild edibles and medicinals], learning tracking/hunting skills, learning about the movement of the constellations and the moon. Obtain a Yield You can’t work on an empty stomach. [and neither can anyone else] Definition of system yield: System yield is the sum total of surplus energy produced by, stored, conserved, reused or converted by the design. Energy is in surplus once the system itself has available all it needs for growth, reproduction and maintenance. As PC designers, we are creating “cultivated ecosystems” – cultivated to produce a yield of food, shelter, fiber, medicine, or other product/service useful to humans. -
GIORGIO BARBA NAVARETTI CURRICULUM VITAE January 2019
GIORGIO BARBA NAVARETTI CURRICULUM VITAE January 2019 Department of Economics Management and Quantative Methods, University of Milan, Via Conservatorio 7, 20122 Milano, tel +390236683850, mob +393487064160, email [email protected] Born in Torino, Italy, the 5th of June 1960, Nationality: Italian – French EDUCATION 1987/1991 University of Oxford, D. Phil. in Economics 1985-87 University of Oxford, M.Phil. in Economics 1982 ESSEC, Cergy Pontoise (Paris), Exchange 1979-1984 Università Commerciale Luigi Bocconi Milano, Italy, Laurea in Business Economics, CAREER ACADEMIC AND RESEARCH 2012- SciencesPo, Paris, Distinguished Visiting Faculty 2001 - Università degli Studi, Milano, Full Professor in International Economics 1987- Centro Studi Luca d'Agliano, Milan and Turin, Scientific Director since 1998 1998- 2001 Università degli Studi, Ancona, Associate Professor in Economics 1993-1998 Università degli Studi, Milano, Assistant Professor in Economics 1992- 2001 Fondazione Eni Enrico Mattei, Milan, Research Programme Coordinator and Scientific Editor 1984-85 Università Commerciale Luigi Bocconi, Milan, Italy. Researcher and teaching assistant in industrial economics Paris School of Economics (2009) and Boston College (2005) Invited Visiting Professor The World Bank (1995-6 and 1999-2000) Invited Visiting Researcher CORPORATE 2015- Ricerca e Studi (R&S), Mediobanca, Non-Executive Director 2006 - Aon Italia, Non-Executive Director 2012-2018 Banca Sistema, Non-Executive Director (member of the Executive Committee 2012- of the Internal Control and Risks -
K-12 Educational Technology Curriculum Reference Guide
PLAINVIEW-OLD BETHPAGE CENTRAL SCHOOL DISTRICT K-12 EDUCATIONAL TECHNOLOGY CURRICULUM REFERENCE GUIDE Mrs. Marjorie Alford, Middle School Teacher Ms. Joanne Levy, Elementary Library Media Specialist Mr. Michael Secko, High School Teacher & Mr. Guy A. Lodico, Director of Technology 1 Plainview-Old Bethpage Central School District 106 Washington Avenue, Plainview, New York 11803 Mission Statement The mission of the Plainview-Old Bethpage School District is to provide an academically challenging and stimulating environment for all students, and to enable them to realize their full potential to be happy, ethical and analytical citizens of the world. We do this by: making tolerance, acceptance, respect, honesty and kindness expectations for all students and for members of the Plainview-Old Bethpage school community; identifying each student’s academic, social-emotional, aesthetic and physical needs, and striving to meet those needs; and encouraging communication between and among students, teachers, parents, administrators, and community members. 2 Table of Contents: Introduction 4 Vision Statement / K-12 Technology Curriculum Integration Structure 6-7 Student Technology Assessment Rubric 11 Staff Development Needs 10-12 K-8 Computer Technology Skills 12-19 K-6 Computer Terminology 20-23 K-6 Web Sites 24-27 Seventh & Eighth Grade 27-35 Ninth to Twelfth Grade 36 English Curriculum 36-40 Mathematics Curriculum 41-42 Science Curriculum 43-45 Social Studies Curriculum 45-47 Modern Language Curriculum 47-51 Tools for Developing Internet Materials -
MARKET RESEARCH for FOOD Products and PROCESSES IN
MARKET RESEARCH FOR FOOD PRODUCTS AND PROCESSES IN DEVELOPING COUNTRIES The International Development Research Centre is a public corporation created by the Parliament of Canada in 1970 to support research designed to adapt science and technology to the needs of developing countries. The Centre's activity is concentrated in five sectors: agriculture, food and nutrition sciences; health sciences; information sciences; social sciences; and communi- cations. IDRC is financed solely by the Parliament of Canada; its policies, however, are set by an international Board of Governors. The Centres head- quarters are in Ottawa, Canada. Regional offices are located in Africa, Asia, Latin America, and the Middle East. MARKET RESEARCH FOR FOOD PRODUcTS AND PROCESSES IN DEVELOPING COUNTRIES PROCEEDINGS OF A WORKSHOP HELD IN SINGAPORE, 1-4 APRIL 1986 EDITORS: R.H. YOUNG AND C.W. MACCORMAC ` International Development Research Centre 1987 Postal Address: PO. Box 8500, Ottawa, Ont., Canada K1G 3H9 Young, R.H. MacCormac, C.W. IDRC-249e Market research for food products and processes in developing countries : proceedings of a workshop held in Singapore, 1-4 April 1986. Ottawa, Ont., IDRC, 1987. xii + 144 p.: ill. /Market studies/, /research and development/, /food technology/, /post-harvest systems/, /Asia/ - /human nutrition/, /small-scale industry/, /fish processing/, /drying/, /social aspects/, /case studies/, /conference reports/, lists of participants/, /references/. UDC: 380.13:664 ISBN 0-88936-478-8 Technical Editor: K. Kealey-Vallière A microfiche edition is available. The views expressed in this publication are those of the authors and do not necessarily represent those of the International Development Research Centre. Mention of proprietary names does not constitute endorsement of the product and is given only for information. -
Curriculum Vitae
CURRICULUM VITAE Professor CARLO CARRARO Università Ca’ Foscari Venezia President, European Association of Environmental and Resource Economists Ph.D, Princeton University www.carlocarraro.org PERSONAL INFORMATION AND ADDRESSES Professor President Department of Environmental and European Association of Environmental Computer Science and Resource Economists (EAERE) Science Campus, Via Torino 155 Edificio Porta dell'Innovazione - Piano 2 30170 Venezia-Mestre, Italy Via della Libertà, 12 - 30175 Venezia- Tel.: +39 041 2348198 Marghera, Italy E-mail: [email protected] Website: www.eaere.org Website: www.unive.it/persone/ccarraro President Vice-Chair, Working Group III HforHuman Foundation Intergovernmental Panel on Climate Via Sile 6, 31056, Roncade (TV) Italy Change (IPCC) Tel: +39 0422 789611 C/O World Meteorological Organization E-mail: [email protected] 7bis Avenue de la Paix, C.P. 2300 Website: https://hforhuman.org/ CH- 1211 Geneva 2, Switzerland E-mail: [email protected] Co-Chair, GGKP Scientific Director Green Growth Knowledge Platform Fondazione Nord Est International Environment House Via Torino 151c 11-13 Chemin des Anemones 30172 Venezia-Mestre, Italy 1219 Geneva, Switzerland Tel: 041 2517556 http://www.greengrowthknowledge.org Website: www.fondazionenordest.net SHORT PROFILE Professor Carlo Carraro is President Emeritus and Professor of Environmental Economics at Ca’ Foscari University of Venice. He holds a Ph.D. from Princeton University. He was President of the University of Venice from 2009 to 2014. Previously, he was Director of the Department of Economics from 2006 to 2008 and Vice-Provost for Research Management and Policy from 2001 to 2005. He is President of the European Association of Environmental and Resource Economists (EAERE) for the biennium 2018-2019. -
Implications for the Use of Biofuels with Special Reference to the Caribbean
L IM IT E D LC/C AR/L. 148 14 December 2007 ORIGINAL: ENGLISH IMPLICATIONS FOR THE USE OF BIOFUELS WITH SPECIAL REFERENCE TO THE CARIBBEAN This document has been reproduced without formal editing. Table of contents I. What are Biofuels?............................................................................................................................................. 1 II. Why Biofuels?....................................................................................................................................................2 A. Finite reserves.............................................................................................................................................. 2 B. Environmental pollution...........................................................................................................................3 C. Land benefits........................................................................................................................................... 3 D. Security of supply..................................................................................................................................4 III. Beyond Biofuels...............................................................................................................................................4 IV. Implications for Use of Biofuels................................................................................................................. 5 A. Global warming?..........................................................................................................................................5 -
International Energy R&D Spillovers and the Economics of Greenhouse Gas Atmospheric Stabilization
Fondazione Eni Enrico Mattei Working Papers 8-21-2007 International Energy R&D Spillovers and the Economics of Greenhouse Gas Atmospheric Stabilization Valentina Bosetti Fondazione Eni Enrico Mattei, [email protected] Carlo Carraro Fondazione Eni Enrico Mattei, University of Venice, CEPR, CESifo and CMCC, [email protected] Emanuele Massetti Fondazione Eni Enrico Mattei and Università Cattolica del Sacro Cuore, [email protected] Massimo Tavoni Fondazione Eni Enrico Mattei , Catholic University of Milan and CMCC, [email protected] Follow this and additional works at: http://services.bepress.com/feem Recommended Citation Bosetti, Valentina; Carraro, Carlo; Massetti, Emanuele; and Tavoni, Massimo , "International Energy R&D Spillovers and the Economics of Greenhouse Gas Atmospheric Stabilization" (August 21, 2007). Fondazione Eni Enrico Mattei Working Papers. Paper 138. http://services.bepress.com/feem/paper138 This working paper site is hosted by bepress. Copyright © 2007 by the author(s). Bosetti et al.: International Energy R&D Spillovers and the Economics of Gre International Energy R&D Spillovers and the Economics of Greenhouse Gas Atmospheric Stabilization Valentina Bosetti, Carlo Carraro, Emanuele Massetti, and Massimo Tavoni NOTA DI LAVORO 82.2007 JULY 2007 CCMP – Climate Change Modelling and Policy Valentina Bosetti, Fondazione Eni Enrico Mattei and CMCC Carlo Carraro, Fondazione Eni Enrico Mattei, University of Venice, CEPR, CESifo and CMCC Emanuele Massetti and Massimo Tavoni, Fondazione Eni Enrico Mattei,