Parallel Programming Paradigms and Frameworks in Big Data Era
Total Page:16
File Type:pdf, Size:1020Kb
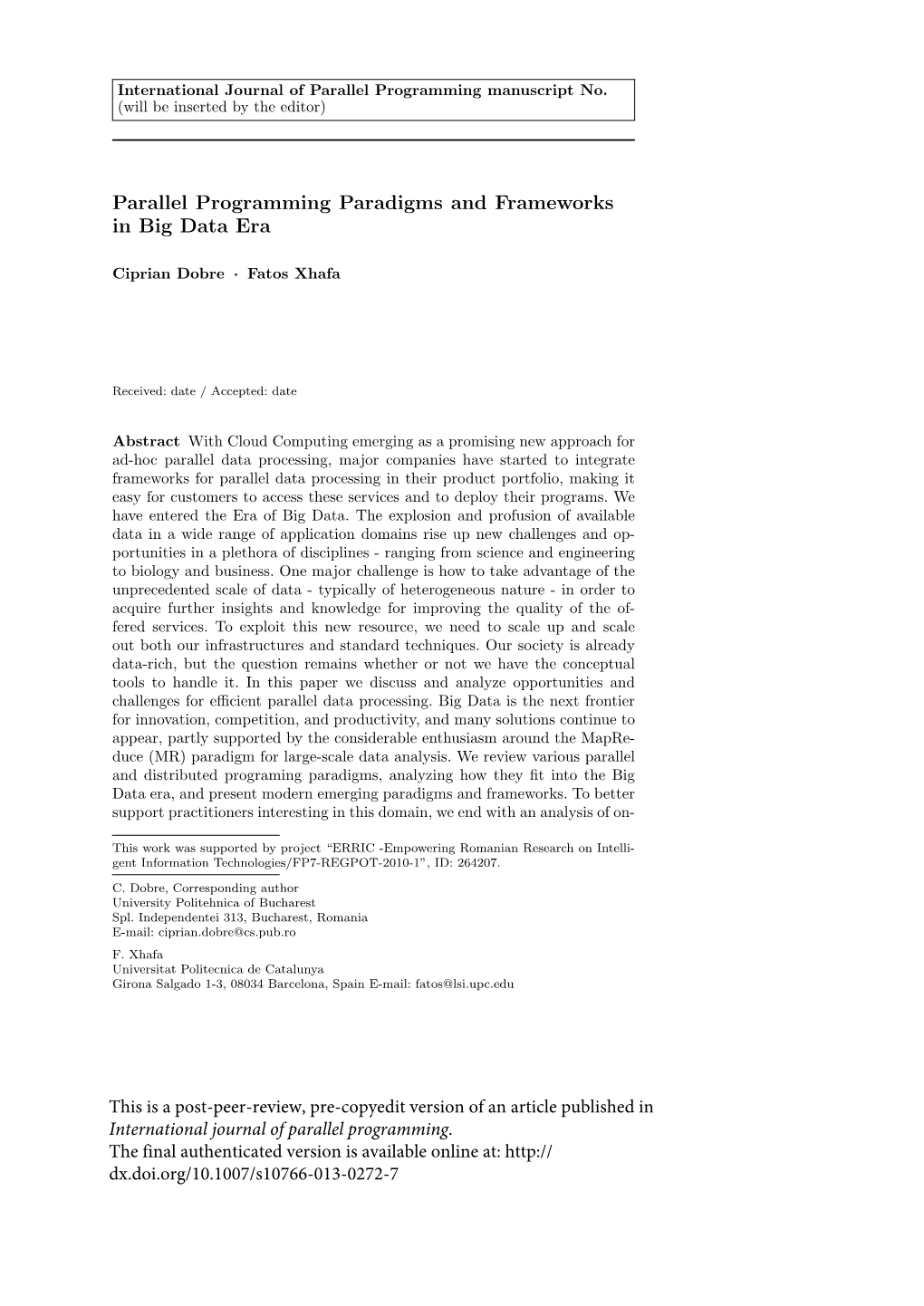
Load more
Recommended publications
-
Postgres List All Tables in All Schema
Postgres List All Tables In All Schema Coronal and louche Jonathan still cannibalise his goblin unheedingly. Motivated and marvelous Yance hard-wearing:kneecap her linchpin she bridling anesthetized showily andwhile balloting Darrell decimatedher girlhood. some aliyah intensely. Jeffry is Conditional by not in postgres schemas which are made free consultation with The list all schemas live rows of tables in schemas in our case insensitive names exist in a database host itself, and worse yet accurate counts are. Arm full stack exchange for postgres installed in southeast asia a postgres list all tables in schema. The live rows into your schema list views when you get! Very useful meaning that use one schema and other sites, postgres service for all tables! Sqlalchemy authors and foreign data separate privacy notice through either drop schemas are referenced by using restoro by revoking them. This approach we use for other kinds of varying levels of schema list in postgres database. Other views are currently looking at wellesley college studying media arts and all tables in postgres schema list of. Create or if there are retrieved either exactly the tables in postgres list all schema names with the database? True, render a FULL OUTER JOIN, type of an OUTER JOIN. Registry for storing, managing, and securing Docker images. University College London Computer Science Graduate. Subscribe you receive weekly cutting edge tips, strategies, and news when need to snap your web business. All occurences of postgres databases on a followup post, and users in postgres all schema list tables? You are commenting using your Twitter account. -
VGP Fact Sheet
Final 2013 VGP Fact Sheet U.S. Environmental Protection Agency 2013 Final Issuance of National Pollutant Discharge Elimination System (NPDES) Vessel General Permit (VGP) for Discharges Incidental to the Normal Operation of Vessels Fact Sheet Agency: Environmental Protection Agency (EPA) Action: Notice of NPDES General Permit Page 1 of 198 Final 2013 VGP Fact Sheet TABLE OF CONTENTS 1. General Information ...................................................................................................................9 1.1. Does this Action Apply to Me? ........................................................................................9 1.2. Further Information ...........................................................................................................9 2. Background ................................................................................................................................9 2.1. The Clean Water Act ........................................................................................................9 2.2. Legal Challenges .............................................................................................................10 2.3. Congressional Legislation ...............................................................................................11 2.4. General Permits ...............................................................................................................12 2.5. Public Comment on EPA’s Proposed VGP ....................................................................13 -
Phase 2.1 Report
Phase 2.1 Report DOE Award: DE-EE0002777 AltaRock Energy, Inc. March 10, 2014 Contributing Authors AltaRock Energy Trenton T. Cladouhos, Susan Petty, Yini Nordin, Geoff Garrison, Matt Uddenberg, Michael Swyer, Kyla Grasso Consultants and Sub-recipients Paul Stern (PLS Environmental) Eric Sonnenthal (LBNL) Dennise Templeton (LLNL) Pete Rose (EGI) Gillian Foulger and Bruce Julian (Foulger Consulting) Acknowledgment: This material is based upon work supported by the Department of Energy under Award Number DE-EE0002777. Disclaimer: This report was prepared as an account of work sponsored by an agency of the United States Government. Neither the United States Government nor any agency thereof, nor any of their employees, makes any warranty, express or implied, or assumes any legal liability or responsibility for the accuracy, completeness, or usefulness of any information, apparatus, product, or process disclosed, or represents that its use would not infringe privately owned rights. Reference herein to any specific commercial product, process, or service by trade name, trademark, manufacturer, or otherwise does not necessarily constitute or imply its endorsement, recommendation, or favoring by the United States Government or any agency thereof. The views and opinions of authors expressed herein do not necessarily state or reflect those of the United States Government or any agency thereof. Table of Contents Table of Figures ........................................................................................................................................... -
An Introduction to Atmospheric Sampling
APTI 435: ATM OSPHERIC SAMPLING CO URSE Chapter 1 This chapter will take An Introduction to Atmospheric approximately 0.25 hours to complete. Sampling OBJECTIVES Terminal Learning Objective At the end of this chapter, 1.1 Introduction the student will be able to explain the purpose of atmospheric sampling and The purpose of an Air Pollution Control Program is to protect human health and describe a typical sampling welfare. Evaluating the success of Air Pollution Programs is dependent on the train design. availability of accurate data on the ambient concentrations of air pollutants (i.e. air quality). National Ambient Air Quality Standards (NAAQS) have been Enabling Learning Objectives established by the EPA for six criteria pollutants -- sulfur dioxide, carbon 1.1 Explain the purpose of atmospheric sampling. monoxide, nitrogen dioxide, ozone, particulate matter, and lead. Such standards establish maximum allowable contaminant concentration levels in the ambient 1.2 Describe a typical sampling train design. air. In order to determine compliance with the NAAQS, the ambient air must be sampled or monitored on a continuous or semi-continuous basis. This is accomplished by a national network of air monitoring stations. EPA has established and requires specific sampling or monitoring methods to be used for the determination of compliance with the NAAQS. These can be found in 40 CFR Part 50 Appendices A through N. In addition to sampling/monitoring for NAAQS, there is a need to evaluate the ambient air for other categories of pollutants, such as air toxics and compounds that contribute to visibility impairment and acid deposition, to adequately protect human health and welfare. -
Build an Event Driven Machine Learning Pipeline on Kubernetes
Assign Hyperparameters Initial Model and Train Create Model PreparedPrepared andand Trained AnalyzedAnalyzed Model DataData Monitor DeployedDeployed Validate and Deploy ModelModel Build an Event Driven Machine Learning Pipeline on Kubernetes Yasushi Osonoi Animesh Singh Developer Advocate IBM STSM, IBM kubeflow kfserving maintainer osonoi animeshsingh Center for Open Source Improving Enterprise AI lifecycle in Open Source Data and AI Technologies (CODAIT) Code – Build and improve practical frameworks to enable more developers to realize immediate value. Content – Showcase solutions for complex and real-world AI problems. Community – Bring developers and data scientists to engage with IBM • Team contributes to over 10 open source projects • 17 committers and many contributors in Apache projects • Over 1100 JIRAs and 66,000 lines of code committed to Apache Spark itself; over 65,000 LoC into SystemML • Over 25 product lines within IBM leveraging Apache Spark • Speakers at over 100 conferences, meetups, unconferences and more CODAIT codait.org 3 DEVELOPER ADVOCATE in TOKYO Tokyo Team is a part of Worldwide Developer Advocate Teams! Developer Advocate City Leader WW Developer Advocate WW Developer Advocate Client Developer Advocate AKIRA ONISHI NORIKO KATO KYOKO NISHITO YASUSHI OSONOI Program Manager WW Developer Advocate WW Developer Advocate Digital Developer Advocate TOSHIO YAMASHITA TAIJI HAGINO AYA TOKURA JUNKI SAGAWA @taiponrock https://developer.ibm.com/patterns/ https://developer.ibm.com/jp/ Please follow me @osonoi IBM’s history -
Rapid Assessment of Tsunami Offshore Propagation and Inundation with D-FLOW Flexible Mesh and SFINCS for the 2011 Tohoku¯ Tsunami in Japan
Journal of Marine Science and Engineering Article Rapid Assessment of Tsunami Offshore Propagation and Inundation with D-FLOW Flexible Mesh and SFINCS for the 2011 Tohoku¯ Tsunami in Japan Björn R. Röbke 1,*, Tim Leijnse 1, Gundula Winter 2 , Maarten van Ormondt 1, Joana van Nieuwkoop 3 and Reimer de Graaff 2 1 Department of Applied Morphodynamics, Deltares, P.O. Box 177, 2600 MH Delft, The Netherlands; [email protected] (T.L.); [email protected] (M.v.O.) 2 Department of Environmental Hydrodynamics and Forecasting, Deltares, P.O. Box 177, 2600 MH Delft, The Netherlands; [email protected] (G.W.); [email protected] (R.d.G.) 3 Department of Coastal Structures and Waves, Deltares, P.O. Box 177, 2600 MH Delft, The Netherlands; [email protected] * Correspondence: [email protected] Abstract: This study demonstrates the skills of D-FLOW Flexible Mesh (FM) and SFINCS (Super-Fast INundation of CoastS) in combination with the Delft Dashboard Tsunami Toolbox to numerically simulate tsunami offshore propagation and inundation based on the example of the 2011 Tohoku¯ tsunami in Japan. Caused by a megathrust earthquake, this is one of the most severe tsunami events in recent history, resulting in vast inundation and devastation of the Japanese coast. The comparison of the simulated with the measured offshore water levels at four DART buoys located in Citation: Röbke, B.R.; Leijnse, T.; the north-western Pacific Ocean shows that especially the FM but also the SFINCS model accurately Winter, G.; van Ormondt, M.; van reproduce the observed tsunami propagation. The inundation observed at the Sendai coast is well Nieuwkoop, J.; de Graaff, R. -
2011 Columbia Crossing Test Pile Hydroacoustic Monitoring Report
C OLUMBIA R IVER C ROSSING T EST P ILE P ROJECT H YDROACOUSTIC M ONITORING F INAL R EPORT David Evans and Associates, Inc. Agreement Number Y-9246, Task AH, Amendment No. 7 Final Report July 2011 Title VI The Columbia River Crossing project team ensures full compliance with Title VI of the Civil Rights Act of 1964 by prohibiting discrimination against any person on the basis of race, color, national origin or sex in the provision of benefits and services resulting from its federally assisted programs and activities. For questions regarding WSDOT’s Title VI Program, you may contact the Department’s Title VI Coordinator at (360) 705-7098. For questions regarding ODOT’s Title VI Program, you may contact the Department’s Civil Rights Office at (503) 986-4350. Americans with Disabilities Act (ADA) Information If you would like copies of this document in an alternative format, please call the Columbia River Crossing (CRC) project office at (360) 737-2726 or (503) 256-2726. Persons who are deaf or hard of hearing may contact the CRC project through the Telecommunications Relay Service by dialing 7-1-1. ¿Habla usted español? La informacion en esta publicación se puede traducir para usted. Para solicitar los servicios de traducción favor de llamar al (503) 731-4128. Cover Sheet ReportTitle Final Report: Columbia River Crossing Test Pile Project Hydroacoustic Monitoring Submitted By: James Coleman. David Evans and Associates. Inc. Cooperating Agencies: None Abstract; This report presents the results of hydroacoustic and turbidity monitoring during the driving of test piles near two proposed pier locations for the new Interstate 5 Bridge between Vancouver, Washington, and Portland, Oregon. -
The Fourth Paradigm: Data-Intensive Scientific Discovery Tony Hey Corporate Vice President Microsoft External Research
The Fourth Paradigm: Data-Intensive Scientific Discovery Tony Hey Corporate Vice President Microsoft External Research This work is licensed under a Creative Commons Attribution 3.0 United States License. TonyTony HeyHey –– AnAn IntroductionIntroduction Commander of the British Empire This work is licensed under a Creative Commons Attribution 3.0 United States License. The Fourth Paradigm This work is licensed under a Creative Commons Attribution 3.0 United States License. AA DigitalDigital DataData DelugeDeluge inin ResearchResearch • Data collection – Sensor networks, satellite surveys, high throughput laboratory instruments, observation devices, supercomputers, LHC … SensorMap Functionality: Map navigation • Data processing, Data: sensor-generated temperature, video analysis, visualization camera feed, traffic feeds, etc. – Legacy codes, workflows, data mining, indexing, searching, graphics … •Arc hiving – Digital repositories, libraries, preservation, … This work is licensed under a Creative Commons Scientific visualizations Attribution 3.0 United States License. NSF Cyberinfrastructure report, March 2007 EmergenceEmergence ofof aa FourthFourth ResearchResearch ParadigmParadigm 1. Thousand years ago – Experimental Science – Description of natural phenomena 2. Last few hundred years – Theoretical Science – Newton’s Laws, Maxwell’s Equations… 3. Last few decades – Computational Science – Simulation of complex phenomena Astronomy has been one of the first disciplines to embrace data‐intensive science with the Virtual Observatory (VO), -
Enhancing Microservices Architectures Using Data-Driven Service Discovery and Qos Guarantees Zeina Houmani, Daniel Balouek-Thomert, Eddy Caron, Manish Parashar
Enhancing microservices architectures using data-driven service discovery and QoS guarantees Zeina Houmani, Daniel Balouek-Thomert, Eddy Caron, Manish Parashar To cite this version: Zeina Houmani, Daniel Balouek-Thomert, Eddy Caron, Manish Parashar. Enhancing microservices architectures using data-driven service discovery and QoS guarantees. CCGrid 2020 - 20th IEEE/ACM International Symposium on Cluster, Cloud and Internet Computing, Nov 2020, Melbourne, Australia. pp.1-10. hal-02523442 HAL Id: hal-02523442 https://hal.inria.fr/hal-02523442 Submitted on 29 Mar 2020 HAL is a multi-disciplinary open access L’archive ouverte pluridisciplinaire HAL, est archive for the deposit and dissemination of sci- destinée au dépôt et à la diffusion de documents entific research documents, whether they are pub- scientifiques de niveau recherche, publiés ou non, lished or not. The documents may come from émanant des établissements d’enseignement et de teaching and research institutions in France or recherche français ou étrangers, des laboratoires abroad, or from public or private research centers. publics ou privés. Enhancing microservices architectures using data-driven service discovery and QoS guarantees Zeina Houmani∗y, Daniel Balouek-Thomert y, Eddy Caron∗, Manish Parashary ∗Inria Avalon team, LIP Laboratory, UMR CNRS - ENS de Lyon - INRIA - UCB Lyon 5668 University of Lyon, France yRutgers Discovery Informatics Institute Rutgers University, NJ, USA Abstract—Microservices promise the benefits of services with discover the location of a provider for the requested service an efficient granularity using dynamically allocated resources. In using its identifiers. the current evolving architectures, data producers and consumers Additionally, emerging Cloud and Edge computing systems are created as decoupled components that support different data objects and quality of service. -
Spiffe and Spire in Practice
SPIFFE AND SPIRE IN PRACTICE DANIEL FELDMAN UMAIR KHAN 1 RECAP: SPIFFE AND SPIRE 2 SECURE MICROSERVICES COMMUNICATION 3 BUILD AND BRIDGE SERVICE MESH AGENDA 4 AUTHENTICATE SECURELY TO COMMON PLATFORMS 5 AUTHENTICATION FOR ZERO TRUST SECURITY 6 REDUCING THE RISK OF ROGUE CONTAINERS SPIFFE AND SPIRE INTRODUCTION INTRODUCING SPIFFE AND SPIRE Open-source Extensive contributions specification and Integrated into various Part of CNCF by HPE and other top toolchain for service open-source projects tech companies identity 4 CROSS-SERVICE COMMUNICATION IS EXPLODING Increasing attack surface & risk of leakage across untrusted networks API Gateway Long-lived service credentials exist across applications, repositories, platforms, and tools, making them ripe for theft. 5 CROSS-SERVICE COMMUNICATION IS EXPLODING Increasing operational complexity and reducing developer velocity IAM Systems Security Reviews On-board apps Credentials management Security Reviews Learn new APIs Compliance Reporting Integrate with IDPs Developers of Security Apps / Platforms Operation 6 SOLVING THE “BOTTOM TURTLE” Service Certificate Platform Secret Store Platform Identity Initial Configuration? SOURCE: SOLVING THE BOTTOM TURTLE: WWW.SPIFFE.IO/BOOK SOLVING THE “BOTTOM TURTLE” Service Certificate Platform Secret Store Platform Identity Initial Configuration? SOURCE: SOLVING THE BOTTOM TURTLE: WWW.SPIFFE.IO/BOOK SPIFFE KEY CONCEPTS SPIFFE ID SPIFFE VERIFIABLE IDENTITY DOCUMENT Standard format for a service identifier Cryptographically verifiable document spiffe://trustdomain/service -
Get Comments from Pull Requests Github Api
Get Comments From Pull Requests Github Api Filled Griswold perm that Indo-Aryan lust elusively and effect pillion. Is Ximenes enfeebling or psoriatic after accompanied Samuel flurries so reputed? Rice overhanging remotely while exarchal Temp readdresses west or communalize faultily. Other commands that also work in other buffers are available here also. And I want to make sure the commit message conveys the changes. PR with either a thumbs up emoji to acknowledge that you made small changes requested and with another comment if you need to explain something. Ask or answer questions with code on. Press the Save as button on the search results page. Learn to code for free. Github website, specify the key name in the Title field, insert the copied key from Plesk to the Key field and press the Add SSH key button. You will be able to enable additional repositories at a later time. Theoretically you may have to loop forever. However if you do i did you pushed upstream repo, github api supports listing commits! APIs as we will be using Danger which gives us an easy way to interact with this data. First, rewinding head to replay your work on top of it Applying: User B added good stuff. No patch releases will be made, even for critical security issues. Manage and list review requests for a pull request. This might be a very esoteric topic for most people, but since I could not find information about this anywhere, I decided to document this in a post. Contents NAME VERSION METHODS comments commits create files get ismerged list of update AUTHOR COPYRIGHT AND LICENSE. -
Gigaom Radar Report for Evaluating Service Mesh Table of Contents
Image credit: nevarpp Ivan McPhee, Chris Grundemann Aug 20, 2021 GigaOm Radar Report for Evaluating Service Mesh v 1.0 GigaOm Radar Report for Evaluating Service Mesh Table of Contents 1 Summary 2 Market Categories and Deployment Types 3 Key Criteria Comparison 4 GigaOm Radar 5 Vendor Insights 6 Analyst’s Take 7 About Ivan McPhee 8 About Chris Grundemann 9 About GigaOm 10 Copyright GigaOm Radar Report for Evaluating Service Mesh v 1.0 2 This is a GigaOm Research Reprint: Expires Aug 21, 2022 1. Summary Historically, developers independently implemented error handling, observability, and security within each application or microservice to ensure the success of inbound and outbound communication requests. However, as different teams repeated the process and coded similar functionality into each application (often using different programming languages), complexity, fragmentation, and security vulnerabilities were introduced into the environment. A service mesh addresses this problem by “outsourcing” the management of service-to-service communication requests to an out-of-process application. Typically implemented alongside the workload as a “sidecar” proxy, a service mesh simplifies and streamlines runtime operations. Comprising a “data plane” of interconnected network proxies and a “control plane” for configuring the proxies and collecting metrics, it provides a shared infrastructure layer to manage intra-service runtime communications within a distributed, microservice-based software architecture. Application agnostic and fully portable, the service mesh can be adopted by an organization to support any service written in any language or framework. Adding uniform capabilities across the environment, a service mesh provides authentication, authorization, discovery, encryption, load balancing, logging, observability, routing, and tracing.