Spontaneous Neural Oscillations Influence Behavior and Sensory Representations by Suppressing Neuronal Excitability
Total Page:16
File Type:pdf, Size:1020Kb
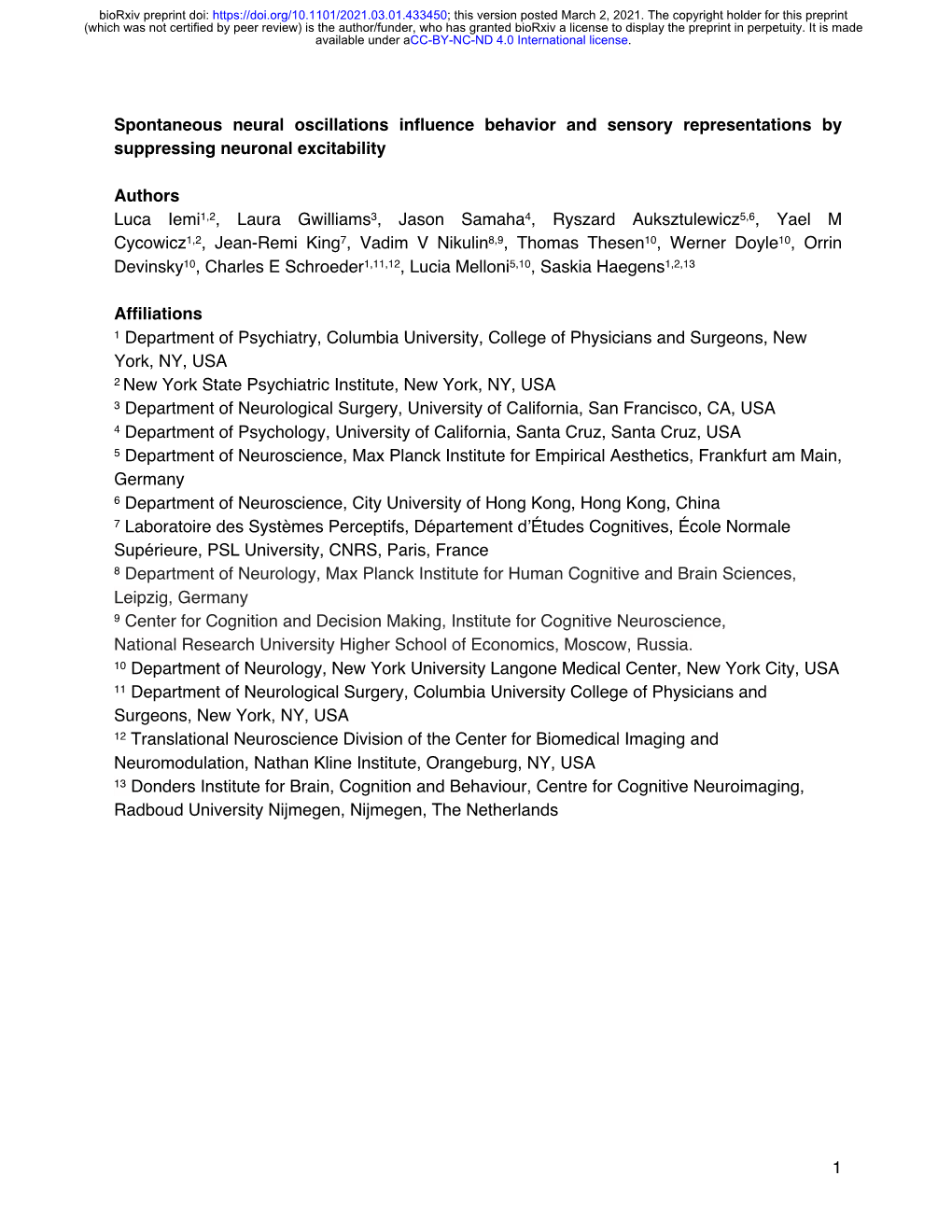
Load more
Recommended publications
-
Part II: Auditory Evoked Potentials, P300 Response and Contingent Negative Variation in Social Drinkers
Article NIMHANS Journal Social Drinking - Part II: Auditory Evoked Potentials, P300 Response and Contingent Negative Variation in Social Drinkers Volume: 14 Issue: 01 January 1996 Page: 23-30 Chitra Andrade &, C R Mukundan Reprints request , - Department of Clinical Psychology, National Institute of Mental Health & Neuro Sciences, Bangalore 560 029, India Abstract The study aimed to determine if any neurophysiological evidence supporting cognitive changes exist in social drinkers. The sample comprised of 44 male social drinkers and 22 teetotallers matched for age and education. Details of drinking habits were elicited through interviews of the subjects. The experiments consisted of tests for late Auditory Evoked Potentials (AEP), Auditory P300 and Contingent Negative Variation (CNV). EEG was recorded from the Fz and Cz electrode sites of the 10-20 International electrode placement system. The two groups were compared on the mean amplitudes and latencies of P1-N1-P2 components of the AEP, the P300 and the early and late components of the CNV. Results showed that the two groups did not differ significantly on both the amplitude and latency measures of the various components, indicating that there is no electrophysiological evidence of change in cognitive processing or brain dysfunction in social drinkers. Key words - Social drinking, Event related potentials, AEP, P 300, CNV Event Related Potential (ERP) responses have been reported to be sensitive to several aspects of alcohol use, including acute intoxication, withdrawal, tolerance, alcohol related chronic brain dysfunction, in utero exposure, social consumptions and familial risk for alcoholism [1], [2], [3], [4], [5]. There have been very few studies in social drinkers (SDs) using ERP measures and the results have been equivocal. -
UC San Diego UC San Diego Electronic Theses and Dissertations
UC San Diego UC San Diego Electronic Theses and Dissertations Title Neurophysiologic correlates to sensory and cognitive processing in altered states of consciousness Permalink https://escholarship.org/uc/item/3gv8j4jk Author Cahn, Baruch Rael Publication Date 2007 Peer reviewed|Thesis/dissertation eScholarship.org Powered by the California Digital Library University of California i UNIVERSITY OF CALIFORNIA, SAN DIEGO Neurophysiologic Correlates to Sensory and Cognitive Processing in Altered States of Consciousness A Dissertation submitted in partial satisfaction of the requirements for the degree Doctor of Philosophy in Neurosciences by Baruch Rael Cahn Committee in charge: Mark Geyer, Chair John Polich, Co-Chair Steve Hillyard Martin Paulus Jaime Pineda Vilayanur Ramachandran Franz Vollenweider 2007 ii Copyright Baruch Rael Cahn, 2007 All Rights Reserved iii The Dissertation of Baruch Rael Cahn is approved, and it is acceptable in quality and form for publication on microfilm: _______________ _______________ _______________ _______________ _______________ _______________ Co-Chair _______________ Chair University of California, San Diego 2007 iii iv DEDICATION I dedicate this thesis in loving memory to my sister Lotus Blossom Cahn who passed away in 2005 from cancer of the thymus. May the love she brought to all those whose lives she touched live on through us and reach out to the world we inhabit. iv v TABLE OF CONTENTS Signature Page ………………………………………………………………..………. iii Dedication ……………….……………………………………………………………. iv Table of Contents ……………………………………………………………..……… -
Spectral Components of the P300 Speller Response in Electrocorticography
Proceedings of the 5th International FrA1.4 IEEE EMBS Conference on Neural Engineering Cancun, Mexico, April 27 - May 1, 2011 Spectral Components of the P300 Speller Response in Electrocorticography Dean J. Krusienski, SeniorMember, IEEE , and Jerry. J. Shih Abstract —Recent studies have demonstrated that the P300 and showed predictable time courses in the delta, theta, and Speller can be used to reliably control a brain-computer alpha bands. With the increased spatial resolution and interface via scalp-recorded electroencephalography (EEG) and spectral bandwidth afforded by ECoG, and the recent intracranial electrocorticography (ECoG). However, the exact association of gamma band features with a wide variety of reasons why some disabled individuals are unable to cortical processes [7][23][32], an examination of the spectral successfully use the P300 Speller remain unknown. The characteristics of the P300 Speller response using ECoG is superior bandwidth and spatial resolution of ECoG compared justified. This paper provides a preliminary spatio-temporal to EEG may provide more insight into the nature of the responses evoked by the P300 Speller. This study examines the analysis of the P300 Speller responses in the conventional spatio-temporal progression of the P300 Speller response in delta, theta, alpha, beta, low-gamma, and high-gamma bands. ECoG for six conventional frequency bands. II. METHODOLOGY I. INTRODUCTION A. Subjects BRAIN -COMPUTER INTERFACE (BCI) is a device that Six subjects with medically intractable epilepsy who Auses brain signals to provide a non-muscular underwent Phase 2 evaluation for epilepsy surgery with communication channel [34], particularly for individuals with temporary placement of intracranial grid or strip electrode severe neuromuscular disabilities. -
A Critical Review of the Deviance Detection Theory of Mismatch Negativity
Review A Critical Review of the Deviance Detection Theory of Mismatch Negativity Jamie A. O’Reilly 1,* and Amonrat O’Reilly 2 1 College of Biomedical Engineering, Rangsit University, Pathumthani 12000, Thailand 2 Thailand Institute for Science and Technological Research, Pathumthani 12120, Thailand; [email protected] * Correspondence: [email protected] Abstract: Mismatch negativity (MMN) is a component of the difference waveform derived from passive auditory oddball stimulation. Since its inception in 1978, this has become one of the most popular event-related potential techniques, with over two-thousand published studies using this method. This is a testament to the ingenuity and commitment of generations of researchers engaging in basic, clinical and animal research. Despite this intensive effort, high-level descriptions of the mechanisms theorized to underpin mismatch negativity have scarcely changed over the past four decades. The prevailing deviance detection theory posits that MMN reflects inattentive detection of difference between repetitive standard and infrequent deviant stimuli due to a mismatch between the unexpected deviant and a memory representation of the standard. Evidence for these mechanisms is inconclusive, and a plausible alternative sensory processing theory considers fundamental principles of sensory neurophysiology to be the primary source of differences between standard and deviant responses evoked during passive oddball stimulation. By frequently being restated without appropri- ate methods to exclude alternatives, the potentially flawed deviance detection theory has remained largely dominant, which could lead some researchers and clinicians to assume its veracity implicitly. Citation: O’Reilly, J.A.; O’Reilly, A. It is important to have a more comprehensive understanding of the source(s) of MMN generation A Critical Review of the Deviance before its widespread application as a clinical biomarker. -
Integration of EEG-FMRI in an Auditory Oddball Paradigm Using Joint Independent Component Analysis Jain Mangalathu Arumana Marquette University
Marquette University e-Publications@Marquette Dissertations (2009 -) Dissertations, Theses, and Professional Projects Integration of EEG-FMRI in an Auditory Oddball Paradigm Using Joint Independent Component Analysis Jain Mangalathu Arumana Marquette University Recommended Citation Mangalathu Arumana, Jain, "Integration of EEG-FMRI in an Auditory Oddball Paradigm Using Joint Independent Component Analysis" (2012). Dissertations (2009 -). Paper 210. http://epublications.marquette.edu/dissertations_mu/210 INTEGRATION OF EEG-FMRI IN AN AUDITORY ODDBALL PARADIGM USING JOINT INDEPENDENT COMPONENT ANALYSIS by Jain Mangalathu Arumana, B.Sc., M.Sc. A dissertation submitted to the Faculty of the Graduate School, Marquette University, in Partial Fulfillment of the Requirements for the Degree of Doctor Philosophy Milwaukee, Wisconsin August 2012 ABSTRACT INTEGRATION OF EEG-FMRI IN AN AUDITORY ODDBALL PARADIGM USING JOINT INDEPENDENT COMPONENT ANALYSIS Jain Mangalathu Arumana, B.Sc., M.Sc. Marquette University, 2012 The integration of event-related potential (ERP) and functional magnetic resonance imaging (fMRI) can contribute to characterizing neural networks with high temporal and spatial resolution. The overall objective of this dissertation is to determine the sensitivity and limitations of joint independent component analysis (jICA) within- subject for integration of ERP and fMRI data collected simultaneously in a parametric auditory oddball paradigm. The main experimental finding in this work is that jICA revealed significantly stronger and more extensive activity in brain regions associated with the auditory P300 ERP than a P300 linear regression analysis, both at the group level and within-subject. The results suggest that, with the incorporation of spatial and temporal information from both imaging modalities, jICA is more sensitive to neural sources commonly observed with ERP and fMRI compared to a linear regression analysis. -
ERP Peaks Review 1 LINKING BRAINWAVES to the BRAIN
ERP Peaks Review 1 LINKING BRAINWAVES TO THE BRAIN: AN ERP PRIMER Alexandra P. Fonaryova Key, Guy O. Dove, and Mandy J. Maguire Psychological and Brain Sciences University of Louisville Louisville, Kentucky Short title: ERPs Peak Review. Key Words: ERP, peak, latency, brain activity source, electrophysiology. Please address all correspondence to: Alexandra P. Fonaryova Key, Ph.D. Department of Psychological and Brain Sciences 317 Life Sciences, University of Louisville Louisville, KY 40292-0001. [email protected] ERP Peaks Review 2 Linking Brainwaves To The Brain: An ERP Primer Alexandra Fonaryova Key, Guy O. Dove, and Mandy J. Maguire Abstract This paper reviews literature on the characteristics and possible interpretations of the event- related potential (ERP) peaks commonly identified in research. The description of each peak includes typical latencies, cortical distributions, and possible brain sources of observed activity as well as the evoking paradigms and underlying psychological processes. The review is intended to serve as a tutorial for general readers interested in neuropsychological research and a references source for researchers using ERP techniques. ERP Peaks Review 3 Linking Brainwaves To The Brain: An ERP Primer Alexandra P. Fonaryova Key, Guy O. Dove, and Mandy J. Maguire Over the latter portion of the past century recordings of brain electrical activity such as the continuous electroencephalogram (EEG) and the stimulus-relevant event-related potentials (ERPs) became frequent tools of choice for investigating the brain’s role in the cognitive processing in different populations. These electrophysiological recording techniques are generally non-invasive, relatively inexpensive, and do not require participants to provide a motor or verbal response. -
Reconfiguration of Brain Network Between Resting-State and Oddball Paradigm
Reconfiguration of Brain Network between Resting-state and Oddball Paradigm Fali Li1, Chanlin Yi1, Yuanyuan Liao1, Yuanling Jiang1, Yajing Si1, Limeng Song1, Tao Zhang1, Dezhong Yao1,2, Yangsong Zhang1,2, 3, Zehong Cao4 and Peng Xu1,2 1The Clinical Hospital of Chengdu Brain Science Institute, MOE Key Lab for NeuroInformation, University of Electronic Science and Technology of China, Chengdu, 611731, China 2School of Life Science and Technology, Center for Information in Medicine, University of Electronic Science and Technology of China, Chengdu, 611731, China 3School of Computer Science and Technology, Southwest University of Science and Technology, Mianyang, 621010, China 4Centre for Artificial Intelligence, Faculty of Engineering and IT, University of Technology Sydney, NSW, Australia Corresponding author: Yangsong Zhang ([email protected]), Peng Xu ([email protected]). This work was supported by the National Key Research and Development Plan of China (#2017YFB1002501), the National Natural Science Foundation of China (#61522105, #61603344, #81401484, and #81330032), the Open Foundation of Henan Key Laboratory of Brain Science and Brain-Computer Interface Technology (No. HNBBL17001), and the Longshan academic talent research supporting program of SWUST (#17LZX692). ABSTRACT The oddball paradigm is widely applied to the investigation of multiple cognitive functions. Prior studies have explored the cortical oscillation and power spectral differing from the resting-state conduction to oddball paradigm, but whether brain networks -
Rapid P300 Brain-Computer Interface Communication with a Head-Mounted Display
ORIGINAL RESEARCH published: 05 June 2015 doi: 10.3389/fnins.2015.00207 Rapid P300 brain-computer interface communication with a head-mounted display Ivo Käthner 1*, Andrea Kübler 1 and Sebastian Halder 1, 2 1 Institute of Psychology, University of Würzburg, Würzburg, Germany, 2 Department of Rehabilitation for Brain Functions, Research Institute of National Rehabilitation Center for Persons with Disabilities, Tokorozawa, Japan Visual ERP (P300) based brain-computer interfaces (BCIs) allow for fast and reliable spelling and are intended as a muscle-independent communication channel for people with severe paralysis. However, they require the presentation of visual stimuli in the field of view of the user. A head-mounted display could allow convenient presentation of visual stimuli in situations, where mounting a conventional monitor might be difficult or not feasible (e.g., at a patient’s bedside). To explore if similar accuracies can be achieved with a virtual reality (VR) headset compared to a conventional flat screen monitor, we conducted an experiment with 18 healthy participants. We also evaluated it with a person in the locked-in state (LIS) to verify that usage of the headset is possible for Edited by: Emanuel Donchin, a severely paralyzed person. Healthy participants performed online spelling with three University of South Florida, USA different display methods. In one condition a 5 × 5 letter matrix was presented on a Reviewed by: conventional 22 inch TFT monitor. Two configurations of the VR headset were tested. In Fabien Lotte, the first (glasses A), the same 5×5 matrix filled the field of view of the user. In the second INRIA (National Institute for Computer Science and Control), France (glasses B), single letters of the matrix filled the field of view of the user. -
Stimulus Frequency and Masking As Determinants of P300 Latency in Event-Related Potentials from Auditory Stimuli *
Biological Psycho&v 21 (1985) 309-318 309 North-Holland STIMULUS FREQUENCY AND MASKING AS DETERMINANTS OF P300 LATENCY IN EVENT-RELATED POTENTIALS FROM AUDITORY STIMULI * John POLICH Department of Neurology, California College of Medicine, University of California, Irvine, CA 92717, U.S.A. Lawrence HOWARD School of Social Sciences, University of California, Irvine, CA 92717, U.S.A Arnold STARR Department of Neurology, California College of Medicine, University of Californra, twine, CA 92717, U.S.A. Accepted for publication 8 May 1985 The effects of target tone frequency, presence of a masking stimulus. and subject sex on the auditory ERP were studied with an ‘oddball’ paradigm. P300 latency became shorter (about 15 msec) as the difference between the standard (1000 Hz) and target tone frequency increased (1500, 2000, 4000 Hz) but became longer (about 10 msec) with the presence of a white noise masking stimulus. Similar results were obtained for both the P3a and P3b subcomponents of the P300 potential. No significant differences between the adult male and female subjects were observed. The role of stimulus parameters in applied testing situations is discussed. 1. Introduction The P300 component of the event-related brain potential (ERP) is a large (5-15 luv), positive-going waveform that occurs with a modal latency of about 300 msec in normal young adults. Although the neurophysiology underlying the P300 is still being explored (Halgren, Squires, Wilson, Rohrbaugh, Bab and Crandall, 1980; Okada, Kaufman and Williamson, 1983), the cognitive events associated with its generation have received considerable attention (Donchin, 1981; Donchin, Ritter and McCallum, 1978; Pritchard, 1981). -
Electrocorticographic (Ecog) Correlates of Human Arm Movements
Exp Brain Res DOI 10.1007/s00221-012-3226-1 RESEARCH ARTICLE Electrocorticographic (ECoG) correlates of human arm movements Nicholas R. Anderson • Tim Blakely • Gerwin Schalk • Eric C. Leuthardt • Daniel W. Moran Received: 23 November 2011 / Accepted: 8 August 2012 Ó Springer-Verlag 2012 Abstract Invasive and non-invasive brain–computer kinematics are encoded. In non-human primates, the rela- interface (BCI) studies have long focused on the motor tionships among these kinematics have been more carefully cortex for kinematic control of artificial devices. Most of documented, and we sought to begin elucidating that these studies have used single-neuron recordings or elec- relationship in humans using ECoG. The largest modula- troencephalography (EEG). Electrocorticography (ECoG) tion in ECoG activity for direction, speed, and velocity is a relatively new recording modality in BCI research that representation was found in the primary motor cortex. We has primarily been built on successes in EEG recordings. also found consistent cosine tuning across both tasks, to We built on prior experiments related to single-neuron hand direction and velocity in the high gamma band recording and quantitatively compare the extent to which (70–160 Hz). Thus, the results of this study clarify the different brain regions reflect kinematic tuning parameters neural substrates involved in encoding aspects of motor of hand speed, direction, and velocity in both a reaching preparation and execution and confirm the important role and tracing task in humans. Hand and arm movement of the motor cortex in BCI applications. experiments using ECoG have shown positive results before, but the tasks were not designed to tease out which Keywords Electrocorticography Á Subdural electroencephalography Á Motor cortex Á Brain–computer interfaces Á Arm tuning Á Cosine tuning N. -
Validating a Novel Oddball Paradigm in Virtual Reality
bioRxiv preprint doi: https://doi.org/10.1101/749192; this version posted March 23, 2020. The copyright holder for this preprint (which was not certified by peer review) is the author/funder. All rights reserved. No reuse allowed without permission. Running head: NOVEL ODDBALL PARADIGM IN VIRTUAL REALITY Real brains in virtual worlds: Validating a novel oddball paradigm in virtual reality Jonathan W. P. Kuziek a, Abdel R. Tayema, Jennifer I. Burrella, Eden X. Redman a, Jeff Murrayc, Jenna Reinenc, Aldis Sipolinsc, Kyle E. Mathewsona,b a Department of Psychology, Faculty of Science, University of Alberta, b Neuroscience and Mental Health Institute, Faculty of Medicine and Dentistry, University of Alberta c IBM Research: Education & Cognitive Sciences Type of article: Full length research paper Number of text pages: 30 (including references) Number of figures: 7 Number of tables: 5 Corresponding Author: Kyle E. Mathewson, Ph.D. Assistant Professor of Cognitive Neuroscience Department of Psychology P-455 Biological Sciences University of Alberta Edmonton, AB, Canada, T6G 2E9 [email protected] bioRxiv preprint doi: https://doi.org/10.1101/749192; this version posted March 23, 2020. The copyright holder for this preprint (which was not certified by peer review) is the author/funder. All rights reserved. No reuse allowed without permission. NOVEL ODDBALL PARADIGM IN VIRTUAL REALITY 1 1 Electroencephalography (EEG) research is typically conducted in controlled laboratory settings. This 2 limits the generalizability to real-world situations. Virtual reality (VR) sits as a transitional tool that 3 provides tight experimental control with more realistic stimuli. To test the validity of using VR for event- 4 related potential (ERP) research we used a well-established paradigm, the oddball task. -
Considerations in Source Estimation of the P3
Source estimation Halgren E. Considerations in Source Estimation of the P3. In: Ikeda I, Inoue Y, eds. Event-related Potentials in Patients with Epilepsy: from Current State to Future Prospects. Paris: John Libbey Eurotext 2008: 71-87. Considerations in Source Estimation of the P3 Eric Halgren, Ph.D. Multimodal Imaging Laboratory Departments of Radiology, Neurosciences and Psychiatry, University of California at San Diego Abstract It is not possible to localize the cerebral structures that generate the P3 from non-invasive recordings. In addition to inherent ambiguities in source localization, many generators that are active during the P3 do not propagate to the scalp and thus would remain invisible to even perfect inverse solutions. Furthermore, scalp recordings are not directly informative with regard to underlying neural processes. In theory, fMRI could help distinguish between alternative P3 generators, but in practice, its utility may be limited. Recordings directly within the brain are limited by incomplete sampling and possible pathology. However, they are the only unambiguous means to demonstrate local generation of potentials which overlap in time with the scalp P3 and possess similar cognitive correlates. Decreases in scalp P3 after brain lesions suggest which structures might contribute directly to the scalp P3, but post-lesion decreases can also be due to disruption of antecedent calculations. Despite these considerations, and remaining dissociations, there is increasing convergence in the distribution of generator structures inferred from different methods. However, bringing the P3s and the cognitive states that they embody into mechanistic neuroscience requires going beyond the localization of generating structures to identifying generating synapses, channels and circuits.