Modes of Policy Learning As Causal Mechanisms: Coming up with a “Policy Learning Measuring Instrument” for Qualitative Research
Total Page:16
File Type:pdf, Size:1020Kb
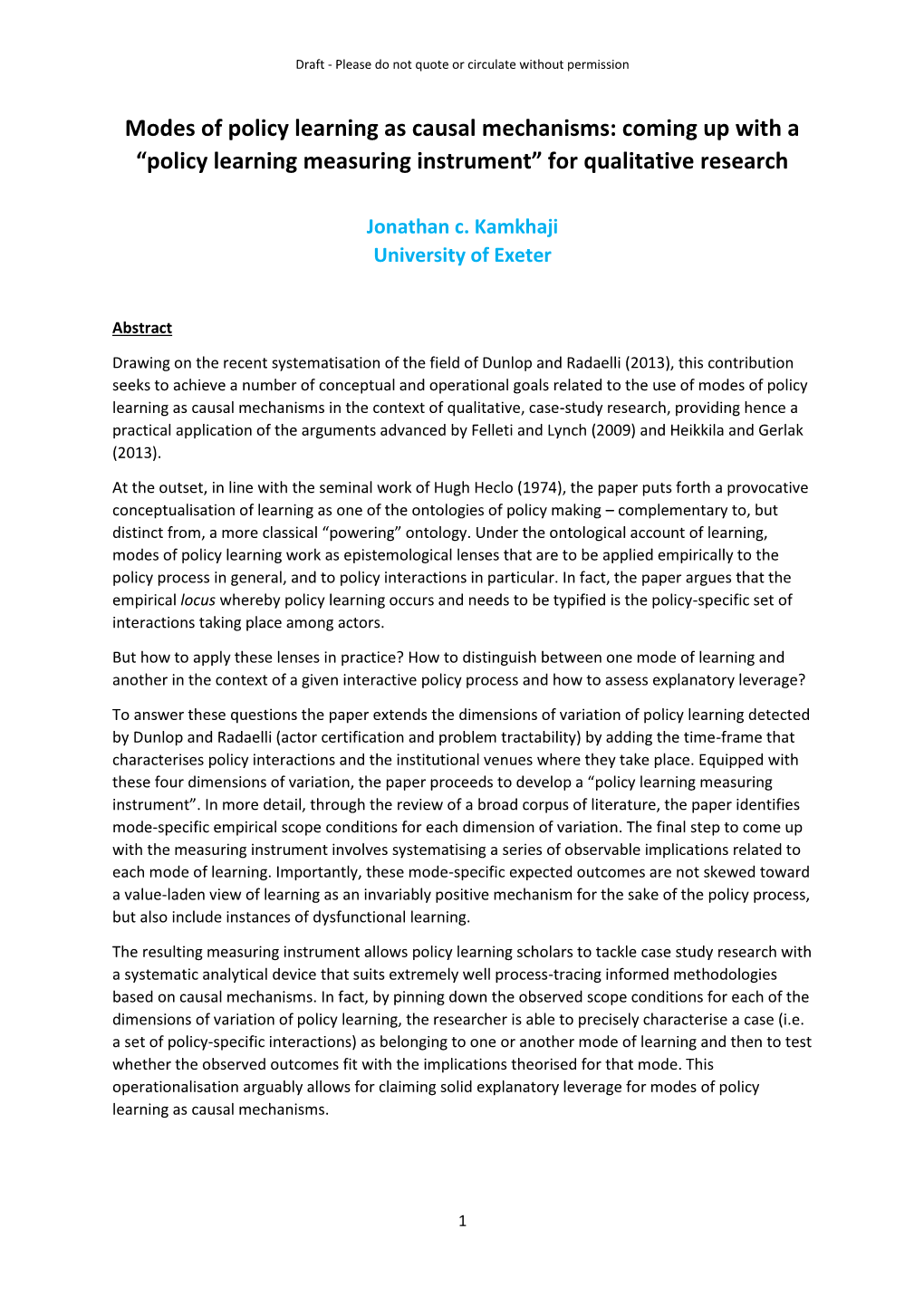
Load more
Recommended publications
-
Chapter 12 Policy Learning and Transfer: Can It Be 'Evidence Based'?
Understanding Public Policy: Theories and Issues 2nd edition Paul Cairney Chapter 12 Policy Learning and Transfer: can it be ‘evidence based’? Key themes of this chapter: Policy learning describes acquiring new policy-relevant knowledge and skills. It sounds like a ‘rational’, ‘evidence based’, and technical process, but it is just as political as other ways to exercise power to address bounded rationality. Policy transfer describes the import and export of policy. It can range from taking broad inspiration to importing a policy programme as a full package. Learning and transfer can be driven by (a) routine searches for evidence, dialogue, consensus-seeking and bargaining, but also (b) coercion, compliance, and a perceived need to keep up with international norms. The potential for ‘evidence-based’ transfer seems limited, so how can we produce more feasible models for learning? Policy learning is a vague but useful term to describe acquiring new knowledge to inform policy and policymaking. Knowledge can be based on information regarding a current policy problem, lessons from the past, or the experience of others. Policy transfer is also a broad term to describe the sharing of policy ideas from one place to another (Dolowitz and Marsh, 1996). Further, transfer is not the only term to describe the exchange of policies and ideas. Lesson-drawing brings together the study of learning from the past as well as other countries (Rose, 1993), policy diffusion describes the spread of solutions among, for example, US states (Walker, 1969), and policy convergence refers to the factors causing similarities in policy across countries (Bennett, 1991a). -
The IMF As a De Facto Institution of the EU: a Multiple Supervisor Approach
The IMF as a de facto institution of the EU: A multiple supervisor approach Dermot Hodson This paper seeks to understand and explain the International Monetary Fund's (IMF) evolving relationship with the European Union (EU) before and after the global financial crisis of 2007–2008. Prior to this crisis, the two sides operated on parallel tracks with little scope for mutual adjustment even during the economic turmoil of the 1970s. After the global financial crisis, the IMF emerged as a de facto institution of the EU thanks to European leaders’ delegation of supervisory powers to both the Fund and the European Commission. The reasons for, and consequences of, this dual delegation are explored here by means of a multiple supervisor variation on the classic principal-agent-supervisor approach. Keywords: European Union, International Monetary Fund, global financial crisis, principal-agent-supervisor I. INTRODUCTION Much has been written about the influence of the European Union (EU) within the International Monetary Fund (IMF). A key question here is whether the euro area's fragmented system of external representation allows its members to speak with one voice within the Fund (McNamara and Meunier, 2002; Cohen, 2003; Bini Smaghi, 2004; Hodson, 2011). Much less has been written about IMF influence within the EU, an issue that is of added significance following the global financial crisis. In October 2008, the IMF and EU agreed to provide loans to Hungary before offering similar terms to two other EU member states: Latvia and Romania. In May 2010, Greece became the first euro area member to receive financial support from the EU and IMF. -
How Nations Learn Praise for the Book
How Nations Learn Praise for the Book ‘The chapters examine how industrial latecomers have crafted strategic and pragmatic policy frameworks to unleash the universal passion for learning into business organ- izational practices that drive production capability development and foster innovation dynamics. The transformational experiences described in the book offer a multitude of ways in which learning is organized and applied to advance a nation’s productive structures and build competitive advantage in the global economy.’ Michael H Best, Professor Emeritus, Author of How Growth Really Happens: The Making of Economic Miracles through Production, Governance and Skills, Winner of the 2018 Schumpeter Prize ‘The analysis of development and catching-up has finally shifted away from sur- real problems of ‘optimal’ market-driven allocation of resources, toward the processes of learning and capability accumulation. This is an important contribution in this perspec- tive: And yet another nail into the coffinofthe“Washington Consensus”.’ Giovanni Dosi, Professor of Economics, Scuola Superiore Sant’ Anna, Pisa, Italy ‘Industrialisation has always been fundamental to sustained economic growth. It separates the world into high and low-income economies. To create inclusive pros- perity, we urgently need to understand How Nations Learn. State-supported innovation is not only cardinal for catch-up, but also to abate climate breakdown (through crowding in new businesses, nurturing experimentation, and ensuring public benefits). By studying the economic history of technological advancement in Africa, Asia, and Latin America, this book makes a powerful case for industrial policy.’ Dr Alice Evans, Lecturer in International Development, King’s College London ‘How Nations Learn is a book based on big ideas. -
The Professional Politics of the Austerity Debate: Comparing the European Central Bank and the International Monetary Fund
CITYPERC Working Paper Series The Professional Politics of the Austerity Debate: Comparing the European Central Bank and the International Monetary Fund Cornel Ban CITYPERC Working Paper No. 2018-01 City Political Economy Research Centre [email protected] / @cityperc City, University of London Northampton Square London EC1V 0HB United Kingdom The Professional Politics of the Austerity Debate: Comparing the European Central Bank and the International Monetary Fund Introduction More than ever before, central banks have become a critical feature of international and domestic public administration. Scholarship on the public administration of central banks is one of the oldest research traditions, with most research focusing on policy outcomes, administrative culture or institutional autonomy (Hawtrey 1925; Day 1961; Young and Ho Park 2013; Zahariadis 2013; Lombardi and Moschella 2016). More recently, however, the focus their economic ideas and discourses has become a focal point in this research (Gabor 2010; Moschella 2011; Johnson 2016; Braun 2016; Matthijs and Blyth 2017). Of particular interest in this regard are the ways in which central bankers act as transnational “issue professionals” asserting scientific authority and building networks of sympathetic interlocutors in order to gain legitimacy, establish cognitive dominance over certain niches (“issue control”) and, consequently, smooth the acts of transnational administration (Seabrooke and Henriksen 2017). This makes them sensitive to what happens in other elite niches of the economics profession, where scientific authority originates. All this begs the question: What professional structures (qualifications, experiences, hierarchies) shape the specific economic ideas with which central bankers derive legitimacy and authority. To find answers to this question, the literature on international financial institutions can be a useful proxy. -
Improving Policy and Provision for Adult Learning in Europe
Education and Training 2020 Improving Policy and Provision for Adult Learning in Europe Acknowledgements The ET2020 Working group on adult learning was established in 2013 as one of six thematic working groups that support Member States in furthering policy development. Its mandate was to support mutual policy learning and develop policy recommendations on: . addressing adult basic skills, . promoting the use of new technologies and Open Educational Resources (OER) in adult learning, and . enhancing the effectiveness, efficiency and coherence of adult learning policies. 33 Member States, other participating countries, social partners and stakeholder groups nominated an adult learning expert to the Group, which started its work in March 2014 and has met in Brussels nine times. Continuous collaboration has been carried out via a web-based communication platform and a number of webinars have been organised between meetings. In addition to this ongoing peer learning, two in-depth country workshops and a seminar have been organised, hosted by Germany, Norway and Belgium. The Group has worked closely with the contractor for the Commission’s study on “Adult learning policies and their effectiveness in Europe” (see Box 16), guiding and commenting on the study, the analytical framework and the prototype web tool that will help countries to self-assess the effectiveness of their adult learning policies. The Group has also followed and contributed to the study on “Adult learners in digital learning environments” (see Box 9.) This report presents the Group's findings and recommendations. The members of the group, nominated by their national authorities, are listed in annex 1. The European Commission acknowledges the contribution of all Working Group members, as well as their external consultants: JD Carpentieri (University College, School of Education, London), Günter Hefler (3s, Vienna) and Jan Hylen (Educationanalytics, Stockholm). -
A Toolbox for EU Regions from Policy Learning to Policy Learning by Doing
From policy learning to policy learning by doing A toolbox for EU regions Our ambition for this Guide: How and why do we have an impact? Following a call for proposals launched by the European Commission, Wallonia has been selected as one of the two European Creative Districts at the end of 2012 with the mission to demonstrate the role of the creative economy and the creative industries in the regeneration of the regional economy. Through Wallonia’s experience gained by implementing the Creative Wallonia programme and the Wallonia European Creative District Project, we have been able to test and analyze the potential of the creative economy and, in a complementary manner, the potential of creative industries to improve the innovation capacity within our regional economy. As a large scale demonstrator, we would like to present the Walloon vision, achievements and project’s developments and to share the up’s and down’s of the process. On the basis of this analysis, we want to assess the reproducibility of our good practices in other territories. This Guide seeks: ¹ To develop a path through which a Creative District can be designed, implemented and assessed at a regional/local level. ² To demonstrate the added value of a Creative District as a component of a regional strategy. ³ To raise awareness about the potential of the creative economy and the creative industries. This Guide builds on the expertise of the Consortium of the Wallonia European Creative District, the High Level Group who advices us on the project’s strategy, external consultants in charge of the assessment of the regional strategy, the European Creative Industry Alliance and many more. -
Comparative Political Economy of Taxation
Philipp Genschel in collaboration with Laura Seelkopf (University Bremen) Florence, 15 March 2015 The comparative political economy of taxation 1. Abstract: Seed money is requested to start a project on the comparative political economy of taxation. The money serves to collect comparative data on the historical development of tax systems worldwide. The focus is on five taxes: Personal income tax, corporate income tax, general sales tax/ VAT, social security contributions, inheritance tax. Information collected includes (a) introduction dates, (b) statutory rates, and (c) revenues. The data collection covers the time period 1850-2010, and aims for the broadest feasible coverage of countries. The data collection will form the basis for a book project on the global diffusion of modern taxation. 2. Objective Taxes are the main source of government revenue. Other revenue sources – foreign conquest, tribute payments, the private wealth of the ruler, public entrepreneurship – have generally lost in importance. Where they have not, as in some developing countries which still rely heavily on foreign aid, import duties or resource rents, this is usually considered as a pathological deviation from the norm of taxation, not as a viable alternative. The modern state is a ‘tax state’ (Schumpeter 1917). The level and structure of tax revenue determines what a government can and cannot do, how many civil servants it can hire, how many services it can deliver, how modestly or ambitiously it can define its goals, and how effectively it can constitute its authority domestically and internationally. The level and structure of taxation also shapes the society the government governs. It determines who has to pay for the state and who not, who gains and who loses, who is empowered and who is disempowered. -
Policy Learning and Policy Change: Learning from Research Citations
Policy Sci DOI 10.1007/s11077-017-9299-8 RESEARCH NOTE Policy learning and policy change: learning from research citations Caner Bakır1 © Springer Science+Business Media, LLC 2017 Abstract Although the seminal article by Bennett and Howlett (Policy Sciences 25: 275– 294, 1992) on policy learning and change has been one of the top five most cited articles in Policy Sciences, no attempt has yet been made to provide a citation analysis showing how its impact has evolved over time. This paper reports the findings of a study that provides a systematic analysis of the citing articles published in academic journals during the period 1992–2017. Keywords Bennett · Howlett · Policy learning · Policy change · Citation analysis · Review Introduction In 2018, Policy Sciences celebrates its 50th anniversary and recognises the five most cited articles from the last five decades. Colin J. Bennett and Michael Howlett’s 1992 article, ‘The lessons of learning: reconciling theories of policy learning and policy change’, is one of these articles. This paper reflects on how the article has been used in learning literature. Bennett and Howlett (1992) offer a review of the literature on policy change based on different conceptualisations of learning. Although the then existing conventional expla- nations of policy change explained state actions and policy change as a product of social pressures and conflicts (i.e. ‘conflict-based explanations’), they recognise the emerging importance of the role of ideas and learning in explaining policy change (i.e. ‘learning- based approaches’). They are also concerned about conceptual ambiguity, under-theorised and fragmented learning literature in the learning subjects, objects and outcomes that constrain the understanding and appreciation of cumulative knowledge and empirical evidence with lack of comparisons and integration in the literature. -
Globalisation, Policy Transfer, and Global Governance: an Assessment in Developing Countries
Globalisation, Policy Transfer, and Global Governance: an Assessment in Developing Countries Laila Kholid Alfirdaus Abstrak Dari perspektif ekonomi politik, globalisasi identik dengan liberalisasi, sedangkan dari perspektif kebijakan publik, globalisasi identik dengan policy transfer. Ekonomi politik menekankan bahwa globalisasi merupakan sistem yang menyediakan ruang bagi pembukaan interelasi dan interaksi ekonomi antar negara, baik dalam bentuk perdagangan bebas, mobilitas aktivitas produksi, dan pertukaran teknologi. Sementara itu, perspektif kebijakan publik mengartikankan globalisasi sebagai ruang yang lebih luas untuk pertukaran pengetahuan yang berguna bagi pembangunan dan pengembangan kebijakan dalam konteks yang disebut policy transfer. Meskipun kedua perspektif seolah-olah memberikan penekanan yang berbeda, pada dasarnya kedua penekanan itu sama-sama mensyaratkan adanya entitas bernama global governance. Bahkan, terkadang entitas ini berperan menentukan dalam keputusan di sebuah negara, mendorong perdebatan pro dan kontra. Pengalaman Indonesia di akhir 1990an, misalnya, menunjukkan kepada kita betapa lembaga global governance berperan dominatif dalam reformasi ekonomi dan politik, memunculkan pertanyaan tentang kedaulatan negara. Paper ini menganalisis keterkaitan globalisasi dan policy transfer serta mendiskusikan peran lembaga-lembaga global governance di dalamnya. Keywords: globalisasi, public policy, policy transfer A. PENDAHULUAN Theories of development may assert that globalisation on the one hand creates the super prosperous countries, those that dominate capital, market and economic benefit, commonly noted as the First countries. On the other hand, globalisation yields the extra poor countries, those that are marginalised and exploited by the first countries, coined as the Third countries. The existance of Trans National Companies and Multi National Companies in the developing countries is often deemed as the symbol of the Fisrt countries‟ dominance and exploitation in the Third ones as an impact of globalisation. -
Impact Assessment in the European Union Claire A. Dunlop
Policy Instruments, Policy Learning and Politics: Impact Assessment in the European Union Claire A. Dunlop and Claudio M. Radaelli 2018 To appear in G. Capano, M. Howlett, M. Ramesh and A. Virani (Eds.) Making Policies Work: First and Second Order Mechanisms in Policy Design. Cheltenham: Edward Elgar Research funded by the ERC Project Protego, Procedural Tools for Effective Governance Abstract One proposition we put forward in this volume is that there is a relationship between policy instruments and mechanisms. To find out how exactly this relationship works, its activators and the causal role of policy instruments, we zoom-in on the case of impact assessment (IA) in the European Union. IA is an evidence-based instrument adopted by the EU in the context of the evidence-based better regulation strategy. The connection between IA and learning is apparently intuitive: IA should bring evidence to bear on the process of selecting policy options, and therefore assist decision-makers in learning from different type of analysis, dialogue with experts and stakeholders, and open consultation. However, we find out that learning comes in different modes (epistemic, reflexive, bargaining and hierarchical) and that the activators, context and results of learning vary across modes. In the conclusions, we reflect on the connections between learning and politics revealed by our approach to policy instruments and varieties of learning. Keywords Causal mechanisms, policy design, policy learning, policy instruments, impact assessment, regulation 1 Introduction What causal mechanisms generate learning and what is their relationship with policy instruments? It is almost obvious to observe that the type and success of policy learning is related to and mediated by the policy process. -
Industrial Policy and Learning Lessons from Latin America
OUP CORRECTED PROOF – FINAL, 29/5/2019, SPi 10 Industrial Policy and Learning Lessons from Latin America Wilson Peres and Annalisa Primi 10.1 Introduction In the last two decades, the world global economic outlook has profoundly changed. The rise of China, the shifts in the global geopolitical equilibria and the digital revolution are configuring a fast-changing economic landscape in which windows of opportunities open and close at high speed. The South East Asia and the Pacific region dominates the headlines due to its growing global relevance. Africa gathers world attention as the continent where most of global population growth is expected to happen and where local leaderships are standing up with a new, shared vision for the future embedded in the Africa Union Commission (AUC) Agenda 2063: the “Africa We Want”, and as a continent for business and investment from traditional and emerging partners. In this context, Latin America appears as a region in slow motion. Despite progress made in poverty reduction, somewhat better income distri- bution, increasing access to education and health, advances in digital connectivity, and an emerging regional start-up scene, still the countries in the region remain among the most unequal in the world, and the region seems trapped in some of the challenges of the past (Prebisch, 1949). How to go beyond being a provider of raw materials and commodities to the global economy? How to benefit more from trade and FDI and ensure that the outcomes of increased participation in the world economy accrue to the domestic stakeholders? This chapter contributes to the understanding of how nations learn by looking at the experience of Latin America. -
Locality, Learning and Reciprocity in Place-Based Practice’
‘Delivering public services: locality, learning and reciprocity in place-based practice’. Ian Marsh, Kate Crowley, Dennis Grube and Richard Eccleston Submitted to the Australian Journal of Public Administration May 2016 Re-submitted August 2016. Abstract Policymakers across myriad jurisdictions are grappling with the challenge of complex policy problems. Multi-faceted, complex, and seemingly intractable, ‘wicked’ problems have exhausted the repertoire of the standard policy approaches. In response, governments are increasingly looking for new options, and one approach that has gained significant scholarly interest, along with increasing attention from practitioners, is ‘place- based’ solutions. This paper surveys conceptual aspects of this approach. It describes practices in comparable jurisdictions – the UK, the EU and the US. And it explores efforts over the past decade to ‘localise’ Indigenous services. It sketches the governance challenge in migrating from top-down or principal-agent arrangements towards place-based practice. The paper concludes that many of the building blocks for this shift already exist but that these need to be re-oriented around ‘learning’. Funding and other administrative protocols may also ultimately need to be redefined. Summary at a glance This paper considers the international application of place-based approaches in order to assess their potential to meet policy challenges in Australian settings. It examines how such approaches have been conceptualised in other jurisdictions, and the challenge in migrating