Deploying Inter-Specific Recombinant Inbred Lines to Map Qtls for Yield-Related Traits in Soybean
Total Page:16
File Type:pdf, Size:1020Kb
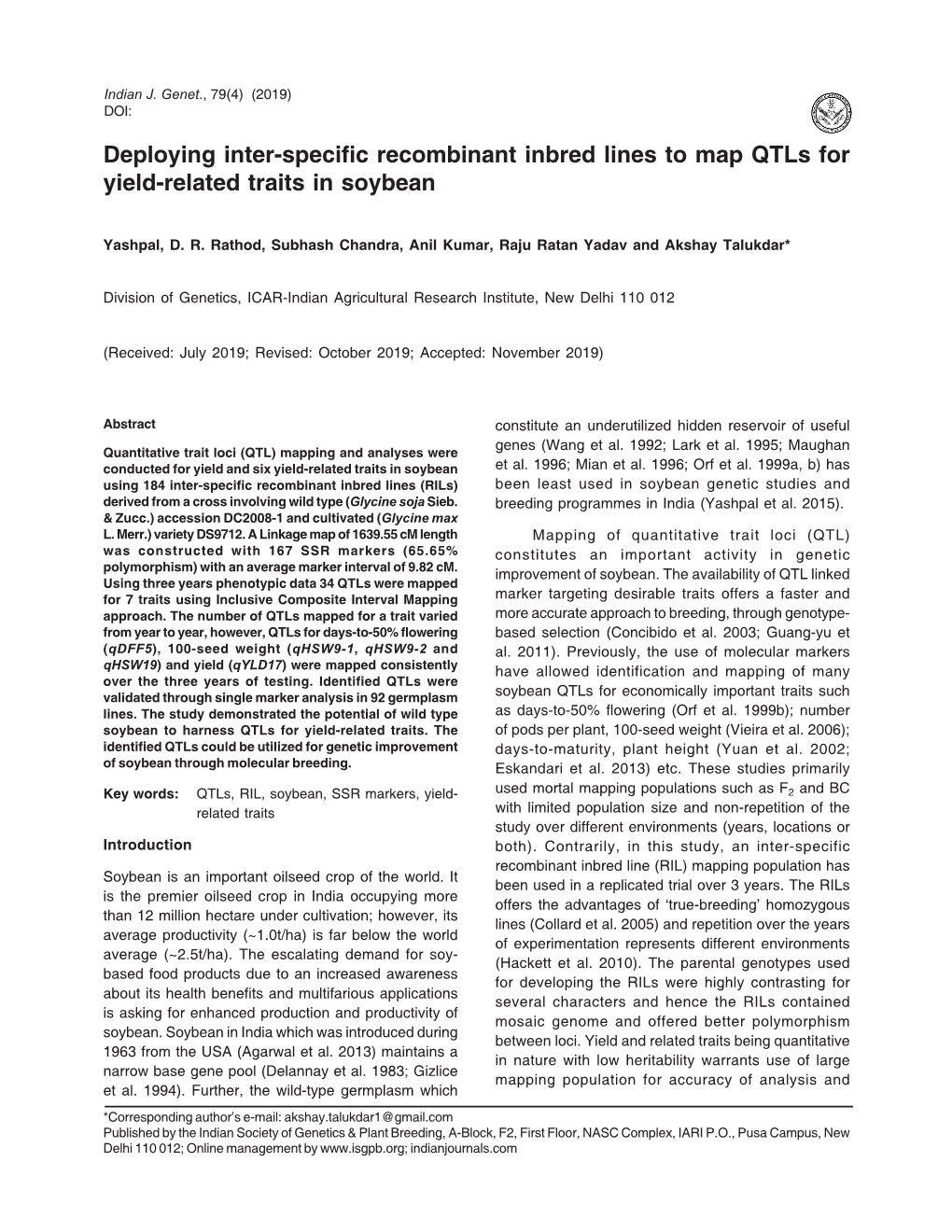
Load more
Recommended publications
-
Genetics of Physiological Traits Associated with Drought Tolerance in Soybean (Glycine Max)
University of Arkansas, Fayetteville ScholarWorks@UARK Theses and Dissertations 7-2020 Genetics of Physiological Traits Associated with Drought Tolerance in Soybean (Glycine max) Sumandeep Kaur Bazzer University of Arkansas, Fayetteville Follow this and additional works at: https://scholarworks.uark.edu/etd Part of the Agronomy and Crop Sciences Commons, Cellular and Molecular Physiology Commons, and the Plant Breeding and Genetics Commons Citation Bazzer, S. K. (2020). Genetics of Physiological Traits Associated with Drought Tolerance in Soybean (Glycine max). Theses and Dissertations Retrieved from https://scholarworks.uark.edu/etd/3819 This Dissertation is brought to you for free and open access by ScholarWorks@UARK. It has been accepted for inclusion in Theses and Dissertations by an authorized administrator of ScholarWorks@UARK. For more information, please contact [email protected]. Genetics of Physiological Traits Associated with Drought Tolerance in Soybean (Glycine max) A dissertation submitted in partial fulfillment of the requirements for the degree of Doctor of Philosophy in Crop, Soil, and Environmental Sciences by Sumandeep Kaur Bazzer Punjab Agricultural University Bachelor of Science in Biotechnology, 2013 Punjab Agricultural University Master of Science in Plant Breeding and Genetics, 2015 July 2020 University of Arkansas This dissertation is approved for recommendation to the Graduate Council. __________________________ Larry C. Purcell, Ph.D. Dissertation Director __________________________ __________________________ Jeffery D. Ray, Ph.D. Richard E. Mason, Ph.D. Committee Member Committee Member __________________________ __________________________ Mary C. Savin, Ph.D. Ken Korth, Ph.D. Committee Member Committee Member Abstract Soybean (Glycine max L.) is one of the major row crops in the United States, and its production is often limited by drought stress. -
Project Summary
Project Summary PROJECT SUMMARY Instructions: The summary is limited to 250 words. The names and affiliated organizations of all Project Directors/Principal Investigators (PD/PI) should be listed in additions to the title of the project. The summary should be a self-contained, specific description of the activity to be undertaken and should focus on: overall project goals(s) and supporting objectives; plans to accomplish project goal(s); and relevance of the project to the goals of the program. The importance of a concise, informative Project summary cannot be overemphasized. Title: Common Bean Coordinated Agricultural Project PD: McClean, Phillip E. Institution: North Dakota State University CO-PD: Garden-Robinson, Julie Institution: North Dakota State University CO-PD: Johnson, Christina Institution: North Dakota State University CO-PD: Osorno, Juan Institution: North Dakota State University CO-PD: Slator, Brian Institution: North Dakota State University CO-PD: Kelly, Jim Institution: Michigan State University CO-PD: Brick, Mark Institution: Colorado State University CO-PD: Ryan, Elizabeth Institution: Colorado State University CO-PD: Thompson, Henry Institution: Colorado State University CO-PD: Myers, Jim Institution: Oregon State University CO-PD: Gepts, Paul Institution: University of California, Davis CO-PD: Urrea, Carlos Institution: University of Nebraska, Lincoln CO-PD: Grusak, Michael A. Institution: USDA Children’s Nutrition Research Center, Houston, TX CO-PD: Cregan, Perry Institution: USDA Soybean Genomics and Improvement -
In Silico Characterization of 1,2-Diacylglycerol Cholinephosphotransferase and Lysophospha- Tidylcholine Acyltransferase Genes in Glycine Max L
In silico characterization of 1,2-diacylglycerol cholinephosphotransferase and lysophospha- tidylcholine acyltransferase genes in Glycine max L. Merrill C.S. Sousa1,2, B.A. Barros3, D. Barh4, P. Ghosh5, V. Azevedo2, E.G. Barros6,7 and M.A. Moreira1 1Departamento de Bioquímica e Biologia Molecular - BIOAGRO, Universidade Federal de Viçosa, Viçosa, MG, Brasil 2Laboratório de Genética Celular e Molecular, Instituto de Ciências Biológicas, Universidade Federal de Minas Gerais, Belo Horizonte, MG, Brasil 3Empresa Brasileira de Pesquisa Agropecuária, Sete Lagoas, MG, Brasil 4Institute of Integrative Omics and Applied Biotechnology, Medinipur, WB, India 5Department of Computer Science, Virginia Commonwealth University, Richmond, VA, USA 6Departamento de Biologia Geral, Universidade Federal de Viçosa, Viçosa, MG, Brasil 7Universidade Católica de Brasília, Brasília, DF, Brasil Corresponding author: C.S. Sousa E-mail: [email protected] Genet. Mol. Res. 15 (3): gmr.15038974 Received July 14, 2016 Accepted August 9, 2016 Published August 26, 2016 DOI http://dx.doi.org/10.4238/gmr.15038974 Copyright © 2016 The Authors. This is an open-access article distributed under the terms of the Creative Commons Attribution ShareAlike (CC BY-SA) 4.0 License. ABSTRACT. The enzymes 1,2-diacylglycerol cholinephosphotrans- ferase (CPT) and lysophosphatidylcholine acyltransferase (LPCAT) are important in lipid metabolism in soybean seeds. Thus, understand- Genetics and Molecular Research 15 (3): gmr.15038974 C.S. Sousa et al. 2 ing the genes that encode these enzymes may enable their modification and aid the improvement of soybean oil quality. In soybean, the genes encoding these enzymes have not been completely described; there- fore, this study aimed to identify, characterize, and analyze the in silico expression of these genes in soybean. -
Combining QTL-Seq and Linkage Mapping to Fine Map a Wild
Zhang et al. BMC Genomics (2018) 19:226 https://doi.org/10.1186/s12864-018-4582-4 RESEARCH ARTICLE Open Access Combining QTL-seq and linkage mapping to fine map a wild soybean allele characteristic of greater plant height Xiaoli Zhang, Wubin Wang, Na Guo, Youyi Zhang, Yuanpeng Bu, Jinming Zhao* and Han Xing* Abstract Background: Plant height (PH) is an important agronomic trait and is closely related to yield in soybean [Glycine max (L.) Merr.]. Previous studies have identified many QTLs for PH. Due to the complex genetic background of PH in soybean, there are few reports on its fine mapping. Results: In this study, we used a mapping population derived from a cross between a chromosome segment substitution line CSSL3228 (donor N24852 (G. Soja), a receptor NN1138–2(G. max)) and NN1138–2 to fine map a wild soybean allele of greater PH by QTL-seq and linkage mapping. We identifiedaQTLforPHina1.73Mbregiononsoybeanchromosome13 through QTL-seq, which was confirmed by SSR marker-based classical QTL mapping in the mapping population. The linkage analysis showed that the QTLs of PH were located between the SSR markers BARCSOYSSR_13_1417 and BARCSOYSSR_13_1421 on chromosome 13, and the physical distance was 69.3 kb. RT-PCR and sequence analysis of possible candidate genes showed that Glyma.13 g249400 revealed significantly higher expression in higher PH genotypes, and the gene existed 6 differences in the amino acids encoding between the two parents. Conclusions: Data presented here provide support for Glyma.13 g249400 as a possible candidate genes for higher PH in wild soybean line N24852. -
Identification of SSR Markers Linked to Partial Resistance to Soybean Rust in Brazil from Crosses Using the Resistant Genotype IAC 100
Identification of SSR markers linked to partial resistance to soybean rust in Brazil from crosses using the resistant genotype IAC 100 F.C. Juliatti1, K.R.C. Santos1, T.P. Morais1,2 and B.C.M. Juliatti3 1 Laboratório de Micologia e Proteção de Plantas, Instituto de Ciências Agrárias, Universidade Federal de Uberlândia, Uberlândia, MG, Brasil 2 Instituto Federal de Educação, Ciência e Tecnologia, IFSULDEMINAS, Machado, MG, Brasil 3Juliagro Bioprodutos, Genética e Proteção de Plantas, Uberlândia, MG,Brasil Corresponding author: F.C. Juliatti E-mail: [email protected] Genet. Mol. Res. 18 (3): gmr18249 Received January 29, 2018 Accepted July 01, 2019 Published August 31, 2019 DOI http://dx.doi.org/10.4238/gmr18249 ABSTRACT. Soybean rust is considered a highly aggressive disease in soybean crops. Most research has focused on obtaining resistant genotypes based on dominant or recessive alleles, which provide vertical resistance. The identification of promising crosses that may be used to develop genotypes with horizontal resistance from IAC 100 may help to increase the longevity of the recommended cultivars. However, this type of resistance is limited by environmental variables that may hinder selection. We ranked crosses based on their response to soybean rust using genetic estimates and predicted gains. It was also an objective to identify quantitative trait loci (QTLs) associated with resistance to soybean rust in two generations derived from the same cross. Eighty- seven F4 progenies from IAC 100 (partial resistance) x BRS Caiapônia (susceptible) cross were field phenotyped. The data divided the DNA samples into two groups for bulked segregant analysis, which was carried out using simple sequence repeat (SSR) primers. -
WO 2012/092461 A2 5 July 20 12 (05.07.2012) W P O P C T
(12) INTERNATIONAL APPLICATION PUBLISHED UNDER THE PATENT COOPERATION TREATY (PCT) (19) World Intellectual Property Organization International Bureau (10) International Publication Number (43) International Publication Date WO 2012/092461 A2 5 July 20 12 (05.07.2012) W P O P C T (51) International Patent Classification: NW 62nd Avenue, Johnston, Iowa 5013 1-0552 (US). C12Q 1/68 (2006.01) MENDEZ, Edwin, J. [US/US]; c/o Pioneer Hi-Bred Inter national, Inc., 7250 NW 62nd Avenue, Johnston, Iowa (21) International Application Number: 5013 1-0552 (US). RYAN-MAHMUTAGIC, Molly PCT/US201 1/067791 [US/US]; c/o Pioneer Hi-Bred International, Inc., 7250 (22) International Filing Date: NW 62nd Avenue, Johnston, Iowa 5013 1-0552 (US). 29 December 201 1 (29. 12.201 1) SANTIAGO-PARTON, Sally, A. [US/US]; c/o Pioneer Hi-Bred International, Inc., 7250 NW 62nd Avenue, John (25) Filing Language: English ston, Iowa 5013 1-0552 (US). SHENDELMAN, Joshua, (26) Publication Language: Ra English M. [US/US]; c/o Pioneer Hi-Bred International, Inc., 7250 NW 62nd Avenue, Johnston, Iowa 5013 1-0552 (US). (30) Priority Data: WOODWARD, John, B. [US/US]; c/o Pioneer Hi-Bred 61/428,306 30 December 2010 (30. 12.2010) US International, Inc., 7250 NW 62nd Avenue, Johnston, Iowa (71) Applicant (for all designated States except US) : PION¬ 5013 1-0552 (US). XIONG, Yanwen [CN/US]; c/o Pion EER HI-BRED INTERNATIONAL, INC. [US/US]; eer Hi-Bred International, Inc., 7250 NW 62nd Avenue, 7100 N.W. 62nd Avenue, Johnston, Iowa 5013 1-1014 Johnston, Iowa 5013 1-0552 (US). -
Phenotypic Evaluation and Genetic Dissection of Resistance To
View metadata, citation and similar papers at core.ac.uk brought to you by CORE provided by Springer - Publisher Connector Huang et al. BMC Genetics (2016) 17:85 DOI 10.1186/s12863-016-0383-4 RESEARCH ARTICLE Open Access Phenotypic evaluation and genetic dissection of resistance to Phytophthora sojae in the Chinese soybean mini core collection Jing Huang1, Na Guo1, Yinghui Li2, Jutao Sun1, Guanjun Hu1, Haipeng Zhang1, Yanfei Li2, Xing Zhang1, Jinming Zhao1, Han Xing1* and Lijuan Qiu2* Abstract Background: Phytophthora root and stem rot (PRR) caused by Phytophthora sojae is one of the most serious diseases affecting soybean (Glycine max (L.) Merr.) production all over the world. The most economical and environmentally-friendly way to control the disease is the exploration and utilization of resistant varieties. Results: We screened a soybean mini core collection composed of 224 germplasm accessions for resistance against eleven P. sojae isolates. Soybean accessions from the Southern and Huanghuai regions, especially the Hubei, Jiangsu, Sichuan and Fujian provinces, had the most varied and broadest spectrum of resistance. Based on gene postulation, Rps1b, Rps1c, Rps4, Rps7 and novel resistance genes were identified in resistant accessions. Consequently, association mapping of resistance to each isolate was performed with 1,645 single nucleotide polymorphism (SNP) markers. A total of 14 marker-trait associations for Phytophthora resistance were identified. Among them, four were located in known PRR resistance loci intervals, five were located in other disease resistance quantitative trait locus (QTL) regions, and five associations unmasked novel loci for PRR resistance. In addition, we also identified candidate genes related to resistance. -
Genome-Wide Dissection and Expression Profiling of Unique Glyoxalase III Genes in Soybean Reveal the Differential Pattern Of
www.nature.com/scientificreports OPEN Genome-wide dissection and expression profling of unique glyoxalase III genes in soybean Received: 29 August 2017 Accepted: 2 March 2018 reveal the diferential pattern of Published: xx xx xxxx transcriptional regulation Tahmina Islam1 & Ajit Ghosh 2,3 Reactive carbonyl species, such as methylglyoxal and glyoxal are very toxic in nature and can inactivate various cellular macromolecules such as DNA, RNA, and protein by forming advanced glycation end products. Conventional glyoxalase pathway with two enzymes- glyoxalase I and glyoxalase II, detoxify MG into D-lactate with the help of reduced glutathione. However, DJ-1/PfpI domain(s) containing DJ-1/ Hsp31 proteins do the same in a single step, and thus termed as “glyoxalase III”. A comprehensive genome-wide analysis of soybean identifed eleven putative glyoxalase III proteins with DJ-1/ PfpI domain encoded by seven genes. Most of these proteins are predicted to be mitochondria and chloroplast localized. In spite of similar function, a diferential evolution pattern was observed between Hsp31 and DJ-1 proteins. Expression of GmDJ-1A, GmDJ-1B, and GmDJ-1D2 transcripts was found to be constitutive in diferent tissues and developmental stages. Transcript profling revealed the strong substrate-specifc upregulation of GmDJ-1 genes in response to exogenous methylglyoxal exposure. Out of seven genes, GmDJ-1D1 and GmDJ-1D2 showed maximum upregulation against salinity, dehydration, and oxidative stresses. Moreover, GmDJ-1D2 showed functional glyoxalase III enzyme activity by utilizing MG as a substrate. Overall, this study identifes some novel tissue-specifc and abiotic stress-responsive GmDJ-1 genes that could be investigated further. -
Development of High-Resolution Simple Sequence Repeat Markers Through Expression Profiling of Genes Associated with Pod Maturity of Soybean
applied sciences Article Development of High-Resolution Simple Sequence Repeat Markers through Expression Profiling of Genes Associated with Pod Maturity of Soybean , Myoung Ryoul Park * y , Inhye Lee y , Min-Jung Seo and Hong-Tae Yun Central Area Crop Breeding Div., National Institute of Crop Science, Suwon, Gyeonggi-do 16429, Korea; [email protected] (I.L.); [email protected] (M.-J.S.); [email protected] (H.-T.Y.) * Correspondence: [email protected]; Tel.: +82-31-695-4051; Fax: +82-31-695-4029 These authors contributed equally to this study. y Received: 25 July 2020; Accepted: 11 September 2020; Published: 12 September 2020 Featured Application: Use of high-resolution molecular markers for studying genetic diversity of soybean pods. Abstract: In soybeans (Glycine max L.), the time required to attain maturity is a quantitative trait controlled by multiple genes and quantitative trait loci (QTL), which enable soybean cultivars to adapt to various regions with diverse day lengths. In this study, depending on the days to maturity, 100 soybean varieties were classified into eight maturity groups numbered from 0 to VII. The maturity groups were further sorted into three maturity ecotypes: early, middle, and late maturity. The analysis of 55,589 soybean genes revealed a total of 1147 related to the growth and development of soybean pods, including 211 genes with simple sequence repeats (SSRs). We further identified 42 SSR markers that amplified over two alleles in three different ecotypes, including six genes that were up- or downregulated in pods of more than one ecotype. The agglomerative hierarchical tree constructed for the newly identified SSR markers had three clusters. -
Modification of Fatty Acid Composition in Soybean Seeds to Improve
MODIFICATION OF FATTY ACID COMPOSITION IN SOYBEAN SEEDS TO IMPROVE SOYBEAN OIL QUALITY AND FUNCTIONALITY A Dissertation Presented to The Faculty of the Graduate School At the University of Missouri In Partial Fulfillment Of the Requirements for the Degree DOCTOR OF PHILOSOPHY By Anh TUNG PHAM Dr. Kristin Bilyeu, Dissertation Supervisor July 2011 The undersigned, appointed by the dean of the Graduate School, have examined the Dissertation entitled MODIFICATION OF FATTY ACID COMPOSITION IN SOYBEAN SEEDS TO IMPROVE SOYBEAN OIL QUALITY AND FUNCTIONALITY Presented by Anh Tung Pham A candidate for the degree of DOCTOR OF PHILOSOPHY OF PLANT BIOLOGY AND GENETICS And hereby certify that, in their opinion, it is worthy of acceptance. Dr. Kristin D. Bilyeu Dr. David A. Sleper Dr. J. Grover Shannon Dr. Emmanual Liscum ACKNOWLEDGEMENTS I would like to express my gratitude to Dr. Kristin Bilyeu not only for giving me the opportunity to join her research group and conduct exciting research in plant biology and genetics, but also for her continuous advising, support, and encouragement during my doctoral studies. The quality of this study was greatly influenced by her help in avoiding adversities and in solving problems which arose during the course of the research project, and also by her research ethics and her perseverance for a deeper understanding of this project. I would also like to thank Dr. Grover Shannon for his collaboration and contribution to my dissertation projects. I would like to thank Dr. David Sleper for your guidance and support. I would like to thank Dr. David Sleper, Dr. Grover Shannon, Dr. -
Soybean NAC Gene Family: Sequence Analysis and Expression Under Low Nitrogen Supply
DOI: 10.1007/s10535-016-0693-4 BIOLOGIA PLANTARUM 61 (3): 473-482, 2017 Soybean NAC gene family: sequence analysis and expression under low nitrogen supply X. WANG1,2*, D. LI1,2, J. JIANG1,2, Z. DONG1, and Y. MA1 Department of Agronomy Sciences, Anhui Agricultural University, Hefei, 230036, P.R. China1 Key Laboratory of Wheat Biology and Genetic Improvement on South Yellow & Huai River Valley, Ministry of Agriculture, Hefei, 230036, P.R. China2 Abstract NAM, ATAF1/2, and CUC2 (NAC) proteins are plant-specific transcription factors playing essential roles in plant development and various abiotic stress responses. In the present study, we identified 173 full-length NAC genes in soybean, which were phylogenetically clustered into 15 groups (NACa - NACo). The soybean NAC genes (GmNACs) were non-randomly located across the 20 chromosomes, and 128 genes (86.5 %) were preferentially located in duplicated regions of chromosome arms, which implied long segmental duplication and contributed to evolution of the GmNAC gene family. Most GmNACs genes showed a distinct tissue-specific expression pattern and the redundant expression patterns of active duplicate genes suggested that GmNACs have been retained by substantial subfunctionalization during soybean evolution. Furthermore, active GmNACs genes that had undergone strong artificial selection during soybean domestication were identified based on selection analysis. After low nitrogen treatment, enhanced expression of some selected GmNAC genes were noticed in soybean shoot and root, which implied that GmNACs might play an important role in nitrogen metabolism. Here, we summarize the sequence and expression analysis of the NAC gene family in the soybean. Additional key words: chromosome location, evolution, Glycine max, nitrogen metabolism, transcription factors. -
Copyright 2016 Noor Syahira Nasarudin
Copyright 2016 Noor Syahira Nasarudin GENOME-WIDE ASSOCIATION MAPPING OF TEMPERATURE RESPONSE IN AGRONOMICALLY IMPORTANT TRAITS OF SOYBEAN BY NOOR SYAHIRA NASARUDIN THESIS Submitted in partial fulfillment of the requirements for the degree of Master of Science in Crop Sciences in the Graduate College of the University of Illinois at Urbana-Champaign, 2016 Urbana, Illinois Master’s Committee: Assistant Professor Yoshie Hanzawa, Chair Assistant Professor Alexander E. Lipka Professor Randall L. Nelson ABSTRACT Seed emergence and plant growth are important agronomic traits affected by environmental fluctuations. A better understanding of the genetic basis of temperature response in important traits of soybean [Glycine max (L.) Merr.] may contribute to improving seed quality and plant development under temperature stress. Genome-wide association study (GWAS) using high- density and high-quality single nucleotide polymorphisms (SNPs) is a powerful tool to associate markers and important traits. The aims of this study are: 1) to characterize the effects of low and high ambient temperature in soybean seed emergence and plant growth; and 2) to identify the genetic factors regulating soybean response to ambient temperature in seed emergence and growth- related traits. A collection of 440 diverse soybean genotypes and SoySNP50K data were used for GWAS. Seeds were germinated and plants were grown under constant 20°C (low) and 30°C (high) temperature in the Plant Care Facility at University of Illinois, Urbana-Champaign. Seed emergence and plant growth data were analyzed using a best linear unbiased prediction (BLUP) model and subjected to GWAS using 36,499 SNPs. For seed emergence in association panel 1 & 2, only 1 SNP on chromosome 9 was significantly associated with relative mean difference for days to initial emergence.