Abstract Assessing Spatial and Temporal Patterns Of
Total Page:16
File Type:pdf, Size:1020Kb
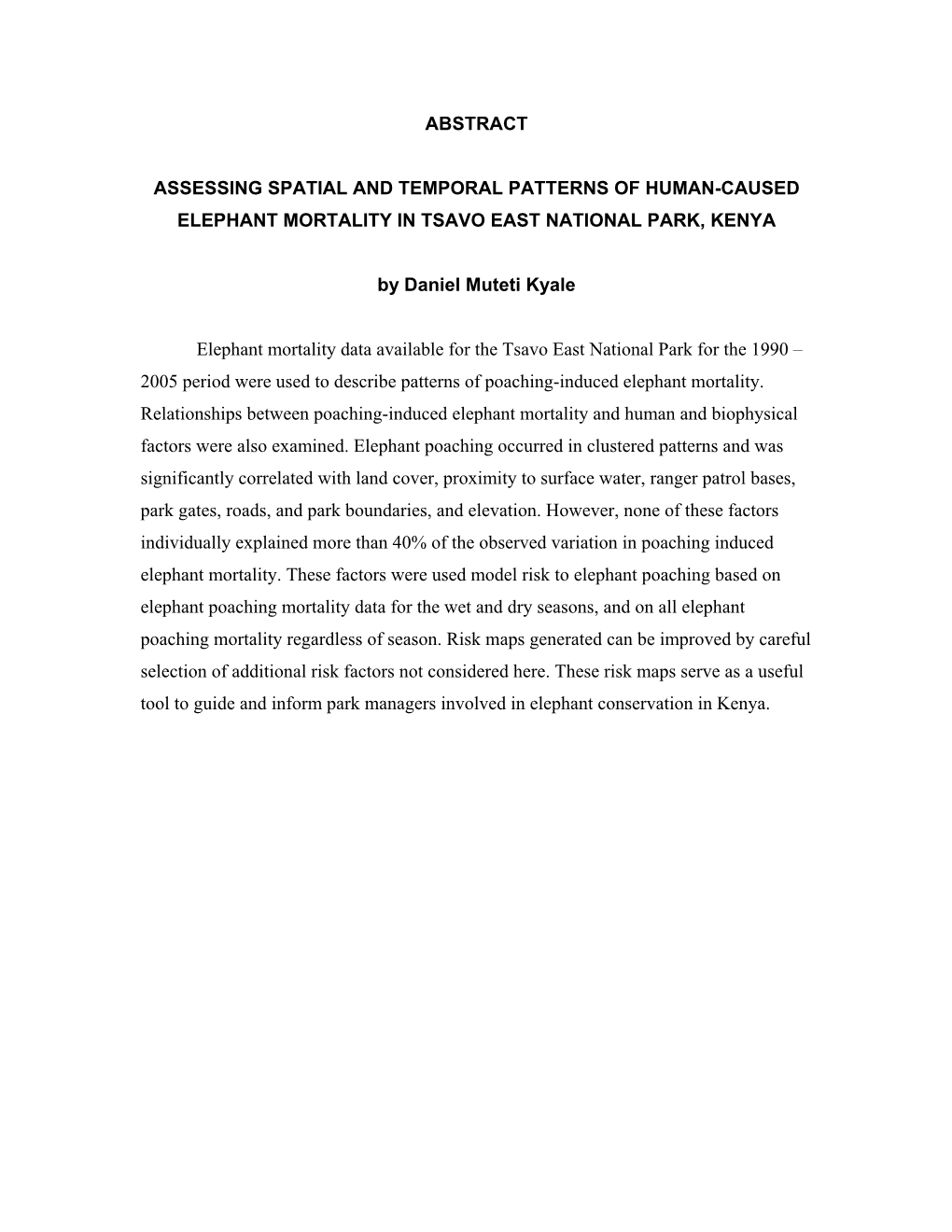
Load more
Recommended publications
-
2014 State of Ivory Demand in China
2012–2014 ABOUT WILDAID WildAid’s mission is to end the illegal wildlife trade in our lifetimes by reducing demand through public awareness campaigns and providing comprehensive marine protection. The illegal wildlife trade is estimated to be worth over $10 billion (USD) per year and has drastically reduced many wildlife populations around the world. Just like the drug trade, law and enforcement efforts have not been able to resolve the problem. Every year, hundreds of millions of dollars are spent protecting animals in the wild, yet virtually nothing is spent on stemming the demand for wildlife parts and products. WildAid is the only organization focused on reducing the demand for these products, with the strong and simple message: when the buying stops, the killing can too. Via public service announcements and short form documentary pieces, WildAid partners with Save the Elephants, African Wildlife Foundation, Virgin Unite, and The Yao Ming Foundation to educate consumers and reduce the demand for ivory products worldwide. Through our highly leveraged pro-bono media distribution outlets, our message reaches hundreds of millions of people each year in China alone. www.wildaid.org CONTACT INFORMATION WILDAID 744 Montgomery St #300 San Francisco, CA 94111 Tel: 415.834.3174 Christina Vallianos [email protected] Special thanks to the following supporters & partners PARTNERS who have made this work possible: Beijing Horizonkey Information & Consulting Co., Ltd. Save the Elephants African Wildlife Foundation Virgin Unite Yao Ming Foundation -
Destruction of Confiscated Elephant Ivory in Times Square: Questions and Answers
U.S. Fish & Wildlife Service Destruction of Confiscated Elephant Ivory in Times Square: Questions and Answers Why is the United States destroying ivory than we needed for these purposes elephant ivory? and decided to destroy that stockpile as We want to send a clear message that a demonstration of our commitment to the United States will not tolerate combating wildlife trafficking. ivory trafficking and is committed to protecting elephants from extinction. The Have other countries destroyed ivory toll these crimes are taking on elephant stockpiles? populations, particularly in Africa, is Yes. The following lists the governments at its worst in decades. The United and the year they destroyed ivory: States believes that it is important Kenya, 1989, 1991, 2011, 2015; Zambia, to destroy ivory seized as a result of 1992; United Arab Emirates, 1992, 2015; law enforcement investigations and at Gabon, 2012; Philippines, 2013; China, international ports of entry. Destroying 2014, 2015; Chad, 2014; France, 2014; this ivory tells criminals who engage in Belgium 2014; Hong Kong 2014; Ethiopia poaching and trafficking that the United 2015; and Congo, 2015. States will take all available measures to disrupt and prosecute those who prey Why doesn’t the Service sell the ivory? on, and profit from, the deaths of these The Service does not sell confiscated magnificent animals. wildlife or products derived from endangered and threatened species. Has the U.S. Government ever done this Illegal ivory trade is driving a dramatic before? increase in African elephant poaching, Yes. On November 14, 2013, at the U.S. Photo: Samples of seized ivory. -
Tsavo: Small Steps, Big Impacts ©IFAW/D
Tsavo: Small Steps, Big Impacts July 2007 - June 2008 Tsavo: Small Steps, Big Impacts Tsavo: A Front cover: Elephants ©IFAW/D. Willetts Community ©IFAW/E. Indakwa A desert rose in full bloom in Tsavo IFAW/D. Willetts IFAW/D. © IFAW/D. Willetts IFAW/D. © Great white egrets at Lake Jipe in Tsavo West IFAW/D. Willetts IFAW/D. © Tsavo: Small Steps, Big Impacts Tsavo: Eland strut the Tsavo landscape 1 IFAW/D. Willetts IFAW/D. Message from James Isiche © Investing in a Worthy Cause The third financial year for the Tsavo Conservation Area Project (TCAP) commenced on an exciting note for IFAW and Kenya Wildlife Service (KWS). Set objectives were on course; Kenya’s economy was booming; tourist numbers and park revenues were at an all-time high; and KWS seemed poised to achieve financial self- sustenance by 2013. Unfortunately, two unrelated events patrols and maintenance of fire breaks in pummeled the financial fortunes of the fire-prone sections of the park were done. Tsavo Parks and left the country’s wildlife – elephants in particular – in great peril. These huge challenges cannot, however, obscure the tremendous gains made during The decision by CITES in 2007 to allow this period. Our support for innovative four Southern African states to offload their community conservation projects aimed ivory stockpiles to Japan and China placed at reducing human-wildlife conflicts and a threat on elephants in other countries uplifting livelihoods will certainly enhance within the continent. Then, an eruption of community support for conservation. violence after Kenya’s disputed presidential Most heartening, also, is the unwavering election at the close of 2007 followed. -
The Status of Kenya's Elephants
The status of Kenya’s elephants 1990–2002 C. Thouless, J. King, P. Omondi, P. Kahumbu, I. Douglas-Hamilton The status of Kenya’s elephants 1990–2002 © 2008 Save the Elephants Save the Elephants PO Box 54667 – 00200 Nairobi, Kenya first published 2008 edited by Helen van Houten and Dali Mwagore maps by Clair Geddes Mathews and Philip Miyare layout by Support to Development Communication CONTENTS Acknowledgements iv Abbreviations iv Executive summary v Map of Kenya viii 1. Introduction 1 2. Survey techniques 4 3. Data collection for this report 7 4. Tsavo 10 5. Amboseli 17 6. Mara 22 7. Laikipia–Samburu 28 8. Meru 36 9. Mwea 41 10. Mt Kenya (including Imenti Forest) 42 11. Aberdares 47 12. Mau 51 13. Mt Elgon 52 14. Marsabit 54 15. Nasolot–South Turkana–Rimoi–Kamnarok 58 16. Shimba Hills 62 17. Kilifi District (including Arabuko-Sokoke) 67 18. Northern (Wajir, Moyale, Mandera) 70 19. Eastern (Lamu, Garissa, Tana River) 72 20. North-western (around Lokichokio) 74 Bibliography 75 Annexes 83 The status of Kenya’s elephants 1990–2002 AcKnowledgemenTs This report is the product of collaboration between Save the Elephants and Kenya Wildlife Service. We are grateful to the directors of KWS in 2002, Nehemiah Rotich and Joseph Kioko, and the deputy director of security at that time, Abdul Bashir, for their support. Many people have contributed to this report and we are extremely grateful to them for their input. In particular we would like to thank KWS field personnel, too numerous to mention by name, who facilitated our access to field records and provided vital information and insight into the status of elephants in their respective areas. -
Quarterlyspring 2000 Volume 49 Number 2
AWI QuarterlySpring 2000 Volume 49 Number 2 ABOUT THE COVER For 25 years, the tiger (Panthera tigris) has been on Appendix I of the Conven- tion on International Trade in Endangered Species of Wild Fauna and Flora (CITES), but an illegal trade in tiger skins and bones (which are used in traditional Chinese medicines) persists. Roughly 5,000 to 7,000 tigers have survived to the new millennium. Without heightened vigilance to stop habitat destruction, poaching and illegal commercialization of tiger parts in consuming countries across the globe, the tiger may be lost forever. Tiger Photos: Robin Hamilton/EIA AWI QuarterlySpring 2000 Volume 49 Number 2 CITES 2000 The Future of Wildlife In a New Millennium The Eleventh Meeting of the Conference of the Parties (COP 11) to the Convention on International Trade in Endangered Species of Wild Fauna and Flora (CITES) will take place in Nairobi, Kenya from April 10 – 20, 2000. Delegates from 150 nations will convene to decide the fate of myriad species across the globe, from American spotted turtles to Zimbabwean elephants. They will also examine ways in which the Treaty can best prevent overexploitation due to international trade by discussing issues such as the trade in bears, bushmeat, rhinos, seahorses and tigers. Adam M. Roberts and Ben White will represent the Animal Welfare Institute at the meeting and will work on a variety of issues of importance to the Institute and its members. Pages 8–13 of this issue of the AWI Quarterly, written by Adam M. Roberts (unless noted otherwise), outline our perspectives on a few of the vital issues for consideration at the CITES meeting. -
An Illusion of Complicity: Terrorism and the Illegal Ivory Trade in East Africa Occasional Paper
Over 180 years of independent defence and security thinking The Royal United Services Institute is the UK’s leading independent think-tank on international defence and security. Its mission is to be an analytical, research-led global Royal United Services Institute forum for informing, influencing and enhancing public debate on a safer and more stable for Defence and Security Studies world. Since its foundation in 1831, RUSI has relied on its members to support its activities, sustaining its political independence for over 180 years. Occasional Paper London | Brussels | Nairobi | Doha | Tokyo | Washington, DC An Illusion of Complicity Terrorism and the Illegal Ivory Trade in East Africa Tom Maguire and Cathy Haenlein An Illusion of Complicity: Terrorism and the Illegal Ivory Trade in East Africa Occasional Paper Royal United Services Institute for Defence and Security Studies Whitehall London SW1A 2ET United Kingdom +44 (0)20 7747 2600 www.rusi.org RUSI is a registered charity (No. 210639) An Illusion of Complicity Terrorism and the Illegal Ivory Trade in East Africa Tom Maguire and Cathy Haenlein Occasional Paper, September 2015 Royal United Services Institute for Defence and Security Studies Over 180 years of independent defence and security thinking The Royal United Services Institute is the UK’s leading independent think-tank on international defence and security. Its mission is to be an analytical, research-led global forum for informing, influencing and enhancing public debate on a safer and more stable world. Since its foundation in 1831, RUSI has relied on its members to support its activities, sustaining its political independence for over 180 years. -
Preliminary Indications of the Effect of Infrastructure Development on Ecosystem Connectivity in Tsavo National Parks, Kenya
FIELD NOTES Preliminary indications of the effect of infrastructure development on ecosystem connectivity in Tsavo National Parks, Kenya Benson Okita-Ouma1,*, Fredrick Lala2,4,*, Richard Moller3, Michael Koskei1, Sospeter Kiambi4, David Dabellen1, Chris Leadismo1, Domnic Mijele4, Jeremiah Poghon2,4, Lucy King1, Frank Pope1, George Wittemyer1,5, Jake Wall1,6, Suzannah Goss7, Robert Obrien2, 4 and Iain Douglas-Hamilton1,8. 1Save The Elephants, PO Box 54667 - 00100, Nairobi, Kenya. 2Tsavo East National Park, PO Box 14 - 80300, Voi Kenya 3Tsavo Trust PO Box 204 - 90128, Mtito Andei, Kenya 4Kenya Wildlife Service, PO Box 40241 - 00100, Nairobi, Kenya. 5Department of Fish, Wildlife, and Conservation Biology Colorado State University 6Lab for Advanced Spatial Analysis, Geography, University of British Columbia, Vancouver, Canada 7National Environmental Management Authority (Lead expert), PO Box 24126, Nairobi, Kenya 8Department of Zoology, Oxford University, United Kingdom *corresponding author: [email protected] and [email protected] Balancing conservation and species. Here we report on a research project that aims infrastructure development to contribute to optimizing the design and manage the existing wildlife crossing structures by monitoring Conserving land and ecosystem connectivity for the movement of elephants in a conservation area of wildlife is increasingly a global challenge as demand global significance that is affected by major–ongoing for infrastructure development to meet growing human and planned–rail and road construction projects. population needs encroaches in many traditional wildlife areas. The survival of wildlife species in arid and semi-arid systems requires interconnected The infrastructure landscapes, and limiting animal movement greatly reduces the system’s ability to sustain viable In 2014 the Kenyan government initiated construction wildlife populations (Vasudev et al. -
De-Snaring Report May 2013 Ziwani/Simba Team
THE DAVID SHELDRICK WILDLIFE TRUST PO BOX 15555 Mbagathi 00503 Nairobi Kenya Tel: 020 230 1396 Email: [email protected] www.sheldrickwildlifetrust.org DE-SNARING REPORT MAY 2013 ZIWANI/SIMBA TEAM Ziwani/Simba Team De-Snaring Report for May, 2013 Participants Peterson Ngari Marayion Lelekoiten Ali Diba Noah Lesimirdana Stephen Musau Areas Patrolled Mbulia Daka Dima Irima Kangenchwa Kishushe Ranch Kudu Lion Hill Lumo Community Wild Aruba Manyani Ziwani Murka Mzima Springs Ndii Salt Lick Satao Voi Wanjala Wundanyi Maktau Arrests 3 Arrest(s) this month 18 June 2013 Page 1 of 6 Ziwani/Simba Team De-Snaring Report for May, 2013 frmQuarterlyPatrolReport_3A 18 June 2013 Page 2 of 6 Ziwani/Simba Team De-Snaring Report for May, 2013 Snares Collected Snare Type Snare Size Snare Description No. Confiscated Thin Wire Small Line 26 Thin Wire Small Cluster 6 Thin Wire Medium Cluster 66 Cable Large Line 2 Total Snares Collected: 100 18 June 2013 Page 3 of 6 Ziwani/Simba Team De-Snaring Report for May, 2013 Monthly Summary Report summary The team began its operation in Tsavo East National Park in the first 15 days of the month before it shifted to Tsavo West National park. In this regard the team managed to patrol Aruba, Ndara, Ndii, Irima, Lion hill park boundary, Maktau hills, Ziwani, Kishushe, Saltlick, Wanjala, Kangeshwa, Lumo community ranch and along Mzima water pipeline. Following the worrying trend of killing of our God given wild animals, Simba team decided to sweep out all wildlife threat related activity whatsoever .In Tsavo east National park at Irima and Lion hill, the team managed to lift 8 medium snares which targeted Impalas and other antelopes. -
Opportunities
WILDI N V E S T M E N T OPPORTUNITIES SAFARI LODGES AND ADVENTURE PROSPECTUS INVEST IN KENYA SAFARI LODGES PROSPECTUS INVESTMENT OPPORTUNITIES FOR DEVELOPMENT AND MANAGEMENT OF SAFARI LODGES & FACILITIES IN KENYA’S NATIONAL PARKS 2018 CONTENTS 2 3 PROPOSED TOURISM DEVELOPMENT SITES 34 36 38 40 42 Sibiloi NP Malka Mari NP 4 4 #019 Central Turkana Island NP Mandera Marsabit South Island NP 5#0 Marsabit NR 2 South 2 Turkana NR Wajir West Pokot Losai NR Samburu Mt. Elgon NP Elgeyo #08 Trans Marakwet Nzoia Isiolo Bungoma Uasin Baringo Shaba NR Gishu Busia 15#0 L.Bogoria NR Laikipia 12 Kakamega #0 Nandi Meru #011 ¯ Vihiga 2 Meru NP 0 Siaya #0 0 Nyandarua 18 Kisumu Mt. Kenya NP Ndere Island#0 Tharaka-Nithi Kora NP Aberdare 7 Mt. Kenya NR Kericho Nakuru NP #0 Homa Bay Nyeri Garissa Ruma #0 3 Embu NP #0 6 Kisii Bomet Murang'a Migori Kiambu Arawale Narok Nairobi NP #09 Machakos NR Masai Kitui Mara NR 10 Tana River Boni NR South Tana River Kitui NR Primate NR Dodori NR 2 2 - Lamu - Kajiado Makueni 21 16 #0 Chyulu #01 #0 Hills NP Tsavo Amboseli NP Code Site Name National Park East NP 1 Kithasyu Gate Chyulu Hills NP 14 2 Sirimon Glade Mt. Kenya NP #0 #017 3 Game Farm KWSTI 13 #0 Kilifi 4 4 Malindi Cafeteria Malindi Marine NP #0 Malindi Tsavo Marine NP 5 Sokorta Diko Marsabit NP West NP 6 Nyati Campsite Ruma NP Taita Taveta 7 Tusk Camp Aberdares NP #020 8 Kasawai Gate Mt. -
The Ivory Trade: the Single Greatest Threat to Wild Elephants
THE IVORY TRADE: THE SINGLE GREATEST THREAT TO WILD ELEPHANTS Elephants have survived in the wild for 15 million years, but today this iconic species is threatened with extinction due to ongoing poaching for ivory. As long as there is demand for ivory, elephants will continue to be killed for their tusks. According to best estimates, as many as 26,000 elephants are killed every year simply to extract their tusks. A Brief History of the Ivory Trade Demand for ivory first skyrocketed in the 1970s and 1980s. The trade in ivory was legal then -- although for the most part it was taken illegally. During those decades, approximately 100,000 elephants per year were being killed. The toll on African elephant populations was shocking: over the course of a single decade, their numbers dropped by half. In October of 1989 the UN Convention on International Trade in Endangered Species (CITES) voted to move African elephants from Appendix II to Appendix I, affording the now highly endangered animal the maximum level of protection, effectively ending trade in all elephant parts, including ivory. With the new Appendix 1 listing, the poaching of elephants literally stopped overnight. The ban brought massive awareness of the plight of elephants, the bottom dropped out of the market, and prices plummeted. Ivory was practically unsellable. For 10 years, the global ban stood strong and it seemed the crisis had ended. Elephant numbers began to recover. Unfortunately, the resurgence in elephant populations, while nowhere near the numbers prior to the spike in trade, was nevertheless a catalyst for some African countries to consider reopening the trade. -
KENYA SAFARI ITINERARY Elephant Monitoring TSAVO EAST NATIONAL PARK JUNE OR NOVEMBER 2017 OPTION 03
KENYA SAFARI ITINERARY Elephant Monitoring TSAVO EAST NATIONAL PARK JUNE OR NOVEMBER 2017 OPTION 03 'Tel: +254 20 2666313/11, + 254 734 600673 | * E-mail: [email protected] www.maniagosafaris.com SAFARI DETAILS DAY 01 : ARRIVE IN NAIROBI, KENYA Arrive at Jomo Kenyatta International Airport, met by your tour Director Ms Pat Awori inside the airport and she will assist you through the Immigration and Customs formalities, then proceed to your waiting safari vehicle and be and transferred to House of Waine House of Waine is nestled in the leafy residential area of Karen and set in beautiful tranquil gardens. The House has the charm of a family home and accommodation is in spacious, individually styled rooms with mini bar, telephone, TV and tea and coffee-making facilities. Meals are served in various areas of the house and there are two bars exclusively for use of guests. There is also a heated swimming pool and a conference room. Dinner will be at House of waine. Overnight at House of Waine (Meal: Dinner) DAY 02 : NAIROBI After breakfast, depart for a full day excursion in Karen area. Begin by a visit to the Giraffe Centre which offers the unique experience of hand-feeding these gentle giants at eye level from a raised platform, while watching the warthogs below snuffle around looking for titbits. The centre is the headquarters of the African Fund for Endangered Wildlife (AFEW) which was setup to raise money to relocate the endangered Rothschild giraffe to safe areas 2 Lunch today will be at the Tamambo Karen Blixen Coffee Garden, which is located in a beautiful tranquil garden setting and offers a wide variety of dishes including seafood, steak and poultry. -
Doc. 6.21 CONVENTION on INTERNATIONAL TRADE IN
Doc. 6.21 CONVENTION ON INTERNATIONAL TRADE IN ENDANGERED SPECIES OF WILD FAUNA AND FLORA Sixth Meeting of the Conference of the Parties Ottawa (Canada), 12 to 24 July 1987 Interpretation and Implementation of the Convention Trade in Ivory from African Elephants SECRETARIAT REPORT ON OPERATION OF THE QUOTA SYSTEM 1. At its fifth meeting (Buenos Aires, 1985), the Conference of the Parties adopted Resolution Conf. 5.12, "Trade in Ivory from African Elephants", establishing new procedures for the control of international trade in ivory from African elephants. These procedures are collectively referred to as the "ivory export quota system", and the key element is the opportunity for establishment of an annual ivory export quota by each state having a population of African elephants and wishing to export raw ivory. 2. Non-Party producer states may also submit an export quota, and any non-Party wishing to import, export or re-export raw ivory must meet all requirements of the Resolution. Unless a non-Party has informed to the contrary, it is assumed to be not conforming with the requirements. 3. The Secretariat was directed to co-ordinate the implementation of the system including maintaining a central database, receiving annual quotas from producer countries and circulating them, preparing a manual of procedures for implementing the system and providing advice on the conservation status of African elephants. An implementation manual, "Ivory Trade Control Procedures", was written by the Secretariat and distributed to all Party and non-Party countries in November 1985. The report, "Establishment of African Ivory Export Quotas and Associated Control Procedures" prepared by Rowan B.