Insights from a New High-Resolution Drought Atlas for the Caribbean Spanning 1950–2016
Total Page:16
File Type:pdf, Size:1020Kb
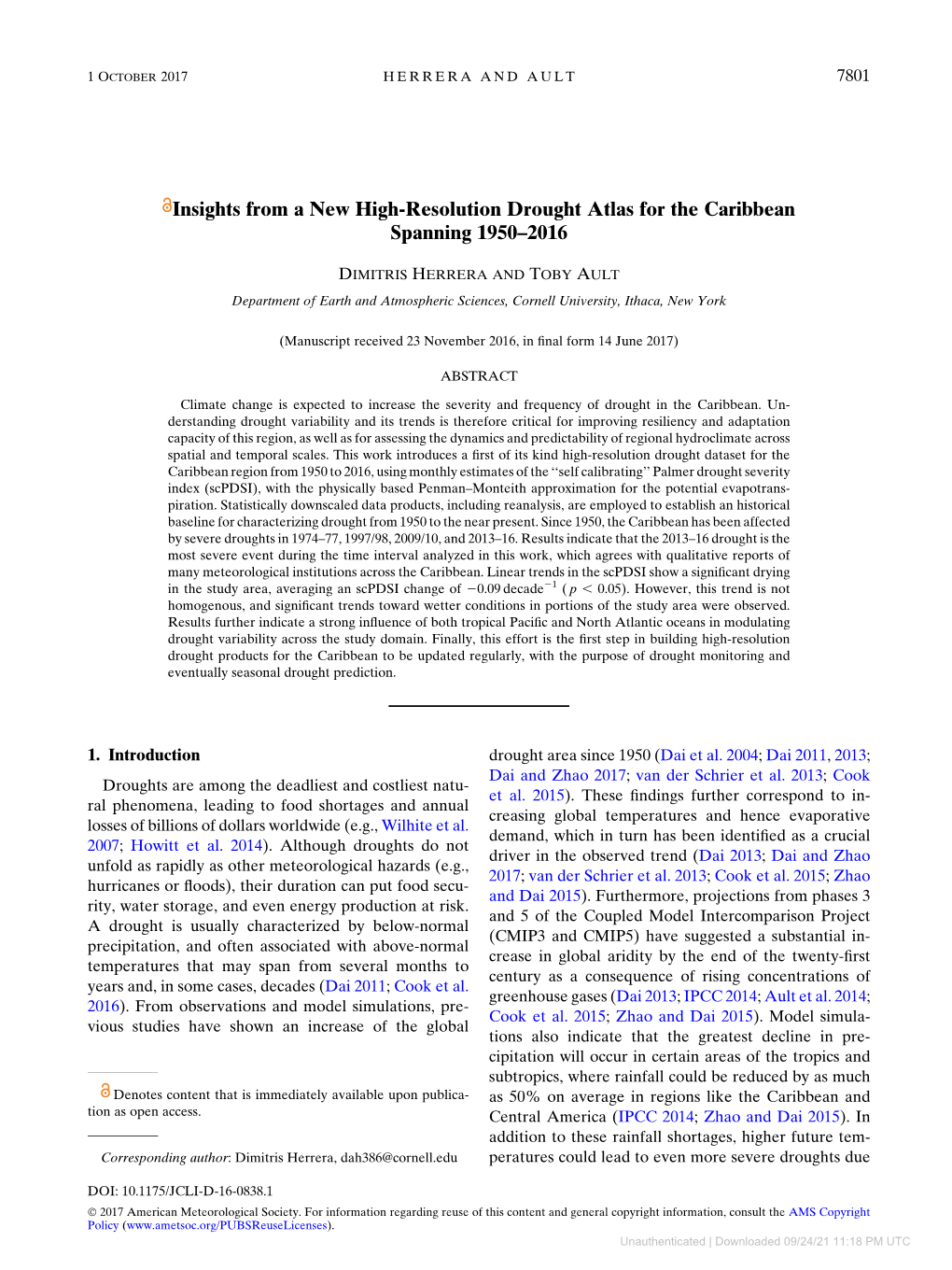
Load more
Recommended publications
-
Disaster Risk Programme to Strengthen Resilience in the Dry Corridor in Central America 1 DISASTER RISK in the DRY CORRIDOR
Disaster Risk Programme to strengthen resilience in the Dry Corridor in Central America El Salvador-Guatemala Honduras-Nicaragua 2015-2018 ©FAO/Orlando Sierra ©FAO/Orlando CONTEXT Central America is one of the regions most vulnerable to disaster risks due to its geographical location, high climate variability, exposure to extreme hazards and the institutional and socio-economic weaknesses of its population. Agriculture and food and nutrition security face multiple threats that can cause damage and disrupt regular weather conditions. One of the areas most affected by extreme It is estimated that in Central America’s Dry hazards, in particular natural hazards, is the Corridor more than 1 million families rely on Dry Corridor. The term Dry Corridor, although subsistence farming. The levels of poverty and referred to a climatic phenomenon, has malnutrition are alarming and mainly affect an ecological basis and defines a group of rural populations and indigenous communities. ecosystems in the eco-region of dry tropical Most livelihoods depend on climate whereas the forests in Central America covering the lowlands percentage of producers of basic grains varies of the Pacific coastal area, and most of central from 54 percent in El Salvador and Honduras to pre-mountain region of El Salvador, Guatemala, 67 percent in Guatemala. Honduras, Nicaragua, Guanacaste in Costa Rica and Panama’s Arco Seco area. The Food and Agriculture Organization of the United Nations (FAO) – in collaboration with its Climate risks in the Dry Corridor are mainly partners and counterparts – is supporting the represented by recurrent droughts, excessive countries of the Dry Corridor in Central America rains and severe flooding affecting agricultural to increase the resilience of households, production, with greater intensity in degraded communities and institutions to prevent and areas. -
Drought: the Silent Harm of Migration from Central America During the Period 1990 to 2019
Preprints (www.preprints.org) | NOT PEER-REVIEWED | Posted: 25 June 2021 doi:10.20944/preprints202106.0612.v1 Articule Drought: the silent harm of migration from Central America during the period 1990 to 2019 Sazcha Olivera 1*, María del Pilar Fuerte-Celis 2 and Bernardo Bolaños 1, 1 universidad Autónoma Metropolitana 1; [email protected]; [email protected] 2 Centro de Investigación en Ciencias de la Información Geoespacial (Centro Geo); [email protected] * Correspondence: [email protected]; Tel.: (52 5535705169) Abstract: The worldwide number of migrants has had a rapid increase during the last fifteen years. Despite the extensive research studies that elucidate the in- crease in migrants' recipient countries, we know little about the relationship be- tween the climate factors and human mobility in the countries of origin. Hence, this study focuses on the effects of weather and the propensity of individuals to leave a territory by measuring the importance of rain precipitation or the lack of it in one of the critical food corridors of Central America, formed by El Salvador, Honduras, and Guatemala. To study the mobility process, we develop a stochas- tic frontier model; the main result shows a greater propensity to migrate when there is a significant drought event in the place of origin. Some other factors that motivate people to leave their homeland are the effect of other climate events measured through the control system (ENSO), homicide levels, economic per- formance, and exchange rate. The findings allow differentiating between drought and excess precipitation on a population and mobility to other territories. In ad- dition, these results permit us to derive observable implications of the different effects of flooding and drought and create public policies of prevention, mitiga- tion, and resilience. -
How Climate Change Is Driving Migration in Central America
College of Saint Benedict and Saint John's University DigitalCommons@CSB/SJU Celebrating Scholarship and Creativity Day Undergraduate Research 4-23-2020 "When Home Won't Let You Stay:" How Climate Change is Driving Migration in Central America Alejandra Gallardo College of Saint Benedict/Saint John's University, [email protected] Follow this and additional works at: https://digitalcommons.csbsju.edu/ur_cscday Recommended Citation Gallardo, Alejandra, ""When Home Won't Let You Stay:" How Climate Change is Driving Migration in Central America" (2020). Celebrating Scholarship and Creativity Day. 102. https://digitalcommons.csbsju.edu/ur_cscday/102 This Poster is brought to you for free and open access by DigitalCommons@CSB/SJU. It has been accepted for inclusion in Celebrating Scholarship and Creativity Day by an authorized administrator of DigitalCommons@CSB/ SJU. For more information, please contact [email protected]. “WHEN HOME WON’T LET YOU STAY:” ALEJANDRA GALLARDO FACULTY ADVISOR: JEAN LAVIGNE HOW CLIMATE CHANGE IS DRIVING SPRING 2020 CENTRAL AMERICAN MIGRATION SENIOR THESIS INTRODUCTION METHODS • Climate change acts as a “threat multiplier” that Original research — Central American amplifies social issues and inequities Delegation Trip: • As the impacts of climate change worsen, we expect to I collected a week’s worth of fieldnotes from 1 my participation in a delegation trip in see a greater number of people migrating Map Legend November 2019 with a grassroots organization • Agriculture-dependent communities have already -
Adaptation and Mitigation of Climate-Change Effects on Food and Nutrition Security in Honduras
LATIN AMERICA REGION Adaptation and mitigation of climate-change effects on food and nutrition security in Honduras José Lino Pacheco is Director of the Food and Nutrition Security Technical Unit, Secretary of General Coordination of the Government of Honduras and the SUN country Focal Point. Susan Lopez is a Food and Nutrition Security Specialist in the Food and Nutrition Security Technical Unit and Secretary of General Coordination in the Government of Honduras. Adriana Hernandez is an Associate Professor of Human Nutrition and Food Security in the Department of Food Sciences at Zamorano University, Honduras. Lucía Escobar is an Associate of the Nutrition Programme for the UN World Food Programme, Honduras. Introduction Policy and plans in Honduras to Honduras is a Central American country with a population of address climate change over nine million, comprising nine different ethnic groups. The main policy in Honduras that seeks to help the population Hunger is on the rise and is estimated to affect 1.4 million adapt to the effects of climate change is the Climate Change 1 people (2017) . Almost one in four (23%) children under Policy Framework. This includes objectives for agriculture, soil five years old (CU5) are stunted; in the more vulnerable and food security, as well as improving the resilience of crops 2 areas, this increases to one in every two . At the same time, and pastures to thermal and water stress and preventing or rapid urbanisation is leading to increased consumption of reducing the incidence of pests and diseases. Other objectives processed foods and lower levels of physical activity, which include combatting soil erosion, loss of productivity and are contributing to rising levels of overweight and obesity and desertification in order to preserve and improve the nutritional risk of nutrition-related non-communicable diseases (NCDs). -
Special Report Central America
SPECIAL REPORT www.cropmonitor.org SPECIAL REPORT www.cropmonitor.org updated October 21, 2019 Second consecutive year of failed yields for subsistence farmers in Central America’s Dry Corridor Highlights • Harvest of Primera (main season) maize and beans completed in September across Central America. • While national production was generally average across the region, with exception of Honduras, due to an increase in area planted, final yields were reduced due to irregular weather conditions during the start of the season including high temperatures, below-average and irregular rainfall, and extended dry spells, which resulted in severe soil moisture deficits. • In particular, subsistence and some larger-scale farmers along the Dry Corridor of Guatemala, Honduras, and Nicaragua without access to irrigation systems or riverine areas experienced Figure 1. Post-harvest conditions for the 2019 Primera season. source GEOGLAM Crop Monitor for Early Warning Bulletin, October 2019. significant crop losses ranging from 50 to 75 percent. • This is the second consecutive year of poor and failure Primera season harvests across the Dry Corridor, after the severe drought of 2018, and food security is of increasing concern for subsistence farmers. • Postrera (second maize season) planting, was delayed in some regions with rainfall deficits during late-August and was resumed in mid-September with increased rainfall amounts. Overview: Last year during the 2018 main producing Primera season, severe drought impacted large parts of Central America resulting in poor and failure conditions (See Figure 2). According to the UN FAO, the 2018 drought led to the loss of 280,000 hectares of beans and maize in Guatemala, El Salvador, Honduras, and Nicaragua, affecting the food security of more than two million people. -
Dry Spell in Northern Nicaragua Briefing Note – 24 July 2019
NICARAGUA Dry spell in northern Nicaragua Briefing note – 24 July 2019 A n El Niño phenomenon, although weak, has developed since February affecting several Central Accumulated Precipitation between 1 and 15 July American countries, in particular Guatemala, Honduras, El Salvador and Nicaragua, known as the Dry Corridor. Below-average rainfall since mid-June in northern Nicaragua is decreasing water availability and will likely result in a poor Primera harvest in August. Abnormally dry conditions are decreasing livelihood opportunities and will drive food insecurity. The most affected areas in northern Nicaragua are projected to experience Stressed (IPC-2) food security outcomes until January 2020. The most vulnerable households are likely to face Crisis (IPC-3) outcomes and are likely to resort to negative coping strategies, including reduced food consumption and sale of productive assets. Needs are likely to include food assistance, livelihood support and WASH. Source: INETER as of 15/07/2019 Key priorities Humanitarian constraints No significant access constraints reported. Food and Livelihoods Impacted by drought conditions Limitations Drinking water Up-to-date data on people in the affected area and their humanitarian For affected population, livestock conditions and needs is not currently available. Information regarding response and preparedness, as well as lessons learned from previous emergencies, is limited. Any questions? Please contact our analysis team leader, Benedetta Cordaro: [email protected] ACAPS Briefing Note: Drought Drivers of the crisis Although no widespread deterioration of vegetation health has been observed so far, the lack of rainfall is decreasing water availability and will likely result in a poor Primera A weak El Niño Southern Oscillation (ENSO) phenomenon has developed primarily in harvest in August (NOAA 18/07/2019; FEWS NET 06/2019). -
Food Security and Emigration in El Salvador, Guatemala and Honduras
FOOD SECURITY AND EMIGRATION Why people f lee and the impact on family members left behind in El Salvador, Guatemala and Honduras August 2017 FOOD SECURITY AND EMIGRATION To access the research report that informs this summary, follow this link/QR code https://docs.wfp.org/api/documents/WFP-0000019629/download/ © All rights reserved. No part of this publication may be reproduced, stored in a retrieval system, or transmitted in any form or by any means (electronic, mechanical, photocopying, recording or otherwise) without prior permission. This document has not been subject to an editorial review. This material has been funded by the World Food Programme, the Inter-American Development Bank and the International Fund for Agricultural Development; however the views expressed do not necessarily reflect the official position of IADB, IFAD, IOM, OAS or WFP. The responsibility for the opinions expressed in this report rests solely with the authors. 2 Foreword Migration, food security, violence and climate variability have been studied increasingly in recent years, both in Latin America and beyond, in an effort to better understand what drives people to leave their homes and countries. Yet often these issues have been examined separately rather than together. Migration from Central America to North America is not a new phenomenon, nor is it one that is likely to end soon. The number of irregular migrants apprehended at the United States of America border with Mexico increased fivefold from 2010 to 2015. The numbers of unaccompanied children picked up arriving from El Salvador, Guatemala, and Honduras surged between 2015 and 2016. This flow of people coincided with a period of heightened food insecurity in the Dry Corridor that traverses these three countries, known for its prolonged dry spells and droughts. -
FOOD SECURITY and EMIGRATION Why People F Lee and the Impact on Family Members Left Behind in El Salvador, Guatemala and Honduras
FOOD SECURITY AND EMIGRATION Why people f lee and the impact on family members left behind in El Salvador, Guatemala and Honduras Research report September 2017 FOOD SECURITY AND EMIGRATION A summary of this report is available at the following link/QR code: https://docs.wfp.org/api/documents/WFP-0000019632/download/ © All rights reserved. No part of this publication may be reproduced, stored in a retrieval system, or transmitted in any form or by any means (electronic, mechanical, photocopying, recording or otherwise) without prior permission. This document has not been subject to an editorial review. This material has been funded by the World Food Programme, the Inter-American Development Bank and the International Fund for Agricultural Development; however the views expressed do not necessarily reflect the official position of IADB, IFAD, IOM, OAS or WFP. The responsibility for the opinions expressed in this report rests solely with the authors. 2 Foreword Migration, food security, violence and climate variability have been studied increasingly in recent years, both in Latin America and beyond in an effort to better understand what drives people to leave their homes and countries. Yet often these issues have been examined separately rather than together. Migration from Central America to North America is not a new phenomenon, nor is it one that is likely to end soon. The number of irregular migrants apprehended at the United States of America border with Mexico increased fivefold from 2010 to 2015. The numbers of unaccompanied children picked up arriving from El Salvador, Guatemala, and Honduras surged between 2015 and 2016. -
Water Security in the Dry Corridor of Honduras (Phase 1) (P169901)
The World Bank Water Security in the Dry Corridor of Honduras (Phase 1) (P169901) Public Disclosure Authorized Project Information Document (PID) Public Disclosure Authorized Concept Stage | Date Prepared/Updated: 29-Jan-2019 | Report No: PIDC26169 Public Disclosure Authorized Public Disclosure Authorized Jan 22, 2019 Page 1 of 10 The World Bank Water Security in the Dry Corridor of Honduras (Phase 1) (P169901) BASIC INFORMATION A. Basic Project Data OPS TABLE Country Project ID Parent Project ID (if any) Project Name Honduras P169901 Water Security in the Dry Corridor of Honduras (Phase 1) (P169901) Region Estimated Appraisal Date Estimated Board Date Practice Area (Lead) LATIN AMERICA AND Aug 19, 2019 Dec 19, 2019 Water CARIBBEAN Financing Instrument Borrower(s) Implementing Agency Investment Project Financing Secretaria de Finanzas de Honduran Strategic Honduras (SEFIN) Investment Office (INVEST- H) Proposed Development Objective(s) The Project Development Objective (PDO) is to strengthen capacity for water resources management and improve water harvesting in the Dry Corridor of Honduras. PROJECT FINANCING DATA (US$, Millions) SUMMARY-NewFin1 Total Project Cost 60.00 Total Financing 60.00 of which IBRD/IDA 60.00 Financing Gap 0.00 DETAILS-NewFinEnh1 World Bank Group Financing International Development Association (IDA) 60.00 IDA Credit 60.00 Jan 22, 2019 Page 2 of 10 The World Bank Water Security in the Dry Corridor of Honduras (Phase 1) (P169901) Environmental and Social Risk Classification Concept Review Decision High Track II-The review did authorize the preparation to continue Other Decision (as needed) B. Introduction and Context Regional Context 1. The Central America Dry Corridor – stretching from Southern Mexico down to Panama - suffers from severe weather instability due to El Niño-Southern Oscillation (ENSO), and has highly vulnerable to climate shocks. -
Climate Change in Central America | Potential Impacts and Public Policy Options
1 Climate Change in Central America | Potential Impacts and Public Policy Options Thank you for your interest in this ECLAC publication ECLAC Publications Please register if you would like to receive information on our editorial products and activities. When you register, you may specify your particular areas of interest and you will gain access to our products in other formats. www.cepal.org/en/suscripciones Climate Change in Central America: Potential Impacts and Public Policy Options ALICIA BÁRCENA Executive Secretary MARIO CIMOLI Deputy Executive Secretary HUGO EDUARDO BETETA Director ECLAC Subregional Headquarters in Mexico JOSELUIS SAMANIEGO Director Sustainable Development and Human Settlements Division LUIS MIGUEL GALINDO Chief of the Climate Change Unit Sustainable Development and Human Settlements Division JULIE LENNOX Focal Point of Climate Change and Chief of the Agricultural Development Unit DIANA RAMÍREZ AND JAIME OLIVARES Researchers of the Agricultural Development and Economics of Climate Change Unit ECLAC Subregional Headquarters in Mexico This publication was based on analysis between 2008 and 2015 within the framework of “The Economics of Climate Change in Central America Initiative”, coordinated between the Ministries of Environment, Treasury or Finance, their Ministerial Councils and Executive Secretariats of the Central American Commission for Environment and Development (CCAD) and the Council of Ministers of Finance/Treasury of Central America and Dominic Republic (COSEFIN), and the Secretariat for Central American Economic Integration (SIECA), as bodies of the Central American Integration System (SICA) and the ECLAC Subregional Headquarters in Mexico; with financial support from UKAID/DFID and DANIDA. The agricultural series was coordinated with the Ministries of Agriculture of SICA, their Ministerial Council (CAC), its Executive Secretariat and Technical Group on Climate Change and Integrated Risk Management (GTCCGIR). -
How Climate Awareness Influences Farmers' Adaptation Decisions in Central America?
Journal of Rural Studies 64 (2018) 11–19 Contents lists available at ScienceDirect Journal of Rural Studies journal homepage: www.elsevier.com/locate/jrurstud How climate awareness influences farmers’ adaptation decisions in Central America? T ∗ Kauê de Sousaa,b,c, , Fernando Casanovesd, Jorge Sellaree, Alejandra Ospinad, ∗∗ Jose Gabriel Suchinif, Amilcar Aguilarg, Leida Mercadoa, a Tropical Agriculture and Higher Education Center (CATIE), Research Program in Development, Economy and Environment, Turrialba, Costa Rica b Inland Norway University of Applied Sciences, Department of Agricultural Sciences, Hamar, Norway c Bioversity International, Costa Rica Office, Turrialba, Costa Rica d Tropical Agriculture and Higher Education Center (CATIE), Biostatistics Unit, Turrialba, Costa Rica e University of Goettingen, Department of Agricultural Economics and Rural Development, Goettingen, Germany f Tropical Agriculture and Higher Education Center (CATIE), Guatemala Technical Office, Esquipulas, Guatemala g Tropical Agriculture and Higher Education Center (CATIE), Nicaragua Technical Office, Managua, Nicaragua ARTICLE INFO ABSTRACT Keywords: Central America is one of the regions with the highest vulnerability to climate change, with negative effects Adaptation to climate change projected to affect its economy and food security. To address this issue, an integrative farm management ap- Bradley-Terry model proach such as Climate-Smart Agriculture can help reorient agricultural practices towards climate adaptation Central America dry corridor and food security. Past studies have shown that several factors can either hinder or encourage the adoptions of Climate change Climate-Smart practices, including subjective expectations and perceptions. Building on this literature, we Climate-smart agriculture analyze farmers' climate awareness and their perceptions regarding the change in climate patterns as well as Farmer field schools Reforestation their choices of farming practices to adapt to these changes. -
Institutionality and Governance in the Central American Dry Corridor
Policy Brief 2014 Institutionality and Governance in the Central American Dry Corridor SALVADORAN RESEARCH PROGRAM ON DEVELOPMENT AND ENVIRONMENT INTRODUCTION ISSUE Climate change posits a new series of enormous challenges for development in Central America. The many threats and impacts that it now faces include temperature changes and new temporal and spatial patterns of precipitation, as well as extreme events and droughts. These many threats and impacts from climate change converge in a particularly dramatic way in the Central American Dry Corridor (CADC), a region that includes approximately 30% of the territory of Central America (ACH and FAO, 2012) and is home to the greatest density of population and important economic activities in the region. dljlajlsjllklkjljlkjlk Climate change magnifies the existing situation of environmental degradation in the CADC and amplifies the vulnerability of the area. This affects agricultural production and threatens food security. Accordingly, it represents a threat to the region’s governance and development opportunities. TERRITORIES, The CADC is a space with common characteristics but also with unique aspects that stem ACTORS AND from the diverse territories that comprise it, including such subnational territories as the dry STRATEGIES east (Oriente Seco) of Guatemala and such trinational territories as the Gulf of Fonseca, each emblematic in the way that they allow us to understand the diversity of existing initiatives, actors, and challenges for governance. National and local governments, rural