Data Quality and Reproducibility in Preclinical Research
Total Page:16
File Type:pdf, Size:1020Kb
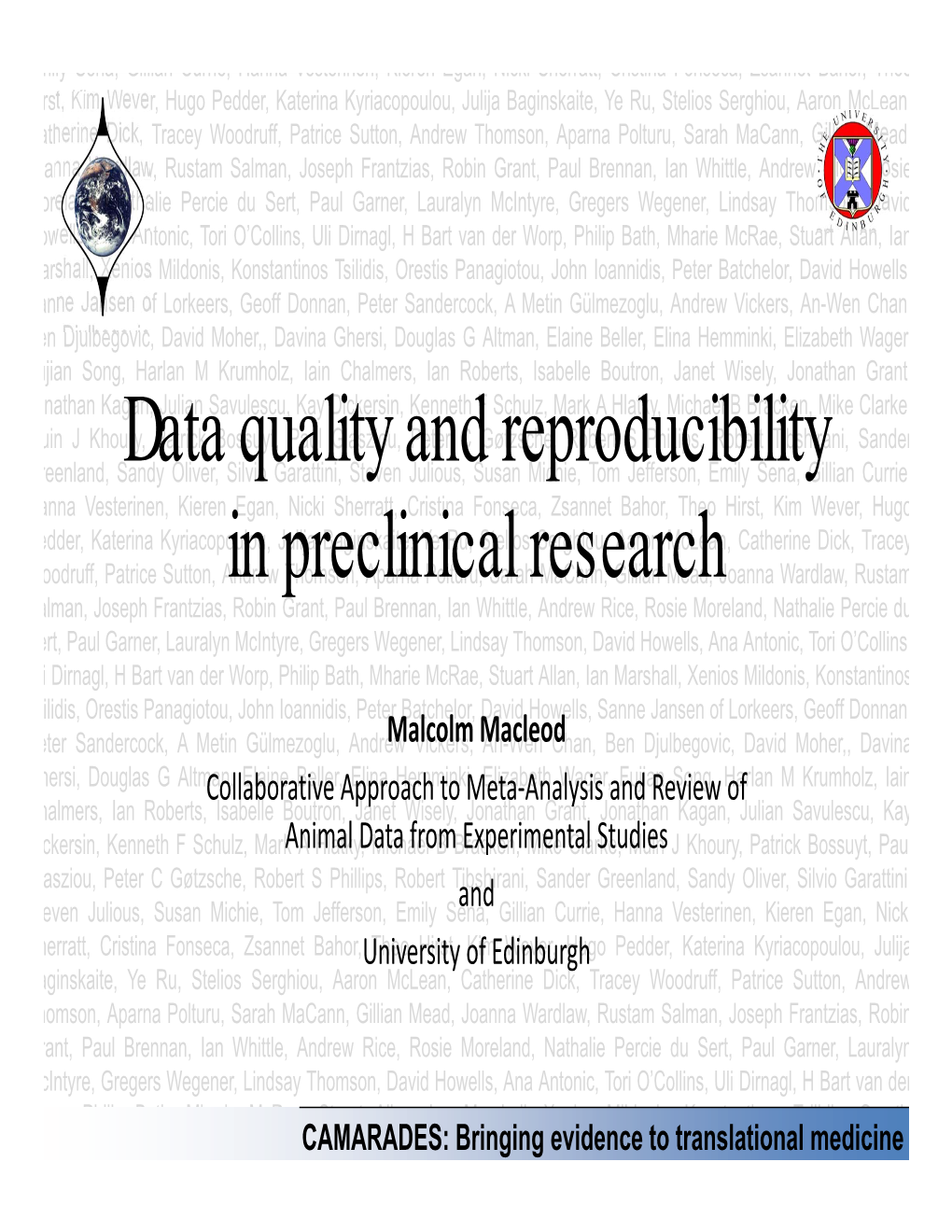
Load more
Recommended publications
-
Reproducibility, Replicability, and Generalization in the Social, Behavioral, and Economic Sciences
REPRODUCIBILITY, REPLICABILITY, AND GENERALIZATION IN THE SOCIAL, BEHAVIORAL, AND ECONOMIC SCIENCES Report of the Subcommittee on Replicability in Science of the SBE Advisory Committee to the National Science Foundation 13 May 2015 Presentation at SBE AC Spring Meeting by K. Bollen. Report of the Subcommittee on Replicability in Science Advisory Committee to the NSF SBE Directorate Subcommittee Members: Kenneth Bollen (University of North Carolina at Chapel Hill, Cochair) John T. Cacioppo (University of Chicago, Cochair) Robert M. Kaplan (Agency for Healthcare Research and Quality) Jon A. Krosnick (Stanford University) James L. Olds (George Mason University) Staff Assistance Heather Dean (National Science Foundation) TOPICS I. Background II. Subcommittee Report III. Definitions IV. Recommendations V. Final Comments Background • Spring, 2013 o NSF SBE Advisory Committee establishes subcommittee on how SBE can promote robust research practices • Summer & Fall 2013 o Subcommittee proposal for workshop on “Robust Research in the Social, Behavioral, and Economic Sciences.” • February 20-21, 2014 o Workshop convened o Participants drawn from variety of universities, funding agencies, scientific associations, and journals o Cover broad range of issues from extent and cause of problems to possible solutions o Details are in the appendix of the circulated report • Post-workshop period o Document & digest workshop content o Discuss and propose recommendations o Complete report Subcommittee Report • DEFINITIONS o No consensus in science on the meanings -
Meta Analyses – Pros and Cons
Meta analyses – pros and cons Emily S Sena, PhD Centre for Clinical Brain Sciences, University of Edinburgh @camarades_ CAMARADES: Bringing evidence to translational medicine CAMARADES • Collaborative Approach to Meta-Analysis and Review of Animal Data from Experimental Studies • Look systematically across the modelling of a range of conditions • Data Repository – 30 Diseases – 40 Projects – 25,000 studies – from over 400,000 animals CAMARADES: Bringing evidence to translational medicine Why do we do meta-analysis of animal studies? • Animal models are generally performed to inform human health but when should you be convinced to move to the next step? • Systematic reviews & meta-analyses: – assess the quality and range of evidence – identify gaps in the field – quantify relative utility of outcome measures – inform power/sample size calculations – assess for publication bias – try to explain discrepancies between preclinical and clinical trial results – inform clinical trial design CAMARADES: Bringing evidence to translational medicine Systematic review and meta-analysis • Pros – What have we learnt about…….. • Translation? • Quality? • The 3Rs? • Cons – What are the limitations? • As good as the data that goes in? • Rapidly outdated • The Impact CAMARADES: Bringing evidence to translational medicine Systematic review and meta-analysis • Pros – What have we learnt about…….. • Translation? • Quality? • The 3Rs? • Cons – What are the limitations? • As good as the data that goes in? • Rapidly outdated • The Impact CAMARADES: Bringing evidence -
Melanoma: Epidemiology, Risk Factors, Pathogenesis, Diagnosis and Classification
in vivo 28: 1005-1012 (2014) Review Melanoma: Epidemiology, Risk Factors, Pathogenesis, Diagnosis and Classification MARCO RASTRELLI1, SAVERIA TROPEA1, CARLO RICCARDO ROSSI2 and MAURO ALAIBAC3 1Melanoma and Sarcoma Unit, Veneto Institute of Oncology, IOV- IRCCS, Padova, Italy; 2Melanoma and Sarcoma Unit, Veneto Institute of Oncology, IOV-IRCCS and Department of Surgery, Oncology and Gastroenterology, University of Padova, Padova, Italy; 3Dermatology Unit, University of Padova, Padova, Italy Abstract. This article reviews epidemiology, risk factors, Epidemiology pathogenesis and diagnosis of melanoma. Data on melanoma from the majority of countries show a rapid At the start of 21st century, melanoma remains a potentially increase of the incidence of this cancer, with a slowing of fatal malignancy. At a time when the incidence of many the rate of incidence in the period 1990-2000. Males are tumor types is decreasing, melanoma incidence continues to approximately 1.5-times more likely to develop melanoma increase (1). Although most patients have localized disease than females, while according to other studies, the different at the time of the diagnosis and are treated by surgical prevalence in both sexes must be analyzed in relation with excision of the primary tumor, many patients develop age: the incidence rate of melanoma is grater in women metastases (2). than men until they reach the age of 40 years, however, by The incidence of malignant melanoma has been increasing 75 years of age, the incidence is almost 3-times as high in worldwide, resulting in an important socio-economic men versus women. The most important and potentially problem. From being a rare cancer one century ago, the modifiable environmental risk factor for developing average lifetime risk for melanoma has now reached 1 in 50 malignant melanoma is the exposure to ultraviolet (UV) in many Western populations (3). -
Optimizing Product Lifecycle Management with Real World
1/17/2019 Optimizing Product Lifecycle Management With Real-World Evidence :: Medtech Insight This copy is for your personal, non-commercial use. For high-quality copies or electronic reprints for distribution to colleagues or customers, please call +44 (0) 20 3377 3183 Printed By Optimizing Product Lifecycle Management With Real- World Evidence 27 Dec 2018 SPONSORED Executive Summary Thought Leadership In Association With IQVIA An increasingly data-rich healthcare sector presents both opportunities and challenges for the MedTech industry, just as it does for the health systems and patients served by MedTech products. Used intelligently and appropriately, the vast quantities of real-world data emanating from multiple healthcare sources, such as electronic medical records (EMRs), claims databases, products and disease registries, provide the raw material for real-world evidence (RWE) that can inform strategy and decision- making throughout the MedTech-product lifecycle. An increasingly data-rich healthcare sector presents both opportunities and challenges for the MedTech industry, just as it does for the health systems and patients served by MedTech products. Used intelligently and appropriately, the vast quantities of real-world data emanating from multiple healthcare sources, such as electronic medical records (EMRs), claims databases, products and disease registries, provide the raw material for real-world evidence (RWE) that can inform strategy and decision-making throughout the MedTech-product lifecycle. “What we’re seeing in the industry -
Adaptive Enrichment Designs in Clinical Trials
Annual Review of Statistics and Its Application Adaptive Enrichment Designs in Clinical Trials Peter F. Thall Department of Biostatistics, M.D. Anderson Cancer Center, University of Texas, Houston, Texas 77030, USA; email: [email protected] Annu. Rev. Stat. Appl. 2021. 8:393–411 Keywords The Annual Review of Statistics and Its Application is adaptive signature design, Bayesian design, biomarker, clinical trial, group online at statistics.annualreviews.org sequential design, precision medicine, subset selection, targeted therapy, https://doi.org/10.1146/annurev-statistics-040720- variable selection 032818 Copyright © 2021 by Annual Reviews. Abstract All rights reserved Adaptive enrichment designs for clinical trials may include rules that use in- terim data to identify treatment-sensitive patient subgroups, select or com- pare treatments, or change entry criteria. A common setting is a trial to Annu. Rev. Stat. Appl. 2021.8:393-411. Downloaded from www.annualreviews.org compare a new biologically targeted agent to standard therapy. An enrich- ment design’s structure depends on its goals, how it accounts for patient heterogeneity and treatment effects, and practical constraints. This article Access provided by University of Texas - M.D. Anderson Cancer Center on 03/10/21. For personal use only. first covers basic concepts, including treatment-biomarker interaction, pre- cision medicine, selection bias, and sequentially adaptive decision making, and briefly describes some different types of enrichment. Numerical illus- trations are provided for qualitatively different cases involving treatment- biomarker interactions. Reviews are given of adaptive signature designs; a Bayesian design that uses a random partition to identify treatment-sensitive biomarker subgroups and assign treatments; and designs that enrich superior treatment sample sizes overall or within subgroups, make subgroup-specific decisions, or include outcome-adaptive randomization. -
Use of in Vivo and in Vitro Models to Guide Dose Selection for Neonatal Infections
Use of In Vivo and In Vitro Models to Guide Dose Selection for Neonatal Infections Professor William Hope Antimicrobial Pharmacodynamics & Therapeutics University of Liverpool September 2016 Disclosures • William Hope has received research funding from Pfizer, Gilead, Astellas, AiCuris, Amplyx, Spero Therapeutics and F2G, and acted as a consultant and/or given talks for Pfizer, Basilea, Astellas, F2G, Nordic Pharma, Medicines Company, Amplyx, Mayne Pharma, Spero Therapeutics, Auspherix, Cardeas and Pulmocide. First of all BIOMARKER OUTCOME OF CLINICAL DOSE INTEREST/IMPORTANCE •Decline in Log10CFU/mL •Linked to an outcome of clinical interest •Survival 7 6 5 4 CFU/g) CNS 10 3 Effect (log 2 1 0 0.01 0.1 1 10 100 1000 Total doseDose 5FC (mg/kg) PHARMACOKINETICS PHARMACODYNAMICS Concept of Expensive Failure COST Derisking White Powder Preclinical Program Clinical Program Concept from Trevor Mundell & Gates Foundation And this… Normal Therapeutics Drug A, Dose A1 versus Drug B, Dose B1 Pharmacodynamics is the Bedrock of ALL Therapeutics…it sits here without being seen One of the reasons simple scaling does not work is the fact pharmacodynamics are different Which means the conditions that govern exposure response relationships in neonates need to be carefully considered …Ignore these at your peril Summary of Preclinical Pharmacodynamic Studies for Neonates** • Hope el al JID 2008 – Micafungin for neonates – Primary question of HCME – Rabbit model with hematogenous dissemination • Warn et al AAC 2010 – Anidulafungin for neonates – Primary question -
Metabolic Sensor Governing Bacterial Virulence in Staphylococcus Aureus
Metabolic sensor governing bacterial virulence in PNAS PLUS Staphylococcus aureus Yue Dinga, Xing Liub, Feifei Chena, Hongxia Dia, Bin Xua, Lu Zhouc, Xin Dengd,e, Min Wuf, Cai-Guang Yangb,1, and Lefu Lana,1 aDepartment of Molecular Pharmacology and bChinese Academy of Sciences Key Laboratory of Receptor Research, Shanghai Institute of Materia Medica, Chinese Academy of Sciences, Shanghai 201203, China; cDepartment of Medicinal Chemistry, School of Pharmacy, Fudan University, Shanghai 201203, China; dDepartment of Chemistry and eInstitute for Biophysical Dynamics, The University of Chicago, Chicago, IL 60637; and fDepartment of Basic Sciences University of North Dakota School of Medicine and Health Sciences, Grand Forks, ND 58203 Edited by Richard P. Novick, New York University School of Medicine, New York, NY, and approved October 14, 2014 (received for review June 13, 2014) An effective metabolism is essential to all living organisms, in- To survive and replicate efficiently in the host, S. aureus has cluding the important human pathogen Staphylococcus aureus.To developed exquisite mechanisms for scavenging nutrients and establish successful infection, S. aureus must scavenge nutrients adjusting its metabolism to maintain growth while also coping with and coordinate its metabolism for proliferation. Meanwhile, it also stress (6, 11). On the other hand, S. aureus produces a wide array must produce an array of virulence factors to interfere with host of virulence factors to evade host immune defenses and to derive defenses. However, the ways in which S. aureus ties its metabolic nutrition either parasitically or destructively from the host during state to its virulence regulation remain largely unknown. Here we infections (6). -
Principles of Validation of Diagnostic Assays for Infectious Diseases1
PRINCIPLES OF VALIDATION OF DIAGNOSTIC ASSAYS FOR INFECTIOUS DISEASES1 R. H. JACOBSON, New York State College of Veterinary Medicine, Cornell University, Ithaca, New York, USA Abstract PRINCIPLES OF VALIDATION OF DIAGNOSTIC ASSAYS FOR INFECTIOUS DISEASES. Assay validation requires a series of inter-related processes. Assay validation is an experimental process: reagents and protocols are optimized by experimentation to detect the analyte with accuracy and precision. Assay validation is a relative process: its diagnostic sensitivity and diagnostic specificity are calculated relative to test results obtained from reference animal populations of known infection/exposure status. Assay validation is a conditional process: classification of animals in the target population as infected or uninfected is conditional upon how well the reference animal population used to validate the assay represents the target population; accurate predictions of the infection status of animals from test results (PV+ and PV−) are conditional upon the estimated prevalence of disease/infection in the target population. Assay validation is an incremental process: confidence in the validity of an assay increases over time when use confirms that it is robust as demonstrated by accurate and precise results; the assay may also achieve increasing levels of validity as it is upgraded and extended by adding reference populations of known infection status. Assay validation is a continuous process: the assay remains valid only insofar as it continues to provide accurate and precise results as proven through statistical verification. Therefore, the work required for validation of diagnostic assays for infectious diseases does not end with a time-limited series of experiments based on a few reference samples rather, to assure valid test results from an assay requires constant vigilance and maintenance of the assay, along with reassessment of its performance characteristics for each unique population of animals to which it is applied. -
New NIH Guidelines to Improve Experimental Rigor and Reproducibility
New NIH guidelines to improve Experimental Rigor and Reproducibility Oswald Steward Professor, Department of Anatomy & Neurobiology Senior Associate Dean for Research University of California School of Medicine What I’ll tell you 1) Background: Increasing reports of failures to replicate 2) Previous actions by NIH to enhance rigor 3) New requirements for grants submitted after January 2016 4) What NIH will be looking for in new required sections 5) Some suggested best practices to meet new standards for scientific rigor • The Problem: Numerous recent reports of findings that can’t be replicated. Failure to replicate key results from preclinical cancer studies: 1) Prinz et al. (2011) Believe it or not: How much can we rely on published data on potential drug targets? Nature Reviews Drug Discovery. Inconsistencies were found in 2/3 studies 2) Begley and Ellis (2012) Raise standards for preclinical cancer research. Nature. Only 6/53 “landmark” papers replicated 3) Begley (2013) Six red flags for suspect work. Nature. -Were experiments performed blinded? -Were basic experiments repeated? -Were all the results presented? -Were there positive and negative controls? -Were reagents validated? -Were statistical tests appropriate? • In ALS field, Scott et al., (2008) re-assessed 70 drugs that had been reported to increase lifespan in an animal model of ALS (SOD1-G93A knockout mice). • 221 separate studies were carried out over a five year period that involved over 18,000 mice. • Some drugs were already in clinical trials. • Outcome: There was no statistically significant positive effect for any of the 70 compounds tested. • Conclusion: Previously published effects were measurements of noise in the distribution of survival means as opposed to an actual drug effect. -
Studies of Staphylococcal Infections. I. Virulence of Staphy- Lococci and Characteristics of Infections in Embryonated Eggs * WILLIAM R
Journal of Clinical Investigation Vol. 43, No. 11, 1964 Studies of Staphylococcal Infections. I. Virulence of Staphy- lococci and Characteristics of Infections in Embryonated Eggs * WILLIAM R. MCCABE t (From the Research Laboratory, West Side Veterans Administration Hospital, and the Department of Medicine, Research and Educational Hospitals, University of Illinois College of Medicine, Chicago, Ill.) Many of the determinants of the pathogenesis niques still require relatively large numbers of and course of staphylococcal infections remain staphylococci to produce infection (19). Fatal imprecisely defined (1, 2) despite their increas- systemic infections have been equally difficult to ing importance (3-10). Experimental infections produce in animals and have necessitated the in- in suitable laboratory animals have been of con- jection of 107 to 109 bacteria (20-23). A few siderable assistance in clarifying the role of host strains of staphylococci have been found that are defense mechanisms and specific bacterial virulence capable of producing lethal systemic infections factors with a variety of other infectious agents. with inocula of from 102 to 103 bacteria (24) and A sensitive experimental model would be of value have excited considerable interest (25-27). The in defining the importance of these factors in virulence of these strains apparently results from staphylococcal infections, but both humans and an unusual antigenic variation (27, 28) which, the usual laboratory animals are relatively re- despite its interest, is of doubtful significance in sistant. Extremely large numbers of staphylo- human staphylococcal infection, since such strains cocci are required to produce either local or sys- have been isolated only rarely from clinical in- temic infections experimentally. -
Role and Limitations of Epidemiology in Establishing a Causal Association Eduardo L
Seminars in Cancer Biology 14 (2004) 413–426 Role and limitations of epidemiology in establishing a causal association Eduardo L. Franco a,∗, Pelayo Correa b, Regina M. Santella c, Xifeng Wu d, Steven N. Goodman e, Gloria M. Petersen f a Departments of Epidemiology and Oncology, McGill University, 546 Pine Avenue West, Montreal, QC, Canada H2W1S6 b Department of Pathology, Louisiana State University Health Sciences Center, New Orleans, LA, USA c Department of Environmental Health Sciences, Mailman School of Public Health, Columbia University, New York, NY, USA d Department of Epidemiology, University of Texas MD Anderson Cancer Center, Houston, TX, USA e Department of Biostatistics, Bloomberg School of Public Health, Johns Hopkins University, Baltimore, MD, USA f Department of Health Sciences Research, Mayo Clinic College of Medicine, Rochester, MN, USA Abstract Cancer risk assessment is one of the most visible and controversial endeavors of epidemiology. Epidemiologic approaches are among the most influential of all disciplines that inform policy decisions to reduce cancer risk. The adoption of epidemiologic reasoning to define causal criteria beyond the realm of mechanistic concepts of cause-effect relationships in disease etiology has placed greater reliance on controlled observations of cancer risk as a function of putative exposures in populations. The advent of molecular epidemiology further expanded the field to allow more accurate exposure assessment, improved understanding of intermediate endpoints, and enhanced risk prediction by incorporating the knowledge on genetic susceptibility. We examine herein the role and limitations of epidemiology as a discipline concerned with the identification of carcinogens in the physical, chemical, and biological environment. We reviewed two examples of the application of epidemiologic approaches to aid in the discovery of the causative factors of two very important malignant diseases worldwide, stomach and cervical cancers. -
On the Reproducibility of Meta-Analyses.Docx
On the Reproducibility of Meta-Analyses 1 1 RUNNING HEAD: ON THE REPRODUCIBILITY OF META-ANALYSES 2 3 4 On the Reproducibility of Meta-Analyses: Six Practical Recommendations 5 6 Daniël Lakens 7 Eindhoven University of Technology 8 Joe Hilgard 9 University of Missouri - Columbia 10 Janneke Staaks 11 University of Amsterdam 12 13 In Press, BMC Psychology 14 15 Word Count: 6371 16 17 Keywords: Meta-Analysis; Reproducibility; Open Science; Reporting Guidelines 18 19 Author Note: Correspondence can be addressed to Daniël Lakens, Human Technology 20 Interaction Group, IPO 1.33, PO Box 513, 5600MB Eindhoven, The Netherlands. E-mail: 21 [email protected]. We would like to thank Bianca Kramer for feedback on a previous draft 22 of this manuscript. 23 24 Competing Interests: The authors declared that they had no competing interest with respect 25 to their authorship or the publication of this article. 26 27 Author Contributions: All authors contributed writing this manuscript. DL drafted the 28 manuscript, DL, JH, and JS revised the manuscript. All authors read and approved the final 29 manuscript. 30 31 Email Addresses: D. Lakens: [email protected] 32 Joe Hilgard: [email protected] 33 Janneke Staaks: [email protected] On the Reproducibility of Meta-Analyses 2 34 Abstract 35 Background 36 Meta-analyses play an important role in cumulative science by combining information 37 across multiple studies and attempting to provide effect size estimates corrected for 38 publication bias. Research on the reproducibility of meta-analyses reveals that errors are 39 common, and the percentage of effect size calculations that cannot be reproduced is much 40 higher than is desirable.