Malliavin Calculus and Its Applications
Total Page:16
File Type:pdf, Size:1020Kb
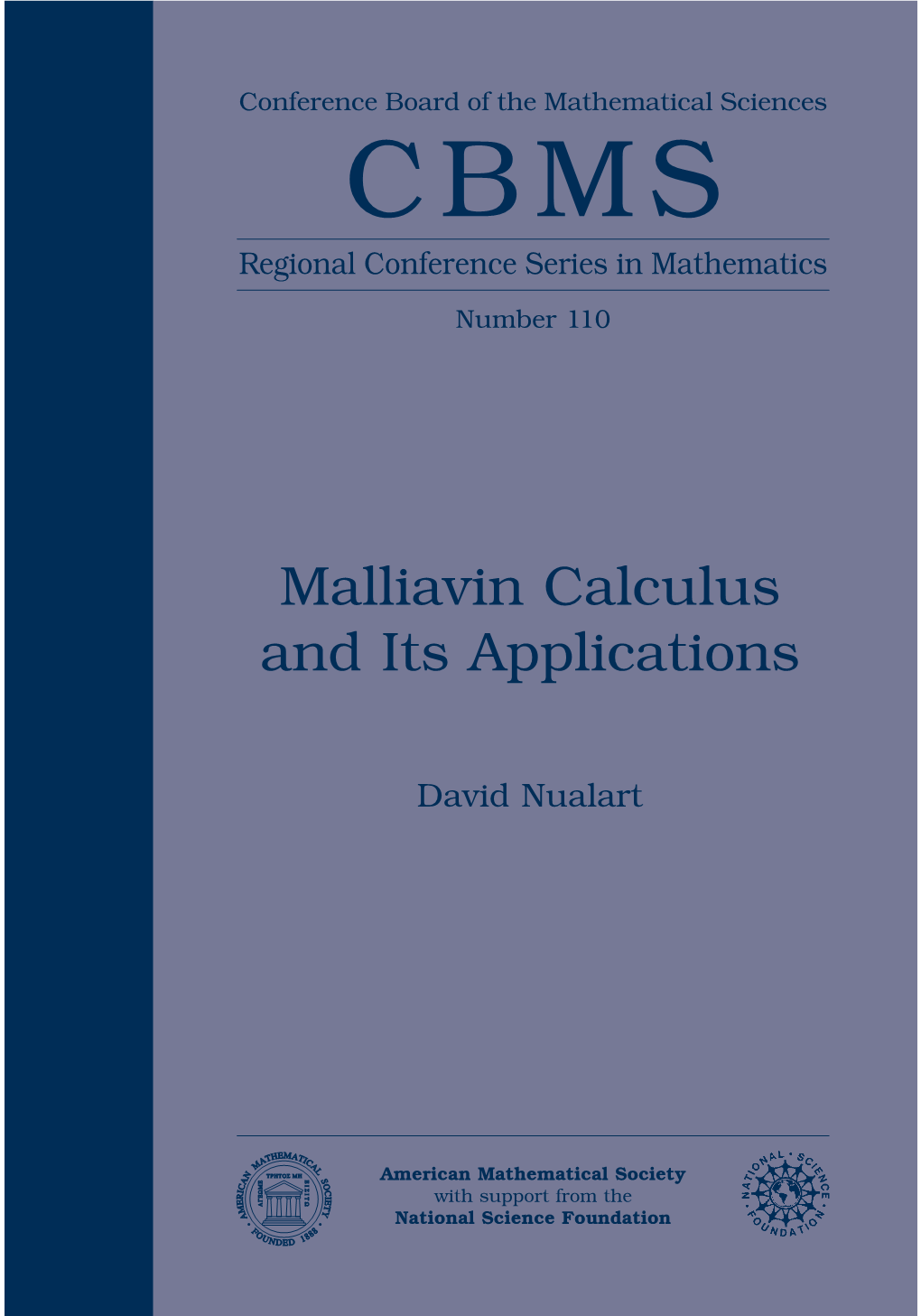
Load more
Recommended publications
-
Application of Malliavin Calculus and Wiener Chaos to Option Pricing Theory
Application of Malliavin Calculus and Wiener Chaos to Option Pricing Theory by Eric Ben-Hamou The London School of Economics and Political Science Thesis submitted in partial fulfilment of the requirements of the degree of Doctor of Philosophy at the University of London UMI Number: U615447 All rights reserved INFORMATION TO ALL USERS The quality of this reproduction is dependent upon the quality of the copy submitted. In the unlikely event that the author did not send a complete manuscript and there are missing pages, these will be noted. Also, if material had to be removed, a note will indicate the deletion. Dissertation Publishing UMI U615447 Published by ProQuest LLC 2014. Copyright in the Dissertation held by the Author. Microform Edition © ProQuest LLC. All rights reserved. This work is protected against unauthorized copying under Title 17, United States Code. ProQuest LLC 789 East Eisenhower Parkway P.O. Box 1346 Ann Arbor, Ml 48106-1346 weseS F 7855 % * A bstract This dissertation provides a contribution to the option pricing literature by means of some recent developments in probability theory, namely the Malliavin Calculus and the Wiener chaos theory. It concentrates on the issue of faster convergence of Monte Carlo and Quasi-Monte Carlo simulations for the Greeks, on the topic of the Asian option as well as on the approximation for convexity adjustment for fixed income derivatives. The first part presents a new method to speed up the convergence of Monte- Carlo and Quasi-Monte Carlo simulations of the Greeks by means of Malliavin weighted schemes. We extend the pioneering works of Fournie et al. -
Malliavin Calculus in the Canonical Levy Process: White Noise Theory and Financial Applications
Purdue University Purdue e-Pubs Open Access Dissertations Theses and Dissertations January 2015 Malliavin Calculus in the Canonical Levy Process: White Noise Theory and Financial Applications. Rolando Dangnanan Navarro Purdue University Follow this and additional works at: https://docs.lib.purdue.edu/open_access_dissertations Recommended Citation Navarro, Rolando Dangnanan, "Malliavin Calculus in the Canonical Levy Process: White Noise Theory and Financial Applications." (2015). Open Access Dissertations. 1422. https://docs.lib.purdue.edu/open_access_dissertations/1422 This document has been made available through Purdue e-Pubs, a service of the Purdue University Libraries. Please contact [email protected] for additional information. Graduate School Form 30 Updated 1/15/2015 PURDUE UNIVERSITY GRADUATE SCHOOL Thesis/Dissertation Acceptance This is to certify that the thesis/dissertation prepared By Rolando D. Navarro, Jr. Entitled Malliavin Calculus in the Canonical Levy Process: White Noise Theory and Financial Applications For the degree of Doctor of Philosophy Is approved by the final examining committee: Frederi Viens Chair Jonathon Peterson Michael Levine Jose Figueroa-Lopez To the best of my knowledge and as understood by the student in the Thesis/Dissertation Agreement, Publication Delay, and Certification Disclaimer (Graduate School Form 32), this thesis/dissertation adheres to the provisions of Purdue University’s “Policy of Integrity in Research” and the use of copyright material. Approved by Major Professor(s): Frederi Viens Approved by: Jun Xie 11/24/2015 Head of the Departmental Graduate Program Date MALLIAVIN CALCULUS IN THE CANONICAL LEVY´ PROCESS: WHITE NOISE THEORY AND FINANCIAL APPLICATIONS A Dissertation Submitted to the Faculty of Purdue University by Rolando D. Navarro, Jr. -
Weak Approximations for Arithmetic Means of Geometric Brownian Motions and Applications to Basket Options Romain Bompis
Weak approximations for arithmetic means of geometric Brownian motions and applications to Basket options Romain Bompis To cite this version: Romain Bompis. Weak approximations for arithmetic means of geometric Brownian motions and applications to Basket options. 2017. hal-01502886 HAL Id: hal-01502886 https://hal.archives-ouvertes.fr/hal-01502886 Preprint submitted on 6 Apr 2017 HAL is a multi-disciplinary open access L’archive ouverte pluridisciplinaire HAL, est archive for the deposit and dissemination of sci- destinée au dépôt et à la diffusion de documents entific research documents, whether they are pub- scientifiques de niveau recherche, publiés ou non, lished or not. The documents may come from émanant des établissements d’enseignement et de teaching and research institutions in France or recherche français ou étrangers, des laboratoires abroad, or from public or private research centers. publics ou privés. Weak approximations for arithmetic means of geometric Brownian motions and applications to Basket options ∗ Romain Bompis y Abstract. In this work we derive new analytical weak approximations for arithmetic means of geometric Brownian mo- tions using a scalar log-normal Proxy with an averaged volatility. The key features of the approach are to keep the martingale property for the approximations and to provide new integration by parts formulas for geometric Brownian motions. Besides, we also provide tight error bounds using Malliavin calculus, estimates depending on a suitable dispersion measure for the volatilities and on the maturity. As applications we give new price and im- plied volatility approximation formulas for basket call options. The numerical tests reveal the excellent accuracy of our results and comparison with the other known formulas of the literature show a valuable improvement. -
Chaotic Decompositions in Z2-Graded Quantum Stochastic Calculus
QUANTUM PROBABILITY BANACH CENTER PUBLICATIONS, VOLUME 43 INSTITUTE OF MATHEMATICS POLISH ACADEMY OF SCIENCES WARSZAWA 1998 CHAOTIC DECOMPOSITIONS IN Z2-GRADED QUANTUM STOCHASTIC CALCULUS TIMOTHY M. W. EYRE Department of Mathematics, University of Nottingham Nottingham, NG7 2RD, UK E-mail: [email protected] Abstract. A brief introduction to Z2-graded quantum stochastic calculus is given. By inducing a superalgebraic structure on the space of iterated integrals and using the heuristic classical relation df(Λ) = f(Λ+dΛ)−f(Λ) we provide an explicit formula for chaotic expansions of polynomials of the integrator processes of Z2-graded quantum stochastic calculus. 1. Introduction. A theory of Z2-graded quantum stochastic calculus was was in- troduced in [EH] as a generalisation of the one-dimensional Boson-Fermion unification result of quantum stochastic calculus given in [HP2]. Of particular interest in Z2-graded quantum stochastic calculus is the result that the integrators of the theory provide a time-indexed family of representations of a broad class of Lie superalgebras. The notion of a Lie superalgebra was introduced in [K] and has received considerable attention since. It is essentially a Z2-graded analogue of the notion of a Lie algebra with a bracket that is, in a certain sense, partly a commutator and partly an anticommutator. Another work on Lie superalgebras is [S] and general superalgebras are treated in [C,S]. The Lie algebra representation properties of ungraded quantum stochastic calculus enabled an explicit formula for the chaotic expansion of elements of an associated uni- versal enveloping algebra to be developed in [HPu]. -
A Stochastic Fractional Calculus with Applications to Variational Principles
fractal and fractional Article A Stochastic Fractional Calculus with Applications to Variational Principles Houssine Zine †,‡ and Delfim F. M. Torres *,‡ Center for Research and Development in Mathematics and Applications (CIDMA), Department of Mathematics, University of Aveiro, 3810-193 Aveiro, Portugal; [email protected] * Correspondence: delfi[email protected] † This research is part of first author’s Ph.D. project, which is carried out at the University of Aveiro under the Doctoral Program in Applied Mathematics of Universities of Minho, Aveiro, and Porto (MAP). ‡ These authors contributed equally to this work. Received: 19 May 2020; Accepted: 30 July 2020; Published: 1 August 2020 Abstract: We introduce a stochastic fractional calculus. As an application, we present a stochastic fractional calculus of variations, which generalizes the fractional calculus of variations to stochastic processes. A stochastic fractional Euler–Lagrange equation is obtained, extending those available in the literature for the classical, fractional, and stochastic calculus of variations. To illustrate our main theoretical result, we discuss two examples: one derived from quantum mechanics, the second validated by an adequate numerical simulation. Keywords: fractional derivatives and integrals; stochastic processes; calculus of variations MSC: 26A33; 49K05; 60H10 1. Introduction A stochastic calculus of variations, which generalizes the ordinary calculus of variations to stochastic processes, was introduced in 1981 by Yasue, generalizing the Euler–Lagrange equation and giving interesting applications to quantum mechanics [1]. Recently, stochastic variational differential equations have been analyzed for modeling infectious diseases [2,3], and stochastic processes have shown to be increasingly important in optimization [4]. In 1996, fifteen years after Yasue’s pioneer work [1], the theory of the calculus of variations evolved in order to include fractional operators and better describe non-conservative systems in mechanics [5]. -
A Short History of Stochastic Integration and Mathematical Finance
A Festschrift for Herman Rubin Institute of Mathematical Statistics Lecture Notes – Monograph Series Vol. 45 (2004) 75–91 c Institute of Mathematical Statistics, 2004 A short history of stochastic integration and mathematical finance: The early years, 1880–1970 Robert Jarrow1 and Philip Protter∗1 Cornell University Abstract: We present a history of the development of the theory of Stochastic Integration, starting from its roots with Brownian motion, up to the introduc- tion of semimartingales and the independence of the theory from an underlying Markov process framework. We show how the development has influenced and in turn been influenced by the development of Mathematical Finance Theory. The calendar period is from 1880 to 1970. The history of stochastic integration and the modelling of risky asset prices both begin with Brownian motion, so let us begin there too. The earliest attempts to model Brownian motion mathematically can be traced to three sources, each of which knew nothing about the others: the first was that of T. N. Thiele of Copen- hagen, who effectively created a model of Brownian motion while studying time series in 1880 [81].2; the second was that of L. Bachelier of Paris, who created a model of Brownian motion while deriving the dynamic behavior of the Paris stock market, in 1900 (see, [1, 2, 11]); and the third was that of A. Einstein, who proposed a model of the motion of small particles suspended in a liquid, in an attempt to convince other physicists of the molecular nature of matter, in 1905 [21](See [64] for a discussion of Einstein’s model and his motivations.) Of these three models, those of Thiele and Bachelier had little impact for a long time, while that of Einstein was immediately influential. -
Stochastic Differential Equations and Hypoelliptic
. STOCHASTIC DIFFERENTIAL EQUATIONS AND HYPOELLIPTIC OPERATORS Denis R. Bell Department of Mathematics University of North Florida Jacksonville, FL 32224 U. S. A. In Real and Stochastic Analysis, 9-42, Trends Math. ed. M. M. Rao, Birkhauser, Boston. MA, 2004. 1 1. Introduction The first half of the twentieth century saw some remarkable developments in analytic probability theory. Wiener constructed a rigorous mathematical model of Brownian motion. Kolmogorov discovered that the transition probabilities of a diffusion process define a fundamental solution to the associated heat equation. Itˆo developed a stochas- tic calculus which made it possible to represent a diffusion with a given (infinitesimal) generator as the solution of a stochastic differential equation. These developments cre- ated a link between the fields of partial differential equations and stochastic analysis whereby results in the former area could be used to prove results in the latter. d More specifically, let X0,...,Xn denote a collection of smooth vector fields on R , regarded also as first-order differential operators, and define the second-order differen- tial operator n 1 L ≡ X2 + X . (1.1) 2 i 0 i=1 Consider also the Stratonovich stochastic differential equation (sde) n dξt = Xi(ξt) ◦ dwi + X0(ξt)dt (1.2) i=1 where w =(w1,...,wn)isann−dimensional standard Wiener process. Then the solution ξ to (1.2) is a time-homogeneous Markov process with generator L, whose transition probabilities p(t, x, dy) satisfy the following PDE (known as the Kolmogorov forward equation) in the weak sense ∂p = L∗p. ∂t y A differential operator G is said to be hypoelliptic if, whenever Gu is smooth for a distribution u defined on some open subset of the domain of G, then u is smooth. -
Informal Introduction to Stochastic Calculus 1
MATH 425 PART V: INFORMAL INTRODUCTION TO STOCHASTIC CALCULUS G. BERKOLAIKO 1. Motivation: how to model price paths Differential equations have long been an efficient tool to model evolution of various mechanical systems. At the start of 20th century, however, science started to tackle modeling of systems driven by probabilistic effects, such as stock market (the work of Bachelier) or motion of small particles suspended in liquid (the work of Einstein and Smoluchowski). For us, the motivating questions are: Is there an equation describing the evolution of a stock price? And, perhaps more applicably, how can we simulate numerically a seemingly random path taken by the stock price? Example 1.1. We have seen in previous sections that we can model the distribution of stock prices at time t as p 2 St = S0 exp (µ − σ =2)t + σ tY ; (1.1) where Y is standard normal random variable. So perhaps, for every time t we can generate a standard normal variable, substitute it into equation (1.1) and plot the resulting St as a function of t? The result is shown in Figure 1 and it definitely does not look like a price path of a stock. The underlying reason is that we used equation (1.1) to simulate prices at different time points independently. However, if t1 is close to t2, the prices cannot be completely independent. It is the change in price that we assumed (in “Efficient Market Hypothesis") to be independent of the previous price changes. 2. Wiener process Definition 2.1. Wiener process (or Brownian motion) is a random function of t, denoted Wt = Wt(!) (where ! is the underlying random event) such that (a) Wt is a.s. -
Lecture Notes on Stochastic Calculus (Part II)
Lecture Notes on Stochastic Calculus (Part II) Fabrizio Gelsomino, Olivier L´ev^eque,EPFL July 21, 2009 Contents 1 Stochastic integrals 3 1.1 Ito's integral with respect to the standard Brownian motion . 3 1.2 Wiener's integral . 4 1.3 Ito's integral with respect to a martingale . 4 2 Ito-Doeblin's formula(s) 7 2.1 First formulations . 7 2.2 Generalizations . 7 2.3 Continuous semi-martingales . 8 2.4 Integration by parts formula . 9 2.5 Back to Fisk-Stratonoviˇc'sintegral . 10 3 Stochastic differential equations (SDE's) 11 3.1 Reminder on ordinary differential equations (ODE's) . 11 3.2 Time-homogeneous SDE's . 11 3.3 Time-inhomogeneous SDE's . 13 3.4 Weak solutions . 14 4 Change of probability measure 15 4.1 Exponential martingale . 15 4.2 Change of probability measure . 15 4.3 Martingales under P and martingales under PeT ........................ 16 4.4 Girsanov's theorem . 17 4.5 First application to SDE's . 18 4.6 Second application to SDE's . 19 4.7 A particular case: the Black-Scholes model . 20 4.8 Application : pricing of a European call option (Black-Scholes formula) . 21 1 5 Relation between SDE's and PDE's 23 5.1 Forward PDE . 23 5.2 Backward PDE . 24 5.3 Generator of a diffusion . 26 5.4 Markov property . 27 5.5 Application: option pricing and hedging . 28 6 Multidimensional processes 30 6.1 Multidimensional Ito-Doeblin's formula . 30 6.2 Multidimensional SDE's . 31 6.3 Drift vector, diffusion matrix and weak solution . -
An Intuitive Introduction to Fractional and Rough Volatilities
mathematics Review An Intuitive Introduction to Fractional and Rough Volatilities Elisa Alòs 1,* and Jorge A. León 2 1 Department d’Economia i Empresa, Universitat Pompeu Fabra, and Barcelona GSE c/Ramon Trias Fargas, 25-27, 08005 Barcelona, Spain 2 Departamento de Control Automático, CINVESTAV-IPN, Apartado Postal 14-740, 07000 México City, Mexico; [email protected] * Correspondence: [email protected] Abstract: Here, we review some results of fractional volatility models, where the volatility is driven by fractional Brownian motion (fBm). In these models, the future average volatility is not a process adapted to the underlying filtration, and fBm is not a semimartingale in general. So, we cannot use the classical Itô’s calculus to explain how the memory properties of fBm allow us to describe some empirical findings of the implied volatility surface through Hull and White type formulas. Thus, Malliavin calculus provides a natural approach to deal with the implied volatility without assuming any particular structure of the volatility. The aim of this paper is to provides the basic tools of Malliavin calculus for the study of fractional volatility models. That is, we explain how the long and short memory of fBm improves the description of the implied volatility. In particular, we consider in detail a model that combines the long and short memory properties of fBm as an example of the approach introduced in this paper. The theoretical results are tested with numerical experiments. Keywords: derivative operator in the Malliavin calculus sense; fractional Brownian motion; future average volatility; Hull and White formula; Itô’s formula; Skorohod integral; stochastic volatility models; implied volatility; skews and smiles; rough volatility Citation: Alòs, E.; León, J.A. -
Introduction to Stochastic Calculus
Introduction Conditional Expectation Martingales Brownian motion Stochastic integral Ito formula Introduction to Stochastic Calculus Rajeeva L. Karandikar Director Chennai Mathematical Institute [email protected] [email protected] Rajeeva L. Karandikar Director, Chennai Mathematical Institute Introduction to Stochastic Calculus - 1 Introduction Conditional Expectation Martingales Brownian motion Stochastic integral Ito formula A Game Consider a gambling house. A fair coin is going to be tossed twice. A gambler has a choice of betting at two games: (i) A bet of Rs 1000 on first game yields Rs 1600 if the first toss results in a Head (H) and yields Rs 100 if the first toss results in Tail (T). (ii) A bet of Rs 1000 on the second game yields Rs 1800, Rs 300, Rs 50 and Rs 1450 if the two toss outcomes are HH,HT,TH,TT respectively. Rajeeva L. Karandikar Director, Chennai Mathematical Institute Introduction to Stochastic Calculus - 2 Introduction Conditional Expectation Martingales Brownian motion Stochastic integral Ito formula Rajeeva L. Karandikar Director, Chennai Mathematical Institute Introduction to Stochastic Calculus - 3 Introduction Conditional Expectation Martingales Brownian motion Stochastic integral Ito formula A Game...... Now it can be seen that the expected return from Game 1 is Rs 850 while from Game 2 is Rs 900 and so on the whole, the gambling house is set to make money if it can get large number of players to play. The novelty of the game (designed by an expert) was lost after some time and the casino owner decided to make it more interesting: he allowed people to bet without deciding if it is for Game 1 or Game 2 and they could decide to stop or continue after first toss. -
Stochastic Calculus of Variations for Stochastic Partial Differential Equations
JOURNAL OF FUNCTIONAL ANALYSIS 79, 288-331 (1988) Stochastic Calculus of Variations for Stochastic Partial Differential Equations DANIEL OCONE* Mathematics Department, Rutgers Universiiy, New Brunswick, New Jersey 08903 Communicated by Paul Malliavin Receved December 1986 This paper develops the stochastic calculus of variations for Hilbert space-valued solutions to stochastic evolution equations whose operators satisfy a coercivity con- dition. An application is made to the solutions of a class of stochastic pde’s which includes the Zakai equation of nonlinear filtering. In particular, a Lie algebraic criterion is presented that implies that all finite-dimensional projections of the solution define random variables which admit a density. This criterion generalizes hypoelhpticity-type conditions for existence and regularity of densities for Iinite- dimensional stochastic differential equations. 0 1988 Academic Press, Inc. 1. INTRODUCTION The stochastic calculus of variation is a calculus for functionals on Wiener space based on a concept of differentiation particularly suited to the invariance properties of Wiener measure. Wiener measure on the space of continuous @-valued paths is quasi-invariant with respect to translation by a path y iff y is absolutely continuous with a square-integrable derivative. Hence, the proper definition of the derivative of a Wiener functional considers variation only in directions of such y. Using this derivative and its higher-order iterates, one can develop Wiener functional Sobolev spaces, which are analogous in most respects to Sobolev spaces of functions over R”, and these spaces provide the right context for discussing “smoothness” and regularity of Wiener functionals. In particular, functionals defined by solving stochastic differential equations driven by a Wiener process are smooth in the stochastic calculus of variations sense; they are not generally smooth in a Frechet sense, which ignores the struc- ture of Wiener measure.