Classifying the Phase Transition Threshold for Ackermannian Functions
Total Page:16
File Type:pdf, Size:1020Kb
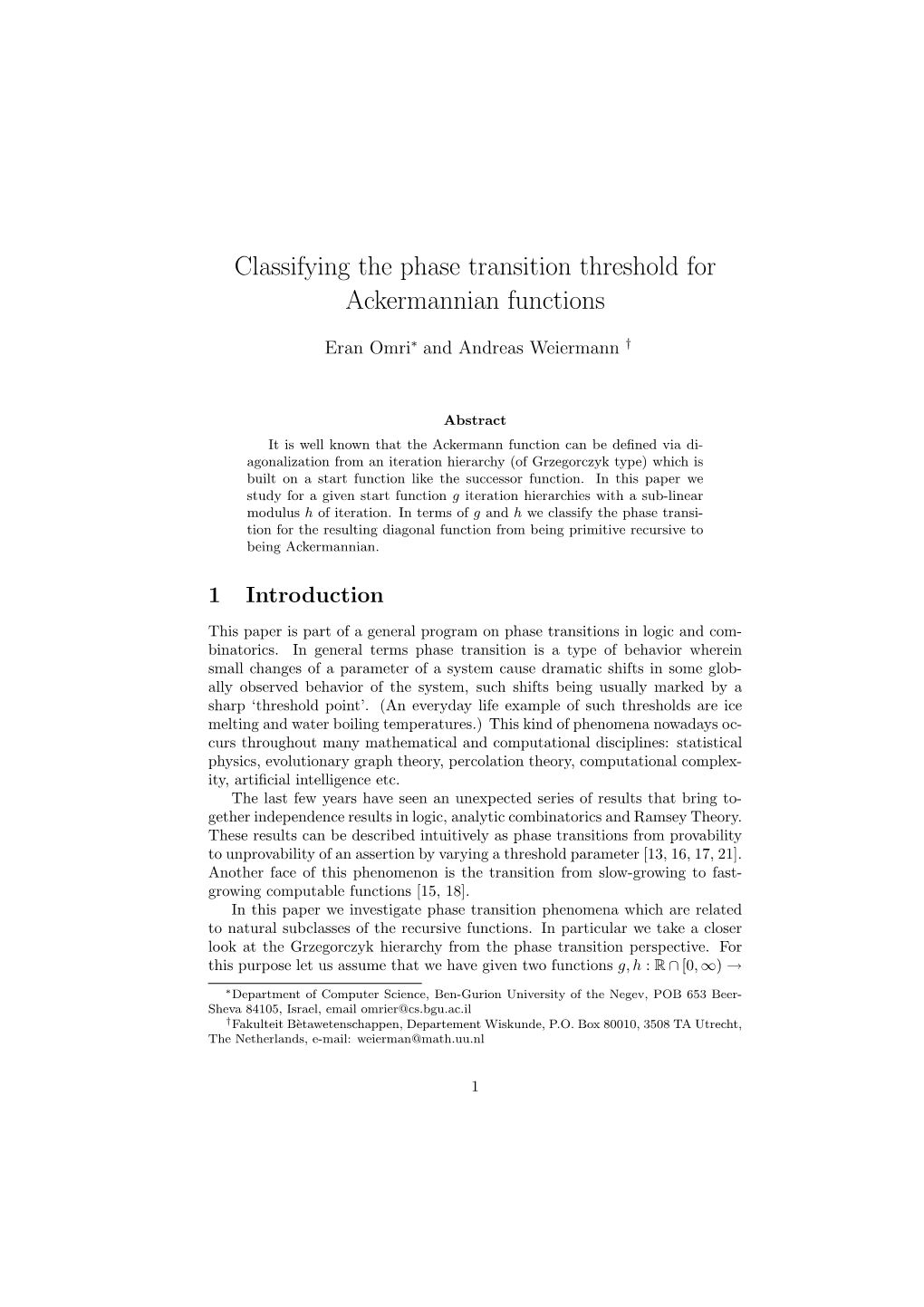
Load more
Recommended publications
-
Slides: Exponential Growth and Decay
Exponential Growth Many quantities grow or decay at a rate proportional to their size. I For example a colony of bacteria may double every hour. I If the size of the colony after t hours is given by y(t), then we can express this information in mathematical language in the form of an equation: dy=dt = 2y: A quantity y that grows or decays at a rate proportional to its size fits in an equation of the form dy = ky: dt I This is a special example of a differential equation because it gives a relationship between a function and one or more of its derivatives. I If k < 0, the above equation is called the law of natural decay and if k > 0, the equation is called the law of natural growth. I A solution to a differential equation is a function y which satisfies the equation. Annette Pilkington Exponential Growth dy(t) Solutions to the Differential Equation dt = ky(t) It is not difficult to see that y(t) = ekt is one solution to the differential dy(t) equation dt = ky(t). I as with antiderivatives, the above differential equation has many solutions. I In fact any function of the form y(t) = Cekt is a solution for any constant C. I We will prove later that every solution to the differential equation above has the form y(t) = Cekt . I Setting t = 0, we get The only solutions to the differential equation dy=dt = ky are the exponential functions y(t) = y(0)ekt Annette Pilkington Exponential Growth dy(t) Solutions to the Differential Equation dt = 2y(t) Here is a picture of three solutions to the differential equation dy=dt = 2y, each with a different value y(0). -
The Exponential Constant E
The exponential constant e mc-bus-expconstant-2009-1 Introduction The letter e is used in many mathematical calculations to stand for a particular number known as the exponential constant. This leaflet provides information about this important constant, and the related exponential function. The exponential constant The exponential constant is an important mathematical constant and is given the symbol e. Its value is approximately 2.718. It has been found that this value occurs so frequently when mathematics is used to model physical and economic phenomena that it is convenient to write simply e. It is often necessary to work out powers of this constant, such as e2, e3 and so on. Your scientific calculator will be programmed to do this already. You should check that you can use your calculator to do this. Look for a button marked ex, and check that e2 =7.389, and e3 = 20.086 In both cases we have quoted the answer to three decimal places although your calculator will give a more accurate answer than this. You should also check that you can evaluate negative and fractional powers of e such as e1/2 =1.649 and e−2 =0.135 The exponential function If we write y = ex we can calculate the value of y as we vary x. Values obtained in this way can be placed in a table. For example: x −3 −2 −1 01 2 3 y = ex 0.050 0.135 0.368 1 2.718 7.389 20.086 This is a table of values of the exponential function ex. -
Asynchronous Exponential Growth of Semigroups of Nonlinear Operators
View metadata, citation and similar papers at core.ac.uk brought to you by CORE provided by Elsevier - Publisher Connector JOURNAL OF MATHEMATICAL ANALYSIS AND APPLICATIONS 167, 443467 (1992) Asynchronous Exponential Growth of Semigroups of Nonlinear Operators M GYLLENBERG Lulea University of Technology, Department of Applied Mathematics, S-95187, Lulea, Sweden AND G. F. WEBB* Vanderbilt University, Department of Mathematics, Nashville, Tennessee 37235 Submitted by Ky Fan Received August 9, 1990 The property of asynchronous exponential growth is analyzed for the abstract nonlinear differential equation i’(f) = AZ(~) + F(z(t)), t 2 0, z(0) =x E X, where A is the infinitesimal generator of a semigroup of linear operators in the Banach space X and F is a nonlinear operator in X. Asynchronous exponential growth means that the nonlinear semigroup S(t), I B 0 associated with this problem has the property that there exists i, > 0 and a nonlinear operator Q in X such that the range of Q lies in a one-dimensional subspace of X and lim,,, em”‘S(r)x= Qx for all XE X. It is proved that if the linear semigroup generated by A has asynchronous exponential growth and F satisfies \/F(x)11 < f( llxll) ilxll, where f: [w, --t Iw + and 1” (f(rYr) dr < ~0, then the nonlinear semigroup S(t), t >O has asynchronous exponential growth. The method of proof is a linearization about infinity. Examples from structured population dynamics are given to illustrate the results. 0 1992 Academic Press. Inc. INTRODUCTION The concept of asynchronous exponential growth arises from linear models of cell population dynamics. -
6.4 Exponential Growth and Decay
6.4 Exponential Growth and Decay EEssentialssential QQuestionuestion What are some of the characteristics of exponential growth and exponential decay functions? Predicting a Future Event Work with a partner. It is estimated, that in 1782, there were about 100,000 nesting pairs of bald eagles in the United States. By the 1960s, this number had dropped to about 500 nesting pairs. In 1967, the bald eagle was declared an endangered species in the United States. With protection, the nesting pair population began to increase. Finally, in 2007, the bald eagle was removed from the list of endangered and threatened species. Describe the pattern shown in the graph. Is it exponential growth? Assume the MODELING WITH pattern continues. When will the population return to that of the late 1700s? MATHEMATICS Explain your reasoning. To be profi cient in math, Bald Eagle Nesting Pairs in Lower 48 States you need to apply the y mathematics you know to 9789 solve problems arising in 10,000 everyday life. 8000 6846 6000 5094 4000 3399 1875 2000 1188 Number of nesting pairs 0 1978 1982 1986 1990 1994 1998 2002 2006 x Year Describing a Decay Pattern Work with a partner. A forensic pathologist was called to estimate the time of death of a person. At midnight, the body temperature was 80.5°F and the room temperature was a constant 60°F. One hour later, the body temperature was 78.5°F. a. By what percent did the difference between the body temperature and the room temperature drop during the hour? b. Assume that the original body temperature was 98.6°F. -
Control Structures in Programs and Computational Complexity
Control Structures in Programs and Computational Complexity Habilitationsschrift zur Erlangung des akademischen Grades Dr. rer. nat. habil. vorgelegt der Fakultat¨ fur¨ Informatik und Automatisierung an der Technischen Universitat¨ Ilmenau von Dr. rer. nat. Karl–Heinz Niggl 26. November 2001 2 Referees: Prof. Dr. Klaus Ambos-Spies Prof. Dr.(USA) Martin Dietzfelbinger Prof. Dr. Neil Jones Day of Defence: 2nd May 2002 In the present version, misprints are corrected in accordance with the referee’s comments, and some proofs (Theorem 3.5.5 and Lemma 4.5.7) are partly rearranged or streamlined. Abstract This thesis is concerned with analysing the impact of nesting (restricted) control structures in programs, such as primitive recursion or loop statements, on the running time or computational complexity. The method obtained gives insight as to why some nesting of control structures may cause a blow up in computational complexity, while others do not. The method is demonstrated for three types of programming languages. Programs of the first type are given as lambda terms over ground-type variables enriched with constants for primitive recursion or recursion on notation. A second is concerned with ordinary loop pro- grams and stack programs, that is, loop programs with stacks over an arbitrary but fixed alphabet, supporting a suitable loop concept over stacks. Programs of the third type are given as terms in the simply typed lambda calculus enriched with constants for recursion on notation in all finite types. As for the first kind of programs, each program t is uniformly assigned a measure µ(t), being a natural number computable from the syntax of t. -
Computational Complexity: a Modern Approach PDF Book
COMPUTATIONAL COMPLEXITY: A MODERN APPROACH PDF, EPUB, EBOOK Sanjeev Arora,Boaz Barak | 594 pages | 16 Jun 2009 | CAMBRIDGE UNIVERSITY PRESS | 9780521424264 | English | Cambridge, United Kingdom Computational Complexity: A Modern Approach PDF Book Miller; J. The polynomial hierarchy and alternations; 6. This is a very comprehensive and detailed book on computational complexity. Circuit lower bounds; Examples and solved exercises accompany key definitions. Computational complexity: A conceptual perspective. Redirected from Computational complexities. Refresh and try again. Brand new Book. The theory formalizes this intuition, by introducing mathematical models of computation to study these problems and quantifying their computational complexity , i. In other words, one considers that the computation is done simultaneously on as many identical processors as needed, and the non-deterministic computation time is the time spent by the first processor that finishes the computation. Foundations of Cryptography by Oded Goldreich - Cambridge University Press The book gives the mathematical underpinnings for cryptography; this includes one-way functions, pseudorandom generators, and zero-knowledge proofs. If one knows an upper bound on the size of the binary representation of the numbers that occur during a computation, the time complexity is generally the product of the arithmetic complexity by a constant factor. Jason rated it it was amazing Aug 28, Seller Inventory bc0bebcaa63d3c. Lewis Cawthorne rated it really liked it Dec 23, Polynomial hierarchy Exponential hierarchy Grzegorczyk hierarchy Arithmetical hierarchy Boolean hierarchy. Convert currency. Convert currency. Familiarity with discrete mathematics and probability will be assumed. The formal language associated with this decision problem is then the set of all connected graphs — to obtain a precise definition of this language, one has to decide how graphs are encoded as binary strings. -
Large Numbers from Wikipedia, the Free Encyclopedia
Large numbers From Wikipedia, the free encyclopedia This article is about large numbers in the sense of numbers that are significantly larger than those ordinarily used in everyday life, for instance in simple counting or in monetary transactions. The term typically refers to large positive integers, or more generally, large positive real numbers, but it may also be used in other contexts. Very large numbers often occur in fields such as mathematics, cosmology, cryptography, and statistical mechanics. Sometimes people refer to numbers as being "astronomically large". However, it is easy to mathematically define numbers that are much larger even than those used in astronomy. Contents 1 Using scientific notation to handle large and small numbers 2 Large numbers in the everyday world 3 Astronomically large numbers 4 Computers and computational complexity 5 Examples 6 Systematically creating ever faster increasing sequences 7 Standardized system of writing very large numbers 7.1 Examples of numbers in numerical order 8 Comparison of base values 9 Accuracy 9.1 Accuracy for very large numbers 9.2 Approximate arithmetic for very large numbers 10 Large numbers in some noncomputable sequences 11 Infinite numbers 12 Notations 13 See also 14 Notes and references Using scientific notation to handle large and small numbers See also: scientific notation, logarithmic scale and orders of magnitude Scientific notation was created to handle the wide range of values that occur in scientific study. 1.0 × 109, for example, means one billion, a 1 followed by nine zeros: 1 000 000 000, and 1.0 × 10−9 means one billionth, or 0.000 000 001. -
Lesson 6: Exponential Growth—U.S. Population and World Population
NYS COMMON CORE MATHEMATICS CURRICULUM Lesson 6 M3 ALGEBRA I Lesson 6: Exponential Growth—U.S. Population and World Population Student Outcomes . Students compare linear and exponential models of population growth. Classwork Mathematical Modeling Exercise 1 (8 minutes) Give students time to review the two graphs. Ask them to compare the two graphs and make conjectures about the rates of change. As students share the conjectures with the class, respond without judgment as to accuracy. Simply suggest that the class investigate further and see which conjectures are correct. Mathematical Modeling Exercise 1 Callie and Joe are examining the population data in the graphs below for a history report. Their comments are as follows: Callie: It looks like the U.S. population grew the same amount as the world population, but that can’t be right, can it? Joe: Well, I don’t think they grew by the same amount, but they sure grew at about the same rate. Look at the slopes. World Population U.S. Population 7000 300 6000 250 5000 200 4000 150 3000 2000 100 1000 50 Population (in millions) (in Population millions) (in Population 0 0 1950 1960 1970 1980 1990 2000 1950 1960 1970 1980 1990 2000 Year Year Be aware that students frequently ignore scale on graphs and may offer incorrect observations as a result. If students respond incorrectly to the prompts, direct partners or groups to discuss why the response or observation is incorrect. Lesson 6: Exponential Growth—U.S. Population and World Population 61 This work is licensed under a This work is derived from Eureka Math ™ and licensed by Great Minds. -
Exponential Growth Series and Benford's Law ABSTRACT
Exponential Growth Series and Benford’s Law ABSTRACT Exponential growth occurs when the growth rate of a given quantity is proportional to the quantity's current value. Surprisingly, when exponential growth data is plotted as a simple histogram disregarding the time dimension, a remarkable fit to the positively skewed k/x distribution is found, where the small is numerous and the big is rare, and with a long tail falling to the right of the histogram. Such quantitative preference for the small has a corresponding digital preference known as Benford’s Law which predicts that the first significant digit on the left-most side of numbers in typical real-life data is proportioned between all possible 1 to 9 digits approximately as in LOG(1 + 1/digit), so that low digits occur much more frequently than high digits in the first place. Exponential growth series are nearly perfectly Benford given that plenty of elements are considered and that (for low growth) order of magnitude is approximately an integral value. An additional constraint is that the logarithm of the growth factor must be an irrational number. Since the irrationals vastly outnumber the rationals, on the face of it, this constraint seems to constitute the explanation of why almost all growth series are Benford, yet, in reality this is all too simplistic, and the real and more complex explanation is provided in this article. Empirical examinations of close to a half a million growth series via computerized programs almost perfectly match the prediction of the theoretical study on rational versus irrational occurrences, thus in a sense confirming both, the empirical work as well as the theoretical study. -
A Function Which Models Exponential Growth Or Decay Can Be Written in Either the Form
A function which models exponential growth or decay can be written in either the form t kt P (t) = P0b or P (t) = P0e : In either form, P0 represents the initial amount. kt The form P (t) = P0e is sometimes called the continuous exponential model. The constant k is called t the continuous growth (or decay) rate. In the form P (t) = P0b , the growth rate is r = b − 1. The constant b is sometimes called the growth factor. The growth rate and growth factor are not the same. kt It is a simple matter to change from one model to the other. If we are given P (t) = P0e , and want t kt kt to write it in the form P (t) = P0b , all that is needed is to note that P (t) = P0e = P0 e , so if we let k t kt b = e , we have the desired form. If we want to switch from P (t) = P0b to P (t) = P0e , it again is just a matter of noting that b = ek, and solving for k in this case. That is, k = ln b. Template for solving problems: Given a rate of growth or decay r, • If r is given as a constant rate of change (some fixed quantity per unit), then the equation is linear (i.e. P = P0 + rt) • If r is given as a percentage increase or decrease, then the equation is exponential t { if the rate of growth is r, then use the model P (t) = P0b , where b = 1 + r t { if the rate of decay is r, then use the model P (t) = P0b , where b = 1 − r { if the continuous rate of growth is k, then use the model P (t) = P ekt, with k positive { if the continuous rate of decay is k, then use the model P (t) = P ekt, with k negative kt Thus, the form P (t) = P0e should be used if the rate of growth or decay is stated as a continuous rate. -
Population Project [Maggie Hughes]
Performance Based Learning and Assessment Task Population Project I. ASSESSSMENT TASK OVERVIEW & PURPOSE: In the Population Project, students will deepen their understanding of exponential growth and decay by researching and analyzing a country’s population growth or decay every decade over a 100-year period. With this information, students will model the population graphically, make predictions about the country’s current and future population, create a poster or brochure describing the country and its population trends, present their findings, and take a brief quiz on a different country’s population. II. UNIT AUTHOR: Maggie Hughes, Hidden Valley High School, Roanoke County Public Schools III. COURSE: Algebra II IV. CONTENT STRAND: Functions V. OBJECTIVES: • The student will be able to collect, organize, analyze, and present population data using their knowledge of exponential growth and decay. • The student will be able to model population by creating exponential equations and graphs. • The student will be able to use exponential equations and graphs to make predictions. • The student will be able to understand and describe exponential growth and decay. • The student will be able to correctly use and explain the A = Pert formula. • The student will be able to make predictions using the information they’ve found and developed. • The student will be able to analyze the process he/she uses to find their answers since their work is being recorded. I. REFERENCE/RESOURCE MATERIALS: • Attached Population Project handout (1 per student) (page 7) • Attached Population Quiz (1 per student) (page 8) • Attached Assessment & Rubric Sheets (pages 9 & 10) • Poster board or brochure paper (1 per group) • Laptops • Library access for books on various countries • Notes on exponential growth and decay • Classroom set of graphing calculators II. -
Chapter 4 Complexity As a Discourse on School Mathematics Reform
Chapter 4 Complexity as a Discourse on School Mathematics Reform Brent Davis Abstract This writing begins with a brief introduction of complexity thinking, coupled to a survey of some of the disparate ways that it has been taken up within mathematics education. That review is embedded in a report on a teaching experi- ment that was developed around the topic of exponentiation, and that report is in turn used to highlight three elements that may be critical to school mathematics reform. Firstly, complexity is viewed in curricular terms for how it might affect the content of school mathematics. Secondly, complexity is presented as a discourse on learning, which might influence how topics and experiences are formatted for stu- dents. Thirdly, complexity is interpreted as a source of pragmatic advice for those tasked with working in the complex space of teaching mathematics. Keywords Complexity thinking • School mathematics • Mathematics curriculum One of the most common criticisms of contemporary school mathematics is that its contents are out of step with the times. The curriculum, it is argued, comprises many facts and skills that have become all but useless, while it ignores a host of concepts and competencies that have emerged as indispensible. Often the problem is attrib- uted to a system that is prone to accumulation and that cannot jettison its history. Programs of study have thus become not-always-coherent mixes of topics drawn from ancient traditions, skills imagined necessary for a citizen of the modern (read: industry-based, consumption-driven) world, necessary preparations for postsecond- ary study, and ragtag collections of other topics that were seen to add some prag- matic value at one time or another over the past few centuries – all carried along by a momentum of habit and familiarity.