Does Losses Loom Larger Than Gains in Decisions from Experience
Total Page:16
File Type:pdf, Size:1020Kb
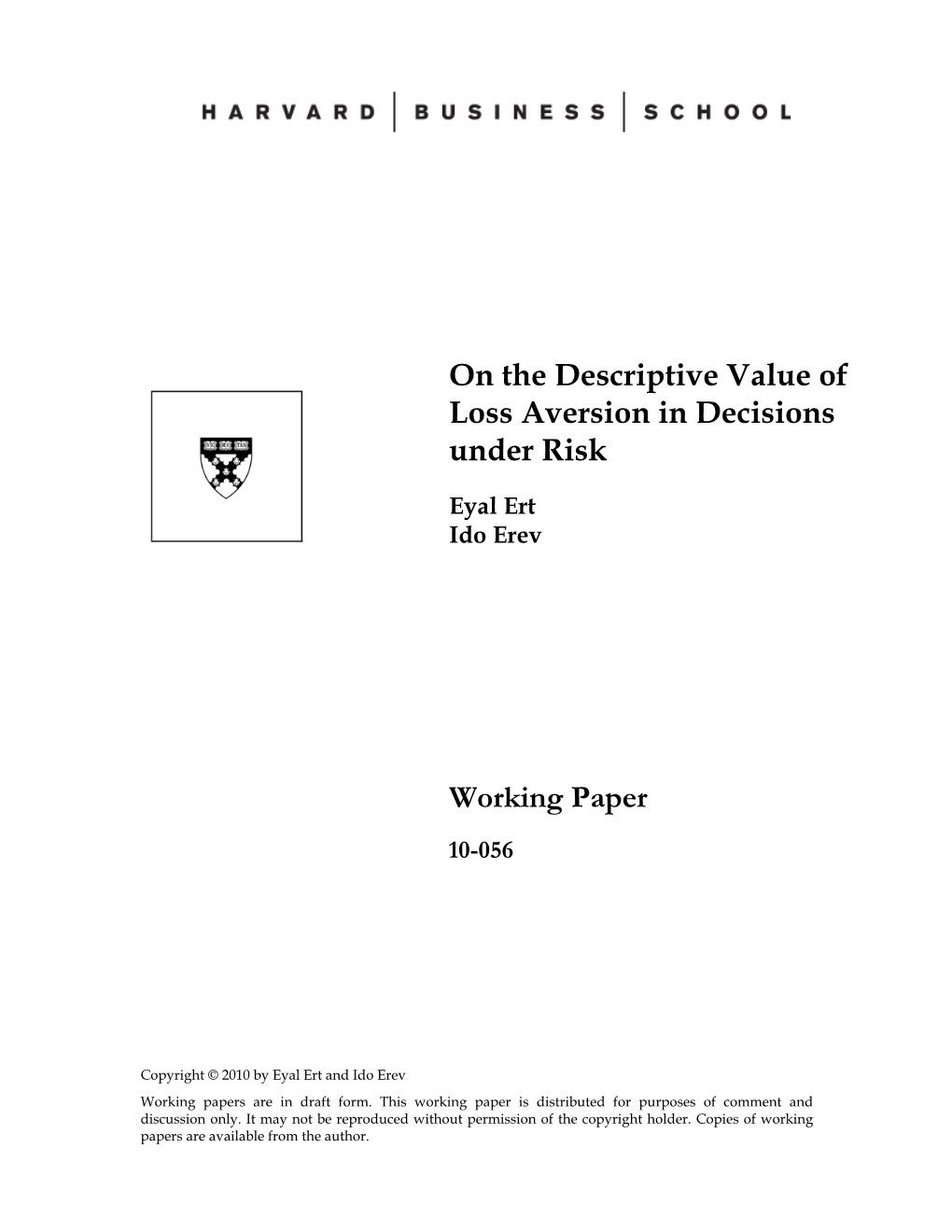
Load more
Recommended publications
-
Punishment in the Cingulate Cortex Segregated and Integrated Coding
Zurich Open Repository and Archive University of Zurich Main Library Strickhofstrasse 39 CH-8057 Zurich www.zora.uzh.ch Year: 2009 Segregated and integrated coding of reward and punishment in the cingulate cortex Fujiwara, J ; Tobler, Philippe N ; Taira, M ; Iijima, T ; Tsutsui, K I Abstract: Reward and punishment have opposite affective value but are both processed by the cingulate cortex. However, it is unclear whether the positive and negative affective values of monetary reward and punishment are processed by separate or common subregions of the cingulate cortex. We performed a functional magnetic resonance imaging study using a free-choice task and compared cingulate activations for different levels of monetary gain and loss. Gain-specific activation (increasing activation for increasing gain, but no activation change in relation to loss) occurred mainly in the anterior part of the anterior cingulate and in the posterior cingulate cortex. Conversely, loss-specific activation (increasing activation for increasing loss, but no activation change in relation to gain) occurred between these areas, in the middle and posterior part of the anterior cingulate. Integrated coding of gain and loss (increasing activation throughout the full range, from biggest loss to biggest gain) occurred in the dorsal part of the anterior cingulate, at the border with the medial prefrontal cortex. Finally, unspecific activation increases to both gains and losses (increasing activation to increasing gains and increasing losses, possibly reflecting attention) occurred in dorsal and middle regions of the cingulate cortex. Together, these results suggest separate and common coding of monetary reward and punishment in distinct subregions of the cingulate cortex. -
Testing Loss Aversion in an Adventure Game
See discussions, stats, and author profiles for this publication at: https://www.researchgate.net/publication/345198400 Risking Treasure: Testing Loss Aversion in an Adventure Game Conference Paper · November 2020 DOI: 10.1145/3410404.3414250 CITATIONS READS 0 89 6 authors, including: Natanael Bandeira Romão Tomé Madison Klarkowski University of Saskatchewan University of Saskatchewan 1 PUBLICATION 0 CITATIONS 17 PUBLICATIONS 99 CITATIONS SEE PROFILE SEE PROFILE Carl Gutwin Cody Phillips University of Saskatchewan University of Saskatchewan 332 PUBLICATIONS 13,451 CITATIONS 13 PUBLICATIONS 101 CITATIONS SEE PROFILE SEE PROFILE Some of the authors of this publication are also working on these related projects: HandMark Menus: Use Hands as Landmarks View project Personalized and Adaptive Persuasive Technologies for behaviour change View project All content following this page was uploaded by Natanael Bandeira Romão Tomé on 02 November 2020. The user has requested enhancement of the downloaded file. Risking Treasure: Testing Loss Aversion in an Adventure Game Natanael Bandeira Romão Madison Klarkowski Carl Gutwin Tomé Department of Computer Science Department of Computer Science Department of Computer Science University of Saskatchewan University of Saskatchewan University of Saskatchewan Saskatoon, Canada Saskatoon, Canada Saskatoon, Canada [email protected] [email protected] [email protected] Cody Phillips Regan L. Mandryk Andy Cockburn Department of Computer Science Department of Computer Science Department of Computer Science University of Saskatchewan University of Saskatchewan University of Canterbury Saskatoon, Canada Saskatoon, Canada Christchurch, New Zealand [email protected] [email protected] [email protected] ABSTRACT Symposium on Computer-Human Interaction in Play (CHI PLAY ’20), Novem- Loss aversion is a cognitive bias in which the negative feelings ber 2–4, 2020, Virtual Event, Canada. -
Cognitive Bias in Emissions Trading
sustainability Article Cognitive Bias in Emissions Trading Jae-Do Song 1 and Young-Hwan Ahn 2,* 1 College of Business Administration, Chonnam National University, Gwangju 61186, Korea; [email protected] 2 Korea Energy Economics Institute, 405-11 Jongga-ro, Jung-gu, Ulsan 44543, Korea * Correspondence: [email protected]; Tel.: +82-52-714-2175; Fax: +82-52-714-2026 Received: 8 February 2019; Accepted: 27 February 2019; Published: 5 March 2019 Abstract: This study investigates whether cognitive biases such as the endowment effect and status quo bias occur in emissions trading. Such cognitive biases can serve as a barrier to trade. This study’s survey-based experiments, which include hypothetical emissions trading scenarios, show that the endowment effect does occur in emissions trading. The status quo bias occurs in only one of the three experiments. This study also investigates whether accumulation of experience can reduce cognitive bias as discovered preference hypothesis expects. The results indicate that practitioners who are supposed to have more experience show no evidence of having less cognitive bias. Contrary to the conventional expectation, the practitioners show significantly higher level of endowment effect than students and only the practitioners show a significant status quo bias. A consignment auction situation, which is used in California’s cap-and-trade program, is also tested; no significant difference between general permission trading and consignment auctions is found. Keywords: emissions trading; cognitive bias; consignment auction; climate policy 1. Introduction Emissions trading allows entities to achieve emission reduction targets in a cost-effective way through buying and selling emission allowances in emissions trading markets [1,2]. -
Reduced Loss Aversion in Pathological Gambling and Alcohol Dependence Is Associated with Differential Alterations in Amygdala An
www.nature.com/scientificreports OPEN Reduced loss aversion in pathological gambling and alcohol dependence is associated with Received: 9 March 2017 Accepted: 13 November 2017 diferential alterations in amygdala Published: xx xx xxxx and prefrontal functioning Alexander Genauck 1,2, Saskia Quester1,3, Torsten Wüstenberg1, Chantal Mörsen1, Andreas Heinz1 & Nina Romanczuk-Seiferth 1 Diagnostic criteria for pathological gambling and alcohol dependence (AD) include repeated addictive behavior despite severe negative consequences. However, the concept of loss aversion (LA) as a facet of value-based decision making has not yet been used to directly compare these disorders. We hypothesized reduced LA in pathological gamblers (PG) and AD patients, correlation of LA with disorder severity, and reduced loss-related modulation of brain activity. 19 PG subjects, 15 AD patients and 17 healthy controls (HC) engaged in a LA task in a functional magnetic resonance imaging setting. Imaging analyses focused on neural gain and loss sensitivity in the meso-cortico-limbic network of the brain. Both PG and AD subjects showed reduced LA. AD subjects showed altered loss-related modulation of activity in lateral prefrontal regions. PG subjects showed indication of altered amygdala-prefrontal functional connectivity. Although we observed reduced LA in both a behavioral addiction and a substance-related disorder our neural fndings might challenge the notion of complete neuro-behavioral congruence of substance-use disorders and behavioral addictions. Value-based decisions are ubiquitous in every-day life. Tey can be anything from short-term and mundane (tea or cofee) to long-term and life changing (law or medical school). In all of these decisions we need to incorpo- rate magnitude, delay and probability of possible rewards and losses to compute subjective values of the availa- ble options1. -
COMPUTATIONAL BIASES in DECISION-MAKING Thesis By
COMPUTATIONAL BIASES IN DECISION-MAKING Thesis by Vanessa Janowski In Partial Fulfillment of the Requirements for the Degree of Doctor of Philosophy CALIFORNIA INSTITUTE OF TECHNOLOGY Pasadena, California 2012 (Defended May 9, 2012) ii 2012 Vanessa Janowski All Rights Reserved iii ACKNOWLEDGEMENTS I would like to thank everyone who has helped and supported me throughout my Ph.D. To start, I would like to thank my undergraduate mentors, Arturo Bris and Christos Cabolis, for introducing me to economic research and for their support and advice over the years. I would also like to thank my mother, Jadwiga Rogowska, for introducing me to neuroscience and functional magnetic resonance imaging (fMRI). They helped me to embark on the path to a Ph.D. I would like to thank Ralph Adolphs for helping to convince me to come to Caltech and taking an interest in my development as a graduate student. He showed me what a successful scientist is like and inspired me to pursue my research in a thoughtful, determined way. On a similar note, I would like to thank Colin Camerer for his mentorship in my first fMRI study and the always-stimulating discussions in lab meetings and in the classroom. He never fails to make me laugh and has inspired me to pursue better research. iv I would also like to thank Peter Bossaerts, Pietro Ortoleva and Kota Saito for their help and advice along the way, as well as for serving on my committee. I would like to thank my co-authors Antonio Rangel, Colin Camerer, Eric Johnson, Martijn Willemsen and Erik Madsen for their contributions. -
Overcoming the Loss Aversion Obstacle in Negotiation
\\jciprod01\productn\H\HNR\25-2\HNR202.txt unknown Seq: 1 30-SEP-20 12:42 Overcoming the Loss Aversion Obstacle in Negotiation Yair Livneh* The cognitive bias of loss aversion—the phenomenon that losses loom larger than gains—poses an obstacle in negotiation. Each party’s own concessions loom larger than those of the other party, which makes it difficult to reach an agreement by trading conces- sions. This Article suggests ways to overcome this obstacle. Two types of solutions are presented: substantive solutions, which fo- cus on features in an agreement that may counter loss aversion; and tactical solutions, which focus on presentation strategies to counter loss aversion. This Article also considers whether several well-known but undertheorized negotiation practices owe their effectiveness to their capacity to counter loss aversion bias. CONTENTS I. The Loss Aversion Cognitive Bias .................... 188 R A. The Status Quo Bias, Endowment Effect, and Offer-Asking Gap ................................. 189 R B. Loss Aversion in the Legal Field .................. 190 R II. The Loss Aversion Obstacle in Negotiation ........... 191 R III. Overcoming the Loss Aversion Obstacle .............. 192 R A. Substantive Aspects: Designing an Offer in Light of Loss Aversion ............................ 192 R 1. Making Effective Concessions: Decreasing an Adversary’s Losses Adds More Value than Increasing Their Gains .................. 193 R * Yair Livneh is an Israeli lawyer, veteran of the Israeli tech industry, former commercial litigator, corporate in-house lawyer, and a seasoned negotiator. Yair earned his LL.B. from Tel Aviv University (1995) and his LL.M. from the Hebrew University in Jerusalem (2003), clerked in the chamber of the honorable Justice E. -
Exploring the Nature of Loss Aversion
A Service of Leibniz-Informationszentrum econstor Wirtschaft Leibniz Information Centre Make Your Publications Visible. zbw for Economics Johnson, Eric J.; Gächter, Simon; Herrmann, Andreas Working Paper Exploring the nature of loss aversion IZA Discussion Papers, No. 2015 Provided in Cooperation with: IZA – Institute of Labor Economics Suggested Citation: Johnson, Eric J.; Gächter, Simon; Herrmann, Andreas (2006) : Exploring the nature of loss aversion, IZA Discussion Papers, No. 2015, Institute for the Study of Labor (IZA), Bonn This Version is available at: http://hdl.handle.net/10419/33324 Standard-Nutzungsbedingungen: Terms of use: Die Dokumente auf EconStor dürfen zu eigenen wissenschaftlichen Documents in EconStor may be saved and copied for your Zwecken und zum Privatgebrauch gespeichert und kopiert werden. personal and scholarly purposes. Sie dürfen die Dokumente nicht für öffentliche oder kommerzielle You are not to copy documents for public or commercial Zwecke vervielfältigen, öffentlich ausstellen, öffentlich zugänglich purposes, to exhibit the documents publicly, to make them machen, vertreiben oder anderweitig nutzen. publicly available on the internet, or to distribute or otherwise use the documents in public. Sofern die Verfasser die Dokumente unter Open-Content-Lizenzen (insbesondere CC-Lizenzen) zur Verfügung gestellt haben sollten, If the documents have been made available under an Open gelten abweichend von diesen Nutzungsbedingungen die in der dort Content Licence (especially Creative Commons Licences), you genannten Lizenz gewährten Nutzungsrechte. may exercise further usage rights as specified in the indicated licence. www.econstor.eu IZA DP No. 2015 Exploring the Nature of Loss Aversion Eric J. Johnson Simon Gächter Andreas Herrmann DISCUSSION PAPER SERIES DISCUSSION PAPER March 2006 Forschungsinstitut zur Zukunft der Arbeit Institute for the Study of Labor Exploring the Nature of Loss Aversion Eric J. -
Losses, Gains, and Brains: Neuroeconomics Can Help to Answer Open Questions About Loss Aversion
Available online at www.sciencedirect.com Journal of CONSUMER PSYCHOLOGY Journal of Consumer Psychology 21 (2011) 453–463 Losses, gains, and brains: Neuroeconomics can help to answer open questions about loss aversion Scott Rick Department of Marketing, Ross School of Business, University of Michigan, 701 Tappan Street, Ann Arbor, MI 48109, USA Received 19 February 2010; accepted 27 April 2010 Available online 7 June 2010 Abstract Much is understood about loss aversion (the tendency for losses to have greater hedonic impact than comparable gains), but open questions remain. First, there is debate about whether loss aversion is best understood as the byproduct of a single system within the brain that treats losses and gains asymmetrically or the interaction of separate deliberative and emotional systems. Second, some have questioned whether loss aversion alone is the best account for the endowment effect. Alternative accounts, based on the differential focus induced by buying versus selling, the order in which buyers and sellers consider positive and negative aspects of the good, the extent to which ownership induces liking, and the desire to avoid making a bad deal, have been proposed. Third, it is unclear whether losses are actually experienced more intensely than comparable gains, or whether people simply behave as if they were. Some have argued that loss aversion is nothing more than an affective forecasting error, while others have argued that there are many situations in which losses are actually more impactful than comparable gains. This review synthesizes the insights that behavioral researchers and neuroeconomists have contributed to each debate, and highlights potential avenues for future research. -
Behavioral and Neural Correlates of Loss Aversion and Risk Avoidance in Adolescents and Adults
Developmental Cognitive Neuroscience 3 (2013) 72–83 View metadata, citation and similar papers at core.ac.uk brought to you by CORE Contents lists available at SciVerse ScienceDirect provided by Elsevier - Publisher Connector Developmental Cognitive Neuroscience j ournal homepage: http://www.elsevier.com/locate/dcn Behavioral and neural correlates of loss aversion and risk avoidance in adolescents and adults a a,1 a,b,∗ Emily E. Barkley-Levenson , Linda Van Leijenhorst , Adriana Galván a Department of Psychology, University of California Los Angeles, 1285 Franz Hall, Box 951563, Los Angeles, CA 90095-1563, USA b Brain Research Institute, University of California Los Angeles, Box 951761, Los Angeles, CA 90095-1761, USA a r t i c l e i n f o a b s t r a c t Article history: Individuals are frequently faced with risky decisions involving the potential for both gain Received 1 June 2012 and loss. Exploring the role of both potential gains and potential losses in predicting risk Received in revised form 8 September 2012 taking is critical to understanding how adolescents and adults make the choice to engage Accepted 19 September 2012 in or avoid a real-life risk. This study aimed to examine the impact of potential losses as well as gains on adolescent decisions during risky choice in a laboratory task. Adolescent Keywords: (n = 18) and adult (n = 16) participants underwent functional magnetic resonance imaging Loss aversion Risk (fMRI) during a mixed gambles task, and completed questionnaires measuring real-world Decision-making risk-taking behaviors. While potential loss had a significantly greater effect on choice than Adolescence potential gain in both adolescents and adults and there were no behavioral group differ- Development ences on the task, adolescents recruited significantly more frontostriatal circuitry than fMRI adults when choosing to reject a gamble. -
The Psychological and Neural Basis of Loss Aversion
View metadata, citation and similar papers at core.ac.uk brought to you by CORE provided by UCL Discovery The Psychological and Neural Basis of Loss Aversion Peter Sokol-Hessnera,1, Robb B. Rutledgeb,c a Department of Psychology, University of Denver, Denver, CO 80208, USA b Wellcome Centre for Human Neuroimaging, University College London, London WC1N 3BG, United Kingdom c Max Planck University College London Centre for Computational Psychiatry and Ageing Research, London WC1B 5EH, United Kingdom ABSTRACT: (183 words) Loss aversion is a central element of Prospect Theory, the dominant theory of decision- making under uncertainty for the past four decades, and refers to the overweighting of potential losses relative to equivalent gains, a critical determinant of risky decision-making. Recent advances in affective and decision neuroscience have shed new light on the psychological and neurobiological mechanisms underlying loss aversion. Here, integrating across disparate literatures, from the level of neurotransmitters to subjective reports of emotion, we propose a novel neural and computational framework that links norepinephrine to loss aversion and identifies a distinct role for dopamine in risk taking for rewards. We also propose that loss aversion specifically relates to anticipated emotions and aspects of the immediate experience of realized gains and losses but not their long- term emotional consequences, highlighting an underappreciated temporal structure. Finally, we discuss challenges to loss aversion and the relevance of loss aversion to understanding psychiatric disorders. Refining models of loss aversion will have broad consequences for the science of decision-making, and for how we understand individual variation in economic preferences and psychological well-being across both healthy and psychiatric populations. -
Examining the Role of the Insular Cortex in Addiction- Relevant Behaviours
Examining the role of the insular cortex in addiction- relevant behaviours by Abhiram Pierre Pushparaj A thesis submitted in conformity with the requirements for the degree of Doctor of Philosophy Department of Pharmacology & Toxicology University of Toronto © Copyright by Abhiram Pierre Pushparaj 2016 Examining the role of the insular cortex in addiction-relevant behaviours Abhiram Pierre Pushparaj Doctor of Philosophy Department of Pharmacology University of Toronto 2016 Abstract INTRODUCTION: Recently recognized as a critical brain region underlying the neurocircuitry of addiction, the body of evidence supporting the role of the insular cortex across multiple aspects of addiction is growing rapidly. Understanding the complex role of the insular cortex is crucial to moving forward with the development of therapeutics targeting this brain region for substance-related and addictive disorders. OBJECTIVES: The current series of experiments were conducted to investigate the effects of modulating activity in two insular subregions, through the use of multiple modalities (chemical lesions, pharmacological inactivation or electrical stimulation), on behaviours relevant to addictive disorders, including drug self-administration, reinstatement, and decision-making. METHODS: In the first series of experiments, we investigated the effects of chemical lesions or transient pharmacological inactivation of two distinct subregions of the insular cortex, the rostral agranular (RAIC) vs. caudal granular (CGIC), on the acquisition or performance of choice behaviour in the Rat Gambling Task (rGT). In the next series of experiments, we examined the effects of pharmacological inactivation of the RAIC on nicotine self-administration under a fixed ratio-5 (FR-5) schedule of reinforcement and the reinstatement of nicotine-seeking induced by ii nicotine-associated cues. -
The Functional and Structural Neural Basis of Individual Differences in Loss Aversion
The Journal of Neuroscience, September 4, 2013 • 33(36):14307–14317 • 14307 Behavioral/Cognitive The Functional and Structural Neural Basis of Individual Differences in Loss Aversion Nicola Canessa,1,2,3,4,5 Chiara Crespi,1,2 Matteo Motterlini,1,2,5 Gabriel Baud-Bovy,1,6 Gabriele Chierchia,1,2 Giuseppe Pantaleo,1 Marco Tettamanti,2,7 and Stefano F. Cappa1,2,3,4,5 1Vita-Salute San Raffaele University, 20132 Milan, Italy, 2Center for Cognitive Neuroscience, Vita-Salute San Raffaele University, 20132 Milan, Italy, 3Division of Neuroscience, San Raffaele Scientific Institute, 20132 Milan, Italy, 4CERMAC, San Raffaele Scientific Institute, 20132 Milan, Italy, 5CRESA, Vita- Salute San Raffaele University, 20132 Milan, Italy, 6Robotics, Brain, and Cognitive Sciences Department, Istituto Italiano di Tecnologia, 16163 Genoa, Italy, and 7Nuclear Medicine Department, San Raffaele Scientific Institute, 20132 Milan, Italy Decision making under risk entails the anticipation of prospective outcomes, typically leading to the greater sensitivity to losses than gains known as loss aversion. Previous studies on the neural bases of choice-outcome anticipation and loss aversion provided inconsistent results, showing either bidirectional mesolimbic responses of activation for gains and deactivation for losses, or a specific amygdala involvement in processing losses. Here we focused on loss aversion with the aim to address interindividual differences in the neural bases of choice-outcome anticipation. Fifty-six healthy human participants accepted or rejected 104 mixed gambles offering equal (50%) chances of gaining or losing different amounts of money while their brain activity was measured with functional magnetic resonance imaging (fMRI). We report both bidirectional and gain/loss-specific responses while evaluating risky gambles, with amygdala and posterior insula specifically tracking the magnitude of potential losses.