GLOBAL Posmonlng SYSTEM and TOTAL STATION FIELD METHODS UTILIZED to DERIVE the ACCURACY of USGS Lo-METER DIGITAL ELEVATION MODELS on OAHU
Total Page:16
File Type:pdf, Size:1020Kb
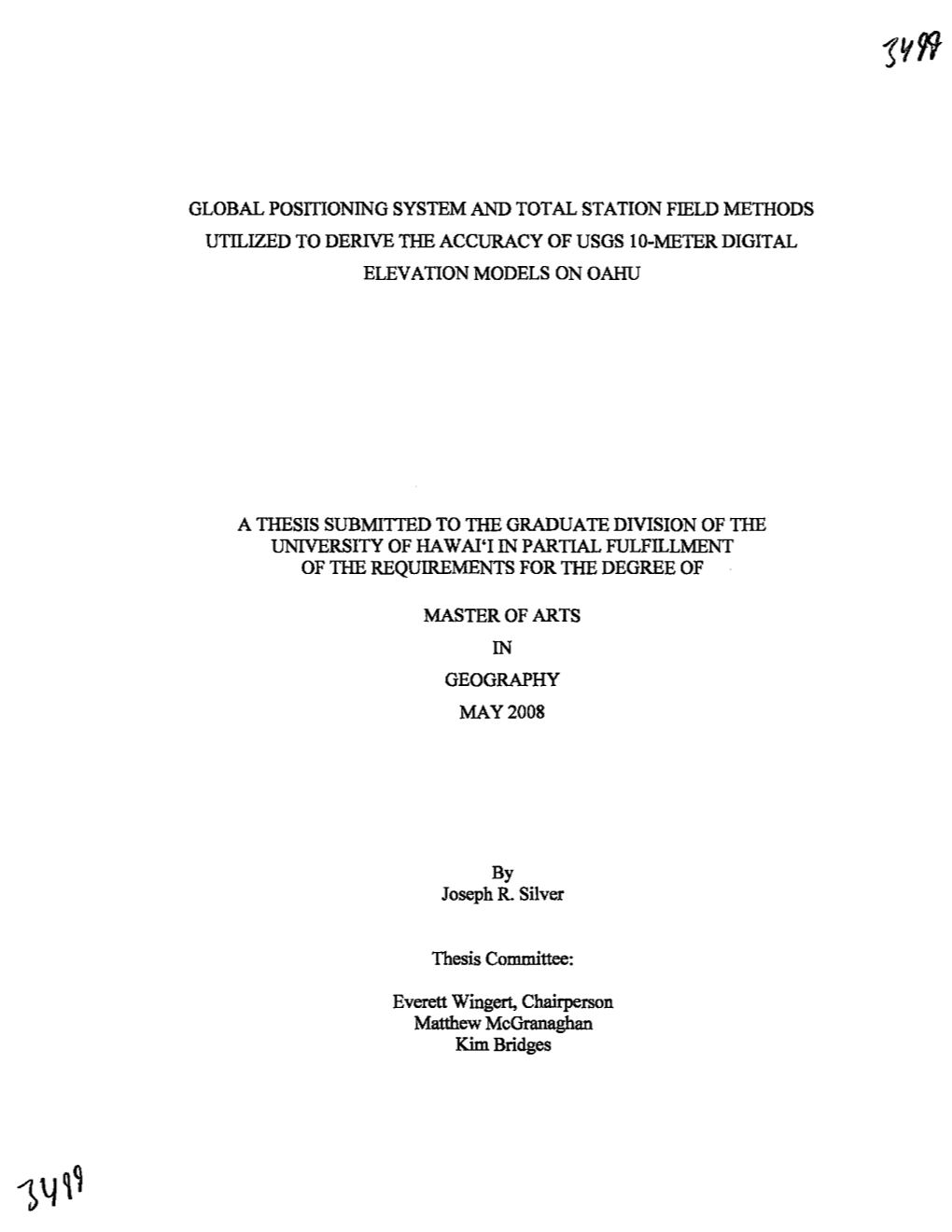
Load more
Recommended publications
-
Traversing the Past: the Total Station Theodolite in Archaeological
Traversing the Past The Total Station Theodolite in Archaeological Landscape Survey Summary This paper is one of a series on archaeological field survey techniques published by Historic England. It covers the electronic total station theodolite (TST) and its use in landscape archaeology. General guidance on the TST and its role in recording archaeological excavations and in surveying historic buildings is available elsewhere (Bettess 1998; Menuge 2006; Andrews et al 2009; 2010). In this paper these topics are only covered in principle. This guidance note has been prepared by Jon Bedford, Trevor Pearson and Bernard Thomason. Case Study 2 was written by Al Oswald. First published by English Heritage April 2011 This edition published by Historic England February 2016. All images © Historic England unless otherwise stated. www.HistoricEngland.org.uk/advice/technical-advice/ Front cover: The English Heritage Archaeological Survey and Investigation Team using a total station theodolite at the Black Beacon on Orford Ness, Suffolk. The Black Beacon is an octagonal building built in 1928 for the Royal Aircraft Establishment, Farnborough, to house radio direction finding equipment. Renovated in 1995, the beacon now provides an elevated viewing area for the visiting public Contents Introduction ..................................1 3 Divorced and Map-based Survey .................... 32 1 Background ................................3 Case Study 4 1.1 Origins.................................................................3 Little Doward, Herefordshire -
Total Station Mapping: Practical Examples from Alta and Baja California Tsim D
Santa Clara University Scholar Commons Faculty Publications Anthropology 12-2008 Total Station Mapping: Practical Examples from Alta and Baja California Tsim D. Schneider Lee M. Panich Santa Clara University, [email protected] Follow this and additional works at: https://scholarcommons.scu.edu/anthro_fac_pubs Part of the Anthropology Commons Recommended Citation Schneider, Tsim D., and Lee M. Panich (2008). Total Station Mapping: Practical Examples from Alta and Baja California. Journal of California and Great Basin Anthropology 28(2):166-183. Copyright © 2008 Malki Museum, Inc. Reprinted with permission. This Article is brought to you for free and open access by the Anthropology at Scholar Commons. It has been accepted for inclusion in Faculty Publications by an authorized administrator of Scholar Commons. For more information, please contact [email protected]. Journal of California and Great Basin Anthropology | Vol, 28, No, 2 (2008) | pp. 166-183 Total Station Mapping: the newer and more popular mapping techniques, such as the use of electronic total stations. Practical Examples from Our goal m this paper is to outhne two strategies for Alta and Baja California designing and implementing a mapping program usmg an electronic total station. We present two case studies TSIM D. SCHNEIDER that are intended as practical examples of total station use in two very different environments: the desert of LEE M. PANICH Baja CaUfomia, Mexico, and a forest m the San Francisco Dept. of Anthropology, University of Cahfornia Berkeley, California 94720 Bay area of California. A Sokkia SET510 electronic total station was used to map the archaeological site of Mission Santa Catalina m northem Baja California and The use of electronic total data stations for mapping a cluster of three sheU mounds in China Camp State archaeological sites is examined through two California Park, Marin County, California (Fig. -
Reflector-Less Total Station Measurements and Their Accuracy
University of Southern Queensland Faculty of Engineering and Surveying Reflectorless Total Station Measurements and their Accuracy, Precision and Reliability. A dissertation submitted by Leigh Herbert Coaker In fulfilment of the requirements of Courses ENG4111and 4112 Research Project Towards the degree of Bachelor of Spatial Science (Surveying) Submitted: October, 2009 Abstract Reflectorless Total Station Measurements and their Accuracy, Precision and Reliability. By Coaker, Leigh., University of Southern Queensland, 2009. Despite the vast technological advancements in equipment, the survey industry continues to struggle with the collection of data relating to inaccessible points. While the introduction of reflector‐less total stations has meant that inaccessible points can now be measured with relative ease, there are some questions as to the accuracy and reliability that can be achieved with such equipment. The object of this study is to determine likely limits for reliability of reflector‐less instruments especially in relation to measurements with large angles of incidence, but also looking at the vagaries caused by differing materials and beam divergence. The study has been carried out in various locations using Trimble S6 and S8 reflector‐less total station equipment to a variety of surfaces materials and shapes. This study suggests that angle of incidence of the measurement signal to the surface of the measured material has a large influence on the accuracy of that measurement. In the search for accurate survey results from -
DEM Development from Ground-Based Lidar Data: a Method to Remove Non-Surface Objects
Remote Sens. 2010, 2, 2629-2642; doi:10.3390/rs2112629 OPEN ACCESS Remote Sensing ISSN 2072-4292 www.mdpi.com/journal/remotesensing Article DEM Development from Ground-Based LiDAR Data: A Method to Remove Non-Surface Objects Maneesh Sharma 1, Ginger B. Paige 2,* and Scott N. Miller 2 1 WV GIS Technical Center, Department of Geology & Geography, 330 Brooks Hall, West Virginia University, Morgantown, WV 26505 USA; E-Mail: [email protected] 2 Department of Renewable Resources, University of Wyoming, Laramie, 1000 E. University Ave. WY 82071, USA; E-Mail: [email protected] * Author to whom correspondence should be addressed; E-Mail: [email protected]; Tel.: +1-307-766-2200; Fax: +1-307-766-6403. Received: 25 September 2010; in revised form: 12 October 2010 / Accepted: 8 November 2010 / Published: 23 November 2010 Abstract: Topography and land cover characteristics can have significant effects on infiltration, runoff, and erosion processes on watersheds. The ability to model the timing and routing of surface water and erosion is affected by the resolution of the digital elevation model (DEM). High resolution ground-based Light Detecting and Ranging (LiDAR) technology can be used to collect detailed topographic and land cover characteristic data. In this study, a method was developed to remove vegetation from ground-based LiDAR data to create high resolution DEMs. Research was conducted on intensively studied rainfall–runoff plots on the USDA-ARS Walnut Gulch Experimental Watershed in Southeast Arizona. LiDAR data were used to generate 1 cm resolution digital surface models (DSM) for 5 plots. DSMs created directly from LiDAR data contain non-surface objects such as vegetation cover. -
Total Station Mapping: Practical Examples from Alta and Baja California
UC Merced Journal of California and Great Basin Anthropology Title Total Station Mapping: Practical Examples from Alta and Baja California Permalink https://escholarship.org/uc/item/0fd610tw Journal Journal of California and Great Basin Anthropology, 28(2) ISSN 0191-3557 Authors Schneider, Tsim D. Panich, Lee M. Publication Date 2008 eScholarship.org Powered by the California Digital Library University of California Journal of California and Great Basin Anthropology | Vol, 28, No, 2 (2008) | pp. 166-183 Total Station Mapping: the newer and more popular mapping techniques, such as the use of electronic total stations. Practical Examples from Our goal m this paper is to outhne two strategies for Alta and Baja California designing and implementing a mapping program usmg an electronic total station. We present two case studies TSIM D. SCHNEIDER that are intended as practical examples of total station use in two very different environments: the desert of LEE M. PANICH Baja CaUfomia, Mexico, and a forest m the San Francisco Dept. of Anthropology, University of Cahfornia Berkeley, California 94720 Bay area of California. A Sokkia SET510 electronic total station was used to map the archaeological site of Mission Santa Catalina m northem Baja California and The use of electronic total data stations for mapping a cluster of three sheU mounds in China Camp State archaeological sites is examined through two California Park, Marin County, California (Fig. 1). In each case case studies. Mission Santa Catalina, located in the high study, we discuss the project area, our project goals, the desert of Baja California, and a cluster of three shell data coUection strategies we employed and the reasoning mounds, located in a forest in the San Francisco Bay behind them, as well as the techniques used for the area, represent two different examples of organizing and production of different digital mapping products. -
An Introduction to Survey Methods and Techniques
An Introduction to Survey Methods and Techniques Course No: A03-009 Credit: 3 PDH J. Paul Guyer, P.E., R.A., Fellow ASCE, Fellow AEI Continuing Education and Development, Inc. 22 Stonewall Court Woodcliff Lake, NJ 076 77 P: (877) 322-5800 [email protected] An Introduction to Survey Methods and Techniques J. Paul Guyer, P.E., R.A. Editor Paul Guyer is a registered civil engineer, mechanical engineer, fire protection engineer and architect with 35 years of experience designing buildings and related infrastructure. For an additional 9 years he was a principal staff advisor to the California Legislature on capital outlay and infrastructure issues. He is a graduate of Stanford University and has held numerous national, state and local offices with the American Society of Civil Engineers, Architectural Engineering Institute and National Society of Professional Engineers. He is a Fellow of ASCE and AEI. © J. Paul Guyer 2015 1 An Introduction to Survey Methods and Techniques J. Paul Guyer, P.E., R.A. Editor The Clubhouse Press El Macero, California © J. Paul Guyer 2015 2 CONTENTS 1. GENERAL 2. TOTAL STATIONS 3. REAL TIME KINEMATIC (RTK) GPS 4. TERRESTRIAL LIDAR (LASER) SCANNING 5. TOPOGRAPHIC DATA COLLECTION PROCEDURES 6. AUTOMATED FIELD DATA COLLECTION 7. METHODS OF DELINEATING AND DENSIFYING TOPOGRAPHIC FEATURES (This publication is adapted from the Unified Facilities Criteria of the United States government which are in the public domain, have been authorized for unlimited distribution, and are not copyrighted.) © J. Paul Guyer 2015 3 1. OLDER TOPOGRAPHIC SURVEYING METHODS. This section provides an overview of the past and present instruments and methods used to perform topographic surveys of sites, facilities, or infrastructure. -
Chapter 1 Surveying Chapter 1 Surveying Part 650 Engineering Field Handbook
United States Department of Part 650 Agriculture Natural Engineering Field Handbook Resources Conservation Service Chapter 1 Surveying Chapter 1 Surveying Part 650 Engineering Field Handbook Issued October 2008 Cover illustration: Roman groma The early Roman surveyors used an instrument called the groma to lay out their cities and roads by right angles. The word “groma” is thought to have come from the root Greek word “gnome,” which is defined as the pointer of a sundial. The importance of turning right angles is shown in the layout of several cities in northern Italy, as well as North Africa. The land was subdi- vided into 2,400 Roman feet square. Each square was called a centuria. The centurias were crossed by road at right angles to each other. The roads that ran north-south were called cardines, and the roads that ran east-west were called decumani. The groma was also used to locate and place monumen- tation for property corners. Once the centurias were in place, maps were drawn on bronze tablets giving a physical representation of the centuria. The U.S. Department of Agriculture (USDA) prohibits discrimination in all its programs and activities on the basis of race, color, national origin, age, disability, and where applicable, sex, marital status, familial status, parental status, religion, sexual orientation, genetic information, political beliefs, re- prisal, or because all or a part of an individual’s income is derived from any public assistance program. (Not all prohibited bases apply to all programs.) Persons with disabilities who require alternative means for communication of program information (Braille, large print, audiotape, etc.) should contact USDA’s TARGET Center at (202) 720-2600 (voice and TDD). -
Surveying Made Easy
30 40 50 Surveying made easy Karl Zeiske presented by: Virtual Archive of WILD Heerbrugg www.wild-heerbrugg.com Introduction This booklet will tell you • What are the main The use of levels and total instruments available today about the basic principles features of these stations is illustrated by a from Leica Geosystems; of surveying. instruments? series of practical neither does it touch on examples. In addition, their individual performance The most important • What needs to be taken applications programs are features. These aspects are instruments for surveying into account when described; these are covered by the com- are levels and total stations; measuring with a level or incorporated into the prehensive brochures, by the they are intended for with a total station? modern total stations technical consultants in the routine survey tasks. manufactured by Leica Leica Geosystems agencies, Anyone wishing to know • What are the effects of Geosystems and they solve and by the home pages in how and where they are instrument errors? survey tasks even more the Internet used will find the answers easily and elegantly. (www.leica-geosystems.com). here. • How can such errors be Equipped with the recognized, determined knowledge in this booklet, and eliminated? and with the help of the appropriate user manual, • How can simple anyone can carry out surveying jobs be simple survey tasks performed? confidently and efficiently. This booklet does not describe the range of 2 Virtual Archive of WILD Heerbrugg www.wild-heerbrugg.com Contents The level