Cell-Type Specific Innervation of Cortical Pyramidal Cells at Their
Total Page:16
File Type:pdf, Size:1020Kb
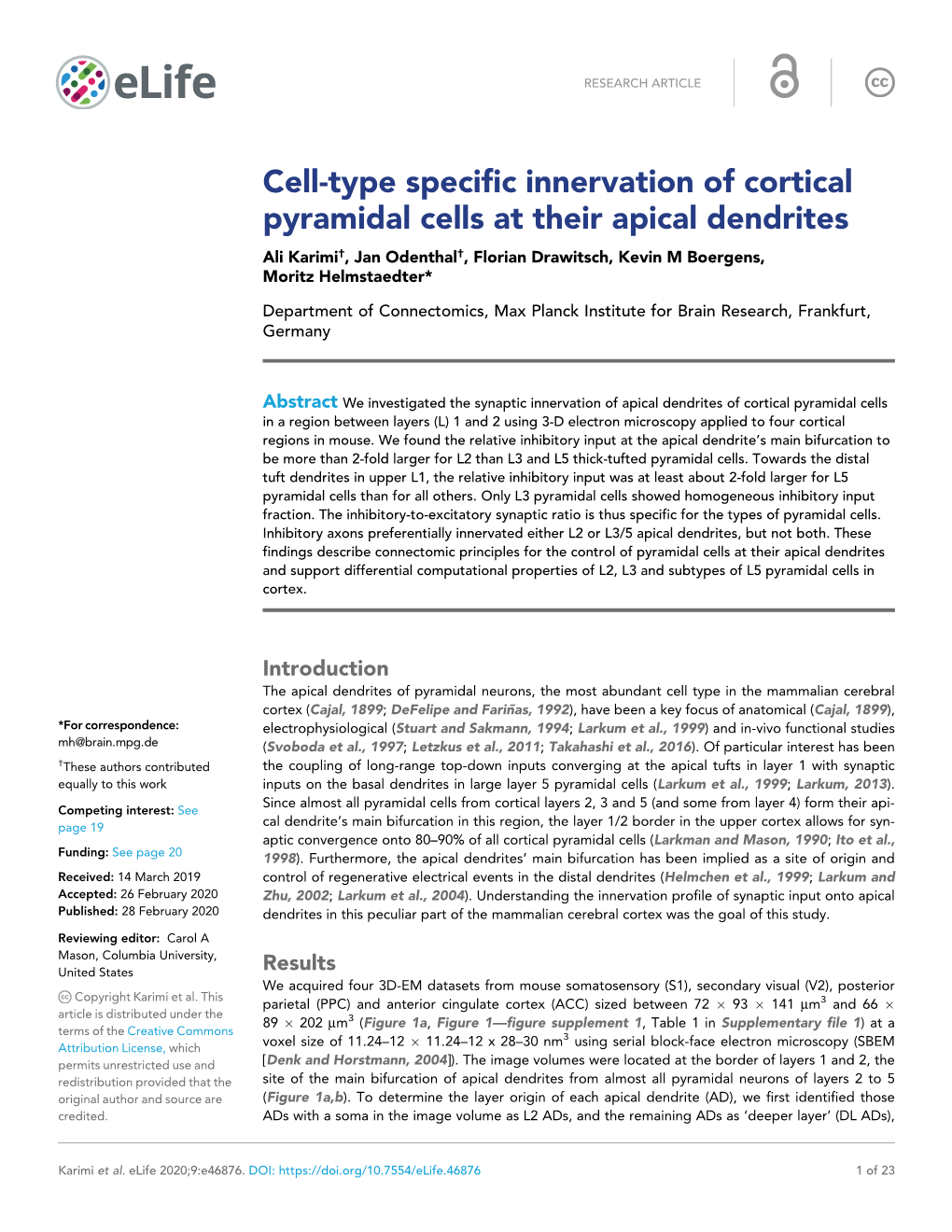
Load more
Recommended publications
-
Resting and Active Properties of Pyramidal Neurons in Subiculum and CA1 of Rat Hippocampus
Resting and Active Properties of Pyramidal Neurons in Subiculum and CA1 of Rat Hippocampus NATHAN P. STAFF, HAE-YOON JUNG, TARA THIAGARAJAN, MICHAEL YAO, AND NELSON SPRUSTON Department of Neurobiology and Physiology, Institute for Neuroscience, Northwestern University, Evanston, Illinois 60208 Received 22 March 2000; accepted in final form 8 August 2000 Staff, Nathan P., Hae-Yoon Jung, Tara Thiagarajan, Michael region (Chrobak and Buzsaki 1996; Colling et al. 1998). Sub- Yao, and Nelson Spruston. Resting and active properties of pyrami- iculum has been shown to be the major output center of the dal neurons in subiculum and CA1 of rat hippocampus. J Neuro- hippocampus, to which it belongs, sending parallel projections physiol 84: 2398–2408, 2000. Action potentials are the end product of synaptic integration, a process influenced by resting and active neu- from various subicular regions to different cortical and subcor- ronal membrane properties. Diversity in these properties contributes tical areas (Naber and Witter 1998). Functionally, the principal to specialized mechanisms of synaptic integration and action potential neurons of the subiculum appear to be “place cells,” similar to firing, which are likely to be of functional significance within neural those of CA1 (Sharp and Green 1994); and in a human mag- circuits. In the hippocampus, the majority of subicular pyramidal netic resonance imaging (MRI) study, subiculum was shown to neurons fire high-frequency bursts of action potentials, whereas CA1 be an area involved in memory retrieval (Gabrieli et al. 1997). pyramidal neurons exhibit regular spiking behavior when subjected to Therefore both CA1 and subiculum have been implicated in direct somatic current injection. -
Long-Term Adult Human Brain Slice Cultures As a Model System to Study
TOOLS AND RESOURCES Long-term adult human brain slice cultures as a model system to study human CNS circuitry and disease Niklas Schwarz1, Betu¨ l Uysal1, Marc Welzer2,3, Jacqueline C Bahr1, Nikolas Layer1, Heidi Lo¨ ffler1, Kornelijus Stanaitis1, Harshad PA1, Yvonne G Weber1,4, Ulrike BS Hedrich1, Ju¨ rgen B Honegger4, Angelos Skodras2,3, Albert J Becker5, Thomas V Wuttke1,4†*, Henner Koch1†* 1Department of Neurology and Epileptology, Hertie-Institute for Clinical Brain Research, University of Tu¨ bingen, Tu¨ bingen, Germany; 2Department of Cellular Neurology, Hertie-Institute for Clinical Brain Research, University of Tu¨ bingen, Tu¨ bingen, Germany; 3German Center for Neurodegenerative Diseases (DZNE), Tu¨ bingen, Germany; 4Department of Neurosurgery, University of Tu¨ bingen, Tu¨ bingen, Germany; 5Department of Neuropathology, Section for Translational Epilepsy Research, University Bonn Medical Center, Bonn, Germany Abstract Most of our knowledge on human CNS circuitry and related disorders originates from model organisms. How well such data translate to the human CNS remains largely to be determined. Human brain slice cultures derived from neurosurgical resections may offer novel avenues to approach this translational gap. We now demonstrate robust preservation of the complex neuronal cytoarchitecture and electrophysiological properties of human pyramidal neurons *For correspondence: in long-term brain slice cultures. Further experiments delineate the optimal conditions for efficient [email protected] viral transduction of cultures, enabling ‘high throughput’ fluorescence-mediated 3D reconstruction tuebingen.de (TVW); of genetically targeted neurons at comparable quality to state-of-the-art biocytin fillings, and [email protected] demonstrate feasibility of long term live cell imaging of human cells in vitro. -
Dendritic Development of Gabaergic Cortical Interneurons Revealed by Biolistic Transfection with GFP
Graduate Theses, Dissertations, and Problem Reports 2002 Dendritic development of GABAergic cortical interneurons revealed by biolistic transfection with GFP Xiaoming Jin West Virginia University Follow this and additional works at: https://researchrepository.wvu.edu/etd Recommended Citation Jin, Xiaoming, "Dendritic development of GABAergic cortical interneurons revealed by biolistic transfection with GFP" (2002). Graduate Theses, Dissertations, and Problem Reports. 1708. https://researchrepository.wvu.edu/etd/1708 This Dissertation is protected by copyright and/or related rights. It has been brought to you by the The Research Repository @ WVU with permission from the rights-holder(s). You are free to use this Dissertation in any way that is permitted by the copyright and related rights legislation that applies to your use. For other uses you must obtain permission from the rights-holder(s) directly, unless additional rights are indicated by a Creative Commons license in the record and/ or on the work itself. This Dissertation has been accepted for inclusion in WVU Graduate Theses, Dissertations, and Problem Reports collection by an authorized administrator of The Research Repository @ WVU. For more information, please contact [email protected]. Dendritic Development of GABAergic Cortical Interneurons Revealed by Biolistic Transfection with GFP Xiaoming Jin Dissertation submitted to the School of Medicine at West Virginia University in partial fulfillment of the requirements for the degree of Doctor of Philosophy in Anatomy Ariel Agmon, Ph.D., Chair Albert Berrebi, Ph.D. Richard Dey, Ph.D. Peter Mathers, Ph.D. Adrienne Salm, Ph.D. Department of Neurobiology and Anatomy Morgantown, West Virginia 2002 Keywords: neocortex, GAD, gene gun, dendrite, growth, BDNF, neuronal activity, organotypic, brain slice, culture. -
Neurotrophins Regulate Dendritic Growth in Developing Visual Codex
Neuron, Vol. 15, 791-803, October, 1995, Copyright © 1995 by Cell Press Neurotrophins Regulate Dendritic Growth in Developing Visual Codex A. Kimberley McAllister, Donald C. Lo, al., 1988; Snider, 1988; Ruit et al., 1990; Ruit and Snider, and Lawrence C. Katz 1991). The neurotrophins comprise at least four structur- Department of Neurobiology ally related proteins: NGF, brain-derived neurotrophic fac- Duke University Medical Center tor (BDNF), neurotrophin-3 (NT-3), and neurotrophin-4/ Durham, North Carolina 27710 neurotrophin-5 (NT-4) (for review, see Lindsay et al., 1994; NT-6 has recently been cloned in fish [Gotz et al., 1994]). Summary Also, neurotrophins exert their effects through activation of members of the Trk family of tyrosine kinase receptors Although dendritic growth and differentiation are criti- (for review, see Chao, 1992). These factors and their re- cal for the proper development and function of neocor- ceptors are expressed in discrete layers of neocortex dur- tex, the molecular signals that regulate these pro- ing the time dendrites develop (Allendoerfer, et al., 1990; cesses are largely unknown. The potential role of Merlio et al., 1992; Cabelli et al., 1993, Soc. Neurosci., neurotrophins was tested by treating slices of devel- abstract; Ringstedt et al., 1993; Lindsay et al., 1994). How- oping visual cortex with NGF, BDNF, NT-3, or NT-4 and ever, assigning specific biological functions to neurotroph- by subsequently visualizing the dendrites of pyramidal ins has been hampered by the difficulty of manipulating neurons using particle-mediated gene transfer. Spe- the cortical environment in vivo and by the loss of laminar cific neurotrophins increased the length and complex- identity and cell polarity in dissociated tissue culture. -
Morphological Characteristics of Electrophysiologically Characterized Layer Vb Pyramidal Cells in Rat Barrel Cortex
RESEARCH ARTICLE Morphological Characteristics of Electrophysiologically Characterized Layer Vb Pyramidal Cells in Rat Barrel Cortex Jochen F. Staiger1*, Alexandre J. C. Loucif2¤, Dirk Schubert3, Martin MoÈ ck1 1 Institute for Neuroanatomy, University Medical Center, Georg-August-University, GoÈttingen, Germany, 2 Institute of Neuroanatomy, Albert-Ludwigs-University, Freiburg, Germany, 3 Donders Institute for Brain, Cognition & Behavior, Centre for Neuroscience, Radboud University Medical Centre, Nijmegen, The Netherlands a11111 ¤ Current address: Neuroscience and Pain Research, Pfizer Worldwide Research and Development; Portway Building, Granta Park, Great Abington, Cambridge, United Kingdom * [email protected] Abstract Layer Vb pyramidal cells are the major output neurons of the neocortex and transmit the OPEN ACCESS outcome of cortical columnar signal processing to distant target areas. At the same time Citation: Staiger JF, Loucif AJC, Schubert D, MoÈck they contribute to local tactile information processing by emitting recurrent axonal collater- M (2016) Morphological Characteristics of Electrophysiologically Characterized Layer Vb als into the columnar microcircuitry. It is, however, not known how exactly the two types of Pyramidal Cells in Rat Barrel Cortex. PLoS ONE 11 pyramidal cells, called slender-tufted and thick-tufted, contribute to the local circuitry. (10): e0164004. doi:10.1371/journal. Here, we investigated in the rat barrel cortex the detailed quantitative morphology of biocy- pone.0164004 tin-filled layer Vb pyramidal cells in vitro, which were characterized for their intrinsic Editor: Gennady Cymbalyuk, Georgia State electrophysiology with special emphasis on their action potential firing pattern. Since we University, UNITED STATES stained the same slices for cytochrome oxidase, we could also perform layer- and column- Received: September 17, 2015 related analyses. -
On the Integration of Subthreshold Inputs from Perforant Path and Schaffer Collaterals in Hippocampal CA1 Pyramidal Neurons
Journal of Computational Neuroscience 14, 185–192, 2003 c 2003 Kluwer Academic Publishers. Manufactured in The Netherlands. On the Integration of Subthreshold Inputs from Perforant Path and Schaffer Collaterals in Hippocampal CA1 Pyramidal Neurons MICHELE MIGLIORE Section of Neurobiology, Yale University School of Medicine, New Haven, CT, USA; Institute of Biophysics, Nat. Res. Council, Palermo, Italy [email protected] Received October 15, 2001; Revised September 6, 2002; Accepted September 6, 2002 Action Editor: E. Bard Ermentrout Abstract. Using a realistic model of a CA1 hippocampal pyramidal neuron, we make experimentally testable predictions on the roles of the non-specific cation current, Ih, and the A-type Potassium current, IA, in modulating the temporal window for the integration of the two main excitatory afferent pathways of a CA1 neuron, the Schaffer Collaterals and the Perforant Path. The model shows that the experimentally observed increase in the dendritic density of Ih and IA could have a major role in constraining the temporal integration window for these inputs, in such a way that a somatic action potential (AP) is elicited only when they are activated with a relative latency consistent with the anatomical arrangement of the hippocampal circuitry. Keywords: dendritic integration, IA, Ih, CA1, modeling Introduction these two conductances between pyramidal neurons of hippocampus and neocortex. The gKA increases with Although important details on how dendrites and their distance from the soma in CA1, whereas in neocor- active properties are involved in neural computation tical neurons it is constant (Korngreen and Sakmann, have been elucidated, the rules according to which 2000; Bekkers, 2000), and it does not seem to play the dendritic trees and, especially, ionic conductances are same role as in CA1 (Stuart and H¨ausser, 2001). -
Differential Timing and Coordination of Neurogenesis and Astrogenesis
brain sciences Article Differential Timing and Coordination of Neurogenesis and Astrogenesis in Developing Mouse Hippocampal Subregions Allison M. Bond 1, Daniel A. Berg 1, Stephanie Lee 1, Alan S. Garcia-Epelboim 1, Vijay S. Adusumilli 1, Guo-li Ming 1,2,3,4 and Hongjun Song 1,2,3,5,* 1 Department of Neuroscience and Mahoney Institute for Neurosciences, Perelman School of Medicine, University of Pennsylvania, Philadelphia, PA 19104, USA; [email protected] (A.M.B.); [email protected] (D.A.B.); [email protected] (S.L.); [email protected] (A.S.G.-E.); [email protected] (V.S.A.); [email protected] (G.-l.M.) 2 Department of Cell and Developmental Biology, Perelman School of Medicine, University of Pennsylvania, Philadelphia, PA 19104, USA 3 Institute for Regenerative Medicine, University of Pennsylvania, Philadelphia, PA 19104, USA 4 Department of Psychiatry, Perelman School of Medicine, University of Pennsylvania, Philadelphia, PA 19104, USA 5 The Epigenetics Institute, Perelman School of Medicine, University of Pennsylvania, Philadelphia, PA 19104, USA * Correspondence: [email protected] Received: 19 October 2020; Accepted: 24 November 2020; Published: 26 November 2020 Abstract: Neocortical development has been extensively studied and therefore is the basis of our understanding of mammalian brain development. One fundamental principle of neocortical development is that neurogenesis and gliogenesis are temporally segregated processes. However, it is unclear how neurogenesis and gliogenesis are coordinated in non-neocortical regions of the cerebral cortex, such as the hippocampus, also known as the archicortex. Here, we show that the timing of neurogenesis and astrogenesis in the Cornu Ammonis (CA) 1 and CA3 regions of mouse hippocampus mirrors that of the neocortex; neurogenesis occurs embryonically, followed by astrogenesis during early postnatal development. -
Astrocyte Calcium Signals at Schaffer Collateral to CA1 Pyramidal Cell Synapses Correlate with the Number of Activated Synapses but Not with Synaptic Strength
HIPPOCAMPUS 00:000–000 (2010) Astrocyte Calcium Signals at Schaffer Collateral to CA1 Pyramidal Cell Synapses Correlate With the Number of Activated Synapses But Not With Synaptic Strength Silke D. Honsek, Corinna Walz, Karl W. Kafitz, and Christine R. Rose* ABSTRACT: Glial cells respond to neuronal activity by transient astrocytes mediate the clearance of glutamate from the increases in their intracellular calcium concentration. At hippocampal extracellular space through high-affinity transporters. Schaffer collateral to CA1 pyramidal cell synapses, such activity-induced This glutamate uptake shapes the time course of syn- astrocyte calcium transients modulate neuronal excitability, synaptic activ- ity, and LTP induction threshold by calcium-dependent release of gliotrans- aptic conductance, moderates the activation of extrasy- mitters. Despite a significant role of astrocyte calcium signaling in plasti- naptic receptors, and limits spillover of glutamate to city of these synapses, little is known about activity-dependent changes of adjacent synapses (Tzingounis and Wadiche, 2007). In astrocyte calcium signaling itself. In this study, we analyzed calcium transi- addition, astrocytes respond to synaptically released stratum radiatum ents in identified astrocytes and NG2-cells located in the glutamate with calcium transients that are mainly in response to different intensities and patterns of Schaffer collateral stimu- lation. To this end, we employed multiphoton calcium imaging with the caused by the activation of metabotropic glutamate low-affinity -
Contribution of Apical and Basal Dendrites to Orientation Encoding in Mouse V1 L2/3 Pyramidal Neurons
ARTICLE https://doi.org/10.1038/s41467-019-13029-0 OPEN Contribution of apical and basal dendrites to orientation encoding in mouse V1 L2/3 pyramidal neurons Jiyoung Park1,2,7*, Athanasia Papoutsi3,7, Ryan T. Ash1,4,5, Miguel A. Marin4,6, Panayiota Poirazi 3,8*& Stelios M. Smirnakis1,8* 1234567890():,; Pyramidal neurons integrate synaptic inputs from basal and apical dendrites to generate stimulus-specific responses. It has been proposed that feed-forward inputs to basal dendrites drive a neuron’s stimulus preference, while feedback inputs to apical dendrites sharpen selectivity. However, how a neuron’s dendritic domains relate to its functional selectivity has not been demonstrated experimentally. We performed 2-photon dendritic micro-dissection on layer-2/3 pyramidal neurons in mouse primary visual cortex. We found that removing the apical dendritic tuft did not alter orientation-tuning. Furthermore, orientation-tuning curves were remarkably robust to the removal of basal dendrites: ablation of 2 basal dendrites was needed to cause a small shift in orientation preference, without significantly altering tuning width. Computational modeling corroborated our results and put limits on how orientation preferences among basal dendrites differ in order to reproduce the post-ablation data. In conclusion, neuronal orientation-tuning appears remarkably robust to loss of dendritic input. 1 Brigham and Women’s Hospital and Jamaica Plain VA Hospital, Harvard Medical School, Boston, MA, USA. 2 Program in Structural and Computational Biology and Molecular Biophysics, Baylor College of Medicine, Houston, TX, USA. 3 Institute of Molecular Biology and Biotechnology (IMBB), Foundation of Research and Technology Hellas (FORTH), Vassilika Vouton, HeraklionCrete, Greece. -
Dendritic Size of Pyramidal Neurons Differs Among Mouse Cortical
Cerebral Cortex July 2006;16:990--1001 doi:10.1093/cercor/bhj041 Advance Access publication September 29, 2005 Dendritic Size of Pyramidal Neurons Differs Ruth Benavides-Piccione1,2, Farid Hamzei-Sichani2, Inmaculada Ballesteros-Ya´n˜ez1, Javier DeFelipe1 and Rafael Yuste1,2 among Mouse Cortical Regions 1Instituto Cajal, Madrid, Spain and 2HHMI, Department of Biological Sciences, Columbia University, New York, USA Downloaded from https://academic.oup.com/cercor/article-abstract/16/7/990/425668 by University of Massachusetts Medical School user on 19 February 2019 Neocortical circuits share anatomical and physiological similarities a series of basic circuit diagrams based on anatomical and among different species and cortical areas. Because of this, electrophysiological data (Douglas et al., 1989, 1995; Douglas a ‘canonical’ cortical microcircuit could form the functional unit and Martin, 1991, 1998, 2004). According to their hypothesis, of the neocortex and perform the same basic computation on the common transfer function that the neocortex performs on different types of inputs. However, variations in pyramidal cell inputs could be related to the amplification of the signal structure between different primate cortical areas exist, indicating (Douglas et al., 1989) or a ‘soft’ winner-take-all algorithm that different cortical areas could be built out of different neuronal (Douglas and Martin, 2004). These ideas agree with the re- cell types. In the present study, we have investigated the dendritic current excitation present in cortical tissue which could then architecture of 90 layer II/III pyramidal neurons located in different exert a top-down amplification and selection on thalamic inputs cortical regions along a rostrocaudal axis in the mouse neocortex, (Douglas et al., 1995). -
Development and Evolution of the Human Neocortex
Leading Edge Review Development and Evolution of the Human Neocortex Jan H. Lui,1,2,3 David V. Hansen,1,2,4 and Arnold R. Kriegstein1,2,* 1Eli and Edythe Broad Center of Regeneration Medicine and Stem Cell Research, University of California, San Francisco, 35 Medical Center Way, San Francisco, CA 94143, USA 2Department of Neurology 3Biomedical Sciences Graduate Program University of California, San Francisco, 513 Parnassus Avenue, San Francisco, CA 94143, USA 4Present address: Department of Neuroscience, Genentech, Inc., 1 DNA Way, MS 230B, South San Francisco, CA 94080, USA *Correspondence: [email protected] DOI 10.1016/j.cell.2011.06.030 The size and surface area of the mammalian brain are thought to be critical determinants of intel- lectual ability. Recent studies show that development of the gyrated human neocortex involves a lineage of neural stem and transit-amplifying cells that forms the outer subventricular zone (OSVZ), a proliferative region outside the ventricular epithelium. We discuss how proliferation of cells within the OSVZ expands the neocortex by increasing neuron number and modifying the trajectory of migrating neurons. Relating these features to other mammalian species and known molecular regulators of the mouse neocortex suggests how this developmental process could have emerged in evolution. Introduction marsupials begin to reveal how differences in neural progenitor Evolution of the neocortex in mammals is considered to be a key cell populations can result in neocortices of variable size and advance that enabled higher cognitive function. However, neo- shape. Increases in neocortical volume and surface area, partic- cortices of different mammalian species vary widely in shape, ularly in the human, are related to the expansion of progenitor size, and neuron number (reviewed by Herculano-Houzel, 2009). -
Sonic Hedgehog Signaling in Astrocytes Mediates Cell Type
RESEARCH ARTICLE Sonic hedgehog signaling in astrocytes mediates cell type-specific synaptic organization Steven A Hill1†, Andrew S Blaeser1†, Austin A Coley2, Yajun Xie3, Katherine A Shepard1, Corey C Harwell3, Wen-Jun Gao2, A Denise R Garcia1,2* 1Department of Biology, Drexel University, Philadelphia, United States; 2Department of Neurobiology and Anatomy, Drexel University College of Medicine, Philadelphia, United States; 3Department of Neurobiology, Harvard Medical School, Boston, United States Abstract Astrocytes have emerged as integral partners with neurons in regulating synapse formation and function, but the mechanisms that mediate these interactions are not well understood. Here, we show that Sonic hedgehog (Shh) signaling in mature astrocytes is required for establishing structural organization and remodeling of cortical synapses in a cell type-specific manner. In the postnatal cortex, Shh signaling is active in a subpopulation of mature astrocytes localized primarily in deep cortical layers. Selective disruption of Shh signaling in astrocytes produces a dramatic increase in synapse number specifically on layer V apical dendrites that emerges during adolescence and persists into adulthood. Dynamic turnover of dendritic spines is impaired in mutant mice and is accompanied by an increase in neuronal excitability and a reduction of the glial-specific, inward-rectifying K+ channel Kir4.1. These data identify a critical role for Shh signaling in astrocyte-mediated modulation of neuronal activity required for sculpting synapses. *For correspondence: DOI: https://doi.org/10.7554/eLife.45545.001 [email protected] †These authors contributed equally to this work Introduction Competing interests: The The organization of synapses into the appropriate number and distribution occurs through a process authors declare that no of robust synapse addition followed by a period of refinement during which excess synapses are competing interests exist.