EFI+ 0044096 Deliverable 3 4 New Metrics Development
Total Page:16
File Type:pdf, Size:1020Kb
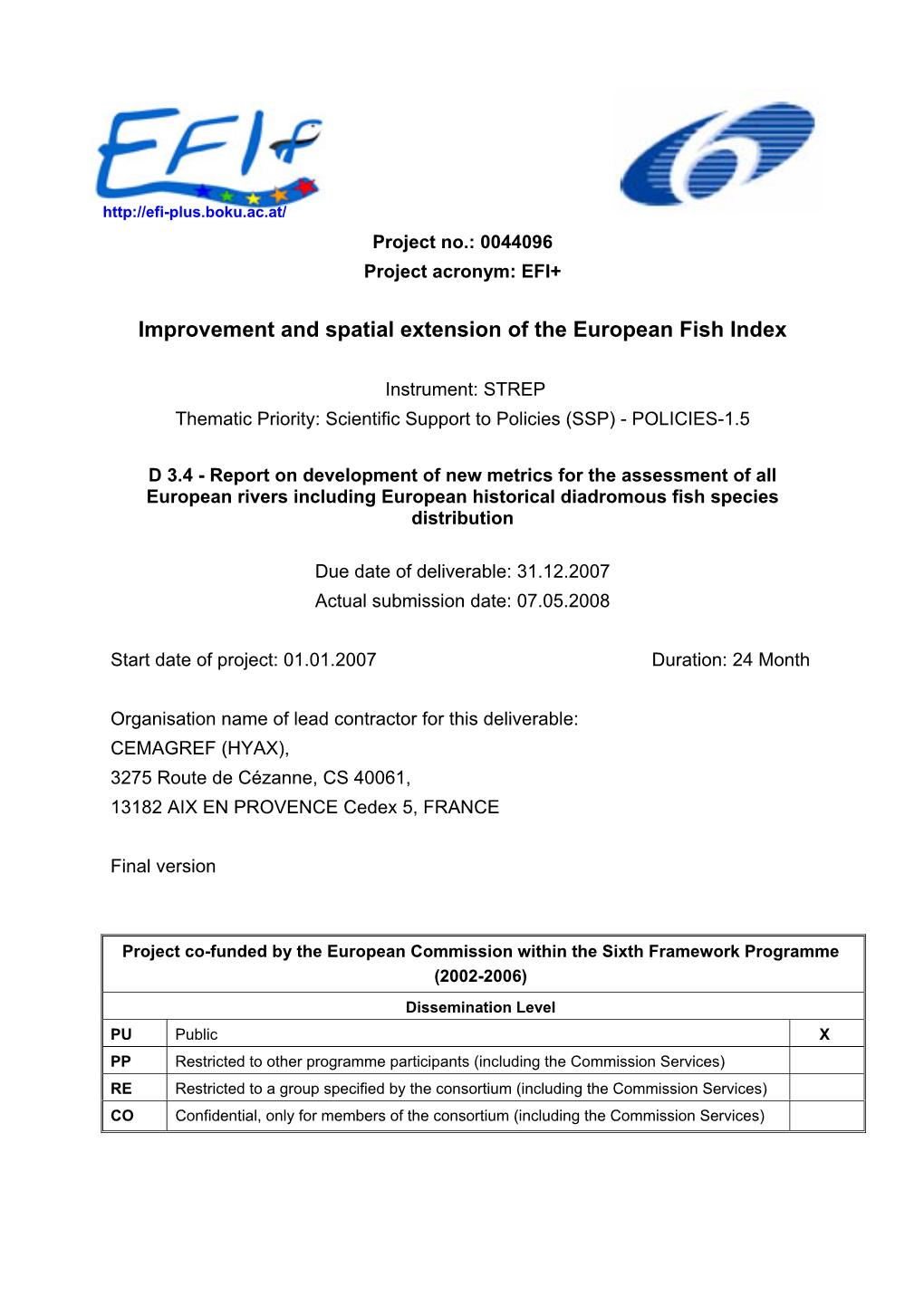
Load more
Recommended publications
-
Artículos Publicados En Revistas Científicas Con Mención Expresa Al Proyecto Migranet En Los Agradecimientos
ARTÍCULOS PUBLICADOS EN REVISTAS CIENTÍFICAS CON MENCIÓN EXPRESA AL PROYECTO MIGRANET EN LOS AGRADECIMIENTOS PROYECTO MIGRANET- “OBSERVATORIO DE LAS POBLACIONES DE PECES MIGRADORES EN EL ESPACIO SUDOE” 1.- Araújo MJ, Ozório ROA, Antunes C (submitted). Linking ecology and nutrition: considerations on spawning migrations of diadromous fish species in Iberian Peninsula. Review. Limnetica. 2.- Araújo MJ, Ozório ROA, Bessa RJB, Kijjoa A, Gonçalves JFM, Antunes C. (submitted). Nutritional status of adult sea lamprey (Petromyzon marinus Linnaeus, 1758) during spawning migration in the Minho River, NW Iberian Peninsula. Journal Applied Ichthiology. 3.- Cobo, F., Sánchez-Hernández, J., Vieira-Lanero, R., & Servia, MJ. 2012. Organic pollution induces domestication-like characteristics in feral populations of brown trout (Salmo trutta). DOI 10.1007/s10750-012-1386-4. 4.- Dias E, Morais P, Antunes C, Hoffman JC (submitted). Organic matter sources supporting the high secondary production of the bivalve Corbicula fluminea in an invaded ecosystem. Biological Invasions. 5.- Sánchez-Hernández, J., Servia, MJ., Vieira-Lanero, R., & Cobo, F. 2012. New record of translocated Phoxinus bigerri Kottelat, 2007 from a river basin in the North- West Atlantic coast of the Iberian Peninsula. BioInvasions Records, 1 (1): 37–39. 6.- Sánchez-Hernández, J., Servia, MJ., Vieira-Lanero, R., & Cobo, F. 2012. Aplicación del análisis de los rasgos ecológicos (“traits”) de las presas para el estudio del comportamiento alimentario en peces bentófagos: el ejemplo del espinoso (Gasterosteus gymnurus Cuvier, 1829). Limnetica, 31 (1): 59-76. 7.- Sánchez-Hernández, J., Servia, MJ., Vieira-Lanero, R., Barca-Bravo, S., & Cobo, F 2012. References data on the growth and population parameters of brown trout in siliceous rivers of Galicia (NW Spain). -
Effects of Invasive Fish and Temperature on the Foraging Success of Southern Iberian Chub
UNIVERSIDADE DE LISBOA FACULDADE DE CIÊNCIAS DEPARTAMENTO DE BIOLOGIA ANIMAL Effects of invasive fish and temperature on the foraging success of Southern Iberian chub Alexa Philippa Kodde Mestrado em Ecologia e Gestão Ambiental Dissertação orientada por: Maria Filomena de Magalhães Christos Gkenas 2017 “But the reason I call myself by my childhood name is to remind myself that a scientist must also be absolutely like a child. If he sees a thing, he must say that he sees it, whether it was what he thought he was going to see or not. See first, think later, then test. But always see first. Otherwise you will only see what you were expecting.” ― Douglas Adams (1984), “So Long, and Thanks for All the Fish”. i ii ACKNOWLEDGMENTS Throughout this thesis I’ve had the pleasure of gaining new skills, experiences and friends, and have so much respect for all those that have helped me on my way to making this work possible. These people have my immense gratitude and thanks. I owe everything to my supervisors, Dr. Maria Filomena Magalhães and Dr. Christos Gkenas, for guiding me in every aspect of this project, from conception to presentation, for their tutoring, advice and support, for teaching me to be able to work both independently and as part of a research group. Many thanks to all those from the university that I’ve worked with in the past months, in the field and in the bioterium, Dr. João Gago, Rui Monteiro, Sara Carona, Diogo Ribeiro, Marian Prodan, Marco Ferreira, Luís Almeida, Nuno Castro, Somayeh Doosti, and a special thanks to António Barata, for all things database and Python related, to Gisela Cheoo, who deserves a medal for all her work and dedication, and to Dr. -
Informe Evaluaciones EICAT UICN
EVALUACIÓN DEL IMPACTO AMBIENTAL DE ESPECIES EXÓTICAS INVASORAS EEN LA CUENCA DEL GUADIANA A TRAVÉS DE LA METODOLOGÍA EICAT Abril 2019 Con el apoyo de: Título Evaluación del impacto ambiental de especies exóticas invasoras en la cuenca del Guadiana a través de la metodología EICAT Versión Abril de 2019 Idioma original Español Unidad responsable Programa de especies UICN‐Med Redactado por Laura Capdevila Argüelles (GEIB), Helena Clavero Sousa (UICN‐ Med) y Catherine Numa (UICN‐Med) Proyecto “Evaluación del impacto potencial de especies introducidas en España: análisis de viabilidad del sistema de clasificación EICAT” financiado con el apoyo del Ministerio para la Transición Ecológica, a través de la Fundación Biodiversidad, y de la Fundación MAVA 1 Índice AGRADECIMIENTOS ................................................................................................................................ 3 ACRÓNIMOS ............................................................................................................................................ 4 ANTECEDENTES ....................................................................................................................................... 5 METODOLOGÍA ....................................................................................................................................... 6 FICHAS‐RESUMEN DE LAS EVALUACIONES EICAT ................................................................................... 7 Azolla filiculoides ................................................................................................................................ -
Cenozoic Tectonic and Climatic Events in Southern Iberian Peninsula: Implications
Research paper Cenozoic tectonic and climatic events in southern Iberian Peninsula: implications for the evolutionary history of freshwater fish of the genus Squalius (Actinopterygii, Cyprinidae) Silvia Perea 1*, Marta Cobo-Simon2 and Ignacio Doadrio1 1 Biodiversity and Evolutionary Group. Museo Nacional de Ciencias Naturales, CSIC, C/ José Gutiérrez Abascal, 2. 28006, Madrid, Spain 2 National Center of Biotechnology, Systems Biology Department, C/ Darwin 3. 28049, Madrid, Spain. *Corresponding author: Silvia Perea; Tel.: +34 91 4111328; fax: +34 91 5645078. Museo Nacional de Ciencias Naturales, CSIC, Biodiversity and Evolutionary Group, C/ José Gutiérrez Abascal, 2. 28006, Madrid, Spain E-mail addresses: SP: [email protected] (Corresponding author) ID: [email protected] MCS: [email protected] 1 ABSTRACT Southern Iberian freshwater ecosystems located at the border between the European and African plates represent a tectonically complex region spanning several geological ages, from the uplifting of the Betic Mountains in the Serravalian-Tortonian periods to the present. This area has also been subjected to the influence of changing climate conditions since the Middle-Upper Pliocene when seasonal weather patterns were established. Consequently, the ichthyofauna of southern Iberia is an interesting model system for analyzing the influence of Cenozoic tectonic and climatic events on its evolutionary history. The cyprinids Squalius malacitanus and Squalius pyrenaicus are allopatrically distributed in southern Iberia and their evolutionary history may have been defined by Cenozoic tectonic and climatic events. We analyzed MT-CYB (510 specimens) and RAG1 (140 specimens) genes of both species to reconstruct phylogenetic relationships and to estimate divergence times and ancestral distribution ranges of the species and their populations. -
Phylogenetic Position of the Fish Genus Ellopostoma (Teleostei: Cypriniformes) Using Molecular Genetic Data
157 Ichthyol. Explor. Freshwaters, Vol. 20, No. 2, pp. 157-162, 2 figs., June 2009 © 2009 by Verlag Dr. Friedrich Pfeil, München, Germany – ISSN 0936-9902 Phylogenetic position of the fish genus Ellopostoma (Teleostei: Cypriniformes) using molecular genetic data Jörg Bohlen* and Vendula Šlechtová* We investigated the phylogenetic position of Ellopostoma based on nuclear sequence data (RAG-1 gene). Ellopo- stoma is a member of the superfamily Cobitoidea (loaches) of Cypriniformes, but does not belong to any of the currently recognised families. It represents an independent lineage, recognised as a distinct new family Ellopo- stomatidae, characterized by a squarish and oblique snout, a minute protrusible mouth, a single pair of barbels, large eyes and 35-38 pharyngeal teeth. Introduction middle stretches of the Kapuas River in western Borneo. It is only in 1976 that the species was With about 3800 recognised species, the freshwa- collected again, also in the Kapuas (Roberts, 1989). ter fish order Cypriniformes (Osteichthyes: Tele- Kottelat (1989) recorded the presence of an un- ostei) is one of the largest recognised to date named Ellopostoma from the Malay Peninsula among vertebrates. It is divided into two main [Tapi River, Thailand], later described by Tan & lineages, the superfamilies Cyprinoidea (carps, Lim (2002) as E. mystax. Kottelat & Widjanarti minnows and related fishes) and Cobitoidea (2005) provide additional records of E. megalo- (loaches and related fishes) (Nelson, 2006). With- mycter, also in the Kapuas drainage. in Cobitoidea seven lineages are recognizable Because of its unique morphological features, (called families by e. g., Šlechtová et al., 2007; Chen the phylogenetic position of Ellopostoma has been & Mayden, 2009). -
Iberian Cyprinids: Habitat Requirements and Vulnerabilities
Session 1 Cyprinid species: ecology and constraints Iberian cyprinids: habitat requirements and vulnerabilities 2nd Stakeholder Workshop – Iberia – Lisboa, Portugal 20.03.2019 Francisco Godinho Hidroerg 20/03/2018 Francisco Godinho 1 Setting the scene 22/03/2018 Francisco Godinho 2 Relatively small river catchmentsIberian fluvial systems Douro/Duero is the largest one, with 97 600 km2 Loire – 117 000 km2, Rhinne -185 000 km2, Vistula – 194 000 km2, Danube22/03/2018 – 817 000 km2, Volga –Francisco 1 380 Godinho 000 km2 3 Most Iberian rivers present a mediterranean hydrological regime (temporary rivers are common) Vascão river, a tributary of the Guadiana river 22/03/2018 Francisco Godinho 4 Cyprinidae are the characteristic fish taxa of Iberian fluvial ecosystems, occurring from mountain streams (up to 1000 m in altitude) to lowland rivers Natural lakes are rare in the Iberian Peninsula and most natural freshwater bodies are rivers and streams 22/03/2018 Francisco Godinho 5 Six fish-based river types have been distinguished in Portugal (INAG and AFN, 2012) Type 1 - Northern salmonid streams Type 2 - Northern salmonid–cyprinid trans. streams Type 3 - Northern-interior medium-sized cyprinid streams Type 4 - Northern-interior medium-sized cyprinid streams Type 5 - Southern medium-sized cyprinid streams Type 6 - Northern-coastal cyprinid streams With the exception of assemblages in small northern, high altitude streams, native cyprinids dominate most unaltered fish assemblages, showing a high sucess in the hidrological singular 22/03/2018 -
Bivalves Nas Lagoas Costeiras Da Barrinha De Mira, Lagoa De Mira, Lagoa Da Vela E Lagoa Das Braças
Caracterização e proteção das espécies nativas de bivalves nas lagoas costeiras da Barrinha de Mira, Lagoa de Mira, Lagoa da Vela e Lagoa das Braças FICHA TÉCNICA título PAELORIS: Guia de Flora e Fauna aquáticas das Lagoas Costeiras do Sítio Rede Natura 2000: Dunas de Mira, Gândara e Gafanhas (código PTCON0055) autores Simone Varandas; Tiago Assunção; Paulo Almeida; Rui Cortes; António Crespí; Pedro Ferreira; Elsa Froufe; Mariana Hinzmann; Joaquim Jesus; Manuel Lopes-Lima; Marisa Lopes; Marco Magalhães; Vítor Pereira; Cátia Santos; Ronaldo Sousa; Amílcar Tei- xeira edição Instituto Politécnico de Bragança Universidade de Trás-os-Montes e Alto Douro Universidade do Minho Universidade do Porto Câmara Municipal da Figueira da Foz Câmara Municipal de Mira fotografias Adriana Terrível, Amílcar Teixeira, Ana Gonçalves, António Crespí, Carlos Antunes, João Carrola, Luís Quinta, Manuel Lopes-Lima, Nuno Rico, Ronaldo Sousa, Rui Cortes, Simone Varandas, Tiago Assunção ilustrações/esquemas Joana Rodrigues | Emanuel Peres conceção e design gráfico Tiago Assunção | Vítor Pereira design gráfico da capa Marco Custódio e contracapa impressão Nome da empresa tiragem 1000 exemplares d. l. 352…/14 isbn 978-972-745-172-2 citação da obra varandas s., assunção t., almeida p., cortes r., crespí a., ferreira p., froufe e., hinzmann m., jesus j., lopes- lima m., lopes m., magalhães m., pereira v., santos c., sousa r., teixeira a. 2014. PAELORIS: Guia de Flora e Fauna aquáticas das Lagoas Costeiras do Sítio Rede Natura 2000: Dunas de Mira, Gândara e Gafanhas (código -
Rutilus Rutilus Linnaeus, 1758. Rutilo EXÓTICA
Atlas y Libro Rojo de los Peces Continentales de España ESPECIE Rutilus rutilus Linnaeus, 1758. Rutilo EXÓTICA ºo'" o< Q DESCRIPCIÓN Es una especie de talla media que no suele sobrepasar los 40 cm de longitud total aunque se co nocen individuos que han alcanzado los 50 cm de longitud y cerca de los 2 kg de peso. Su cuerpo es alto y comprimido lateralmente, con una cabeza pequeña que representa el 25% de la longitud del cuerpo. La aleta dorsal presenta de 9-11 radios blandos y es alta y de perfil cóncavo. La aleta anal es larga con 9-11 radios blandos. Las escamas son grandes y su número en la línea lateral es de 40-45. Sin dientes mandibulares o maxilares los dientes faríngeos se disponen en una fila en nú mero de 5-5. El número de cromosomas es 2n=50, en algunas poblaciónes es 2n=52. Clase: Actinopterygii Orden: Cypriniformes Familia: Cyprinidae Sinonimias: Cyprinus rutilus Linnaeus, 1758. Leuciscus rutilus (Linnaeus, 1758). Rutilus rutilus (Linnaeus, 1758). Cyprinus ruttilus Linnaeus, 1758. Cyprinus ruhellio Leske, 1774. Cyprinus simus Hermann, 1804. Cyprinus lacustris Pallas, 1814. Cy prinus jaculus ]urine, 1825. Leuciscus decipiens Agassiz, 1835. Leuciscus prasinus Agassiz, 1835. Cyprinus fulvus Vallot, 1837. Cyprinus xanthopterus Vallot, 1837. Rutilus heckelii (Nordmann, 1840). Leuciscus heckelii Nordmann, 1840. Leucos ce nisophius Bonaparte, 1841. Gardonus pigulus Bonaparte, 1841. Leuciscus rutiloides Selys-Longchamps, 1842. Leuciscus sely sii Selys-Longchamps, 1842. Leuciscus lividus Heckel, 1843. Leuciscus pausingeri Heckel, 1843. Leucos pigulus Bonaparte, 1844. Leucos cenisophius Bonaparte, 1845. Leuciscus jurinii Dybowski, 1862. Leuciscus rutilus daugawensis Dybowski, 1862. -
Population Genomics Data Supports Introgression Between Western Iberian
bioRxiv preprint doi: https://doi.org/10.1101/585687; this version posted March 22, 2019. The copyright holder for this preprint (which was not certified by peer review) is the author/funder, who has granted bioRxiv a license to display the preprint in perpetuity. It is made available under aCC-BY-NC-ND 4.0 International license. 1 Population genomics data supports introgression between Western Iberian 2 Squalius freshwater fish species from different drainages 3 4 Sofia L. Mendes1, Maria M. Coelho1†, Vitor C. Sousa1†* 5 1 cE3c – Centre for Ecology, Evolution and Environmental Changes, Departamento de 6 Biologia Animal, Faculdade de Ciências da Universidade de Lisboa, Campo Grande, 7 1749-016 Lisbon, Portugal 8 † equal contribution 9 *corresponding authors: [email protected] and [email protected] 1 bioRxiv preprint doi: https://doi.org/10.1101/585687; this version posted March 22, 2019. The copyright holder for this preprint (which was not certified by peer review) is the author/funder, who has granted bioRxiv a license to display the preprint in perpetuity. It is made available under aCC-BY-NC-ND 4.0 International license. 10 Abstract 11 12 In freshwater fish, processes of population divergence and speciation are often linked 13 to the geomorphology of rivers and lakes that create barriers isolating populations. 14 However, current geographical isolation does not necessarily imply total absence of 15 gene flow during the divergence process. Here, we focused on four species of the 16 genus Squalius in Portuguese rivers: S. carolitertii, S. pyrenaicus, S. aradensis and S. 17 torgalensis. Previous studies based on eight nuclear and mitochondrial markers 18 revealed incongruent patterns, with nuclear loci suggesting that S. -
Population Genetic Structure of Indigenous Ornamental Teleosts, Puntius Denisonii and Puntius Chalakkudiensis from the Western Ghats, India
POPULATION GENETIC STRUCTURE OF INDIGENOUS ORNAMENTAL TELEOSTS, PUNTIUS DENISONII AND PUNTIUS CHALAKKUDIENSIS FROM THE WESTERN GHATS, INDIA Thesis submitted in partial fulfillment of the requirement for the Degree of Doctor of Philosophy in Marine Sciences of the Cochin University of Science and Technology Cochin – 682 022, Kerala, India by LIJO JOHN (Reg. No. 3100) National Bureau of Fish Genetic Resources Cochin Unit CENTRAL MARINE FISHERIES RESEARCH INSTITUTE (Indian Council of Agricultural Research) P.B. No. 1603, Kochi – 682 018, Kerala, India. December, 2009. Declaration I do hereby declare that the thesis entitled “Population genetic structure of indigenous ornamental teleosts, Puntius denisonii and Puntius chalakkudiensis from the Western Ghats, India” is the authentic and bonafide record of the research work carried out by me under the guidance of Dr. A. Gopalakrishnan, Principal Scientist and SIC, National Bureau of Fish Genetic Resources (NBFGR) Cochin Unit, Central Marine Fisheries Research Institute, Cochin in partial fulfillment for the award of Ph.D. degree under the Faculty of Marine Sciences of Cochin University of Science and Technology, Cochin and no part thereof has been previously formed the basis for the award of any degree, diploma, associateship, fellowship or other similar titles or recognition. Cochin (Lijo John) 16th December 2009 ®É¹]ÅÒªÉ ¨ÉiºªÉ +ÉxÉÖÖ´ÉÆÆÎ¶ÉE ºÉÆÆºÉÉvÉxÉ ¤ªÉÚ®Éä NATIONAL BUREAU OF FISH GENETIC RESOURCES NBFGR Cochin Unit, CMFRI Campus, P.B. No. 1603, Cochin-682 018, Kerala, India Fax: (0484) 2395570; E-mail: [email protected] Dr. A. Gopalakrishnan, Date: 16.12.2009 Principal Scientist, Officer-in-Charge & Supervising Teacher Certificate This is to certify that this thesis entitled, “Population genetic structure of indigenous ornamental teleosts, Puntius denisonii and Puntius chalakkudiensis from the Western Ghats, India” is an authentic record of original and bonafide research work carried out by Mr. -
Sabanejewia Balcanica ) in River Sava )* +
C ! " # " $"% A. Bajrić et al.: Balkan golden loach ( Sabanejewia balcanica ) in river Sava )* + ,-. /012 34 ,*) /4* ** 5 D&'( CODEN RIBAEG ISSN 1330-061X (print), 1848-0586 (online) BODY PIGMENTATION AND MERISTIC CHARACTERISTICS OF BALKAN GOLDEN LOACH ( Sabanejewia balcanica ) FROM THE WATER CATCHMENT AREA OF THE RIVER SAVA Alen Bajrić1*, Avdul Adrović1, Edina Hajdarević1, Isat Skenderović1, Eldar Tanović 2 1University of Tuzla, Faculty of Natural Sciences and Mathematics. Univerzitetska 4, Tuzla, BiH 2J. P. Elektroprivreda BiH (Public Enterprise Electric Utility of Bosnia and Herzegovina), Mije Keroševića Guje br.1, Tuzla, BiH *Corresponding Author, Email: [email protected] ¢¦§¨ A ¡ ¢£ ¤¥ ABSTRACT Received: 27 October 2017 The taxonomic research of eight populations of Sabanejewia balcanica Received in revised form: 15 December 2017 from the water catchment area of the river Sava included the analysis of Accepted: 10 January 2018 the meristic characteristics and pigmentation of their bodies. The analyzed Available online: 21 February 2018 sample contained 98 specimens collected in the areas of the rivers Turija, Gostelja, Brka, Suturlija, Suha, Tinja and Trebačka. The aim of this paper was to specify the meristic and pigment characteristics of this species at the location of the water catchment area of the river Sava, which are frequently used in describing new taxa. The meristic traits investigation included the analysis of the number of rays in dorsal, ventral, pectoral, anal and caudal Keywords: fins. In the analysis of body pigmentation, the number and arrangement Cobitidae of suborbital spines, blotches or spots were determined. The results of Rays this research indicated that there were no intrapopulation differences in Fins Sabanejewia balcanica from the water catchment area of the river Sava in Intrapopulation differences terms of meristic characteristic and body pigmentation. -
Polish Center for Education. Conference in Augustów 13 - 15 June 2019
Polish Center for Education. Conference in Augustów 13 - 15 June 2019 Polish Center for Education. Conference in Augustów 13 - 15 June 2019 Aneta Trzcińska (BA EDTNA/ERCA) was asked to talk about the Occupational Exposure by the Polish Center for Education. She gave a speech in Augustów, despite the fact that it is a journey of almost 600 kilometers. Aneta spoke about safety at work, occupational exposure and promoted EDTNA/ERCA. After the conference, participants rated the speakers. Aneta Trzcińska received an average grade of 5.5 on a six-point scale. EDTNA/ERCA Secretariat Källstorps Gård / Högs Byväg 118 / SE-246 55 Löddeköpinge / Sweden Phone: +46 46 70 96 24 / e-mail: [email protected] www.edtnaerca.org EDTNA/ERCA Seestrasse 91, CH 6052 Hergiswil, Switzerland Polish Center for Education. Conference in Augustów 13 - 15 June 2019 AUGUSTÓW The town, although small, has many attractions for the visitors. The Oficerski Yacht Club Hotel, built in the 1930s, is an army yacht club that has been restored and converted into a resort. It is located on the edge of one of many lakes in the region. Pope John Paul II has a memorial chair from the first and last time he visited the town right outside the club. Boat tours are also popular and the old town square still has its original cobblestone streets. Every year hundreds of bikers come to Augustowskie Motonoce bikers festival. Official event Facebook page: Augustowskie Motonoce. Bands that over years participated in celebration include Bright Ophidia, AGE, ZZ Top Czech Revival Band, AC/DC Show Ukraina, Kraków Street Band, etc.