“REAL World Data” Using RDB and Hadoop
Total Page:16
File Type:pdf, Size:1020Kb
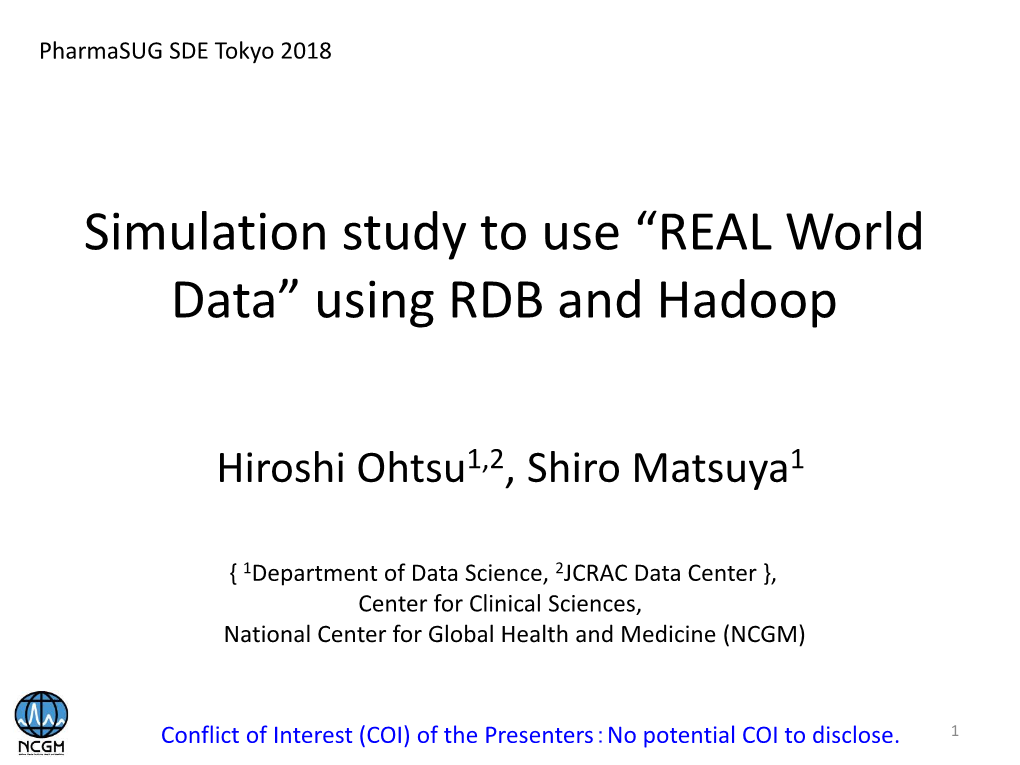
Load more
Recommended publications
-
Treatment of Psychosis: 30 Years of Progress
Journal of Clinical Pharmacy and Therapeutics (2006) 31, 523–534 REVIEW ARTICLE Treatment of psychosis: 30 years of progress I. R. De Oliveira* MD PhD andM.F.Juruena MD *Department of Neuropsychiatry, School of Medicine, Federal University of Bahia, Salvador, BA, Brazil and Department of Psychological Medicine, Institute of Psychiatry, King’s College, University of London, London, UK phrenia. Thirty years ago, psychiatrists had few SUMMARY neuroleptics available to them. These were all Background: Thirty years ago, psychiatrists had compounds, today known as conventional anti- only a few choices of old neuroleptics available to psychotics, and all were liable to cause severe extra them, currently defined as conventional or typical pyramidal side-effects (EPS). Nowadays, new antipsychotics, as a result schizophrenics had to treatments are more ambitious, aiming not only to suffer the severe extra pyramidal side effects. improve psychotic symptoms, but also quality of Nowadays, new treatments are more ambitious, life and social reinsertion. We briefly but critically aiming not only to improve psychotic symptoms, outline the advances in diagnosis and treatment but also quality of life and social reinsertion. Our of schizophrenia, from the mid 1970s up to the objective is to briefly but critically review the present. advances in the treatment of schizophrenia with antipsychotics in the past 30 years. We conclude DIAGNOSIS OF SCHIZOPHRENIA that conventional antipsychotics still have a place when just the cost of treatment, a key factor in Up until the early 1970s, schizophrenia diagnoses poor regions, is considered. The atypical anti- remained debatable. The lack of uniform diagnostic psychotic drugs are a class of agents that have criteria led to relative rates of schizophrenia being become the most widely used to treat a variety of very different, for example, in New York and psychoses because of their superiority with London, as demonstrated in an important study regard to extra pyramidal symptoms. -
Quantitative Resting State Electroencephalography in Patients with Schizophrenia Spectrum Disorders Treated with Strict Monotherapy Using Atypical Antipsychotics
Original Article https://doi.org/10.9758/cpn.2021.19.2.313 pISSN 1738-1088 / eISSN 2093-4327 Clinical Psychopharmacology and Neuroscience 2021;19(2):313-322 Copyrightⓒ 2021, Korean College of Neuropsychopharmacology Quantitative Resting State Electroencephalography in Patients with Schizophrenia Spectrum Disorders Treated with Strict Monotherapy Using Atypical Antipsychotics Takashi Ozaki1,2, Atsuhito Toyomaki1, Naoki Hashimoto1, Ichiro Kusumi1 1Department of Psychiatry, Graduate School of Medicine, Hokkaido University, 2Department of Psychiatry, Hokkaido Prefectural Koyogaoka Hospital, Sapporo, Japan Objective: The effect of antipsychotic drugs on quantitative electroencephalography (EEG) has been mainly examined by the administration of a single test dose or among patients using combinations of other psychotropic drugs. We therefore investigated the effects of strict monotherapy with antipsychotic drugs on quantitative EEG among schizophrenia patients. Methods: Data from 2,364 medical reports with EEG results from psychiatric patients admitted to the Hokkaido University Hospital were used. We extracted EEG records of patients who were diagnosed with schizophrenia spectrum disorders and who were either undergoing strict antipsychotic monotherapy or were completely free of psychotropic drugs. The spectral power was compared between drug-free patients and patients using antipsychotic drugs. We also performed multiple regression analysis to evaluate the relationship between spectral power and the chlorpromazine equivalent daily dose of antipsychotics in all the patients. Results: We included 31 monotherapy and 20 drug-free patients. Compared with drug-free patients, patients receiving antipsychotic drugs demonstrated significant increases in theta, alpha and beta power. When patients taking different types of antipsychotics were compared with drug-free patients, we found no significant change in any spectrum power for the aripiprazole or blonanserin groups. -
New Zealand Data Sheet 1. Product Name
NEW ZEALAND DATA SHEET 1. PRODUCT NAME Norvir 100 mg tablets. 2. QUALITATIVE AND QUANTITATIVE COMPOSITION Each film-coated tablet contains 100 mg ritonavir. For the full list of excipients, see section 6.1. 3. PHARMACEUTICAL FORM White film-coated oval tablets debossed with the corporate Abbott "A" logo and the Abbott-Code “NK”. 4. CLINICAL PARTICULARS 4.1 Therapeutic indications NORVIR is indicated for use in combination with appropriate antiretroviral agents or as monotherapy if combination therapy is inappropriate, for the treatment of HIV-1 infection in adults and children aged 12 years and older. For persons with advanced HIV disease, the indication for ritonavir is based on the results for one study that showed a reduction in both mortality and AIDS defining clinical events for patients who received ritonavir. Median duration of follow-up in this study was 6 months. The clinical benefit from ritonavir for longer periods of treatment is unknown. For persons with less advanced disease, the indication is based on changes in surrogate markers in controlled trials of up to 16 weeks duration (see section 5.1 Pharmacodynamic properties). 4.2 Dose and method of administration General Dosing Guidelines Prescribers should consult the full product information and clinical study information of protease inhibitors if they are co-administered with a reduced dose of ritonavir. The recommended dose of NORVIR is 600 mg (six tablets) twice daily by mouth, and should be given with food. NORVIR tablets should be swallowed whole and not chewed, broken or crushed. NORVIR DS 29 April 2020 Page 1 of 37 Version 35 Paediatric population Ritonavir has not been studied in patients below the age of 12 years; hence the safety and efficacy of ritonavir in children below the age of 12 have not been established. -
A Study on the Efficacy and Safety of Blonanserin in Indian Patients in Ahmedabad: a Randomized, Active Controlled, Phase III Clinical Trial
Lakdawala et al. : Efficacy and Safety of Blonanserin 359 Original Research Article A study on the efficacy and safety of Blonanserin in Indian patients in Ahmedabad: a randomized, active controlled, phase III clinical trial Bhavesh Lakdawala1, Mahemubin S. Lahori2, Ganpat K. Vankar3 1Associate Professor, Dept. of Psychiatry, AMC-MET Medical College and Sheth L.G. General Hospital, Ahmedabad, Gujarat, India. 2Assistant Professor, Dept. of Psychiatry, GMERS Medical College and General Hospital, Sola, Gujarat, India. 3Professor, Dept. of Psychiatry, Jawaharlal Nehru Medical College and Acharya Vinoba Bhave Rural Hospital Wardha, Maharashtra Corresponding author: Dr. Mahemubin Lahori Email – [email protected] ABSTRACT Introduction: Blonanserin is a novel atypical antipsychotic with higher dopamine D2 receptor occupancy and lower serotonin 5-HT2A receptor blocking activity as compared to the other atypical antipsychotics. The objective of this study was to compare the efficacy and safety of blonanserin with haloperidol in Indian patients with schizophrenia. Methodology: This was an 8 week, randomized, open label, active controlled, multicentre study. Patients diagnosed with schizophrenia according to the DSM-IV criteria were enrolled in the study. Patients were randomized to receive either blonanserin (16 mg/day) or haloperidol (4.5 mg/day). Patients were assessed on an out-patient basis after every 2 weeks for clinical efficacy [Positive and Negative Syndrome Scale (PANSS) total and factor scores], Clinical Global Impressions–severity CGI-S, Clinical Global Impressions–Improvement (CGI-I), Global Assessment of Efficacy (CGI-C), adverse events and drug compliance. Results: At our centre, total 60 patients were randomized in the study with 30 patients each in Blonanserin and Haloperidol group. -
Project of Japan Drug Information Institute in Pregnancy
Pharmaceuticals and Medical Devices Safety Information No. 268 April 2010 Table of Contents 1. Manuals for Management of Individual Serious Adverse Drug Reactions ....................................................................................................... 4 2. Project of Japan Drug Information Institute in Pregnancy ........... 9 3. Important Safety Information ................................................................. 11 .1. Atorvastatin Calcium Hydrate, Simbastatin, Pitavastatin Calcium, Pravastatin Sodium, Fluvastatin Sodium, Rosuvastatin Calcium, Amlodipine Besilate/Atorvastatin Calcium Hydrate ·································································································· 11 .2. Cetuximab (Genetical Recombination) ·························································· 16 4. Revision of PRECAUTIONS (No. 215) Aripiprazole (and 6 others)........................................................................................22 5. List of products subject to Early Post-marketing Phase Vigilance ............................................... 25 This Pharmaceuticals and Medical Devices Safety Information (PMDSI) is issued based on safety information collected by the Ministry of Health, Labour and Welfare. It is intended to facilitate safer use of pharmaceuticals and medical devices by healthcare providers. PMDSI is available on the Pharmaceuticals and Medical Devices Agency website (http://www.pmda.go.jp/english/index.html) and on the MHLW website (http://www.mhlw.go.jp/, only available in Japanese -
Effect of Antipsychotics on Breast Tumors by Analysis of the Japanese
Maeshima et al. Journal of Pharmaceutical Health Care and Sciences (2021) 7:13 https://doi.org/10.1186/s40780-021-00199-7 RESEARCH ARTICLE Open Access Effect of antipsychotics on breast tumors by analysis of the Japanese Adverse Drug Event Report database and cell-based experiments Tae Maeshima1, Ryosuke Iijima 2, Machiko Watanabe1, Satoru Yui2 and Fumio Itagaki1* Abstract Background: Since antipsychotics induce hyperprolactinemia via the dopamine D2 receptor, long-term administration may be a risk factor for developing breast tumors, including breast cancer. On the other hand, some antipsychotic drugs have been reported to suppress the growth of breast cancer cells in vitro. Thus, it is not clear whether the use of antipsychotics actually increases the risk of developing or exacerbating breast tumors. The purpose of this study was to clarify the effects of antipsychotic drugs on the onset and progression of breast tumors by analyzing an adverse event spontaneous reporting database and evaluating the proliferation ability of breast cancer cells. Methods: Japanese Adverse Drug Event Report database (JADER) reports from April 2004 to April 2019 were obtained from the Pharmaceuticals and Medical Devices Agency (PMDA) website. Reports of females only were analyzed. Adverse events included in the analysis were hyperprolactinemia and 60 breast tumor-related preferred terms. The reporting odds ratio (ROR), proportional reporting ratio (PRR), and information component (IC) were used to detect signals. Furthermore, MCF-7 cells were treated with haloperidol, risperidone, paliperidone, sulpiride, olanzapine and blonanserin, and cell proliferation was evaluated by WST-8 assay. Results: In the JADER analysis, the IC signals of hyperprolactinemia were detected with sulpiride (IC, 3.73; 95% CI: 1.81–5.65), risperidone (IC, 3.69; 95% CI: 1.71–5.61), and paliperidone (IC, 4.54; 95% CI: 2.96–6.12). -
Molecular Targets of Atypical Antipsychotics: from Mechanism of Action to Clinical Differences
View metadata, citation and similar papers at core.ac.uk brought to you by CORE provided by Queen Mary Research Online JPT-07243; No of Pages 23 Pharmacology & Therapeutics xxx (2018) xxx–xxx Contents lists available at ScienceDirect Pharmacology & Therapeutics journal homepage: www.elsevier.com/locate/pharmthera Molecular targets of atypical antipsychotics: From mechanism of action to clinical differences Stefano Aringhieri a, Marco Carli a, Shivakumar Kolachalam a, Valeria Verdesca a, Enrico Cini a, Mario Rossi b, Peter J. McCormick c, Giovanni U. Corsini a, Roberto Maggio d, Marco Scarselli a,⁎ a Department of Translational Research and New Technologies in Medicine and Surgery, University of Pisa, Italy b Institute of Molecular Cell and Systems Biology, University of Glasgow, UK c William Harvey Research Institute, Barts and the London School of Medicine, Queen Mary University of London, London EC1M 6BQ, UK d Biotechnological and Applied Clinical Sciences Department, University of L'Aquila, Italy article info abstract Keywords: The introduction of atypical antipsychotics (AAPs) since the discovery of its prototypical drug clozapine has been Atypical antipsychotics a revolutionary pharmacological step for treating psychotic patients as these allow a significant recovery not only Clozapine in terms of hospitalization and reduction symptoms severity, but also in terms of safety, socialization and better Monoamine receptors rehabilitation in the society. Regarding the mechanism of action, AAPs are weak D2 receptor antagonists and they Dimerization act beyond D2 antagonism, involving other receptor targets which regulate dopamine and other neurotransmit- Biased agonism ters. Consequently, AAPs present a significant reduction of deleterious side effects like parkinsonism, Therapeutic drug monitoring hyperprolactinemia, apathy and anhedonia, which are all linked to the strong blockade of D2 receptors. -
Fatal Cases Where a Causal Relationship with XEPLION Is Unknown Have Been Reported During the Period of Early Phase Pharmacovigilance
■ Fatal cases where a causal relationship with XEPLION is unknown have been reported during the period of early phase pharmacovigilance. As of April 15th 2014, 14 fatal cases have been granted permission to disclose by the reporting physicians as follows. No ADR Age Gen Pre-administration of XEPLION Latency Medical history Concomitant (MedDRA PT) der Paliperidone or Dose antipsychotic(s) Risperidone/Dose (At first injection) Aripiprazole tablet, Risperidone sustained-release 1 Death 50s F 100 mg 3 days Constipation Quetiapine fumarate suspension for injection/50mg tablet Pulmonary embolism Right ventricular failure Risperidone sustained-release Hyperuricaemia, 2 50s M 150 mg 41 days Olanzapine tablet Rhabdomyolysis suspension for injection/50mg Hepatic steatosis Psychiatric symptom Risperidone sustained-release Quetiapine fumarate 3 Death 60s M 25 mg 14 days Unknown suspension for injection/25mg tablet Hepatitis C, Risperidone sustained-release Hypertension, 4 Death 50s M 75 mg 40 days Zotepine tablet suspension for injection/25mg Abnormal hepatic function Acute myocardial Risperidone oral solution Hyperuricaemia, Aripiprazole tablet, 5 30s M 150 mg 4 days infarction /4mg Obesity Olanzapine tablet Death, Palpitations Hyperglycaemia, 6 50s M None 50 mg 13 days Olanzapine tablet Dyspnoea, Dysarthria Asthma, Back pain Hypothermia Risperidone sustained-release 7 40s M 50 mg 42 days Hyperuricaemia Risperidone tablet Stress fracture suspension for injection/25mg Haloperidol decanoate Mild mental injection, Olanzapine 8 Death 30s M None 150 mg -
A Randomized, Prospective Comparative Study to Evaluate
A RANDOMIZED, PROSPECTIVE COMPARATIVE STUDY TO EVALUATE THE EFFICACY AND SAFETY OF LURASIDONE AND RISPERIDONE IN TREATMENT OF SCHIZOPHRENIA Dissertation Submitted To THE TAMILNADU DR.M.G.R. MEDICAL UNIVERSITY IN PARTIAL FULFILLMENT FOR THE AWARD OF THE DEGREE OF DOCTOR OF MEDICINE IN PHARMACOLOGY DEPARTMENT OF PHARMACOLOGY TIRUNELVELI MEDICAL COLLEGE TIRUNELVELI -11 MAY -2019 BONAFIDE CERTIFICATE This is to certify that the dissertation entitled “A RANDOMIZED, PROSPECTIVE COMPARATIVE STUDY TO EVALUATE THE EFFICACY AND SAFETY OF LURASIDONE AND RISPERIDONE IN TREATMENT OF SCHIZOPHRENIA ” submitted by DR.T.BABY PON ARUNA to the Tamilnadu Dr. M.G.R Medical University, Chennai, in partial fulfillment of the requirement for the award of the Degree of Doctor of Medicine in Pharmacology during the academic period 2016-2019 is a bonafide research work carried out by her under direct supervision & guidance. PROFESSOR AND H.O.D DEAN Department of Pharmacology, Tirunelveli Medical college, Tirunelveli Medical college, Tirunelveli. Tirunelveli. CERTIFICATE This is to certify that the dissertation entitled “A RANDOMIZED, PROSPECTIVE COMPARATIVE STUDY TO EVALUATE THE EFFICACY AND SAFETY OF LURASIDONE AND RISPERIDONE IN TREATMENT OF SCHIZOPHRENIA ” submitted by DR.T.BABY PON ARUNA is an original work done by her in the Department of Pharmacology, Tirunelveli Medical college, Tirunelveli for the award of the Degree of Doctor of Medicine in Pharmacology during the academic period 2016-2019. Place: Tirunelveli GUIDE Date: Department of Pharmacology, Tirunelveli Medical college, Tirunelveli. DECLARATION I solemnly declare that the dissertation titled “A RANDOMIZED, PROSPECTIVE COMPARATIVE STUDY TO EVALUATE THE EFFICACY AND SAFETY OF LURASIDONE AND RISPERIDONE IN TREATMENT OF SCHIZOPHRENIA” is done by me in the Department of Pharmacology, Tirunelveli Medical College, Tirunelveli. -
Dystonia Secondary to Use of Antipsychotic Agents
5 Dystonia Secondary to Use of Antipsychotic Agents Nobutomo Yamamoto and Toshiya Inada Seiwa Hospital, Institute of Neuropsychiatry, Tokyo, Japan 1. Introduction Following the introduction of first-generation antipsychotics (FGAs) in the early 1950s, there was a radical change in the therapeutic regimens for schizophrenia. However, it soon became apparent that these antipsychotic agents produced serious side effects including distressing and often debilitating movement disorders known as extrapyramidal symptoms (EPS). To prevent EPS, second-generation antipsychotics (SGAs) were developed and introduced, including risperidone in 1996, quetiapine, perospirone, and olanzapine in 2001, aripiprazole in 2006, and blonanserin in 2008. Clozapine was approved in 2010 in Japan with strict regulation of its use. These newer medications differ from FGAs, primarily on the basis of their reduced risk of inducing EPS. EPS lie at the interface of neurology and psychiatry and have generated a vast literature in both disciplines. EPS can be categorized as acute (dystonia, akathisia and parkinsonism) and tardive (tardive dyskinesia, tardive akathisia and tardive dystonia). Acute EPS has often been reported as an early sign of predisposition to tardive dyskinesia. Acute and tardive EPS may also adversely influence a patient’s motor and mental performance and reduce compliance to treatment. Poor compliance leads to high relapse rates, with both ethical and economic consequences. Acute dystonic reaction is a common side effect of antipsychotics, but can be caused by any agents that block dopamine receptors, such as the antidepressant amoxapine and anti-emetic drugs such as metoclopramide. Dystonia is characterized by prolonged muscle contraction provoking slow, repetitive, involuntary, often twisting, movements that result in sustained abnormal, and at times bizarre, postures, which eventually become fixed. -
Molecular Targets of Atypical Antipsychotics: from Mechanism of Action to Clinical Differences
JPT-07243; No of Pages 23 Pharmacology & Therapeutics xxx (2018) xxx–xxx Contents lists available at ScienceDirect Pharmacology & Therapeutics journal homepage: www.elsevier.com/locate/pharmthera Molecular targets of atypical antipsychotics: From mechanism of action to clinical differences Stefano Aringhieri a, Marco Carli a, Shivakumar Kolachalam a, Valeria Verdesca a, Enrico Cini a, Mario Rossi b, Peter J. McCormick c, Giovanni U. Corsini a, Roberto Maggio d, Marco Scarselli a,⁎ a Department of Translational Research and New Technologies in Medicine and Surgery, University of Pisa, Italy b Institute of Molecular Cell and Systems Biology, University of Glasgow, UK c William Harvey Research Institute, Barts and the London School of Medicine, Queen Mary University of London, London EC1M 6BQ, UK d Biotechnological and Applied Clinical Sciences Department, University of L'Aquila, Italy article info abstract Keywords: The introduction of atypical antipsychotics (AAPs) since the discovery of its prototypical drug clozapine has been Atypical antipsychotics a revolutionary pharmacological step for treating psychotic patients as these allow a significant recovery not only Clozapine in terms of hospitalization and reduction symptoms severity, but also in terms of safety, socialization and better Monoamine receptors rehabilitation in the society. Regarding the mechanism of action, AAPs are weak D2 receptor antagonists and they Dimerization act beyond D2 antagonism, involving other receptor targets which regulate dopamine and other neurotransmit- Biased agonism ters. Consequently, AAPs present a significant reduction of deleterious side effects like parkinsonism, Therapeutic drug monitoring hyperprolactinemia, apathy and anhedonia, which are all linked to the strong blockade of D2 receptors. This review revisits previous and current findings within the class of AAPs and highlights the differences in terms of receptor properties and clinical activities among them. -
Psychiatry ADVISOR November 22, 2019 Rationale Behind The
Psychiatry ADVISOR November 22, 2019 Rationale Behind the Transdermal Delivery of Antipsychotic Medications Sheila Jacobs The transdermal drug route could potentially increase treatment compliance in patients with schizophrenia. Future advancements in delivery technologies, along with their evaluation in clinical trials, might help to broaden the clinical use of transdermal drug formulations for the treatment of psychiatric disorders. The current study was designed to evaluate progress regarding the use of transdermal antipsychotic drug formulations by conducting a search of papers, patents, and clinical trials that have been published on the topic in the last 10 years. Results of the analysis were published in CNS Drugs. The investigators sought to offer a rationale for the development of transdermal formulations of antipsychotic agents that are currently on the market. Data on antipsychotic transdermal formulations are reported and discussed, with the focus of this analysis being on the characteristics of the dosage forms in use, along with their ability to promote drug absorption. Although a large number of antipsychotic medications are currently available, only a few of these agents (aripiprazole, asenapine, blonanserin, chlorpromazine, haloperidol, olanzapine, prochlorperazine, quetiapine, and risperidone) have actually been developed as transdermal delivery systems. According to some of the papers and patents that were evaluated, such transdermal formulations as patches, gels, creams, solutions, sprays, films, and nanosystems have