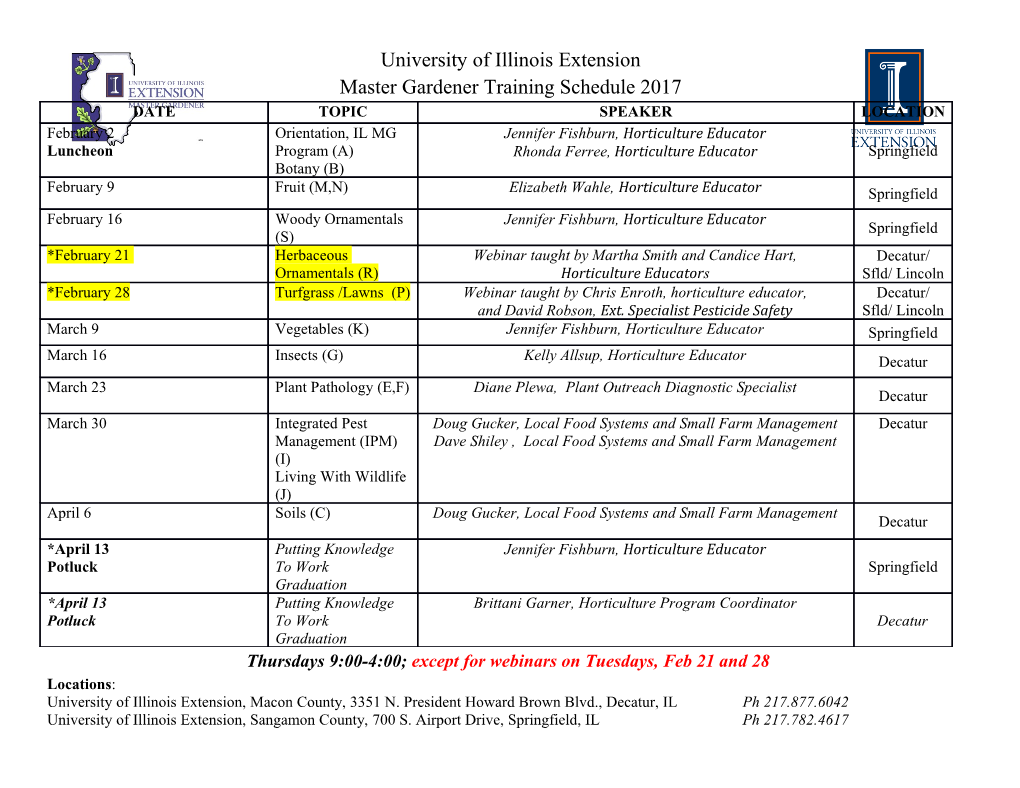
RS – Lecture 12 Lecture 12 Heteroscedasticity 1 Two-Step Estimation of the GR Model: Review • Use the GLS estimator with an estimate of 1. is parameterized by a few estimable parameters, = (θ). Example: Harvey’s heteroscedastic model. 2. Iterative estimation procedure: (a) Use OLS residuals to estimate the variance function. (b) Use the estimated in GLS - Feasible GLS, or FGLS. • True GLS estimator -1 -1 -1 bGLS = (X’Ω X) X’Ω y (converges in probability to .) • We seek a vector which converges to the same thing that this does. Call it FGLS, based on [X -1 X]-1 X-1 y 1 RS – Lecture 12 Two-Step Estimation of the GR Model: Review Two-Step Estimation of the GR Model: FGLS • Feasible GLS is based on finding an estimator which has the same properties as the true GLS. 2 Example: Var[i] = Exp(zi). True GLS: Regress yi/[ Exp((1/2)zi)] on xi/[ Exp((1/2)zi)] FGLS: With a consistent estimator of [,], say [s,c], we do the same computation with our estimates. Note: If plim [s,c] = [,], then, FGLS is as good as true GLS. • Remark: To achieve full efficiency, we do not need an efficient estimate of the parameters in , only a consistent one. 2 RS – Lecture 12 Heteroscedasticity • Assumption (A3) is violated in a particular way: has unequal variances, but i and j are still not correlated with each other. Some observations (lower variance) are more informative than others (higher variance). f(y|x) . E(y|x) = b0 + b1x . x1 x2 x3 x 5 Heteroscedasticity • Now, we have the CLM regression with hetero-(different) scedastic (variance) disturbances. (A1) DGP: y = X + is correctly specified. (A2) E[|X] = 0 2 (A3’) Var[i] = i, i > 0. (CLM => i = 1, for all i.) (A4) X has full column rank – rank(X)=k-, where T ≥ k. 2 • Popular normalization: i i = 1. (A scaling, absorbed into .) • A characterization of the heteroscedasticity: Well defined estimators and methods for testing hypotheses will be obtainable if the heteroscedasticity is “well behaved” in the sense that i / i i → 0 as T → . -i.e., no single observation becomes dominant. (1/T)i i → some stable constant. (Not a plim!) 3 RS – Lecture 12 GR Model and Testing • Implications for conventional OLS and hypothesis testing: 1. b is still unbiased. 2. Consistent? We need the more general proof. Not difficult. 3. If plim b = , then plim s2 = 2 (with the normalization). 4. Under usual assumptions, we have asymptotic normality. • Two main problems with OLS estimation under heterocedasticity: (1) The usual standard errors are not correct. (They are biased!) (2) OLS is not BLUE. • Since the standard errors are biased, we cannot use the usual t- statistics or F --statistics or LM statistics for drawing inferences. This is a serious issue. Heteroscedasticity: Inference Based on OLS • Q: But, what happens if we still use s2(XX)-1? A: It depends on XX - XX. If they are nearly the same, the OLS covariance matrix will give OK inferences. But, when will XX - XX be nearly the same? The answer is based on a property of weighted averages. Suppose i is randomly drawn from a distribution with E[i] = 1. Then, 2 p 2 2 (1/T)i i xi E[x ] --just like (1/T)i xi . • Remark: For the heteroscedasticity to be a significant issue for estimation and inference by OLS, the weights must be correlated with 2 x and/or xi . The higher correlation, heteroscedasticity becomes more important (b is more inefficient). 4 RS – Lecture 12 Finding Heteroscedasticity • There are several theoretical reasons why the i may be related to x 2 and/or xi : 1. Following the error-learning models, as people learn, their errors of 2 behavior become smaller over time. Then, σ i is expected to decrease. 2 2. As data collecting techniques improve, σ i is likely to decrease. Companies with sophisticated data processing techniques are likely to commit fewer errors in forecasting customer’s orders. 3. As incomes grow, people have more discretionary income and, thus, 2 more choice about how to spend their income. Hence, σ i is likely to increase with income. 4. Similarly, companies with larger profits are expected to show greater variability in their dividend/buyback policies than companies with lower profits. Finding Heteroscedasticity • Heteroscedasticity can also be the result of model misspecification. • It can arise as a result of the presence of outliers (either very small or very large). The inclusion/exclusion of an outlier, especially if T is small, can affect the results of regressions. • Violations of (A1) --model is correctly specified--, can produce heteroscedasticity, due to omitted variables from the model. • Skewness in the distribution of one or more regressors included in the model can induce heteroscedasticity. Examples are economic variables such as income, wealth, and education. • David Hendry notes that heteroscedasticity can also arise because of – (1) incorrect data transformation (e.g., ratio or first difference transformations). – (2) incorrect functional form (e.g., linear vs log–linear models). 5 RS – Lecture 12 Finding Heteroscedasticity • Heteroscedasticity is usually modeled using one the following specifications: 2 2 2 - H1 : σt is a function of past εt and past σt (GARCH model). 2 -H2 : σt increases monotonically with one (or several) exogenous variable(s) (x1,, . , xT ). 2 -H3 : σt increases monotonically with E(yt). 2 -H4 : σt is the same within p subsets of the data but differs across the subsets (grouped heteroscedasticity). This specification allows for structural breaks. • These are the usual alternatives hypothesis in the heteroscedasticity tests. Finding Heteroscedasticity • Visual test In a plot of residuals against dependent variable or other variable will often produce a fan shape. 180 160 140 120 100 Series1 80 60 40 20 0 050100150 12 6 RS – Lecture 12 Testing for Heteroscedasticity • Usual strategy when heteroscedasticity is suspected: Use OLS along the White estimator. This will give us consistent inferences. • Q: Why do we want to test for heteroscedasticity? A: OLS is no longer efficient. There is an estimator with lower asymptotic variance (the GLS/FGLS estimator). 2 2 2 • We want to test: H0: E(ε |x1, x2,…, xk) = E(ε ) = 2 2 2 • The key is whether E[ ] = i is related to x and/or xi . Suppose we suspect a particular independent variable, say X1, is driving i. • Then, a simple test: Check the RSS for large values of X1, and the RSS for small values of X1. This is the Goldfeld-Quandt test. Testing for Heteroscedasticity • The Goldfeld-Quandt test - Step 1. Arrange the data from small to large values of the independent variable suspected of causing heteroscedasticity, Xj. - Step 2. Run two separate regressions, one for small values of Xj and one for large values of Xj, omitting d middle observations (≈ 20%). Get the RSS for each regression: RSS1 for small values of Xj and RSS2 for large Xj’s. - Step 3. Calculate the F ratio GQ = RSS2/RSS1, ~ Fdf,df with df =[(T – d) – 2(k+1)]/2 (A5 holds). If (A5) does not hold, we have GQ is asymptoticallly χ2. 7 RS – Lecture 12 Testing for Heteroscedasticity • The Goldfeld-Quandt test Note: When we suspect more than one variable is driving the i’s, this test is not very useful. • But, the GQ test is a popular to test for structural breaks (two regimes) in variance. For these tests, we rewrite step 3 to allow for different size in the sub-samples 1 and 2. - Step 3. Calculate the F-test ratio GQ = [RSS2/ (T2 – k)]/[RSS1/ (T1 – k)] Testing for Heteroscedasticity: LR Test • The Likelihood Ratio Test Let’s define the likelihood function, assuming normality, for a general case, where we have g different variances: g g T T 1 1 ln L ln2 i ln2 (y X )(y X ) i 2 i i i i 2 i1 2 2 i1 i We have two models: 2 2 (R) Restricted under H0: i = . From this model, we calculate ln L T T ln L [ln(2) 1] ln(ˆ 2 ) R 2 2 (U) Unrestricted. From this model, we calculate the log likelihood. g T Ti 2 2 1 ln LU [ln(2) 1] lnˆ i ; ˆ i (yi X ib)(yi X ib) 2 2 Ti i1 8 RS – Lecture 12 Testing for Heteroscedasticity: LR Test • Now, we can estimate the Likelihood Ratio (LR) test: g ˆ 2 ˆ 2 a 2 LR 2(ln LU ln LR ) T ln Ti lni g1 i1 2 Under the usual regularity conditions, LR is approximated by a χ g-1. 2 • Using specific functions for i , this test has been used by Rutemiller and Bowers (1968) and in Harvey’s (1976) groupwise heteroscedasticity paper. Testing for Heteroscedasticity • Score LM tests 2 2 • We want to develop tests of H0: E(ε |x1, x2,…, xk) = against an H1 with a general functional form. 2 2 • Recall the central issue is whether E[ ] = i is related to x 2 and/or xi . Then, a simple strategy is to use OLS residuals to estimate 2 2 disturbances and look for relationships between ei and xi and/or xi . • Suppose that the relationship between ε2 and X is linear: ε2 = Xα + v Then, we test: H0: α = 0 against H1: α ≠ 0. • We can base the test on how the squared OLS residuals e correlate with X. 9 RS – Lecture 12 Testing for Heteroscedasticity • Popular heteroscedasticity LM tests: - Breusch and Pagan (1979)’s LM test (BP). - White (1980)’s general test. • Both tests are based on OLS residuals. That is, calculated under H0: No heteroscedasticity.
Details
-
File Typepdf
-
Upload Time-
-
Content LanguagesEnglish
-
Upload UserAnonymous/Not logged-in
-
File Pages47 Page
-
File Size-