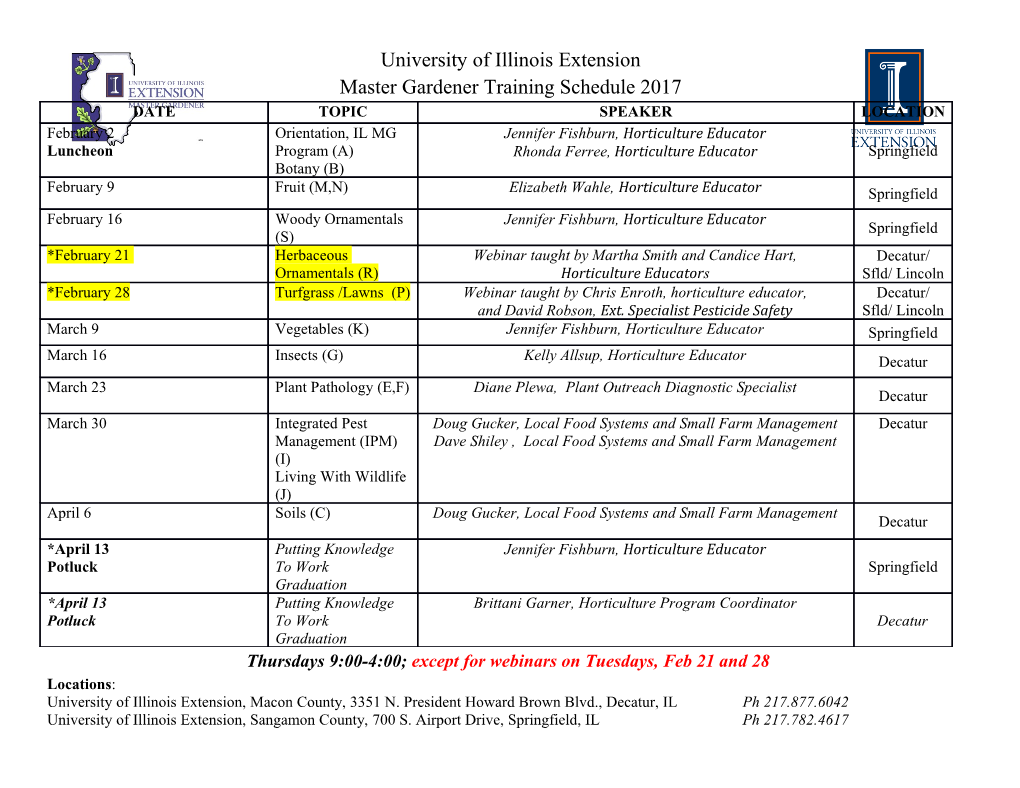
Virtual Screening on Indonesian Herbal Compounds as COVID-19 Supportive Therapy: Machine Learning and Pharmacophore Modeling Approaches Linda Erlina Universitas Indonesia Raka Indah Paramita ( [email protected] ) Universitas Indonesia https://orcid.org/0000-0002-8166-4479 Wisnu Ananta Kusuma Institut Pertanian Bogor Fakultas Matematika dan Ilmu Pengetahuan Alam Fadilah Fadilah Universitas Indonesia Aryo Tedjo Universitas Indonesia Irandi Putra Pratomo Universitas Indonesia Nabila Sekar Ramadhanti Institut Pertanian Bogor Fakultas Matematika dan Ilmu Pengetahuan Alam Ahmad Kamal Nasution Institut Pertanian Bogor Fakultas Matematika dan Ilmu Pengetahuan Alam Fadhlal Khaliq Surado Institut Pertanian Bogor Fakultas Matematika dan Ilmu Pengetahuan Alam Aries Fitriawan Institut Pertanian Bogor Fakultas Matematika dan Ilmu Pengetahuan Alam Khaerunissa Anbar Istiadi Universitas Indonesia Arry Yanuar Universitas Indonesia Research article Keywords: COVID-19, Machine Learning, Pharmacophore Modeling, Molecular Docking, Indonesian Herbal Compounds, 3CLPro, SARS-CoV-2 Posted Date: June 11th, 2020 Page 1/33 DOI: https://doi.org/10.21203/rs.3.rs-29119/v1 License: This work is licensed under a Creative Commons Attribution 4.0 International License. Read Full License Page 2/33 Abstract Background Status of the latest developments from the spread of COVID-19 in Indonesia has reached 15438 cases with 1028 cases of patients died, updated on May 13, 2020. Unfortunately, the number of infected continues to overgrow, and no drugs have been approved for effective treatment. This research aims to nd potential candidate compounds in Indonesian herbal as COVID-19 supportive therapy using machine learning and pharmacophore modeling approach. Methods For a machine learning approach, we used three classication methods that have different principles in decision making, such as SVM, MLP, and Random Forest. By using these different methods, it is expected that more optimal screening results can be obtained than using only one method. Moreover, for a pharmacophore modeling approach, we did the structure-based method on the 3D structure of SARS-CoV- 2 main protease (3CLPro) and using known SARS, MERS, and SARS-CoV-2 repurposing drugs from literature as data sets on the ligand-based method. Lastly, we used molecular docking to analyse the interaction between 3CLpro (main protease) protein with 14 hit compounds from the Indonesian Herbal Database (HerbalDB) and Lopinavir as a positive control. Results The models yielded by SVM, RF, and MLP were used for screening in herbal compounds obtained from HerbalDB and got 125 potential compounds. Whereas the structure-based pharmacophore modeling gave eight hit compounds and the ligand-based methods produced more than a hundred hit compounds. Based on the screening on HerbalDB using these two prediction approaches, we got 14 hit compounds candidates. Further analysis was done using molecular docking to know the interaction between each compound and main protease of SARS-CoV-2 as inhibitory agents. From molecular docking analysis, we got six potential compounds as the main protease of SARS-CoV-2 inhibitor, i.e Hesperidin, Kaempferol- 3,4'-di-O-methyl ether (Ermanin); Myricetin-3-glucoside, Peonidine 3-(4’-arabinosylglucoside); Quercetin 3- (2G-rhamnosylrutinoside); and Rhamnetin 3-mannosyl-(1–2)-alloside. Conclusions Herbal compounds from various plants were potential as candidates of SARS-CoV-2 antivirals. Based on our research and literature study, one of the potential commodity crops in Indonesia is Psidium guajava (guava) and can be directly used by the community. Page 3/33 Introduction The new coronavirus, designated as SARS-CoV-2 (severe acute respiratory syndrome coronavirus 2), was rst identied in Wuhan, China, in December 2019 [1]. SARS-CoV-2 belongs to the family of Coronaviridae, single-stranded RNA virus (+ ssRNA) that spreads widely among humans and other mammals, causing a wide range of infections from common cold symptoms to fatal diseases, such as severe respiratory syndrome [2, 3]. Status of the latest developments from the spread of COVID-19 in Indonesia has reached 15438 cases with 1028 cases of patients died, updated on May 13, 2020 (data taken from www.covid19.go.id). Unfortunately, the number of infected continues to overgrow, and no drugs have been approved to be effective. Therefore, the need to discover and develop drugs for the treatment of the Coronavirus Disease 2019 (COVID-19) is urgent. Potential anti-coronavirus therapies can be divided into two categories depending on the target, one is acting on the human immune system or human cells, and the other is on coronavirus itself. In terms of the human immune system, the innate immune system response plays an important role in controlling the replication and infection of coronavirus, and interferon is expected to enhance the immune response [4]. Blocking the signal pathways of human cells required for virus replication may show a certain antiviral effect. The therapies acting on the coronavirus itself include preventing the synthesis of viral RNA through acting on the genetic material of the virus, inhibiting virus replication through acting on critical enzymes of virus, and blocking the virus binding to human cell receptors or inhibiting the virus’s self-assembly process through acting on some structural proteins [5]. Exploring new medicines for emerging and rapidly spreading diseases such as SARS-CoV-2 could be carried out through drug repurposing strategy to bypass the pre-clinical steps that usually require laborious works and resources. In addition, we also need to consider developing agents which in the future could be more easily utilized by the people. For this purpose, exploration of natural resources that are often used by the people is the best choice. Here, we purpose a research to nd potential candidate compounds in Indonesian plants as COVID-19 supportive therapy by using machine learning and pharmacophore modeling approach. The results of this study produced several potential compound candidates that could be used for supportive purposes and preventive as well, because the candidate plants (especially commodity crops) could be easily used directly by the community. Materials And Methods In this study, we combined two approaches of screening, by machine learning and pharmacophore modeling. The compounds that overlap from two approaches were further analysed using molecular docking. The graphical methods in this study is represented in Fig. 1. Machine Learning In big data analysis, for biomedical research, machine learning can be used to predict the drug-target interactions (DTI) based on chemical structure and genomic sequence information [6]. In this study the Page 4/33 machine learning approach in DTI prediction can be divided into four steps. First, collecting drug and protein target from literature and public domain database as training dataset; second, extracting chemical structure features and genomic sequence features from drug and protein targets dataset; third, training the prediction model using DTI training dataset; last, utilizing the predictive model to make predictions for herbal compounds data set. Dataset The original dataset used in this study, which consisted of drugs and protein targets, is obtained from a published review by Li and Clercq [7] and Wu et. al [5] in 2020. There are 81 virus-based drugs (Table 1), 17 human-based drugs (Table 2), 15 host-based proteins and 8 virus-based proteins (Table 3). To extend the exploration of drug-target interactions, we input protein targets and drugs into SuperTarget web resources [8]. The outputs of SuperTarget were not only the interactions between drugs and protein targets but also the new protein targets and new drugs (Table 4) that were not previously mentioned in previous paper. The total number of data obtained from literature and SuperTarget is 119 drugs, 335 protein targets, and 685 interactions (Additional le 1). Moreover, the total possible interaction that might exist is 119 drugs*335 targets = 39.865 interactions. Thus, there are 39.865–685 = 39.180 unknown interactions. We used 400 herbal compounds obtained from HerbalDB [9] as a testing dataset. The training and testing dataset required to be extracted into features. In this research, PubChem ngerprint and dipeptide descriptor were used as the drug compound features and the protein target features, respectively. PubChem ngerprint was acquired using PubChemPy library in python while dipeptide descriptor was calculated using protr package in R. Each record consists of 881 compound ngerprints and 400 protein dipeptide descriptors. For the training dataset, the drug which had interaction with a target was labeled as 1, otherwise was labeled as 0. Figure 2 shows the process of feature extraction from drug target interactions dataset. Page 5/33 Table 1 List of Potential Virus based Drug Related to COVID-19 Drug name Reference Drug name Reference Alfuzosin [5] Idarubicin [5] Almitrine [5] Indinavir [5] Amodiaquine [10] Iopromide [5] Amprenavir [5] Isotretinoin [5] Atazanavir [5] Itraconazole [5] Atovaquone [5] Lavodropropizine [5] Benzylpenicilloyl G [5] Loperamide [11] Bromocriptine [5] Lopinavir [11–15] b-thymidine [5] Lutein [5] Candoxatril [5] Lymecycline [5] Carvedilol [5] Masoprocol [5] Cefpiramide [5] Meoquine [15] Ceftibuten [5] Mimosine [5] Cefuroxime [5] Montelukast [5] Chenodeoxycholic acid [5] Nafamostat [16, 17] Chloramphenicol [5] Nelvinar [5] Chlorhexidine [5] Nepafenac [5] Cilastatin [5]
Details
-
File Typepdf
-
Upload Time-
-
Content LanguagesEnglish
-
Upload UserAnonymous/Not logged-in
-
File Pages33 Page
-
File Size-