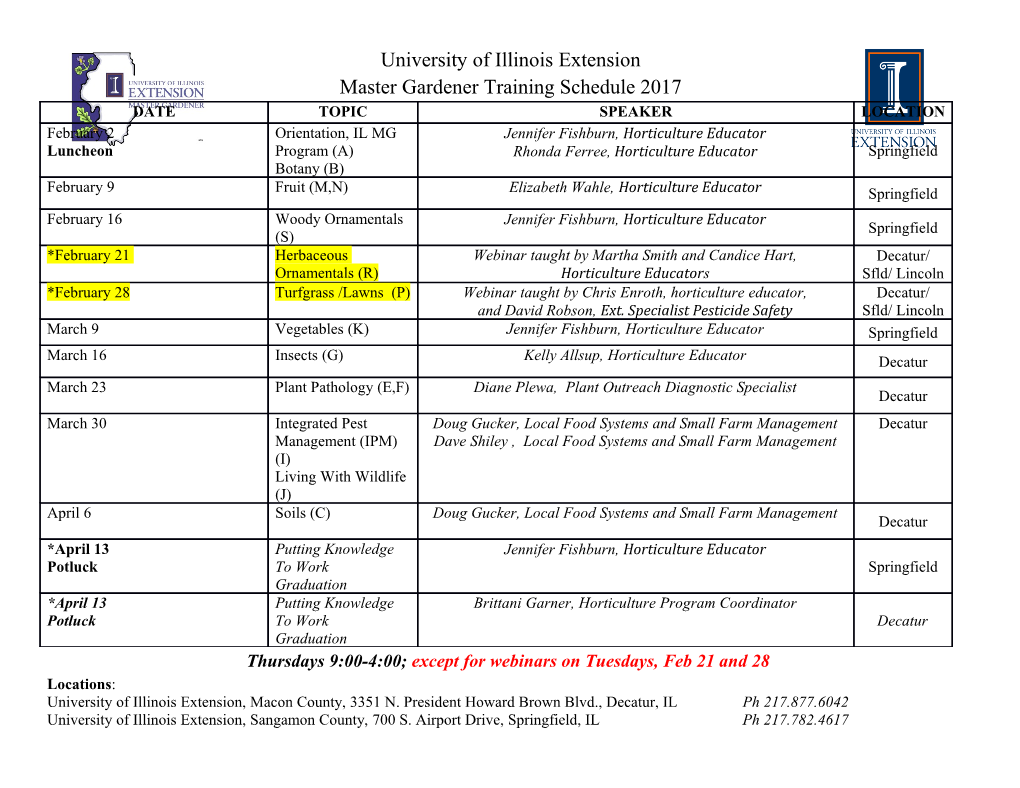
J. theor. Biol. (2002) 218, 309–321 doi:10.1006/yjtbi.3078, available online at http://www.idealibrary.com on Stochastic vs. Deterministic Modeling of Intracellular Viral Kinetics R. Srivastavawz,L.Youw,J.Summersy and J.Yinnw wDepartment of Chemical Engineering, University of Wisconsin, 3633 Engineering Hall, 1415 Engineering Drive, Madison, WI 53706, U.S.A., zMcArdle Laboratory for Cancer Research, University of Wisconsin Medical School, Madison, WI 53706, U.S.A. and yDepartment of Molecular Genetics and Microbiology, University of New Mexico School of Medicine, Albuquerque, NM 87131, U.S.A. (Received on 5 February 2002, Accepted in revised form on 3 May 2002) Within its host cell, a complex coupling of transcription, translation, genome replication, assembly, and virus release processes determines the growth rate of a virus. Mathematical models that account for these processes can provide insights into the understanding as to how the overall growth cycle depends on its constituent reactions. Deterministic models based on ordinary differential equations can capture essential relationships among virus constituents. However, an infection may be initiated by a single virus particle that delivers its genome, a single molecule of DNA or RNA, to its host cell. Under such conditions, a stochastic model that allows for inherent fluctuations in the levels of viral constituents may yield qualitatively different behavior. To compare modeling approaches, we developed a simple model of the intracellular kinetics of a generic virus, which could be implemented deterministically or stochastically. The model accounted for reactions that synthesized and depleted viral nucleic acids and structural proteins. Linear stability analysis of the deterministic model showed the existence of two nodes, one stable and one unstable. Individual stochastic simulation runs could access and remain at the unstable node. In addition, deterministic and averaged stochastic simulations yielded different transient kinetics and different steady-state levels of viral components, particularly for low multiplicities of infection (MOI), where few virus particles initiate the infection. Furthermore, a bimodal population distribution of viral components was observed for low MOI stochastic simulations. The existence of a low-level infected subpopulation of cells, which could act as a viral reservoir, suggested a potential mechanism of viral persistence. r 2002 Elsevier Science Ltd. All rights reserved. Introduction well-characterized. We have developed models Viruses are ideal systems for probing how the for the intracellular growth of bacteriophage T7 staticlinear information encodedby a genome (Endy et al., 1997; You & Yin, 2001) and HIV-1 defines a dynamicnonlinear processof growth (Reddy & Yin, 1999), which have enabled us to and development. Their genomes are small, suggest novel anti-viral strategies (Endy & Yin, ranging in size from 3 to 300 kilobases, and 2000), visualize dynamicpatterns of genetic in many cases their encoded functions regulatory elements (You & Yin, 2000), and are genetically, biochemically, and structurally probe how virus growth depends on genome organization (Endy et al., 2000) and host resources (You et al., 2002). To date, we have formulated our viral models as coupled ordinary nCorresponding author. Tel.: +1-608-265-3779; fax: +1-608-262-5434. differential equations and algebraicexpressions E-mail address: [email protected] (J. Yin). that employ parameters from the literature and 0022-5193/02/$35.00/0 r 2002 Elsevier Science Ltd. All rights reserved. 310 R. SRIVASTAVA ET AL. are numerically integrated to reveal dynamic may be established, suggesting a potential behavior. mechanism for viral persistence. Here, we seek to better understand what differences may result from stochastic and Model Development deterministickineticapproachesto modeling viral kinetics. A virus infection may be initiated A simple network of a model virus, shown by a single virus particle that delivers its genome, in Fig. 1, was developed to explore differences a single molecule of DNA or RNA, to its host between stochastic and deterministic model cell (Jilbert et al., 1996). Under such conditions, implementations. The components studied were the inherently stochastic nature of the ensuing the viral nucleic acids and a viral structural processes may give rise to dynamics that differ protein (struct). The viral nucleic acids were significantly from those predicted by determinis- classified as genomic (gen) or template (tem). The ticmodels. There are many examples of stochas- genome, whether it is DNA, positive-strand ticbiologicalmodels in the literature (Chin & RNA, negative-strand RNA, or some other Willsky, 1989; McAdams & Arkin, 1997; Arkin variant, is the vehicle by which viral genetic et al., 1998; Goss & Peccoud, 1998; Srivastava information is transported. The genome can et al., 2001), as well as examples comparing undergo one of the two fates. The first possibility deterministic and stochastic kinetics of simple is that it may be modified, whether through biochemical systems (Erdi! & Toth,! 1988), and integration into the host genome or some other linear and nonlinear chemical systems (Kurtz, type of processing (reverse transcription, etc.), 1972; Zheng & Ross, 1991). A nonlinear model to form a template; the template refers to the of the intracellular kinetics of a generic non-lytic form of the nucleic acid that is transcribed and virus was developed. For a non-lyticvirus, the involved in catalytically synthesizing every viral level of intracellular viral components must be component. The second possibility for the regulated in some manner to avoid destroying genome is that it may be packaged within the cell simply via excess propagation. The structural proteins to form progeny virus; question of how the levels of components, structural proteins may be capsid proteins or particularly the ones existing at low levels, are envelope proteins. regulated in the face of stochastic fluctuations is The standard sequence of viral replication an intriguing one. The current computational events involves the amplification of the viral study showed differences in stochastic and template after the infection, followed by produc- deterministicimplementations of the viral mod- tion of progeny virus. DNA viruses, for example, el. It also showed how a low-level viral reservoir initially make low levels of non-structural virus k4 degradation or secretion genome (gen) structural k6 k3 k1 protein (struct) template k5 (tem) degradation k2 Fig. 1. Model of viral replication cycle. The model tracks template (tem), genome (gen), and structural protein (struct). The tem is only used catalytically in the synthesis of gen and struct. The catalytic reactions are represented by (- ----)lines. STOCHASTIC VS. DETERMINISTIC VIRAL KINETICS 311 proteins that catalytically amplify the number of (ODE) was extracted: template molecules. Once the number of tem- d½tem plate molecules is sufficiently high, structural ¼ k1½genÀk2½tem; proteins are synthesized for incorporation into dt progeny particles. The timing of replication d½gen events may be tied to external factors, as ¼ k3½temÀk1½genÀk4½gen½struct; dt illustrated by the dependence of human papillo- ma virus replication on the differentiation state d½struct ¼ k ½temÀk ½structÀk ½gen½struct: of its host cell (Knipe & Howley, 2001). In our dt 5 6 4 model, the switch from viral genome amplifica- ð1Þ tion to virus production was regulated through viral mass action kinetics, as was shown through By using an ODE model to describe viral the simulation studies. Because the switch could kinetics, an underlying assumption is made that mathematically be accomplished solely through viral components change continuously with time the dynamics of key viral components, for our (Erdi! & Toth,! 1988). Such an assumption is purposes it was unnecessary to introduce any justified by assuming that the modeling results explicit dependence upon the host. However, an represent the average behavior of a large number implicit host dependence is reflected in the values of infected cells. of the kineticparameters of the model. For example, if in a given host cell a certain amino Parameter Selection acid is limiting, it may be reflected by a lower rate constant for protein synthesis. We assumed that gen was produced at The modeling network employed was lumped, 1 molecule cellÀ1 dayÀ1, the synthesis parameter in the sense that many individual reaction steps of structural protein was 1000-fold greater than were combined into a single step. For example, gen, and the rate of virus production was synthesis of structural protein requires that the 1 molecule temÀ1 cellÀ1 dayÀ1. A steady-state con- viral DNA be transcribed to mRNA and that the straint of 20 tem molecules was applied. By mRNA be translated to generate the structural setting the steady state of gen and struct to protein. For the viral model, it was assumed that 200 molecules and 10 000 molecules, respectively, such reactions could be combined together and we determined all of the parameter values for the characterized using a single-rate parameter. The model, as listed in Table 1. lumped reactions are represented by arrows in Fig. 1. Linear Stability Analysis Further assumptions made were: (1) other components necessary for the reactions to occur Two steady states were calculated for the viral (i.e. nucleic acids, amino acids, etc.) were network. One steady state occurred when available at a constant level, (2) once a cell was tem ¼ gen ¼ struct ¼ 0, the so-called ‘‘trivial’’ infected, it could not be re-infected, even if the solution. The other steady state occurred at the initial infection was cleared (i.e. superinfection could not occur), (3) reactants were distributed in a spatially homogenous manner, (4) a cell was initially infected with a pre-set number of viral Ta ble 1 templates, and (5) cells were terminally differ- Model parameters entiated and did not divide. Parameter Value À1 k1 0.025 day À1 k2 0.25 day À1 k3 1.0 day Deterministic Analysis À6 À1 À1 k4 7.5 Â 10 molecules day À1 Based on the network established in Fig. 1, k5 1000 day k À1 a set of coupled ordinary differential equations 6 1.99 day 312 R.
Details
-
File Typepdf
-
Upload Time-
-
Content LanguagesEnglish
-
Upload UserAnonymous/Not logged-in
-
File Pages13 Page
-
File Size-