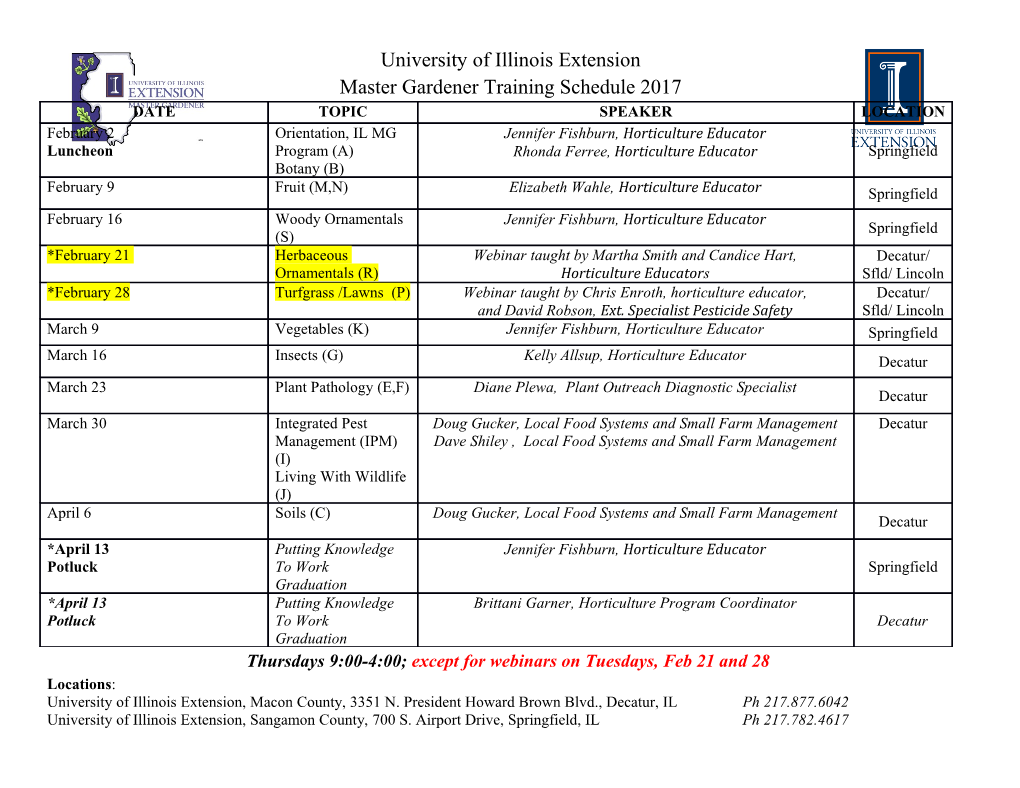
Week 2: Causal Inference Marcelo Coca Perraillon University of Colorado Anschutz Medical Campus Health Services Research Methods I HSMP 7607 2020 These slides are part of a forthcoming book to be published by Cambridge University Press. For more information, go to perraillon.com/PLH. This material is copyrighted. Please see the entire copyright notice on the book's website. 1 Outline Correlation and causation Potential outcomes and counterfactuals Defining causal effects The fundamental problem of causal inference Solving the fundamental problem of causal inference: a) Randomization b) Statistical adjustment c) Other methods The ignorable treatment assignment assumption Stable Unit Treatment Value Assumption (SUTVA) Assignment mechanism 2 Big picture We are going to review the basic framework for understanding causal inference This is a fairly new area of research, although some of the statistical methods have been used for over a century The \new" part is the development of a mathematical notation and framework to understand and define causal effects This new framework has many advantages over the traditional way of understanding causality. For me, the biggest advantage is that we can talk about causal effects without having to use a specific statistical model (design versus estimation) In econometrics, the usual way of understanding causal inference is linked to the linear model (OLS, \general" linear model) { the zero conditional mean assumption in Wooldridge: whether the additive error term in the linear model, i , is correlated with the variable of interest (treatment). The problem is that when you move to circumstances in which a linear model is not the best tool (say, outcome is 1/0 or a count), things are confusing because there is no additive error term in these models 3 Big Picture II - Readings As we will see, there are reasons to even prefer a nonparametric model rather than a linear/OLS model to estimate a conditional expectation function E[yi jxi ] The main ideas of this new framework have been refined recently (last 15 years?), but there is still no common notation or language For the economists in the room: please read Imbens and Wooldridge (2009) \Recent developments in the econometrics of program evaluation" for a road map. Wooldridge (2010) book Chapter 21 has an interesting take. That chapter is the framework of Stata's new(ish) commands teffects and eteffects Angrist and Pischke (2009) also provide a bridge from the statistical literature on causal effects to econometrics, so we will use their notation, although they focus too much on the linear/OLS model. Imbens and Rubin (2015) is a better introduction to these topics (on Canvas) Note that the economics examples are mostly from labor economics. Labor economics is the field where econ PhD students end up if they want to focus on empirical methods 4 Big picture III You have all heard that correlation (association) does not imply causation Causal inference is about understanding under which circumstances correlation (association) does imply causation It's obviously a fundamental question since we want to understand causal effects when doing research and when using statistical models It's fundamental in health services research and health economics But remember that we also use statistical models as descriptive tools and for prediction. Make sure you understand the difference 5 Basics concepts Causality is linked to a manipulation (treatment, intervention, action, strategy) that is applied to a unit A unit could be a person, firm, hospital, country, county, classroom, etc Think of it as the \thing" that received the action or was manipulated The unit could have been exposed to an alternative action For simplicity, only two possibilities: receiving or not receiving the action or treatment (active versus control treatment in Imbens and Rubin, 2015) This is the usual simplification to understand key concepts, but there could be multiple treatment levels that could even be continuous like medication doses or monetary incentives A unit either receiving or not receiving a treatment is linked to a potential outcome 6 Potential outcomes for units The \potential" part refers to the idea that only one outcome is realized after the intervention; the other is, well, potential (Dictionary definition: Potential: having or showing the capacity to become or develop into something in the future) Before the intervention, there are two potential outcomes. Only one is realized after the action is conducted Example: a person may or may not receive a job training program if unemployed. The outcome could be income one year after the program { income if the person participated in the program and the income if the person didn't participate in the program. The outcome could be binary: getting a job or not Jargon alert: economists like to use a priori, a posteriori, ex ante, ex post instead or before and after 7 Notation The observed or realized outcome for a unit i is Yi We will denote the treatment received by a unit i as Di , which could be 0 or 1, so Di 2 f0; 1g The other common notation is for treatment to be W ; Stata manual uses t for treatment We have then two potential outcomes: Y1i if the unit received treatment (Di = 1) and Y0i if not (Di = 0) 0 Other common notation for potential outcomes [control]: Yi or Yi (0); Stata uses y0. Don't get confused with notation It's also helpful to keep the index i. We will extend this notation when the structure of the data changes. Yi is different than, say, Yits . The latter could be the outcome for person i at time t in state s 8 Definition of causal effects The causal effect effect of receiving treatment for a unit i is a comparison of potential outcomes We could compare Y1i − Y0i , the difference between outcomes when units are treated versus not Or we could measure the effect as a relative measure: Y1i . Or any other way, Y0i but the idea is that the causal effect of the treatment a unit received Di is a comparison of potential outcomes Note that the definition of causal effects does not depend on the actual treatment or action taken 9 Causal effects This idea seems a bit confusing at first but it's a deep concept. You just need to get used to it A person i could receive an antidepressant or not. The outcome Yi could be being depression free at two months (a binary outcome) The causal effect of receiving the antidepressant is defined as the comparison of the outcome for the person with the antidepressant and without the antidepressant, potential outcomes that do not depend on what treatment the person gets We could define the outcome as the probability of being depression free at two months, so the causal effect could be P(Y1i ) − P(Y0i ) Again, we could use other ways of measuring effects. For example, the odds-ratio (which is hard to interpret anyway) P(Y1i ) 1−P(Y1i ) P(Y0i ) 1−P(Y0i ) 10 Causal effects This way of thinking about causal effects matches everyday thinking The causal effect of the next election is the comparison of an outcome, say, the status of the pandemic next year if the country reelects the current president or not The causal effect of studying hard for this class is a comparison of two potential outcomes: your grade if you study hard and your grade if you don't. Only one will be observed because you will study hard or not In the movie a It's a Wonderful Life, Clarence the angel shows George Bailey what would have happened had he not been born. In essence showing Bailey the causal effect of him being alive These ideas could be extended to multi-value treatments. Rather than study hard or not, we could have multiple levels of effort that could be measured continuously 11 The fundamental problem of causal inference We now have a definition of causal effects but we also have a BIG PROBLEM The challenge in causal inference is that we do not observe both potential outcomes; we only observe one. (In the stats literature this is called the \fundamental problem of causal inference." In the economics literature, it's called the fundamental problem of program evaluation) Note that in this framework, the same unit receiving a treatment at a different time is a different unit The non-observable or not-realized outocome is called the counterfactual (Dictionary: relating to or expressing what has not happened or is not the case) Said another way, in real life, the problem is that there is no Clarence the angel to tell us the counterfactual This way of understanding causal effects (potential outcomes, counterfactuals) is now called the Rubin causal model (see Imbens and Wooldridge, 2009 for some history, also Imbens and Rubin, 2015, Chapter 2) 12 Counterfactuals Going back to our notation, we can write the observed outcome Yi in terms of the potential outcome as Yi = Y0i + (Y1i − Y0i )Di If Di = 1 then the observed outcome is Yi = Y1i . If Di = 0 then the observed outcome is Yi = Y0i A unit either receives the treatment or not, never both So if a unit receives the treatment the observed outcome is Y1i and counterfactual outcome is Y0i Important (!!): We can think of causal inference as a PREDICTION problem. How could we predict the counterfactual given that we never observe it? 13 Digression for home study You have to read the assigned readings for this week. This is an area that requires a lot of home study In the words of my Jesuit priest high school director: To be a good student you need two things: a big behind and a head.
Details
-
File Typepdf
-
Upload Time-
-
Content LanguagesEnglish
-
Upload UserAnonymous/Not logged-in
-
File Pages46 Page
-
File Size-