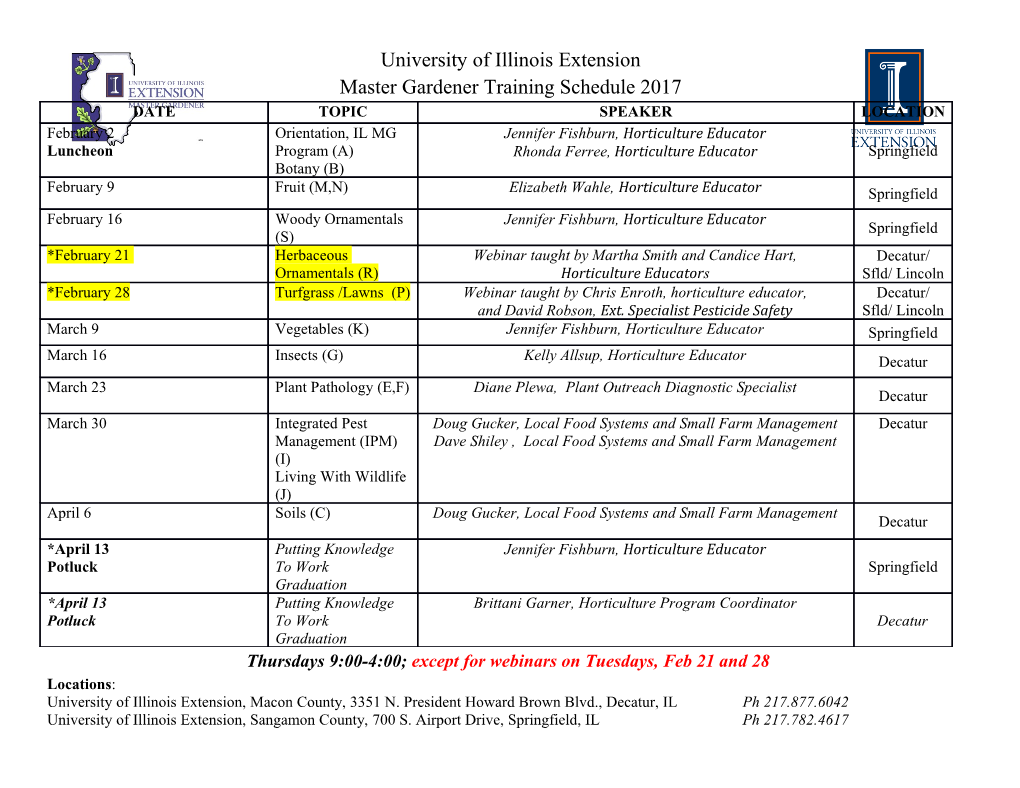
STEREO PANNING FEATURES FOR CLASSIFYING RECORDING PRODUCTION STYLE George Tzanetakis, Randy Jones, and Kirk McNally University of Victoria Computer Science [email protected], [email protected], [email protected] ABSTRACT bum effect. The performance of artist identification sys- tems degrades when music from different albums is used Recording engineers, mixers and producers play im- for training and evaluation [7]. Therefore, the classifi- portant yet often overlooked roles in defining the sound of cation results of such systems are not based entirely on a particular record, artist or group. The placement of dif- the musical content. Various stages of production of the ferent sound sources in space using stereo panning infor- recorded artifact, including recording, mixing, and mas- mation is an important component of the production pro- tering, all have the potential to influence classification. cess. Audio classification systems typically convert stereo This has led to research which attempts to quantify the signals to mono and to the best of our knowledge have not effects of production on acoustic features. By detecting utilized information related to stereo panning. In this pa- equalization curves used in album mastering, it is possible per we propose a set of audio features that can be used to to compensate for the effects of mastering so that multi- capture stereo information. These features are shown to ple instances of the same song on different albums can be provide statistically important information for non-trivial better compared [3]. We believe that other information re- audio classification tasks and are compared with the tra- lated to the recording process, specifically mixing, is an ditional Mel-Frequency Cepstral Coefficients. The pro- important component of understanding modern pop and posed features can be viewed as a first attempt to capture rock music and should be incorporated rather than being extra-musical information related to the production pro- removed from music information retrieval systems. cess through music information retrieval techniques. Our goal is to explore stereo panning information as an aspect of the recording and production process. Stereo 1 INTRODUCTION information has been utilized for source separation pur- poses [2, 9]. However, to the best of our knowledge, it has Starting in the 1960s the recording process for rock and not been used in classification systems for audio signals. popular music moved beyond the convention of recreat- In this paper we show that stereo panning information is ing as faithfully as possible the illusion of a live perfor- indeed useful for automatic music classification. mance. Facilitated by technological advances including multi-track recording, tape editing, equalization and com- 2 STEREO PANNING INFORMATION pression, the creative contributions of record producers EXTRACTION became increasingly important in defining the sound of artists, groups, and styles [4]. Although not as well known In this section we describe the process of calculating stereo as the artists they worked with, legendary producers in- panning information for different frequencies based on the cluding Phil Spector, George Martin, Quincy Jones, and short-time Fourier transform (STFT) of the left and right Brian Eno changed the way music was created. channels. Using the extracted Stereo Panning Spectrum So far, research in music information retrieval has largely we propose features for classification. ignored information about the recording process, focusing instead on capturing information about pitch, rhythm and timbre. A common methodology is to extract features, 2.1 Stereo Panning Spectrum quantifiable attributes of music signals, from recordings, Avendano [2] describes a frequency-domain source iden- then to classify these features into distinct groups using tification system based on a cross-channel metric called machine learning techniques. This two-part process has the panning index. We use the same metric as the basis enabled tasks such as automatic identification of genres, for calculating stereo audio features for classification. For albums and artists. the remainder of the paper the term Stereo Panning Spec- The influence of the recording process on automatic trum (SPS) is used instead of the panning index as we feel classification has been acknowledged and termed the al- it is a more accurate term. The SPS holds the panning values (between -1 and +1 with 0 being center) for each c 2007 Austrian Computer Society (OCG). frequency bin. Stereo Panning Spectrum The derivation of the SPS assumes a simplified model 500 of the stereo signal. In this model each sound source is 450 recorded individually and then mixed into a single stereo 400 350 signal by amplitude panning. Stereo reverberation is then 300 added artificially to the mix. The basic idea behind the 250 SPS is to compare the left and right signals in the time- Frequency Bin 200 frequency plane to derive a two-dimensional map that iden- 150 100 tifies the different panning gains associated with each time- 50 frequency bin. By selecting time-frequency bins with sim- 100 200 300 400 500 600 700 800 900 1000 1100 1200 Time ilar panning it is possible to separate particular sources [2]. In this paper we utilize the SPS directly as the basis Figure 1. Stereo Panning Spectrum of “Hell’s Bells” by for extracting statistical features without attempting any ACDC (approximately 28 seconds). form of source separation. Our SPS definition directly fol- lows Avendano [2]. Stereo Panning Spectrum 500 If we denote the STFT of the left,right signals xl(t); xr(t) for a particular analysis window as X (k);X (k), where 450 l r 400 k is the frequency index we can define the following sim- 350 ilarity measure: 300 250 Frequency Bin jX (k)X∗(k)j 200 l r 150 (k) = 2 ∗ 2 2 (1) jXl(k)j + jXr(k)j 100 50 where ∗ denotes complex conjugation. For a single source 0 200 400 600 800 1000 1200 with amplitude panning the similarity function will have Time a value proportional to the panning coefficient α in those time frequency regions where the source has energy. More Figure 2. Stereo Panning Spectrum of “Supervixen” by specifically if we assume the sinusoidal energy-preserving Garbage (approximately 28 seconds) p 2 panning law: ar = 1 − al then: p Figure 1 shows a visualization of the Stereo Panning (k) = 2α 1 − α2 (2) Spectrum for the song “Hell’s Bells” by ACDC. The visu- If the source is panned to the center (i.e α = 0:7071) then alization is similar to a Spectrogram with the X-axis corre- the function will attain its maximum value of 1, and if sponding to time, measured in number of analysis frames, the source is completely panned to either side the function and the Y-axis corresponding to frequency bin. No pan- will attain its minimum value of zero. The ambiguity with ning is represented by gray, full left panning by black and regards to the later direction of the source can be resolved full right panning by white. The songs starts with four bell using the partial similarity measures: sounds that alternate between slight panning to the left and to the right, visible as changes in grey intensity. Near the end of the first 28 seconds a strong electric guitar enters ∗ ∗ jXl(k)Xr (k)j jXr(k)Xl (k)j on the right channel, visible as white. l = 2 ; r = 2 (3) jXl(k)j jXr(k)j Figure 2 shows a visualization of the SPS for the song “Supervixen” by Garbage. Several interesting stereo ma- and their difference: nipulations can be observed in the figure and heard when listening to the song. The song starts with all instruments ∆(k) = − (4) l r centered for a brief period and then moves them to the left and right creating an explosion like effect. Most of the where positive values of ∆(k) correspond to signals panned sound of a fast repetitive hi-hat is panned to the right (the towards the left and negative values correspond to signals wide dark bar over the narrow horizontal white bar) with panned to the right. Thus we can define the following a small part of it panned to the left (the narrow horizontal ambiguity-resolving function: white bar). Near the end of the first 28 seconds the voice 8 enters with the a crash cymbal panned to the left, visible +1; if ∆(k) > 0 <> as the large black area. ∆(^ k) = 0; if ∆(k) = 0 (5) :> −1; if ∆(k) < 0 2.2 Stereo Panning Spectrum Features Shifting and multiplying the similarity function by ∆(^ k) In this section we describe a set of features that summarize we obtain the Stereo Panning Spectrum (or panning index) the information contained in the Stereo Panning Spectrum as: that can be used for automatic music classification. The main idea is to capture the amount of panning in different SP S(k) = [1 − (k)] ∗ ∆(^ k) (6) frequency bands as well as how it changes over time. We define the Panning Root Mean Square for a partic- Garage/Grunge ZeroR NBC SMO J48 ular frequency band as: SPSF 56.4 77.2 81 84.2 v SMFCC 56.4 74. 6 76.7 71.6 u h u 1 X SPSF+SMFCC 56.4 82.7 83.7 83.2 P = t [SP S(k)]2 (7) l;h h − l k=l Table 1 where l is the lower frequency of the band, h is the high . Classification accuracies for Garage/Grunge frequency of the band, and N is the number of frequency bins. By using RMS we only consider the amount of pan- Acoustic/Electric ZeroR NBC SMO J48 ning without taking into account whether it is to the left SPSF 51.3 99.4 99.7 99.1 or right.
Details
-
File Typepdf
-
Upload Time-
-
Content LanguagesEnglish
-
Upload UserAnonymous/Not logged-in
-
File Pages4 Page
-
File Size-