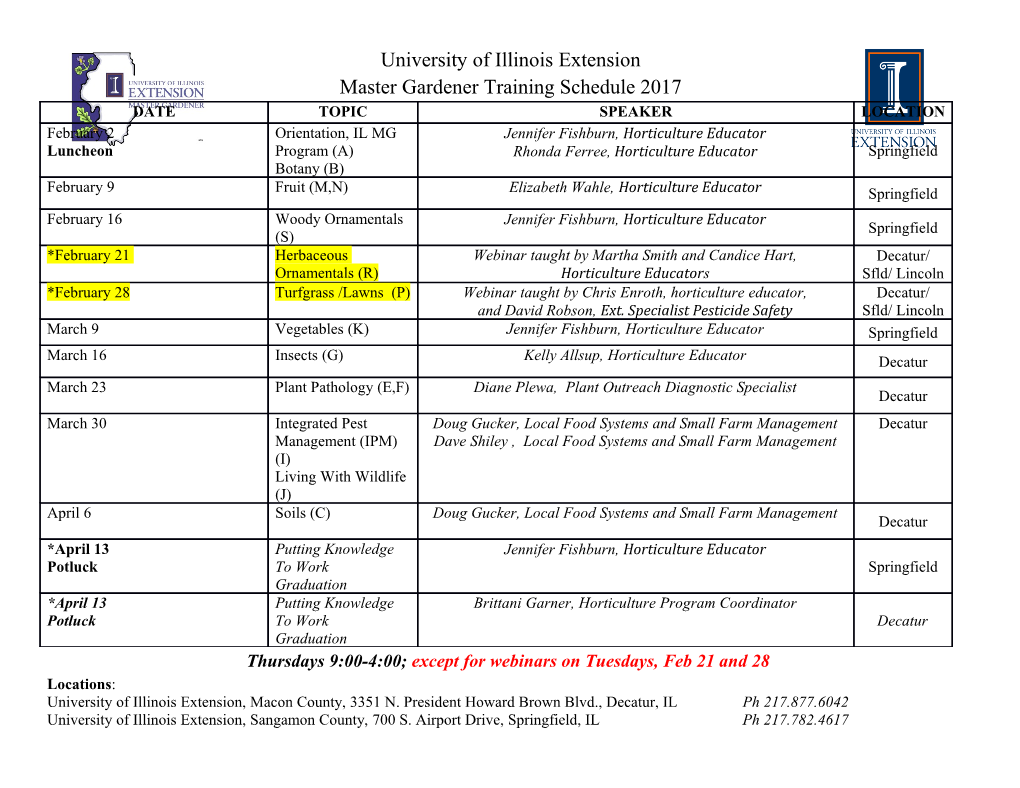
DRAFT V1.2 From Math, Numerics, & Programming (for Mechanical Engineers) Masayuki Yano James Douglass Penn George Konidaris Anthony T Patera September 2012 © The Authors. License: Creative Commons Attribution-Noncommercial-Share Alike 3.0 (CC BY-NC-SA 3.0), which permits unrestricted use, distribution, and reproduction in any medium, provided the original authors and MIT OpenCourseWare source are credited; the use is non-commercial; and the CC BY-NC-SA license is retained. See also http://ocw.mit.edu/terms/. Contents III Linear Algebra 1: Matrices and Least Squares. Regression. 199 15 Motivation 201 16 Matrices and Vectors: Definitions and Operations 203 16.1 Basic Vector and Matrix Operations ........................... 203 16.1.1 Definitions ..................................... 203 Transpose Operation ... ... ... ... ... ... ... ... ... 204 16.1.2 Vector Operations ................................. 205 Inner Product ... ... ... ... ... ... ... ... ... ... 207 Norm (2-Norm) .. ... ... ... ... ... ... ... ... ... 207 Orthogonality ... ... ... ... ... .. ... ... ... ... 211 Orthonormality .. ... ... ... ... ... ... ... ... ... 212 16.1.3 Linear Combinations ................................ 212 Linear Independence .. ... ... ... ... ... ... ... ... 213 Vector Spaces and Bases . ... ... ... ... ... ... ... ... 214 16.2 Matrix Operations ..................................... 217 16.2.1 Interpretation of Matrices ............................. 217 16.2.2 Matrix Operations ................................. 218 Matrix-Matrix Product .............................. 219 16.2.3 Interpretations of the Matrix-Vector Product . 222 Row Interpretation . ... ... ... ... ... ... ... ... ... 222 Column Interpretation .. ... ... ... ... ... ... ... ... 223 Left Vector-Matrix Product .. ... ... ... ... ... ... ... 223 16.2.4 Interpretations of the Matrix-Matrix Product . 224 Matrix-Matrix Product as a Series of Matrix-Vector Products . 224 Matrix-Matrix Product as a Series of Left Vector-Matrix Products . 225 16.2.5 Operation Count of Matrix-Matrix Product ................... 225 16.2.6 The Inverse of a Matrix (Briefly) ......................... 226 16.3 Special Matrices ...................................... 227 16.3.1 Diagonal Matrices ................................. 228 16.3.2 Symmetric Matrices ................................ 228 16.3.3 Symmetric Positive Definite Matrices ....................... 228 16.3.4 Triangular Matrices ................................ 230 16.3.5 Orthogonal Matrices ................................ 230 16.3.6 Orthonormal Matrices ............................... 232 16.4 Further Concepts in Linear Algebra ........................... 233 16.4.1 Column Space and Null Space .......................... 233 16.4.2 Projectors ...................................... 234 3 17 Least Squares 237 17.1 Data Fitting in Absence of Noise and Bias ....................... 237 17.2 Overdetermined Systems .. ... ... ... ... ... ... ... ... ... 242 Row Interpretation . ... ... ... ... ... ... ... ... ... 243 Column Interpretation .. ... ... ... ... ... ... ... ... 244 17.3 Least Squares . ... ... ... ... ... ... ... ... ... ... .. 245 17.3.1 Measures of Closeness ............................... 245 17.3.2 Least-Squares Formulation (`2 minimization) .................. 246 17.3.3 Computational Considerations .......................... 250 QR Factorization and the Gram-Schmidt Procedure . 251 17.3.4 Interpretation of Least Squares: Projection . 253 17.3.5 Error Bounds for Least Squares .......................... 255 Error Bounds with Respect to Perturbation in Data, g (constant model) . 255 Error Bounds with Respect to Perturbation in Data, g (general) ....... 256 Error Bounds with Respect to Reduction in Space, B . ... ... ... 262 18 Matlab Linear Algebra (Briefly) 267 18.1 Matrix Multiplication (and Addition) .......................... 267 18.2 The Matlab Inverse Function: inv . ... ... ... ... ... ... ... 268 18.3 Solution of Linear Systems: Matlab Backslash .. ... ... ... ... ... 269 18.4 Solution of (Linear) Least-Squares Problems . ... ... ... ... ... ... 269 19 Regression: Statistical Inference 271 19.1 Simplest Case . ... ... ... ... ... ... ... .. ... ... ... 271 19.1.1 Friction Coefficient Determination Problem Revisited . 271 19.1.2 Response Model .................................. 272 19.1.3 Parameter Estimation ............................... 275 19.1.4 Confidence Intervals ................................ 277 Individual Confidence Intervals .......................... 278 Joint Confidence Intervals ... ... ... ... ... ... ... ... 279 19.1.5 Hypothesis Testing ................................. 284 19.1.6 Inspection of Assumptions ............................. 285 Checking for Plausibility of the Noise Assumptions . 285 Checking for Presence of Bias .. ... ... .. ... ... ... ... 286 19.2 General Case ........................................ 287 19.2.1 Response Model .................................. 287 19.2.2 Estimation ..................................... 288 19.2.3 Confidence Intervals ................................ 289 19.2.4 Overfitting (and Underfitting) .......................... 290 4 Unit III Linear Algebra 1: Matrices and Least Squares. Regression. 199 Chapter 15 Motivation DRAFT V1.2 © The Authors. License: Creative Commons BY-NC-SA 3.0 . In odometry-based mobile robot navigation, the accuracy of the robot’s dead reckoning pose tracking depends on minimizing slippage between the robot’s wheels and the ground. Even a momentary slip can lead to an error in heading that will cause the error in the robot’s location estimate to grow linearly over its journey. It is thus important to determine the friction coefficient between the robot’s wheels and the ground, which directly affects the robot’s resistance to slippage. Just as importantly, this friction coefficient will significantly affect the performance of the robot: the ability to push loads. When the mobile robot of Figure 15.1 is commanded to move forward, a number of forces come into play. Internally, the drive motors exert a torque (not shown in the figure) on the wheels, which is resisted by the friction force Ff between the wheels and the ground. If the magnitude of Ff dictated by the sum of the drag force Fdrag (a combination of all forces resisting the robot’s motion) and the product of the robot’s mass and acceleration is less than the maximum static friction force max Ff ; static between the wheels and the ground, the wheels will roll without slipping and the robot max will move forward with velocity v = !rwheel. If, however, the magnitude of Ff reaches Ff ; static, the wheels will begin to slip and Ff will drop to a lower level Ff; kinetic, the kinetic friction force. The wheels will continue to slip (v < !rwheel) until zero relative motion between the wheels and the ground is restored (when v = !rwheel). The critical value defining the boundary between rolling and slipping, therefore, is the maximum w v Fdrag W Ff Fnormal, front Fnormal, rear Figure 15.1: A mobile robot in motion. 201 Ffriction vs. Time for 500 Gram Load max, meas 0.9 Ff, static = µs Fnor mal + experimental error 0.8 0.7 0.6 meas F = µk Fnormal + experimental error (Newtons) 0.5 f, kinetic 0.4 friction 0.3 F 0.2 Ff, static = Ftangential, applied µs Fnormal 0.1 ≤ 0 1 2 3 4 5 6 7 8 time (seconds) Figure 15.2: Experimental setup for friction measurement: Force transducer (A) is con- Figure 15.3: Sample data for one friction nected to contact area (B) by a thin wire. measurement, yielding one data point for max; meas Normal force is exerted on the contact area Ff ; static . Data courtesy of James Penn. by load stack (C). Tangential force is ap­ plied using turntable (D) via the friction be­ tween the turntable surface and the contact area. Apparatus and photograph courtesy of James Penn. static friction force. We expect that max Ff ; static = µs Fnormal; rear ; (15.1) where µs is the static coefficient of friction and Fnormal; rear is the normal force from the ground on the rear, driving, wheels. In order to minimize the risk of slippage (and to be able to push large loads), robot wheels should be designed for a high value of µs between the wheels and the ground. This value, although difficult to predict accurately by modeling, can be determined by experiment. max We first conduct experiments for the friction force Ff ; static (in Newtons) as a function of normal 2 load Fnormal; applied (in Newtons) and (nominal) surface area of contact Asurface (in cm ) with the friction turntable apparatus depicted in Figure 15.2. Weights permit us to vary the normal load and “washer” inserts permit us to vary the nominal surface area of contact. A typical experiment (at a particular prescribed value of Fnormal; applied and Asurface) yields the time trace of Figure 15.3 from max; means max which the Ff ; static (our measurement of F f; static) is deduced as the maximum of the response. We next postulate a dependence (or “model”) max Ff ; static(Fnormal; applied;Asurface) = β0 + β1 Fnormal; applied + β2 Asurface ; (15.2) where we expect — but do not a priori assume — from Messieurs Amontons and Coulomb that β0 = 0 and β2 = 0 (and of course β1 ≡ µs). In order to confirm that β0 = 0 and β2 = 0 — or at least confirm that β0 = 0 and β2 = 0 is not untrue — and to find a good estimate for β1 ≡ µs, we must appeal to our measurements. The mathematical techniques by which to determine µs (and β0, β2) “with some confidence” from noisy experimental data is known as regression, which is the subject of Chapter 19. Regression, in turn, is best described
Details
-
File Typepdf
-
Upload Time-
-
Content LanguagesEnglish
-
Upload UserAnonymous/Not logged-in
-
File Pages107 Page
-
File Size-