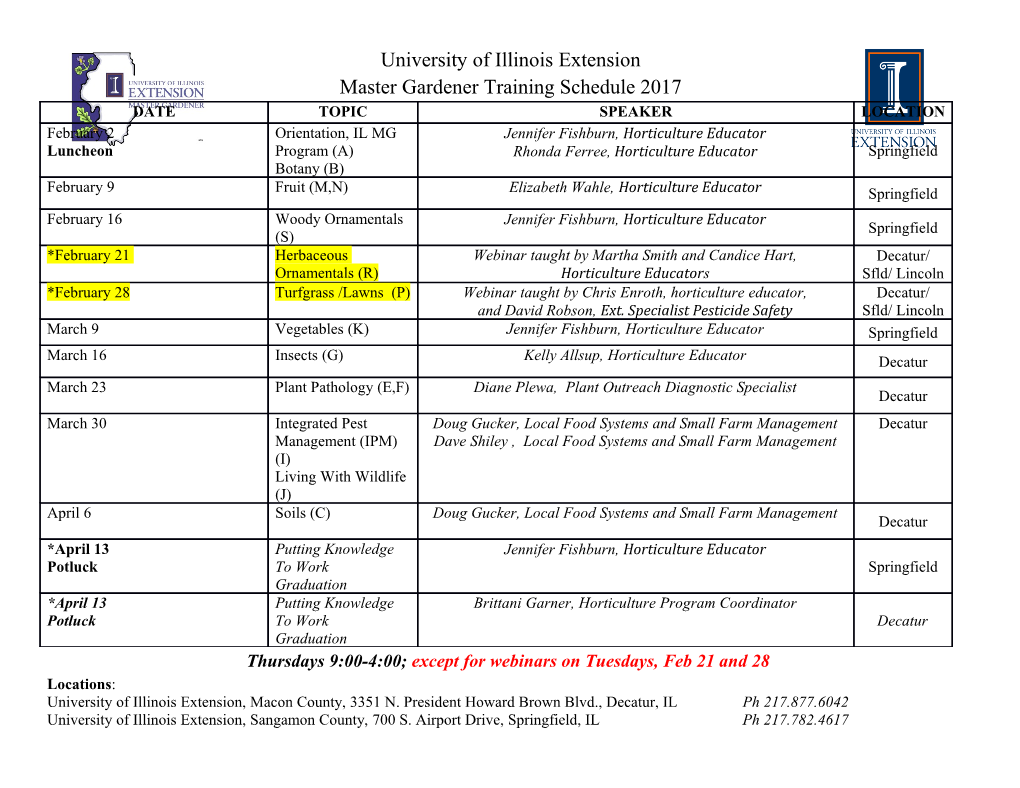
Under review as a conference paper at ICLR 2021 PRACTICAL PHASE RETRIEVAL:LOW-PHOTON HOLOGRAPHY WITH UNTRAINED PRIORS Anonymous authors Paper under double-blind review ABSTRACT Phase retrieval is the inverse problem of recovering a signal from magnitude-only Fourier measurements, and underlies numerous imaging modalities, such as Co- herent Diffraction Imaging (CDI). A variant of this setup, known as holography, includes a reference object that is placed adjacent to the specimen of interest be- fore measurements are collected. The resulting inverse problem, known as holo- graphic phase retrieval, is well-known to have improved problem conditioning relative to the original. This innovation, i.e. Holographic CDI, becomes crucial at the nanoscale, where imaging specimens such as viruses, proteins, and crys- tals require low-photon measurements. This data is highly corrupted by Poisson shot noise, and often lacks low-frequency content as well. In this work, we in- troduce a dataset-free deep learning framework for holographic phase retrieval adapted to these challenges. The key ingredients of our approach are the explicit and flexible incorporation of the physical forward model into an automatic differ- entiation procedure, the Poisson log-likelihood objective function, and an optional untrained deep image prior. We perform extensive evaluation under realistic con- ditions. Compared to competing classical methods, our method recovers signal from higher noise levels and is more resilient to suboptimal reference design, as well as to large missing regions of low frequencies in the observations. To the best of our knowledge, this is the first work to consider a dataset-free machine learning approach for holographic phase retrieval. 1 INTRODUCTION Phase retrieval is a nonlinear inverse problem that arises ubiquitously in imaging sciences, and has gained much recent attention (Shechtman et al., 2015). In this work we focus on a practical instance of the problem that arises in Coherent Diffraction Imaging (CDI). Here, holographic phase retrieval m n consists of recovering an image X0 R × from a set of squared Fourier transform magnitudes 2 Y = F (X + R ) 2 ; (1) j 0 0 j m n where F denotes an oversampled Fourier transform operator and R0 R × is a known reference 2 image whose support does not intersect the support of X0. The known reference image R0 distin- guishes holographic phase retrieval from the (classical) phase retrieval setting, where the goal is to 2 recover X0 from F (X0) alone. We focus entirely on the holographic version of the problem in realistic conditions:j with highj noise levels and missing low-frequency data. The advantage provided by the holographic reference is briefly illustrated on in Figure 1. In the remainder of this introduction we will situate this problem in the context of Coherent Diffrac- tion Imaging, review related works, and list our contributions. Section 2 describes our setup in detail, and Section 3 our reconstruction strategy. In Section 4, we describe extensive experiments and compare to several baseline methods. Section 5 concludes with a general discussion. 1.1 HOLOGRAPHIC COHERENT DIFFRACTION IMAGING AND PHASE RETRIEVAL Coherent Diffraction Imaging (CDI) is a scientific imaging technique used for resolving nanoscale scientific specimens, such as viruses, proteins, and crystals (Miao et al., 1999). In CDI, an image is sought to be reconstructed from X-ray diffraction measurements recorded on a CCD detector plane. By the far-field approximation of optical theory, these measurements are approximately pro- portional to the squared magnitude values of the Fourier transform of the electric field within the 1 Under review as a conference paper at ICLR 2021 HIO HIO HolOpt-P HolOpt-P Ground Truth no reference w. reference adapted no reference w. reference Figure 1: The advantage of using a reference for phase retrieval at Np = 1 photon/pixel. Two algo- rithms, are applied to reconstruct from Fourier magnitude data of image alone and image+reference. Comparing between algorithms, HIO and our HolOpt-P, observe the higher-quality reconstruction when the reference is present. (VIRUS image courtesy of Ghigo et al. (2008)) diffraction area. Thus, the specimen structure (e.g., its electron density) can be determined, in prin- ciple, by solving the phase retrieval problem. Holographic CDI is a popular setup to perform CDI experiments in which the object undergoing diffraction physically consists of a specimen together with a “reference”, i.e. a portion of the object a priori known. This setup is illustrated in Figure 2. The inclusion of a reference in the CDI setup both enhances the quality of image reconstruc- tion, and greatly simplifies the analysis and solution of the corresponding phase retrieval problem (Barmherzig et al., 2019). Nevertheless, holographic CDI remains challenging in practice. Due to the quantum mechanical nature of photon emission and absorption, CDI measurements are inherently corrupted by Poisson shot noise. The severity of this noise corruption is inversely proportional to the strength of the X- ray source in use, which is in turn quantified via Np, the number of photons per pixel reaching the detector plane. Nanoscale applications of CDI often necessitate imaging in the low-photon regime, where measurements are highly corrupted by noise. CDI measurements are also typically lacking low-frequency data, due to the presence of a beamstop apparatus which occludes direct measurement of these values (He et al., 2015). The holographic phase retrieval problem is commonly solved by inverse filtering (Gabor, 1948; Kikuta et al., 1972), which amounts to solving a structured system of linear equations. While straightforward, this method is not well-suited for noisy data. Wiener filtering (Gorkhover et al., 2018) is a variant on this method with some denoising ability. Yet Wiener filtering is derived to account for an additive noise model — an assumption which is not true for Poisson shot noise at low photon counts, and only holds as an approximation at high photon count levels (Salditt et al., 2020). These methods do not account explicitly for missing low-frequency data, and require a minimum separation between the specimen and the reference objects, the holographic separation condition (see Section 4.3). The most popular algorithm for the classical phase retrieval problem is the Hybrid Input-Output (HIO) algorithm (Fienup, 1978), which can as well be adapted to the holographic setting. 1.2 RELATED WORK Machine learning for inverse problems Increasing research effort has been devoted to addressing inverse problems, even beyond phase retrieval, with deep learning approaches (see Ongie et al. (2020) for a recent review). Supervised strategies can be broadly divided into four main categories: end-to-end methods, (e.g. McCann et al. (2017)), “unrolling” algorithms (e.g. Meinhardt et al. (2017)), pretrained image denoisers (e.g. Romano et al. (2017)), and learned generative models as highly informative priors (e.g. Tramel et al. (2016)). All of these approaches require a training set, containing either matched signal-observation pairs or simply typical signals, with a large number of data points. The reconstruction improves drastically when this information is available. However, it is often unrealistic to assume such prior knowledge on the measured signal. Recently, for the reconstruction of images, it was found that untrained generative neural networks with appropriate architectures can still be efficient priors (Lempitsky et al., 2018; Heckel & Hand, 2019). By adjusting their parameters to fit a single output observation, they do not require a training set, and instead encourage naturalistic images due to architecture alone. In this paper we focus on the last approach, as it is widely applicable to image data and satisfies our requirement of applicability in a realistic setting. Machine learning for phase retrieval More specifically, several variants of the phase retrieval problem have received attention in the context of machine learning for inverse problems. Com- 2 Under review as a conference paper at ICLR 2021 (a) Experimental setup. (b) The zero-padded data and its measured Fourier magnitudes. Figure 2: Holographic CDI schematic. The upper portion of the diffraction area contains the speci- men of interest X0, and the adjacent portion consists of a known “reference” R0. The recorded data Y has its low frequencies occluded by a beamstop. (Image courtesy of Saliba et al. (2012).) pressive Gaussian phase retrieval, where one observes the amplitude of random complex Gaussian projections of the signal, is a popular setting in the machine learning community. It is easier than the Fourier phase retrieval problem and often more amenable to theoretical analysis (see e.g. Aubin et al. (2020)). For this version of the problem, trained generative models such as Generative Adver- sarial Networks (Shamshad & Ahmed, 2018; Hand et al., 2018), as well as untrained priors (Jagatap & Hegde, 2019), were found to be very effective on machine learning toy datasets. An increas- ing number of works now consider the more realistic problem of Fourier phase retrieval. Using pre-trained Gaussian denoisers and iterative algorithms, Deep prior-based sparse representation (Shi et al., 2020), prDeep (Metzler et al., 2018) and Deep-ITA (Wang et al., 2020b), are solutions robust to noise in the case where the corruption is small enough to be approximately Gaussian. The end- to-end solution investigated by Uelwer et al. (2019) features some robustness to Poisson shot noise, but struggles to generalize to complicated datasets. Meanwhile, the “physics-informed” architec- ture of Goy et al. (2018), which includes information about the data generating process, is shown to perform well on realistic signals at very low photon counts, but requires a few thousand training examples. Closer to our work, Wang et al. (2020a) proposed a U-net and Bostan et al. (2020) tested the deep decoder, both untrained neural networks, for Fourier phase retrieval, but did not consider the holographic setting. To the best of our knowledge, Rivenson et al.
Details
-
File Typepdf
-
Upload Time-
-
Content LanguagesEnglish
-
Upload UserAnonymous/Not logged-in
-
File Pages28 Page
-
File Size-